How AI Will Step Off the Screen and into the Real World | Daniela Rus | TED
Summary
TLDRA robotics expert reflects on a key lesson learned during a student project—how the unpredictability of the physical world challenges digital systems. The speaker, now leading MIT's Computer Science and AI Lab, discusses the exciting future of combining AI with robotics. Introducing 'physical intelligence,' the fusion of AI's decision-making abilities with robots' physical capabilities, the talk explores how this breakthrough will transform industries, from designing robots using text prompts to creating adaptive AI. The speaker envisions a world where intelligent machines enhance human abilities, urging collaboration to shape the future of technology responsibly.
Takeaways
- 🤖 Robotics requires careful planning to match the robot's capabilities with the physical world, as shown by the mishap with the cake-cutting robot.
- 🎓 The speaker leads MIT's Computer Science and AI lab, where they're merging AI with robotics to bring intelligence into the physical world.
- 🧠 AI and robotics are traditionally separate fields, but now, AI is moving from digital decision-making into real-world physical applications.
- 🔧 Physical intelligence combines AI's decision-making with the mechanical prowess of robots to make machines smarter and more capable in the physical world.
- 💡 Current AI systems are large, error-prone, and frozen after training, while 'liquid networks' offer a more efficient, adaptive, and understandable solution.
- 🦠 Liquid networks are inspired by the simple neural structures of C. elegans, offering compact and explainable AI that continues to learn after deployment.
- 🚗 Liquid networks lead to better performance in tasks like self-driving, where they focus on relevant features, unlike traditional AI, which can be easily confused.
- 🔬 Text-to-robot and image-to-robot technology allows rapid prototyping, transforming digital designs into real-world machines with ease and precision.
- 👨🍳 Robots can learn tasks from humans by collecting physical data from sensors, making them capable of mimicking human actions with grace and agility.
- 🌍 The speaker emphasizes the potential of physical intelligence to amplify human capabilities, improve daily life, and help create a better future for humanity and the planet.
Q & A
What inspired the speaker's interest in robotics?
-The speaker's interest in robotics began during their time as a student when they and a group of friends attempted to program a robot to cut a birthday cake for their professor.
What was the lesson learned from the cake-cutting robot incident?
-The speaker learned that the physical world, with its laws of physics and imprecisions, is far more demanding than the digital world.
How does the speaker describe the current relationship between AI and robotics?
-Currently, AI and robotics are largely separate fields. AI focuses on decision-making and learning in the digital realm, while robots are physical machines that can execute pre-programmed tasks but lack intelligence.
What is 'physical intelligence' according to the speaker?
-'Physical intelligence' refers to the integration of AI's ability to understand data with the physical abilities of robots, enabling machines to think and interact intelligently in the real world.
What are some of the challenges in achieving physical intelligence?
-Challenges include shrinking AI to run on smaller devices, such as robots, and ensuring that AI can adapt to physical environments without making mistakes.
How do liquid networks improve traditional AI models?
-Liquid networks use fewer neurons with more complex mathematical functions, inspired by biological neurons like those in the C. elegans worm. These networks are adaptable and provide more explainable and focused decision-making compared to traditional AI.
What is the difference between traditional AI and liquid networks in adapting to real-world environments?
-Traditional AI systems are fixed after training and cannot adapt, while liquid networks continue to learn and adapt based on the input they receive, even after deployment.
How does the speaker's lab turn text prompts into robots?
-In the lab, a system starts with a language prompt, generates designs for a robot including its shape, materials, and control system, and then refines the design through simulation until it meets specifications.
What is the significance of human-to-robot learning in the speaker's research?
-Human-to-robot learning allows machines to learn tasks from human behavior, such as food preparation and cleaning, by using physical data like muscle movements and gaze patterns.
What are the potential future applications of physical intelligence?
-Physical intelligence could lead to personalized robots that assist in daily life, bespoke machines for work, and robots that can learn and perform tasks with agility and precision, extending human capabilities.
Outlines
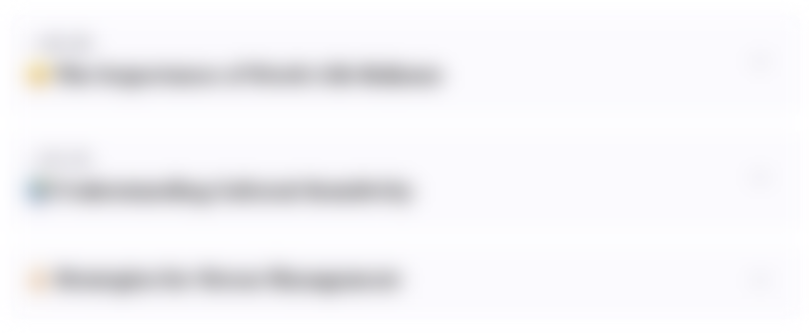
This section is available to paid users only. Please upgrade to access this part.
Upgrade NowMindmap
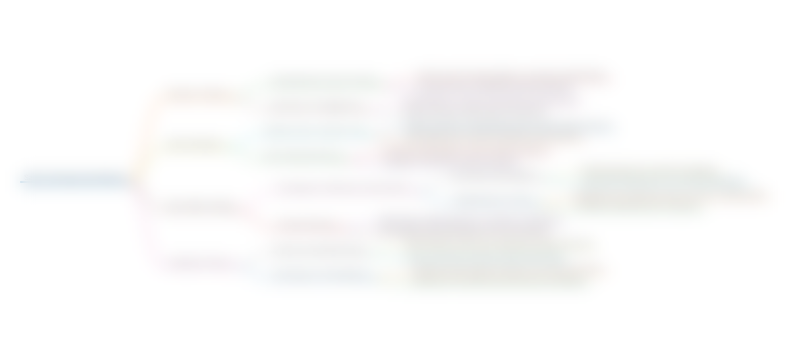
This section is available to paid users only. Please upgrade to access this part.
Upgrade NowKeywords
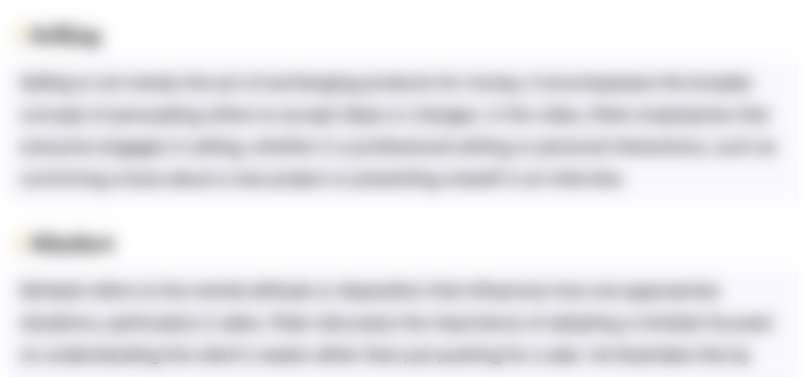
This section is available to paid users only. Please upgrade to access this part.
Upgrade NowHighlights
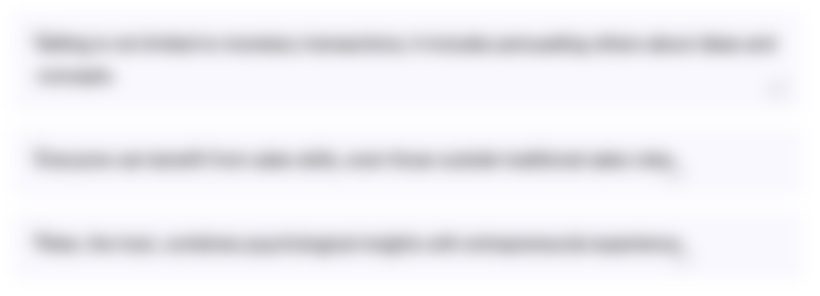
This section is available to paid users only. Please upgrade to access this part.
Upgrade NowTranscripts
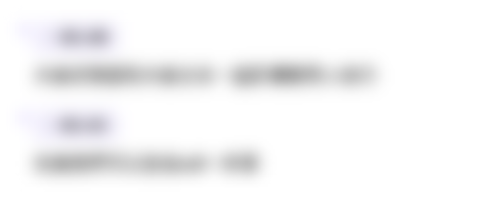
This section is available to paid users only. Please upgrade to access this part.
Upgrade NowBrowse More Related Video
5.0 / 5 (0 votes)