Stanford CS224W: ML with Graphs | 2021 | Lecture 5.2 - Relational and Iterative Classification
Summary
TLDRThis lecture introduces two collective classification approaches: relational classification and iterative classification. Relational classification updates class probabilities based on neighbors' labels, using network structure without node features. Iterative classification enhances this by incorporating node attributes and predicted labels of neighbors through two trained classifiers, Phi_1 and Phi_2, iterating until convergence for accurate label prediction.
Takeaways
- ๐ The lecture introduces two classification methods: relational classification and iterative classification.
- ๐ค Relational classification assumes that the class probability of a node is a weighted average influenced by its neighbors' class probabilities.
- ๐ In iterative classification, nodes update their beliefs about their own label based on the labels of neighboring nodes until convergence.
- ๐ท๏ธ Initially, labeled nodes are fixed to their ground-truth labels, while unlabeled nodes start with a uniform belief (e.g., 0.5 for a binary classification).
- ๐ The update rule for a node's belief involves summing the weights of the edges connecting to neighbor nodes, multiplied by the probability of those neighbors belonging to the same class.
- ๐ The method relies solely on network structure and node labels, not on node attributes or features.
- ๐ Iterative classification improves upon relational classification by incorporating node features and neighbor labels into the classification process.
- ๐ ๏ธ Two classifiers are trained: one based on node features alone (Phi_1) and another that also considers the labels of neighboring nodes (Phi_2).
- ๐ข The summary vector 'z' captures the distribution of labels among a node's neighbors, which can be in the form of a histogram or other statistics.
- ๐ The iterative process involves applying Phi_1 to predict labels, creating summary vectors 'z', applying Phi_2 to refine predictions, and repeating until convergence or a maximum number of iterations is reached.
- ๐ฎ The goal is to predict node labels more accurately by considering both the node's features and the network context provided by neighboring nodes.
Q & A
What is relational classification?
-Relational classification is a method where the class probability of a node is determined as a weighted average of the class probabilities of its neighbors. It uses the network structure and node labels but does not use node attributes.
How does iterative classification differ from relational classification?
-Iterative classification improves upon relational classification by also considering the attribute or feature information of nodes. It classifies nodes based on both their features and the labels of their neighboring nodes.
What role do node labels play in relational classification?
-In relational classification, labeled nodes fix their class labels to the ground-truth labels, while unlabeled nodes initialize their beliefs uniformly. These beliefs are then updated iteratively based on the labels of neighboring nodes until convergence.
Can you explain the concept of belief propagation as mentioned in the script?
-Belief propagation, in this context, refers to the process where nodes update their beliefs or probabilities about their own class labels based on the class probabilities of their neighboring nodes in the network.
What is the importance of the weighted average in relational classification?
-The weighted average in relational classification is important because it allows nodes to update their beliefs about their class labels based on the influence of their neighbors, with the weights representing the strength of the connections.
How is the update formula for class probabilities used in relational classification?
-The update formula is used to calculate the probability of a node belonging to a certain class by averaging the probabilities of its neighbors belonging to the same class, weighted by the edge weights.
What are the limitations of the relational classification model described in the script?
-The relational classification model described does not use node feature information and its convergence is not guaranteed. It solely relies on node labels and network structure.
How does the iterative classification approach utilize node features?
-Iterative classification utilizes node features by training a base classifier that predicts node labels based on feature vectors alone, and then refining these predictions with a second classifier that also considers the labels of neighboring nodes.
Can you describe the process of creating a label summary vector z in iterative classification?
-The label summary vector z in iterative classification is created by summarizing the labels of a node's neighbors, which can be done in various ways such as counting the number or fraction of each label in the neighborhood.
What is the purpose of training two classifiers in the iterative classification approach?
-The purpose of training two classifiers in iterative classification is to first predict node labels based solely on features, and then refine these predictions using a second classifier that also considers the labels of neighboring nodes.
How does the iterative classification approach ensure convergence?
-The iterative classification approach ensures convergence by repeating the process of updating node labels with classifier Phi 2 and updating the label summary vector z until no more changes occur or a maximum number of iterations is reached.
Outlines
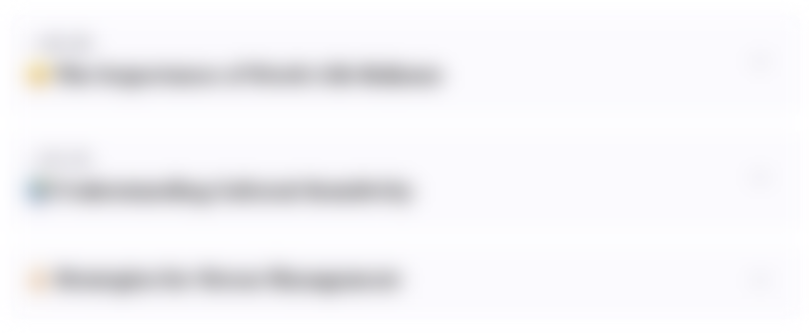
This section is available to paid users only. Please upgrade to access this part.
Upgrade NowMindmap
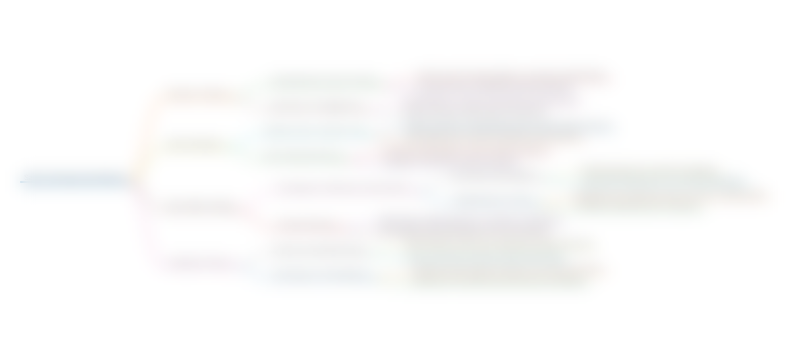
This section is available to paid users only. Please upgrade to access this part.
Upgrade NowKeywords
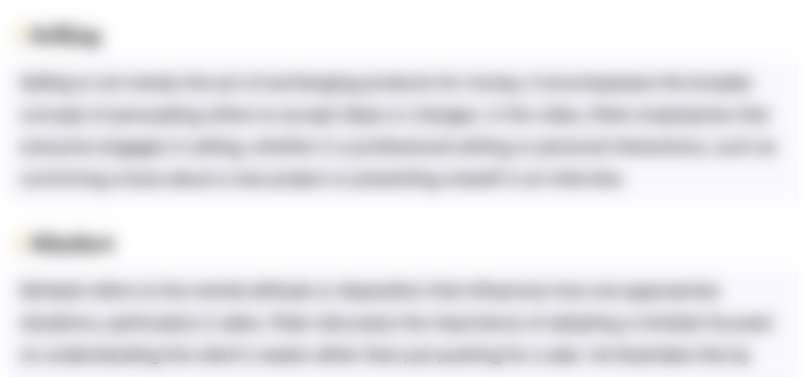
This section is available to paid users only. Please upgrade to access this part.
Upgrade NowHighlights
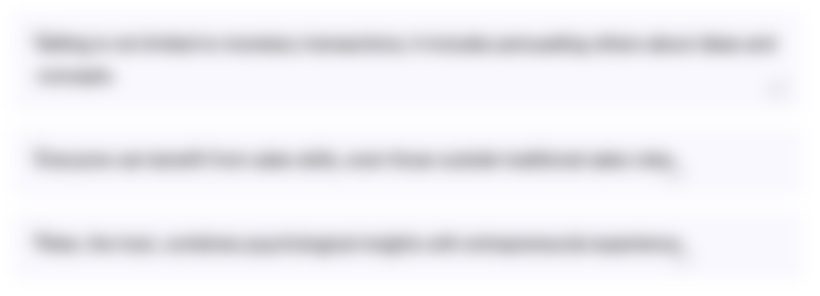
This section is available to paid users only. Please upgrade to access this part.
Upgrade NowTranscripts
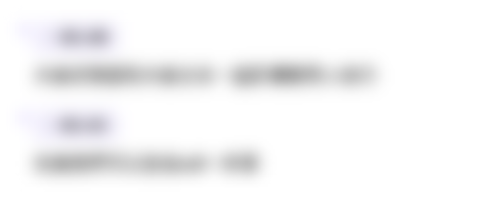
This section is available to paid users only. Please upgrade to access this part.
Upgrade NowBrowse More Related Video
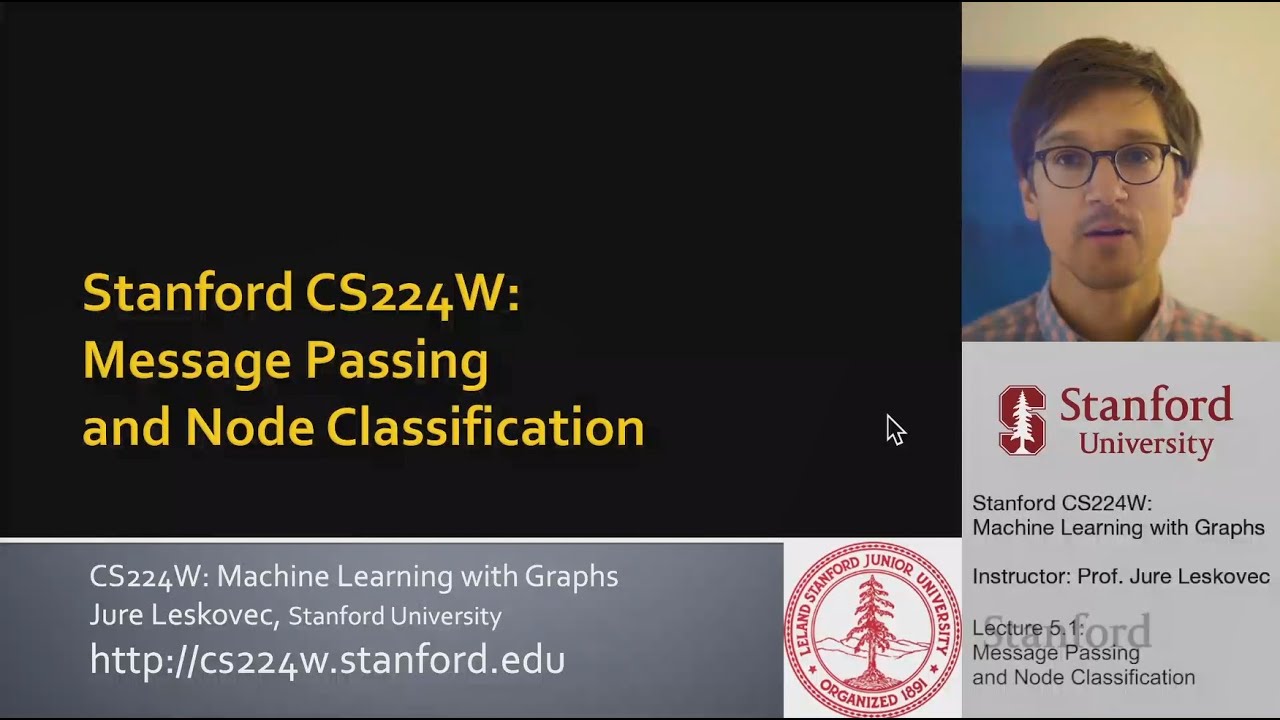
Stanford CS224W: ML with Graphs | 2021 | Lecture 5.1 - Message passing and Node Classification

C_13 Operators in C - Part 1 | Unary , Binary and Ternary Operators in C | C programming Tutorials
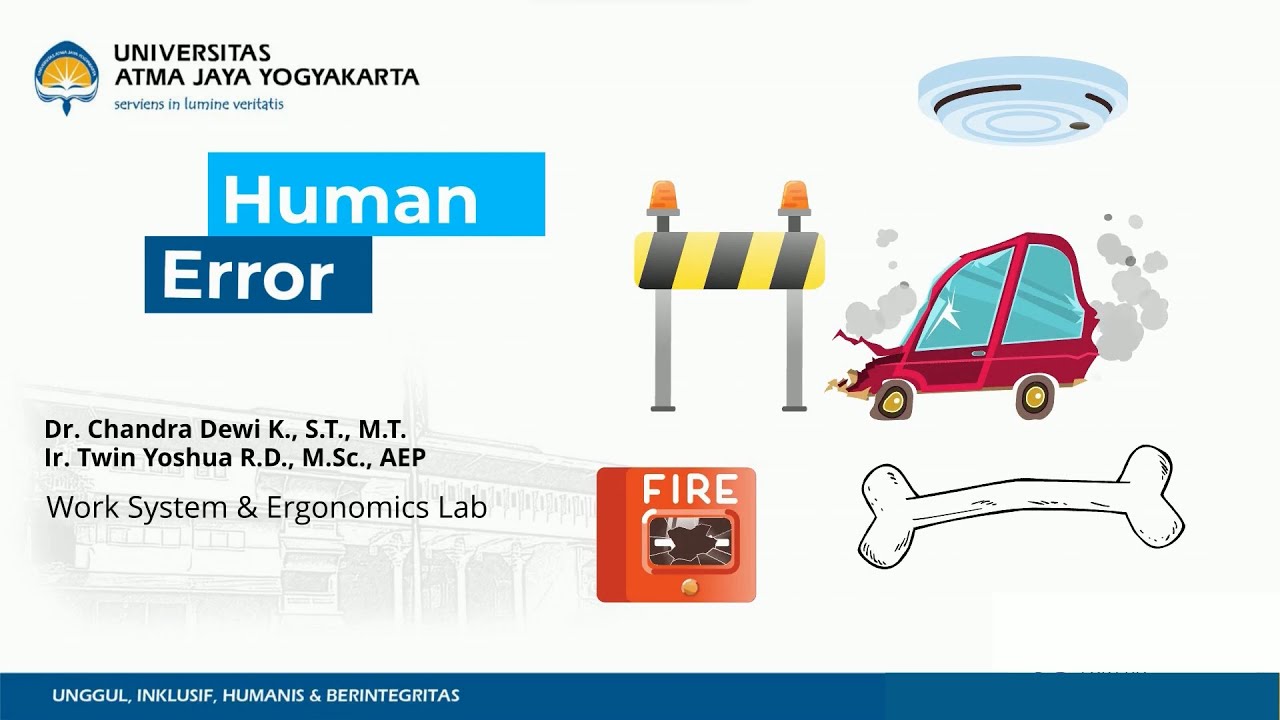
Human Error
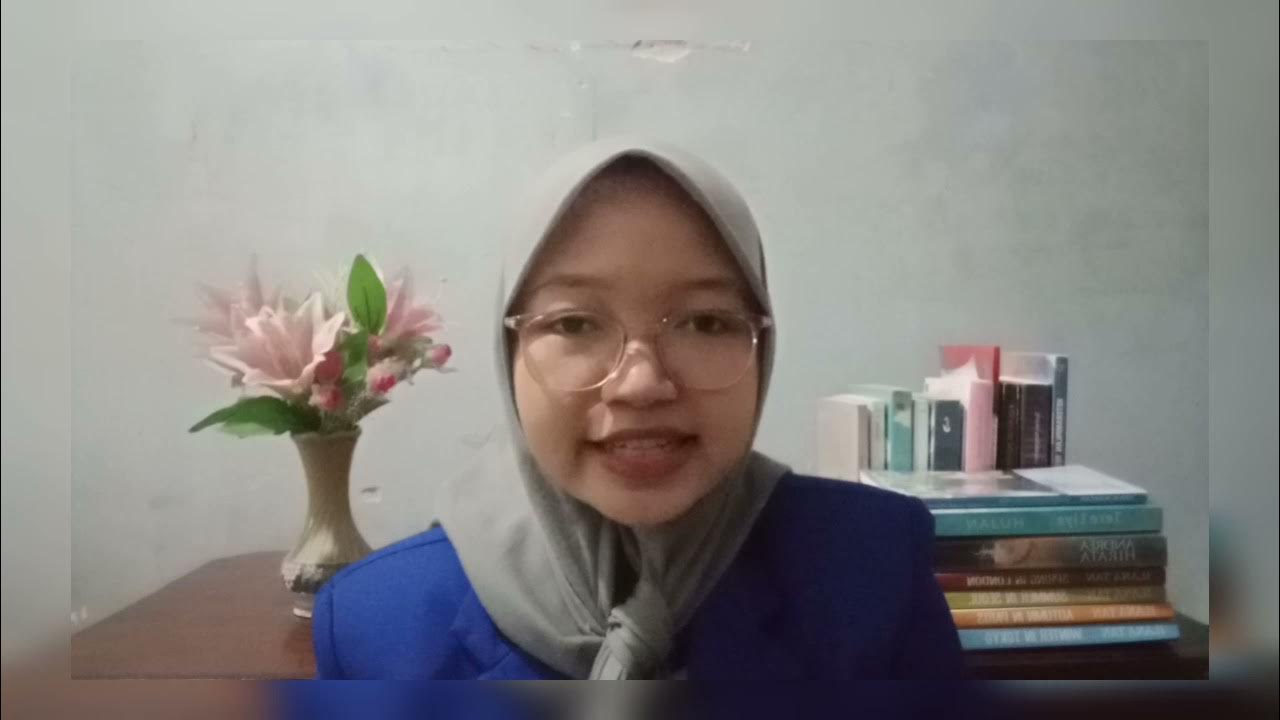
Mengetahui Sistem Klasifikasi Perpustakaan | oleh : Lucky Audrylya Mahatan
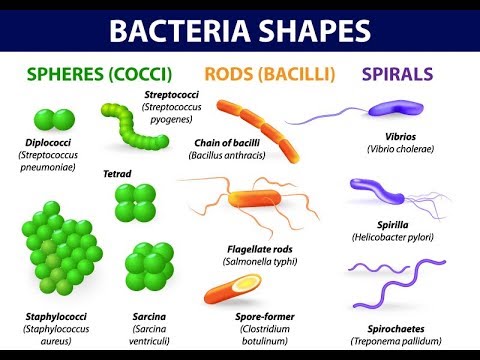
Microbiology of Bacterial Morphology & Shape
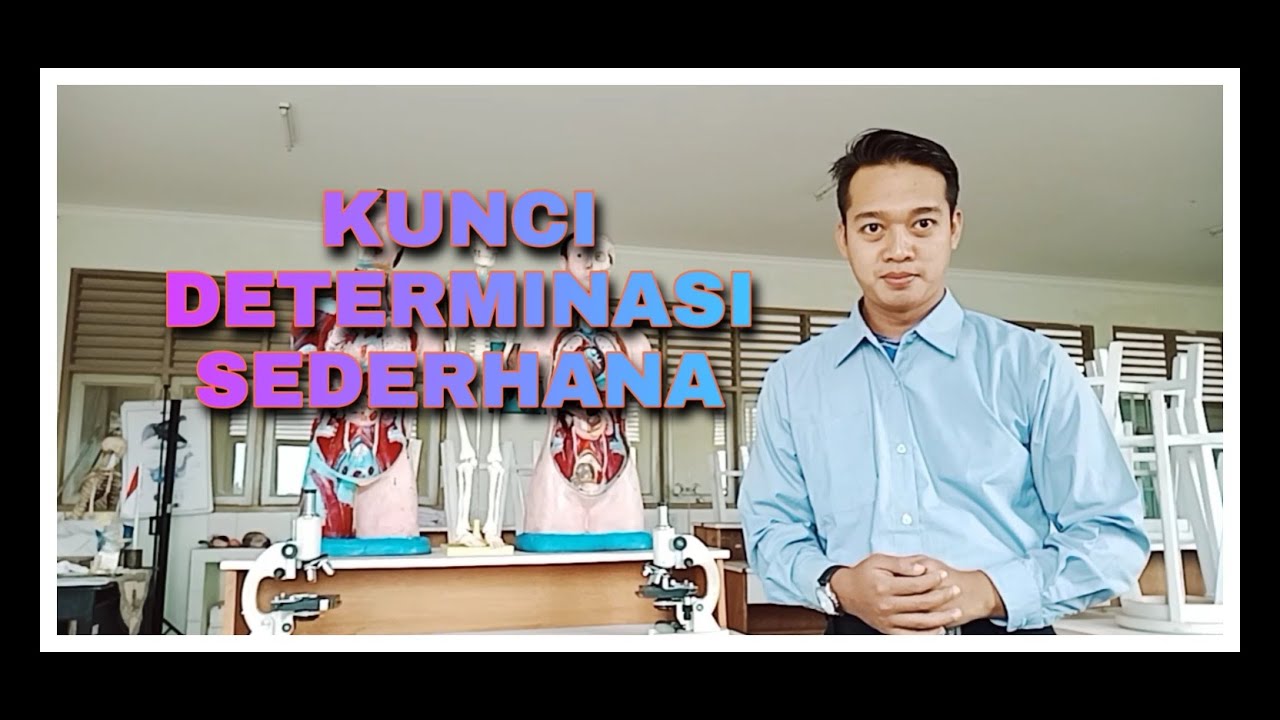
PRAKTIKUM KUNCI DETERMINASI SEDERHANA
5.0 / 5 (0 votes)