What haunts statisticians at night
Summary
TLDRThis video delves into the nuances of causal inference in statistics, highlighting the common misconception that correlation implies causation. It explains the difference between correlation and causation, using the Pearson correlation coefficient as an example, and introduces the counterfactual framework to understand causality. The video also discusses the challenges posed by confounders in statistical analysis and the importance of controlling for them to isolate the true relationship between variables. Sponsored by Brilliant, the video encourages viewers to deepen their understanding of these concepts through interactive learning.
Takeaways
- 😀 Correlation does not imply causation, a principle reminding us that two related events may not have a cause-and-effect relationship.
- 🔍 Causal inference is a statistical subfield dedicated to distinguishing when correlation equals causation and under what conditions.
- 📚 The concept of 'correlation does not equal causation' is a fundamental idea in statistics, important for industries like pharmaceuticals and tech.
- 📈 Pearson's correlation coefficient, denoted by the Greek letter rho (ρ), measures the linear association between two random variables.
- 📊 Correlation indicates a tendency for variables to vary together but does not suggest one causes the other, unlike causation which does.
- 🤔 Causation is complex in statistics due to the presence of randomness and requires a framework like the counterfactual framework to understand.
- 📝 Counterfactuals are hypothetical scenarios that contrast what actually happened, used to estimate the causal effect of a variable.
- 🔧 Controlling for confounders in statistical models is crucial for isolating the relationship between an exposure and an outcome.
- 🔄 Confounders, if not accounted for, can create false associations or distort the true relationship between variables.
- 🧩 Directed acyclic graphs (DAGs) are used to visualize statistical relationships and understand the flow of influence between variables.
- 🚫 The problem of unobserved confounders is a persistent challenge in statistical analysis, as it's impossible to account for variables that are unknown or unmeasured.
Q & A
What is the main topic discussed in the video?
-The main topic discussed in the video is the concept of correlation versus causation in statistics, with a focus on causal inference and the challenges statisticians face in distinguishing between the two.
Outlines
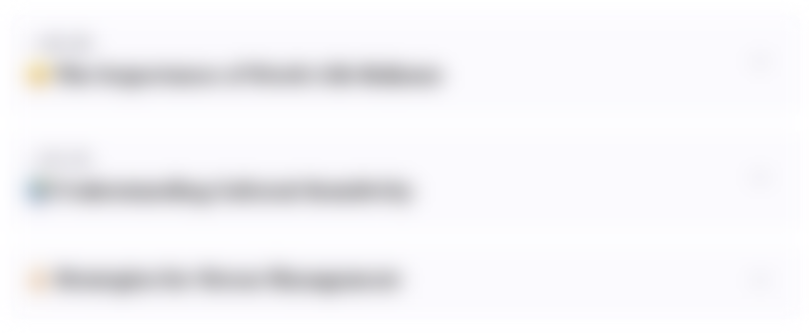
This section is available to paid users only. Please upgrade to access this part.
Upgrade NowMindmap
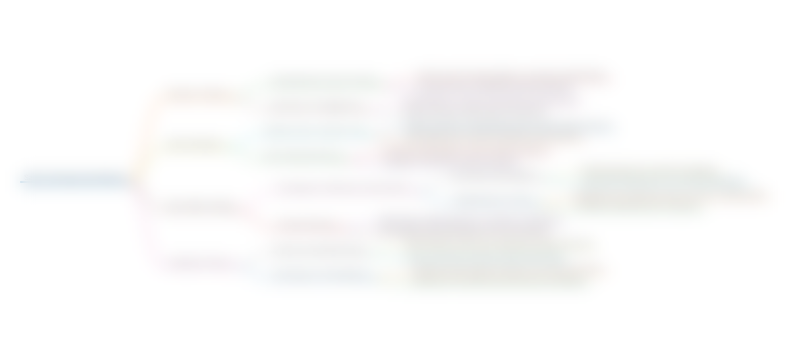
This section is available to paid users only. Please upgrade to access this part.
Upgrade NowKeywords
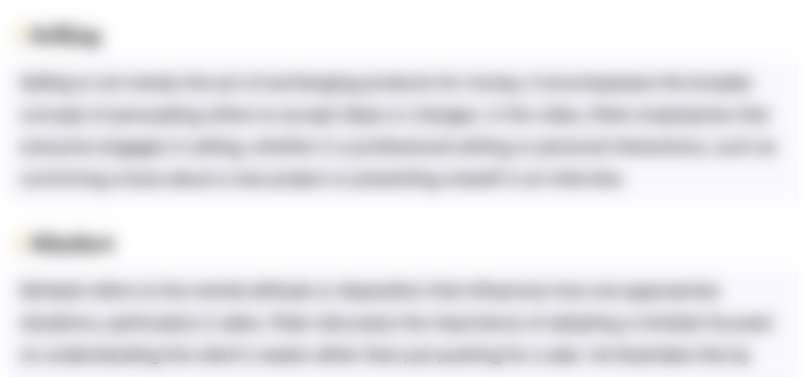
This section is available to paid users only. Please upgrade to access this part.
Upgrade NowHighlights
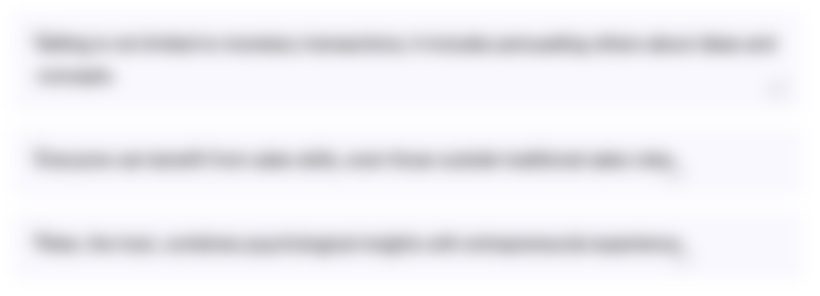
This section is available to paid users only. Please upgrade to access this part.
Upgrade NowTranscripts
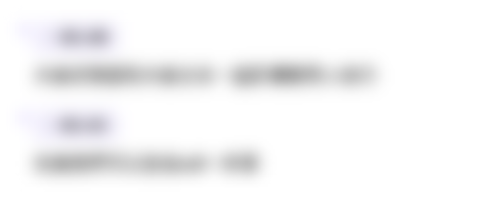
This section is available to paid users only. Please upgrade to access this part.
Upgrade NowBrowse More Related Video

What is Correlational Research? Finding Relationships Between Variables
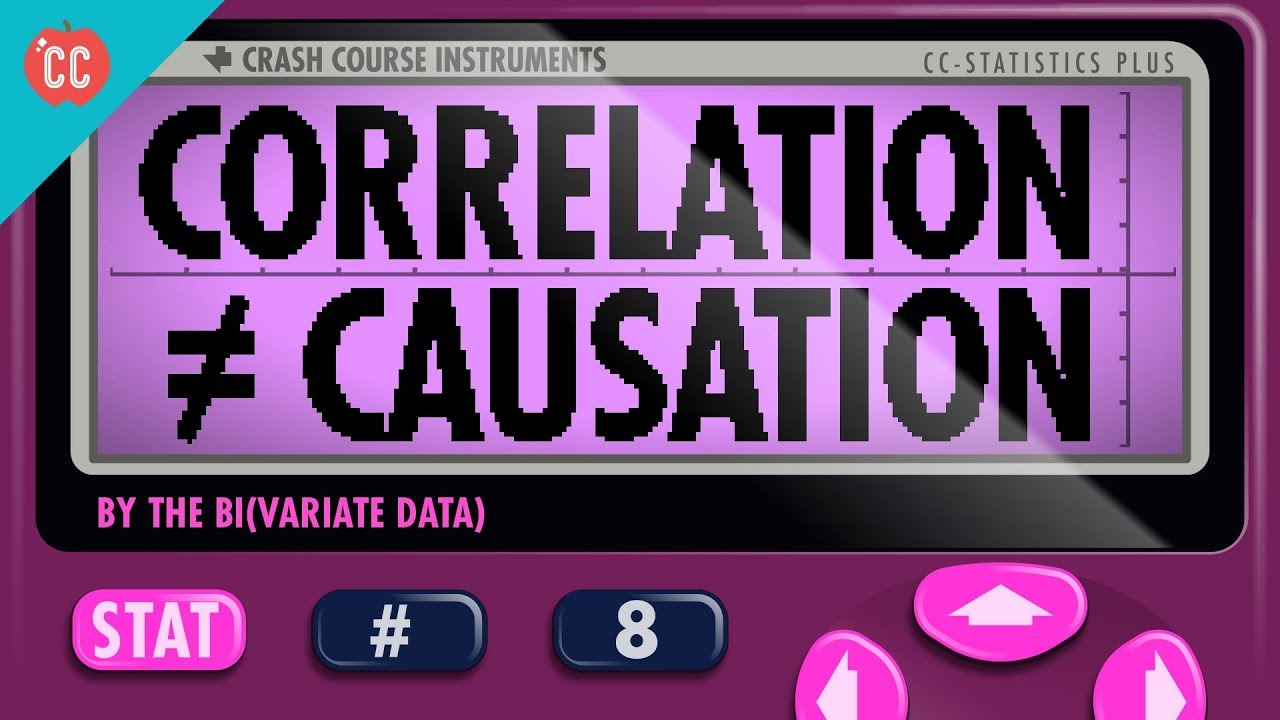
Correlation Doesn't Equal Causation: Crash Course Statistics #8

The most important ideas in modern statistics
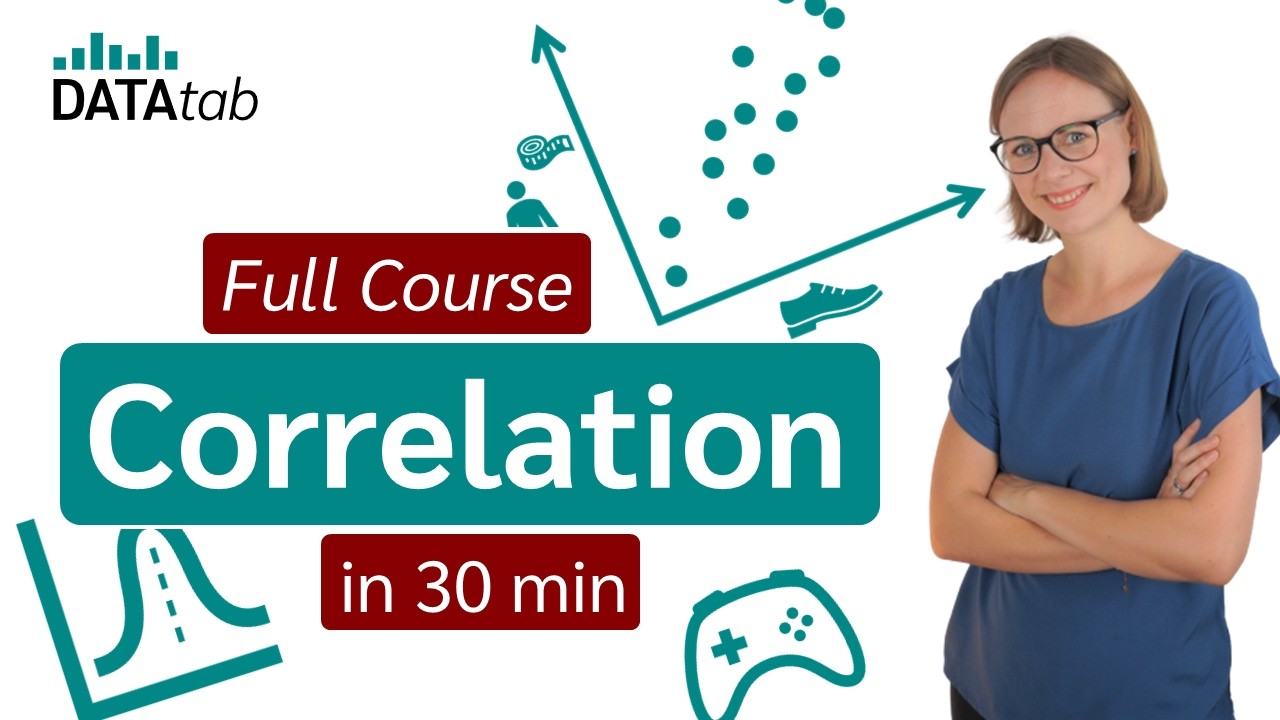
Correlation Analysis - Full Course in 30 min
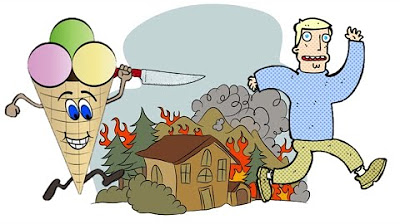
How Ice Cream Kills! Correlation vs. Causation
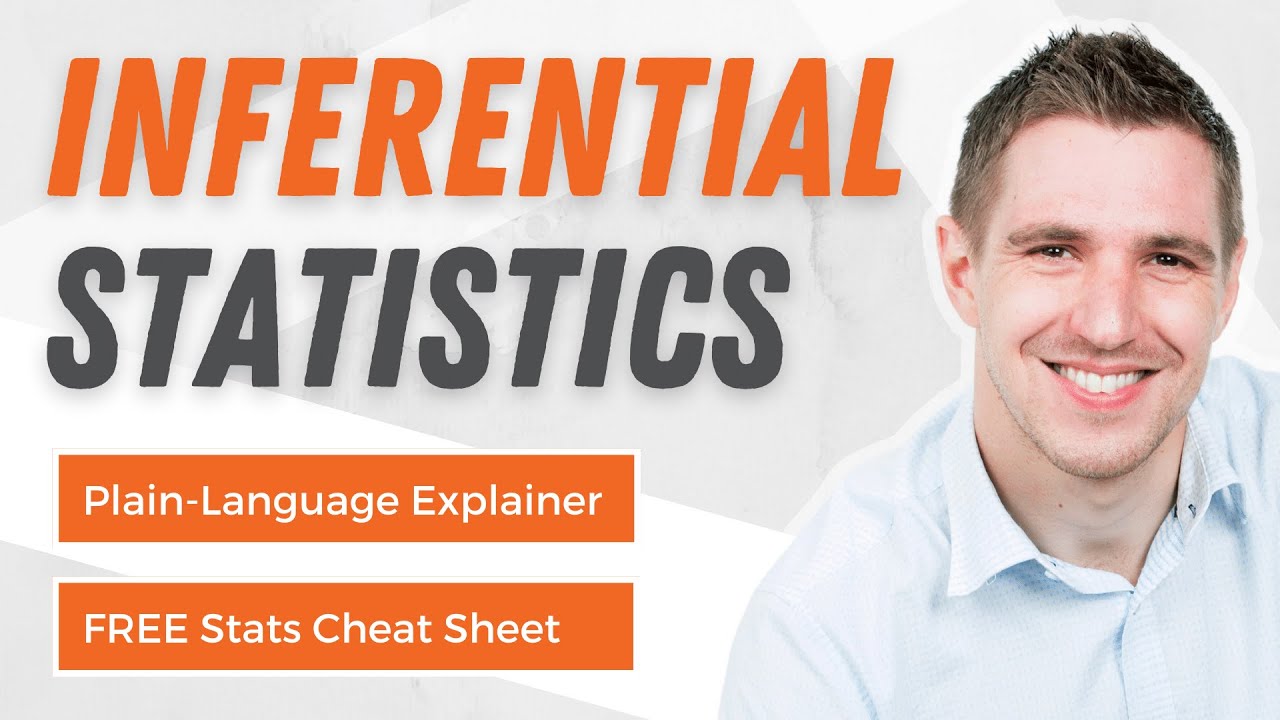
Inferential Statistics FULL Tutorial: T-Test, ANOVA, Chi-Square, Correlation & Regression Analysis
5.0 / 5 (0 votes)