Webinar: How to Be an AI Product Manager by Facebook AI Product Leader, Natalia Burina
Summary
TLDR本次演讲由Facebook的AI产品经理Natalia Barina主讲,她分享了AI产品管理的重要性和AI产品经理的角色。她强调AI在当今社会中无所不在,对决策产生深远影响。AI产品经理需要理解业务问题,制定战略和路线图,并确保AI产品的公平性、隐私性、透明度和可靠性。她还讨论了AI产品管理与传统软件产品管理的不同之处,包括处理AI的不确定性和建立实验文化。最后,她给出了成功作为AI产品经理的三个建议:讲好故事、制定清晰的计划和快速上手新工作。
Takeaways
- 🤖 AI产品管理的重要性日益凸显,因为AI正在以前所未有的规模做出关键决策。
- 🚀 AI产品是自动系统,通过从数据中学习来做出面向用户决策,机器学习使计算机系统通过现有数据训练获得学习能力。
- 🛠️ AI产品经理的角色是为公司最重要的商业问题构想和实现有影响力的AI解决方案。
- 🎯 识别AI可以解决的正确问题和优先级是AI产品经理的关键任务。
- 🔍 AI产品经理的类型包括专注于产品的、平台的、研究的以及专注于构建负责任AI的产品经理。
- 🌟 AI软件开发与传统软件开发最大的不同在于其概率性,机器学习将工程从确定性过程转变为概率性过程。
- 📈 AI产品经理需要掌握的实用技能包括使用创新工具、理解AI产品管道、以及关注业务目标和技术潜力。
- 🔧 构建负责任的AI意味着确保AI的公平性、隐私保护、可解释性、可追责性和可靠性。
- 📊 成功作为AI产品经理需要深入理解问题、内部化业务目标、对齐和跟踪正确的指标,并培养实验文化。
- 🚦 面对AI技术的强大力量,产品经理必须意识到伴随的责任,并始终以不伤害用户和客户为出发点。
- 🌐 作为AI产品经理,应与所有利益相关者合作,特别是领导层,以设计和对齐适当的指标。
Q & A
AI产品经理的主要职责是什么?
-AI产品经理的主要职责是设想和实现对公司有重大影响的AI解决方案,解决最重要的商业问题。他们需要首先理解AI能解决的最重要的商业问题,其次识别和优先处理这些问题,并制定愿景、策略和路线图。最后,他们需要确保这些解决方案得以实现。
AI产品与传统软件产品在产品管理上有何不同?
-AI产品的开发是概率性的,与传统的确定性过程不同。机器学习将工程从硬编码的算法和规则转变为基于大量输入输出对的统计学习过程。这意味着AI产品经理需要适应这种不确定性,并理解模型预测可能会有一定的错误率。
AI产品经理需要具备哪些技能?
-AI产品经理需要具备包括使用设计思维工具(如UX模型、线框图、用户调查等)在内的多种技能。他们需要理解AI产品管道,包括数据、模型、服务基础设施和实验等环节。此外,他们还需要理解机器学习可以解决的问题类型,如排名、推荐、分类、回归、聚类和异常检测等。
如何确保AI产品的公平性和无偏见?
-为确保AI产品的公平性和无偏见,产品经理需要设计和优化AI以避免内置的偏见。这意味着要确保AI的决策基于伦理和包容性原则,同时要意识到数据可能编码了人类和社会的偏见,并采取措施来减轻这些偏见的影响。
AI产品经理如何处理AI产品的隐私和安全性问题?
-AI产品经理需要在构建AI时保护用户隐私,并确保系统能够抵御攻击。他们需要考虑AI可能被欺骗的方式,并采取措施来增强系统的鲁棒性,以防止通过操纵输入数据来破坏算法。
AI产品经理如何确保AI产品的透明度和可解释性?
-AI产品经理应确保AI的决策过程是透明的,并且能够解释AI为何做出特定的决策。这对于高风险的使用案例尤其重要,因为它们可能会直接影响人们的生活。透明度可以通过提供决策背后的逻辑和数据支持来实现。
AI产品经理如何确保AI产品的问责性?
-AI产品经理需要建立检查和平衡机制,进行验证和合规性审查,确保有人类监督。这意味着AI决策过程不仅要自动化,还要能够在必要时进行人工审查和干预。
AI产品经理如何确保AI产品的可靠性和持续高性能?
-AI产品经理需要持续监控AI系统的性能,并及时检测和解决数据问题,以防止模型性能衰退。这包括对系统进行持续的优化和调整,确保AI产品能够持续提供高质量的服务。
AI产品经理如何衡量AI产品成功与否?
-AI产品经理需要与所有利益相关者,特别是领导层,合作设计和对齐适当的度量标准。AI应该支持业务度量标准,并展示其对业务的影响。成功的衡量可能包括早期的胜利、业务指标的提升以及与业务目标的一致性。
AI产品经理如何培养实验文化?
-AI产品经理应该帮助组织建立成功使用AI所需的文化。这包括鼓励智能风险的承担、从失败中学习并不断迭代。他们应该从简单开始,逐步获得早期胜利,并慢慢扩展AI产品的范围。
AI产品经理在开始新工作时应该采取哪些策略?
-AI产品经理在开始新工作时可以采用安德鲁·博斯沃斯的冷启动算法。这包括安排与关键人物的30分钟会议,介绍自己,提出必要的问题,记录详细的笔记,并询问下一步应该与谁交谈。这种方法有助于快速了解新环境并建立联系。
Outlines
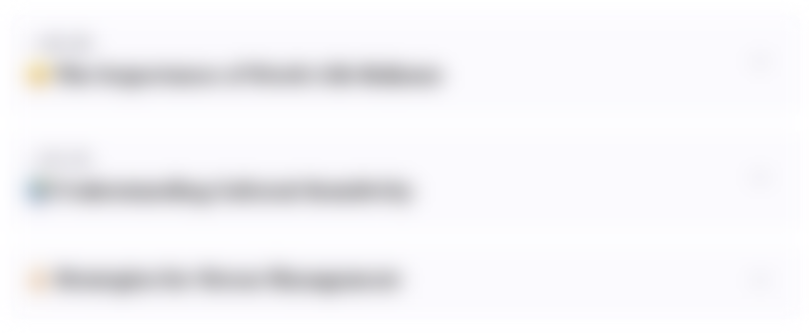
This section is available to paid users only. Please upgrade to access this part.
Upgrade NowMindmap
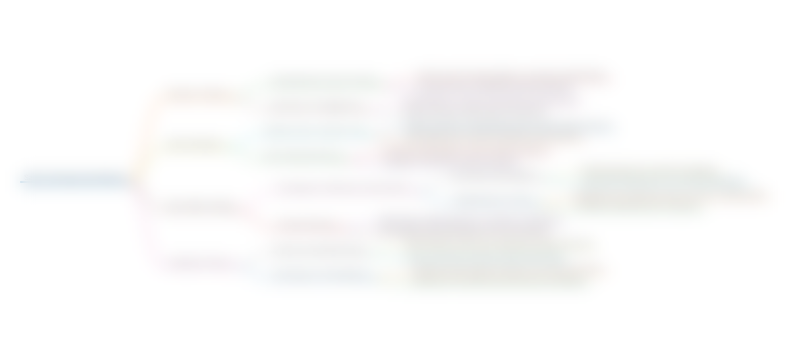
This section is available to paid users only. Please upgrade to access this part.
Upgrade NowKeywords
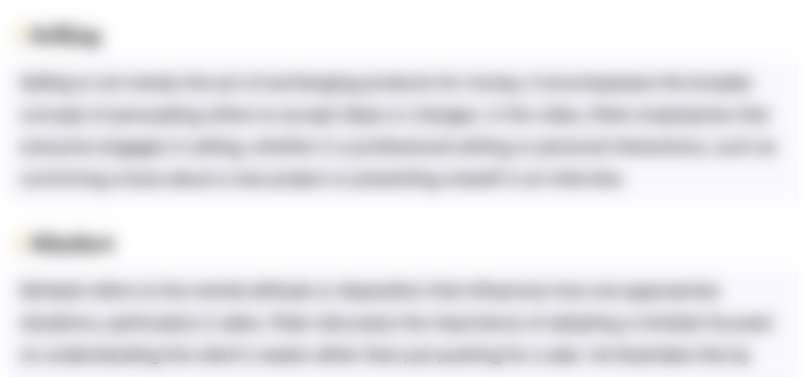
This section is available to paid users only. Please upgrade to access this part.
Upgrade NowHighlights
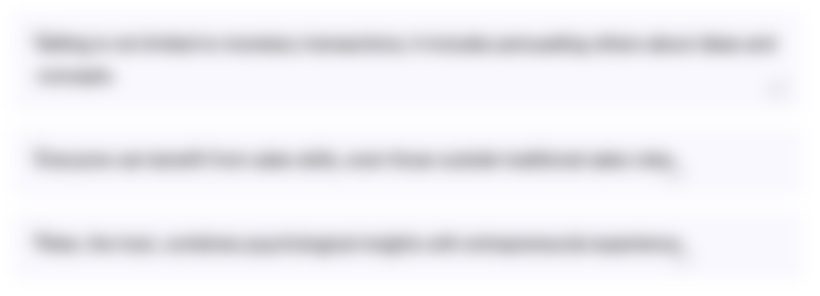
This section is available to paid users only. Please upgrade to access this part.
Upgrade NowTranscripts
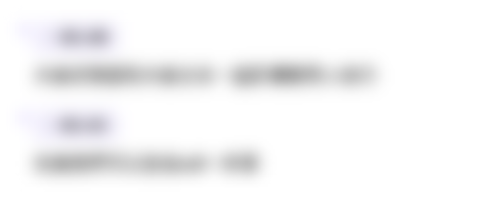
This section is available to paid users only. Please upgrade to access this part.
Upgrade NowBrowse More Related Video
5.0 / 5 (0 votes)