Learning Decision Tree
Summary
TLDRThe transcript discusses the process of decision tree algorithm design, focusing on the importance of attribute selection and example classification. It explains the concept of entropy and its role in determining the purity of a set, guiding the decision-making process in tree splitting. The speaker illustrates how to assess the performance of different attributes using entropy and information gain, emphasizing the goal of maximizing information gain to improve classification accuracy. The summary also touches on the practical application of these concepts in building an optimal decision tree model.
Takeaways
- π The discussion begins with a review of the decision tree algorithm and its foundational structure.
- π The script introduces the concept of using 'd' as a group of decision examples and 'A' as attributes, with two children of 'A' being considered.
- π It explains the process of determining a decision tree by identifying the best attributes to split the data, starting with 'A' and then dividing 'D' into 'D1' and 'D2'.
- π The script discusses the importance of entropy in decision-making within the tree, using examples to illustrate how to calculate and apply it.
- π The concept of choosing the best attribute to split the data is emphasized, with a focus on minimizing entropy.
- π The script provides a method to decide when to stop splitting, considering various scenarios and conditions.
- π‘ It introduces the idea of using information gain to select the best attribute, explaining how it can be calculated and used to make decisions.
- π The script explains how to handle continuous attributes in decision trees, suggesting methods for discretization.
- π The process of building a decision tree is outlined, including the use of examples to illustrate the steps involved.
- π The script concludes with a summary of the decision tree construction process, emphasizing the importance of understanding the underlying principles and calculations.
Q & A
What does the speaker suggest to start with when discussing decision trees?
-The speaker suggests starting with the concept of 'd', which is a group of examples for decision trees, and then moving on to identify 'A' as the attribute to consider, with two children of 'A' and examples for 'D' that are initially related to 'A'.
How does the speaker propose to decide on the division of 'D' into 'D1' and 'D2'?
-The speaker proposes to consider a specific branch as equivalent to 'A', meaning that 'D1' would contain examples equivalent to 'A1', which are similar to 'A', and 'D2' would contain examples equivalent to 'A2', which are similar to 'A' being false.
What is the significance of the examples in 'D1' and 'D2' according to the speaker?
-The examples in 'D1' are significant as they are equivalent to 'A1', which is similar to 'A', and the examples in 'D2' are significant as they are equivalent to 'A2', which is similar to 'A' being false.
What is the purpose of the decision-making process described in the script?
-The purpose of the decision-making process is to determine the best attribute to split on in a decision tree, considering the entropy and information gain at each step.
How does the speaker suggest making decisions about which attribute to split on?
-The speaker suggests making decisions by considering the number of features that need to be nullified, and by looking at the attributes that are most significant in reducing entropy.
What is the role of entropy in the decision-making process as described in the script?
-Entropy plays a crucial role in the decision-making process as it helps in determining the purity of the classes. The goal is to minimize entropy by choosing the attribute that best splits the data into classes with high purity.
How does the speaker explain the concept of information gain in the context of decision trees?
-The speaker explains information gain as the reduction in entropy after a split. It is calculated by comparing the entropy of the parent node to the weighted average entropy of the child nodes resulting from the split.
What is the significance of the attribute 'A1' in the decision tree example provided by the speaker?
-The attribute 'A1' is significant because it is used to further split the data and is considered to have a higher information gain, leading to a more informative decision tree.
How does the speaker use the concept of 'entropy' to decide on the splits in the decision tree?
-The speaker uses the concept of 'entropy' to decide on the splits by calculating the entropy of the examples in each branch and choosing the split that results in the lowest entropy, indicating a more informative split.
What does the speaker mean by 'majority voting' in the context of decision trees?
-The speaker refers to 'majority voting' as a method where the class label is determined by the majority of the training examples in each node of the decision tree.
How does the speaker suggest improving the decision tree model?
-The speaker suggests improving the decision tree model by continuously identifying and splitting on the attributes that provide the highest information gain and lowest entropy.
Outlines
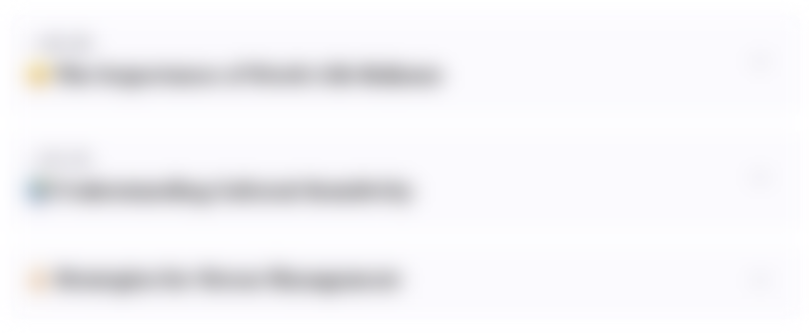
This section is available to paid users only. Please upgrade to access this part.
Upgrade NowMindmap
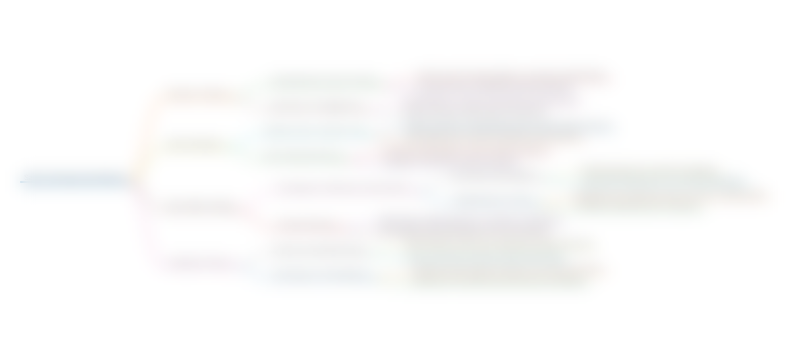
This section is available to paid users only. Please upgrade to access this part.
Upgrade NowKeywords
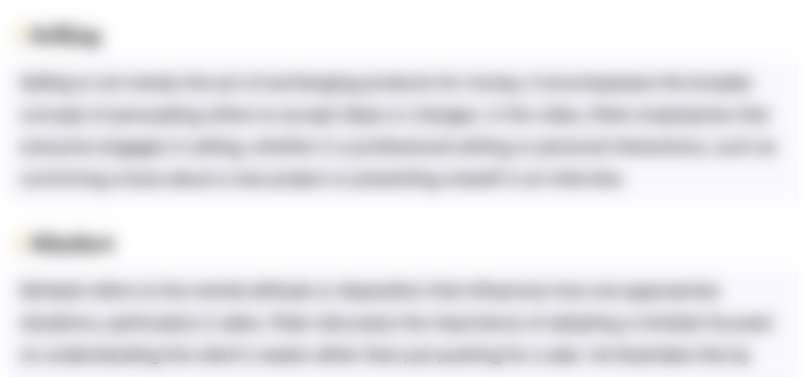
This section is available to paid users only. Please upgrade to access this part.
Upgrade NowHighlights
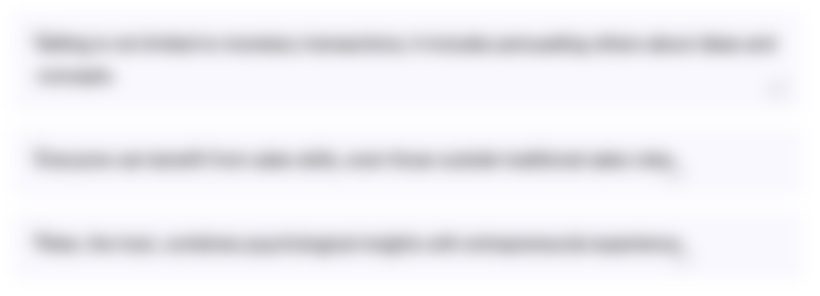
This section is available to paid users only. Please upgrade to access this part.
Upgrade NowTranscripts
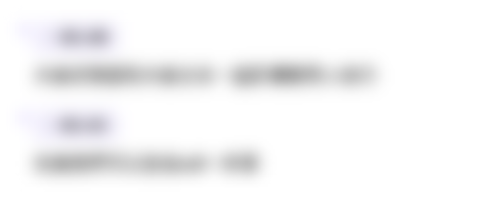
This section is available to paid users only. Please upgrade to access this part.
Upgrade NowBrowse More Related Video

Machine Learning Tutorial Python - 9 Decision Tree
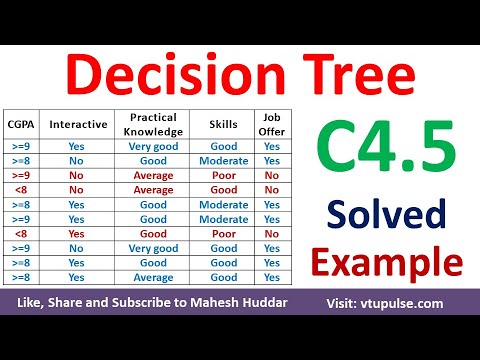
Decision Tree using C4.5 Algorithm Solved Numerical Example | C4.5 Solved Example by Mahesh Huddar
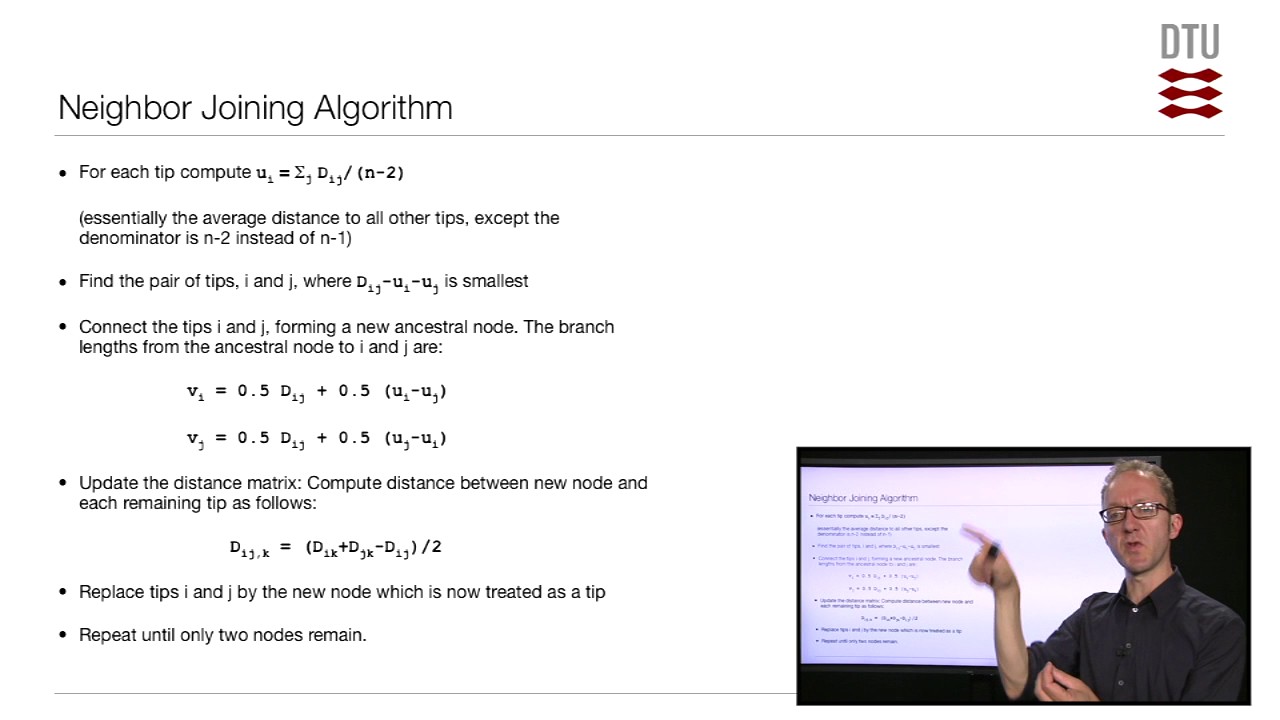
Neighbour Joining

Konsep memahami Algoritma C4.5

Introduction to Decision Trees
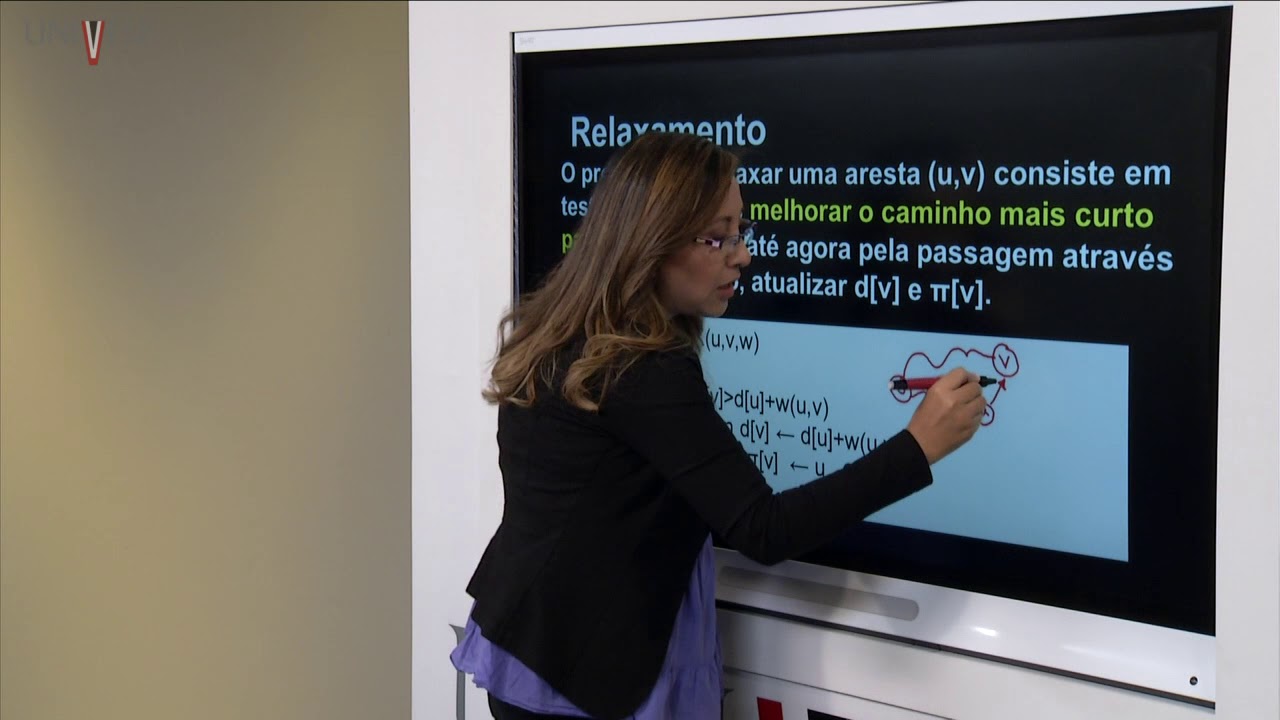
Projeto e AnΓ‘lise de Algoritmos - Aula 13 - Problema do caminho mais curto
5.0 / 5 (0 votes)