Cuidados com análise de correlação
Summary
TLDRThe video script discusses the importance of distinguishing between correlation and causality in data analysis. It uses historical examples, such as the mistaken belief that radio waves caused mental illness, to illustrate the danger of assuming causal relationships from correlated data. The script emphasizes the need for careful analysis and experimental validation to determine true causality, warning against drawing conclusions from mere coincidences, as demonstrated by the humorous example of Nicolas Cage's movies correlating with swimming pool drownings.
Takeaways
- 🔍 The importance of understanding the difference between correlation and causality in data analysis.
- 🧐 Correlation between two variables in a database does not imply that one causes the other.
- 📈 Famous historical example: A strong correlation between radio ownership and mental illness rates in England was misunderstood as causal.
- 🌐 The role of external factors such as World War I, which influenced both the increase in radio ownership and the number of mental health patients.
- 🤔 The need for careful data analysis and controlled experiments to evaluate potential causality.
- 🌟 The concept of spurious correlations, where unrelated factors coincidentally show a correlation.
- 🎬 The humorous example of the correlation between the number of people drowning in swimming pools and the number of Nicolas Cage movies.
- 🎶 Another example of spurious correlation: the relationship between the price of oil and the number of rock albums released.
- ⚠️ The warning to be cautious when analyzing data to avoid drawing incorrect conclusions from coincidental correlations.
- 📊 The use of graphs, such as scatter plots, to illustrate correlations and the potential for misinterpretation.
- 🔑 The key takeaway of the script is the critical evaluation of data relationships and the necessity of experimental validation for causality.
Q & A
What is the main misconception about correlation discussed in the script?
-The main misconception discussed is the assumption that just because two variables are correlated, one must cause the other. This is not necessarily true, as correlation does not imply causation.
What is the historical example used in the script to illustrate the difference between correlation and causality?
-The historical example used is the correlation between the number of radios and the number of mental patients per 100,000 inhabitants in England between 1910 and 1920. The misconception was that radios caused mental illness, but the actual cause was the World War I, which increased both the production of radios and the number of mental patients.
How does the script suggest we should approach data analysis to avoid the correlation-causation fallacy?
-The script suggests that we should always be cautious when analyzing data, perform controlled experiments to evaluate whether a correlation actually implies causation, and not jump to conclusions based solely on observed correlations.
What is the term used in the script to describe absurd correlations found in some data?
-The term used is 'spurious correlations', which refers to correlations that are coincidental and do not have a causal relationship.
How does the script use the example of Nicolas Cage movies to illustrate spurious correlations?
-The script mentions a spurious correlation between the number of people who died from drowning in swimming pools and the number of Nicolas Cage movies. It humorously suggests that Nicolas Cage's presence in movies causes more people to drown, which is, of course, not true.
What is the importance of controlled experiments in data analysis according to the script?
-Controlled experiments are important because they help to determine whether there is actual causation behind observed correlations. They allow analysts to test hypotheses and rule out coincidental relationships.
What is the role of the 'correlation does not imply causation' principle in the field of statistics?
-This principle is crucial in statistics as it serves as a reminder to analysts to not infer causation from mere correlation. It helps prevent the drawing of incorrect conclusions from data analysis.
What is the relevance of the script's discussion on the misuse of correlation in historical context?
-The historical context serves as a cautionary tale about the dangers of misinterpreting data. It emphasizes the need for careful analysis and understanding of the factors that might influence correlations to avoid drawing false conclusions.
How does the script suggest we should interpret correlations found in large databases?
-The script suggests that we should be skeptical of correlations found in large databases and investigate further to determine if they are the result of coincidental relationships or if they indicate a true causal relationship.
What is the significance of the script's mention of a third event causing two correlated phenomena?
-The mention of a third event causing two correlated phenomena highlights the possibility of confounding variables. It underscores the importance of considering all potential factors that could lead to observed correlations and not just the apparent relationship between two variables.
What advice does the script give for concluding an analysis phase?
-The script advises that the most important aspect of concluding an analysis phase is to develop changes and insights from the findings. It emphasizes the need to revisit and re-evaluate the data and analysis to ensure accurate and meaningful conclusions.
Outlines
🔍 Understanding Correlation and Causality
The paragraph discusses the importance of distinguishing between correlation and causality when analyzing data. It uses the historical example of a statistician who found a strong correlation between the number of radio stations and the number of mental health patients in England between 1910 and 1920. However, this correlation was not due to radio stations causing mental health issues, but rather both were influenced by World War I. The paragraph emphasizes the need for careful data analysis and experimental validation to determine if observed relationships imply causality or are mere coincidences. It also mentions a website known for highlighting spurious correlations, such as the number of people dying in swimming pools correlating with the number of Nicolas Cage movies, illustrating the importance of not jumping to conclusions based on correlations alone.
Mindmap
Keywords
💡Correlation
💡Causality
💡Data Analysis
💡Spurious Correlations
💡World War I
💡Statistical Significance
💡Confounding Factors
💡Experiments
💡Rock Music
💡Mental Illness
💡Nicolas Cage
Highlights
Discussing the power of correlation technique and its proper use.
The importance of distinguishing between correlation and causality in data analysis.
The historical example of a strong correlation between radio ownership and mental illness rates in England from 1910 to 1920.
The misconception that radio waves cause mental illness due to a correlation.
The role of World War I in increasing both radio production and the number of mental health patients.
The need for careful data analysis and controlled experiments to assess causality.
The concept of spurious correlations and the famous statistician who debunked the radio and mental illness myth.
The example of the correlation between the number of people who died from drowning in swimming pools and the number of Nicolas Cage movies.
The absurdity of attributing causality to coincidental correlations.
The website that showcases absurd correlations to illustrate the concept of spurious relationships.
The correlation between the price of oil and the number of rock albums released in the 1970s.
The cautionary tale of mistaking correlation for causation in data analysis.
The importance of reevaluating and refining analysis methods based on insights gained from previous phases.
The transition from the analysis phase to the implementation phase.
The value of developing changes and improvements based on the analysis of cheese examples.
The significance of understanding the limitations and potential errors in data analysis techniques.
The reminder to always question and verify the relationships found in data to ensure accurate conclusions.
Transcripts
e a pessoa então agora que a gente já
viu aí essa tudo poder da técnica de
correlação né gente é discutir um pouco
isso eu queria terminar essa parte e
terminar também a nossa fase Dona lá e
com um pequena é
um pequeno aviso na em alguns cuidados
que a gente tem que ter quando a gente
usa gráfico dispersão é análise de
correlação em Minas Gerais tá a primeira
coisa que a gente tem que entender é
sempre com relação né o a relação entre
correlação e causalidade não é porque
dois dados vão estar
correlacionadas no banco de dados nas
duas variáveis estão correlacionadas em
um banco de dados que uma causa a outra
na TV tudo histórico que ficou muito
famoso que era esse daqui dos Rádios não
é feito pelo piercing famoso estatístico
né ele pegou coletou lavar os dados e
uma população é entre os anos de 1910 e
1920 por ele viu lá na que tinha uma
correlação muito forte entre o no é de
rádio e milhões que tinha naquela região
na Inglaterra e a o número de doentes
mentais por 100 mil habitantes traz aqui
já está normalizado população acresceu
que a população cresceu não né ele viu
que nossa tem uma correlação muito forte
entre quanto mais rádios mais a doentes
mentais Tinho podia surgir aquela
questão Nossa olhando esse gráfico os
raios causam loucuras né então nossas
ondas de rádio maligna passam pela
cabeça das pessoas e elas enlouquecem né
era uma discussão por ele tá acontecendo
na época a gente não tinha ainda noção
de que o rádio aí no sensível é então a
gente pode ser isso aqui mas não né no
caso a elas apesar de estarem com
relacionadas elas não têm causalidades
né porque elas estão correlacionados
pode até pensar preparar é parar para
pensar sobre isso né nós que entre 1910
1920 poucos teve lá um grande evento é
que foi a Guerra Mundial e aumentou
substancialmente o número de rádios
produzidos Então pode estão produzindo
rádio lá para o esforço de guerra Ei
também a guerra aumentou o número de
doentes mentais Então apesar de rádio e
do enfrentar estarem com relação às um
não causa outro os dois são causados
pela guerra né esse é o evento que da
liga nos dois a pessoa aqui não tá
ficando louca porque tá passando onda de
rádio na cabeça dela mas a ficando louco
porque ela tá numa de encher mas foi
tirada na casa dela esperam a trincheira
com outra cara deu tiro em cima dele
jogando bomba 24 horas por dia tentando
Natal né esse daí que enlouqueceu e o
raio também verde para subir águia né
então a correlação e causalidade nem
sempre estão andam juntos a gente tem
que sempre tomar muito cuidado com as
nossas análises de dados e fazer
experimentos comprobatórios né pra
avaliar
e se de fato a minha com relação à la
implica numa causalidade ou não e para
colocar essas correlações né até mais é
ilustrativas aí tem até um site né que
ficou conhecido aí que é o site das
correlações espúrias né é que traz
vários dados que se correlacionam bem
Absurdos como por exemplo a o número de
pessoas que morreram Afogados na piscina
com o número de filmes com Nicolas Cage
aparece então tem a Anna a Anna nós que
toda vez que o Nicolas Cage aparece né
que aqui no caso da linha preta morre
mais gente afogada na piscina né tá aqui
uma prova Cabal de que o Nicolas Cage
vendeu a alma para o capeta para ter
sucesso e daí quando está no filme o
capeta venha não tem nada a ver né É só
uma coincidência né Às vezes tem até
porque a gente tem uma palavra para
descrever os tem outras correlações uma
que eu gosto muito é sobre o preço do
petróleo número de CDs de rock lançado
né então na década de 70 pô Ele
acreditou eu penso continuasse álbum de
rock bom para cadernos Stones Beatles
Led Zeppelin todo mundo começando lá né
Não também tem nada a ver só uma
coincidência Tudo bem então a gente tem
essas correlações Então a gente tem que
tomar muito cuidado é para analisar se
as nossas relações são de fato relações
causais ou seleção apenas coincidências
do banco de dados ou dois eventos
causados por um terceiro Beleza a gente
tem que sempre somente com essa gente
encerra a fase de análise né é de novo
Lembrando que o mais importante dessa
fase é você desenvolver as mudanças e
como a gente viu lá nos Queijos nos
exemplos é essas ferramentas eles são
bem outras bem interessante traz na
próxima fase Então a gente vai começar a
falar dor implo até mais
Browse More Related Video
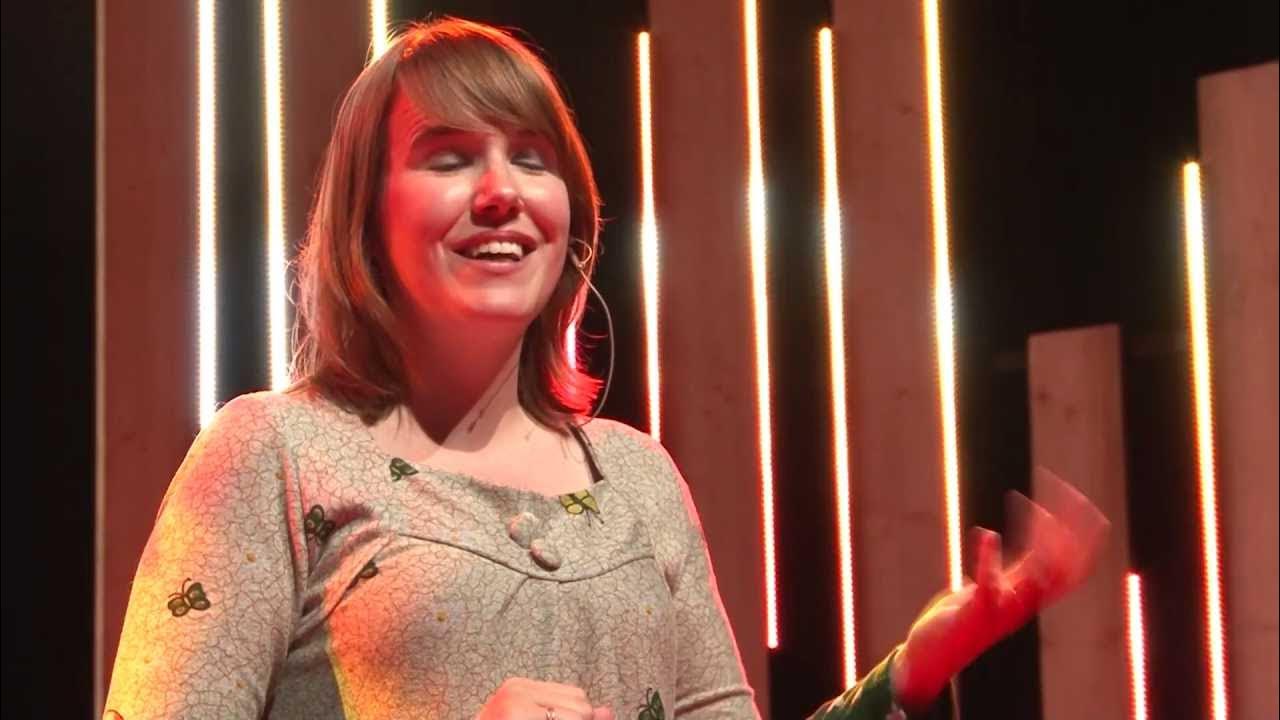
The danger of mixing up causality and correlation: Ionica Smeets at TEDxDelft
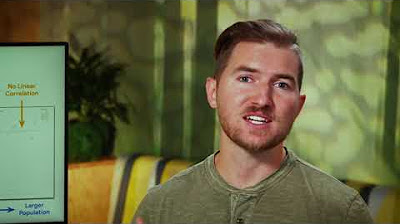
Lesson 32 Good example of a data presentation
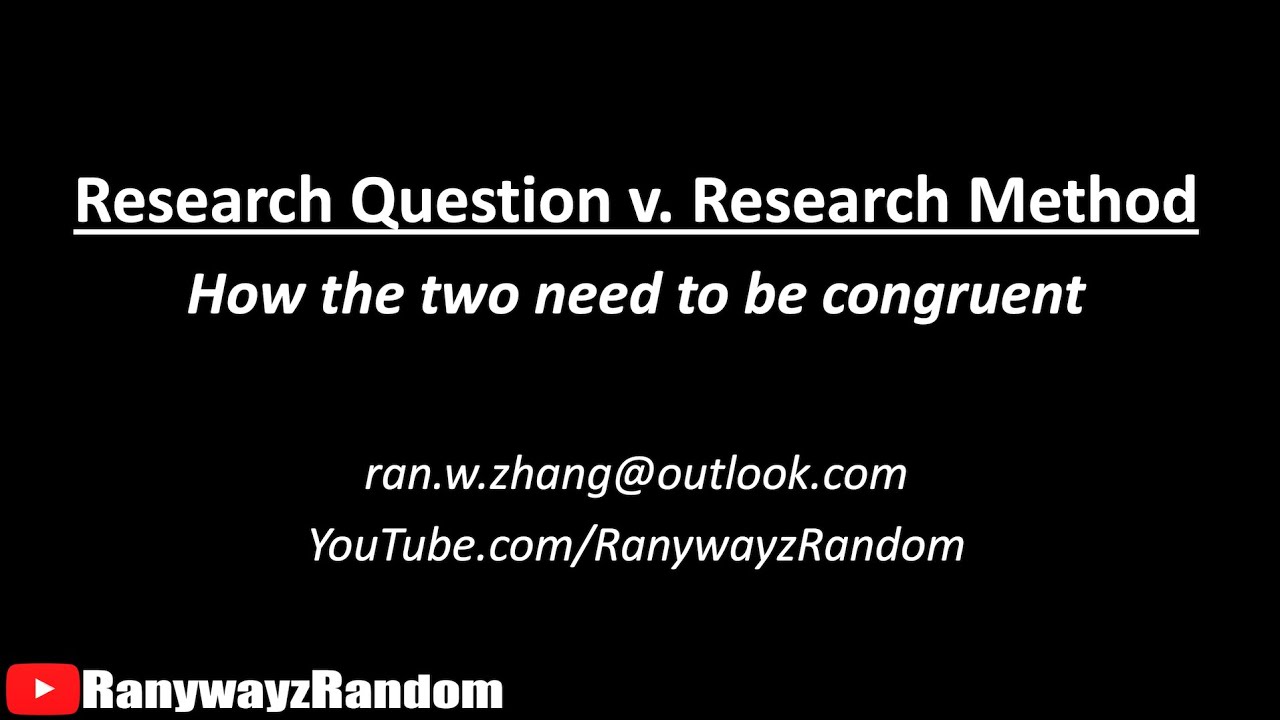
Examples of Causal, Correlational, Descriptive, and Exploratory Research Questions
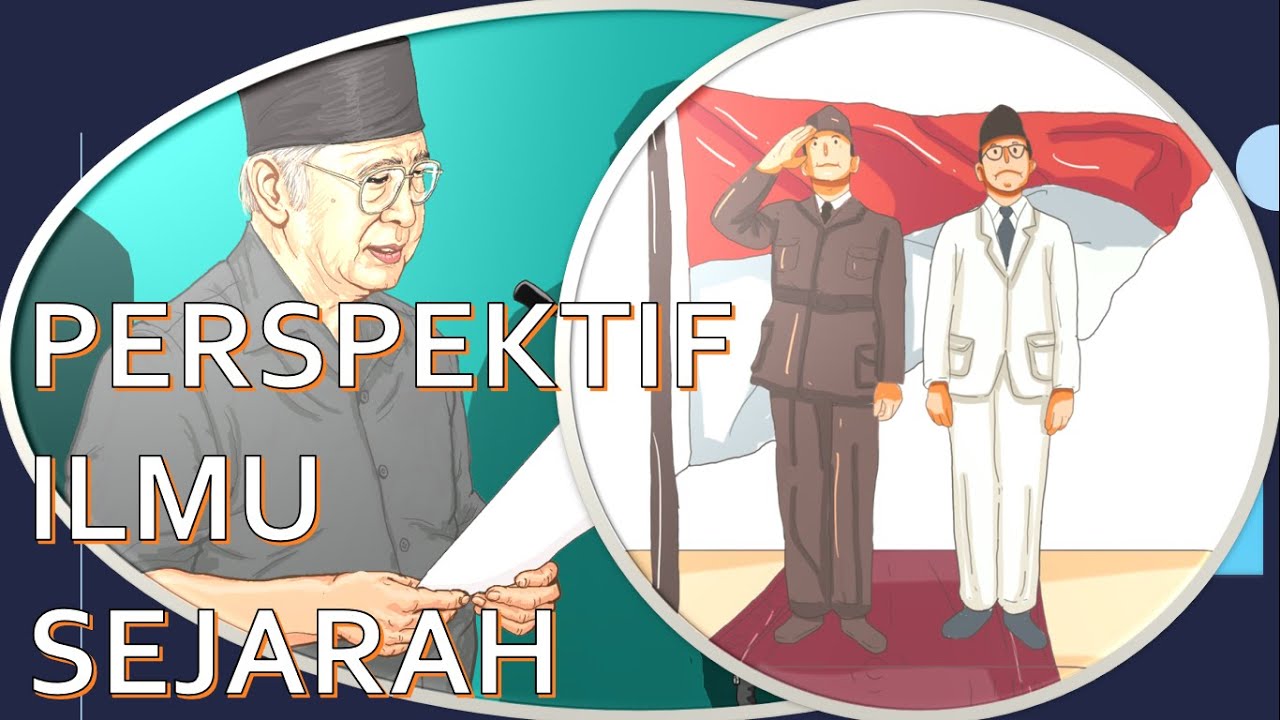
SINKRONIK DAN DIAKRONIK DALAM ILMU SEJARAH || SEJARAH INDONESIA KELAS 10 (SEPULUH) #2
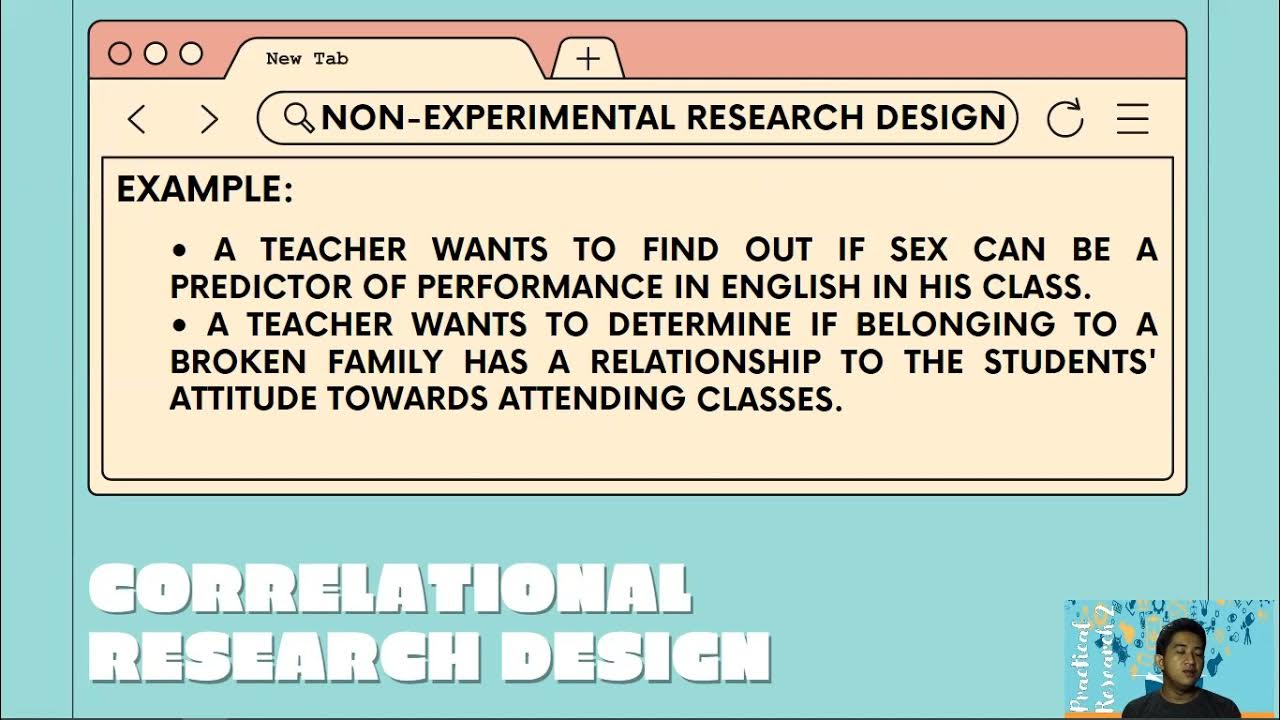
Quantitative Research Design
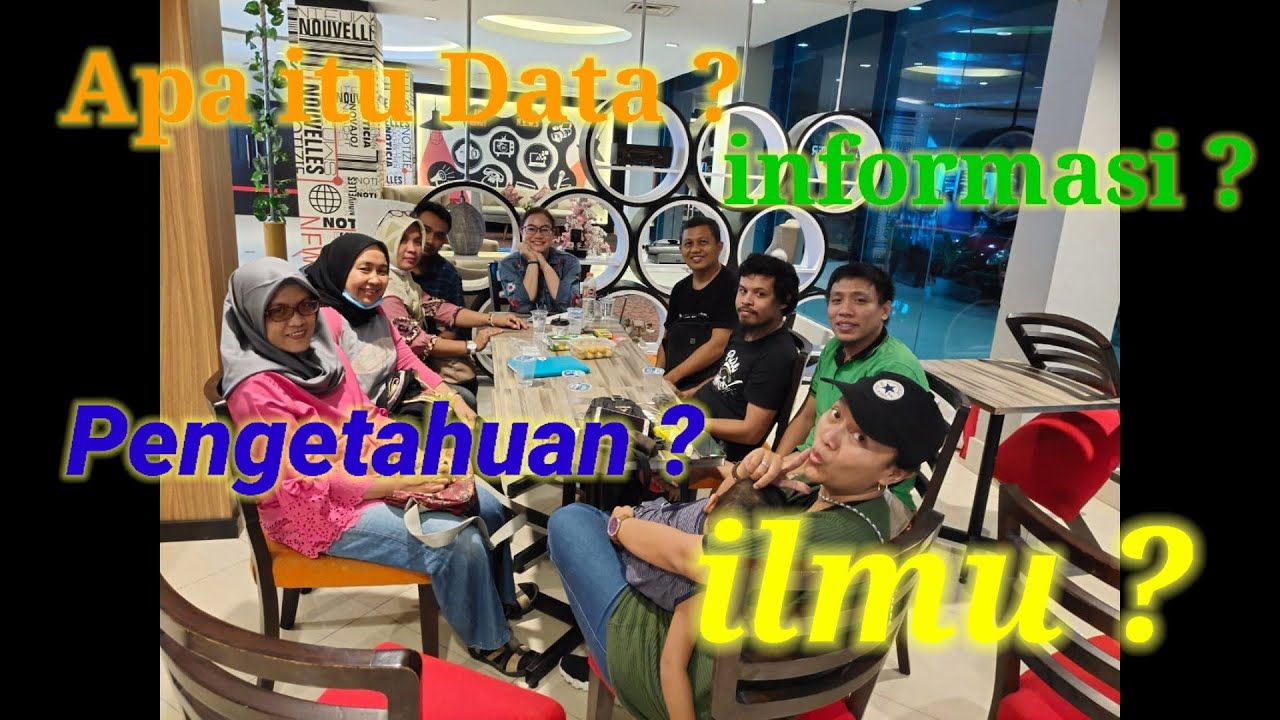
Apa itu Data, Informasi, Pengetahuan dan Ilmu ?
5.0 / 5 (0 votes)