Will "Claude Investor" DOMINATE the Future of Investment Research?" - AI Agent Proliferation Begins
Summary
TLDRThe transcript discusses the rapid advancements in AI, particularly in agentic workflows, which are expected to drive significant progress in the field. It highlights the potential release of GPT-5 and the evolution of AI models like CLA, which has transitioned from creating self-portraits to investment analysis. The importance of iterative processes and multi-agent collaboration is emphasized, with examples such as the development of an open-source alternative to Devon and the iterative improvement in AI's ability to write code and play games like Super Mario 64. The transcript also touches on the educational resources available for learning about AI and the potential impact of AI agents on various industries.
Takeaways
- ๐ Andrew A emphasizes the significance of AI agentic workflows, predicting they will drive substantial AI progress, potentially outpacing the next generation of foundation models like the anticipated GPT-5.
- ๐ฎ Sam Altman expects a major model launch later in the year, with CLA 3 demonstrating a shift from creating self-portraits to more complex tasks like investment analysis.
- ๐ The creator of Claud investor, an AI investment analyst, has open-sourced the tool, showcasing the potential for AI in financial analysis and decision-making.
- ๐ค AI development is moving towards more collaborative and iterative processes, with multiple agents working together and refining tasks through planning, tool use, and reflection.
- ๐ AI's capability in coding is improving, with GPT 3.5 and 4 demonstrating higher correctness rates in coding tasks compared to their predecessors.
- ๐ ๏ธ Tool use is a critical component in AI workflows, allowing AI to gather information, take action, or process data more effectively.
- ๐ Iterative processes and multi-agent collaboration significantly enhance the quality of AI output, yielding results that surpass those of single-pass writing or isolated AI models.
- ๐ Andrew A shares a framework for categorizing design patterns for building agents, highlighting reflection, tool use, planning, and multi-agent collaboration as key elements.
- ๐ Claud Investor illustrates the application of AI in investment analysis, providing an example of how AI can synthesize financial data and news to make investment recommendations.
- ๐ฎ Experiments with GPT-4's ability to play games like Super Mario 64 show the potential for AI in interactive environments, despite latency and decision-making challenges.
Q & A
What does Andrew A. urge everyone to pay attention to?
-Andrew A. urges everyone to pay attention to AI agentic workflows, as he believes they will drive massive AI progress.
What is expected to be released later this year in terms of AI foundation models?
-GPT-5, the next generation of OpenAI's foundation models, is expected to be released later this year.
What is CLA, and what is its recent development?
-CLA is an AI model that has evolved from drawing somewhat disturbing self-portraits to trying its hand at beating Warren Buffett as an investor.
What does the creator behind Claud investor say about potentially open-sourcing it?
-The creator behind Claud investor has mentioned that they may open-source it the next day, and indeed, they do open-source it.
What is Devon and how was it received in the AI community?
-Devon is an open-source alternative to impressive seeming AI agents that was recently released and is currently available to a small group of testers.
What is the significance of Andrew A.'s statement about agentic workflows being more important than the next generation of foundation models?
-Andrew A.'s statement highlights the potential impact of agentic workflows on AI progress, suggesting that their importance may surpass that of foundational models like GPT-5.
How does an agentic workflow with an LM (Language Model) differ from a zero-shot approach?
-An agentic workflow involves the LM iterating over a document multiple times, planning, researching, writing drafts, and revising, whereas a zero-shot approach involves the LM answering a question or completing a task in one go without prior examples or iterations.
What is the 'Reflection' tool use case mentioned in the script?
-In the context of AI, 'Reflection' refers to the AI examining its own work to come up with ways to improve it, which is a critical part of an agentic workflow.
How does multi-agent collaboration improve the results of AI tasks?
-Multi-agent collaboration involves more than one AI agent working together, splitting up tasks, and discussing or debating ideas to come up with better solutions than a single agent would be able to achieve.
What is the improvement rate when incorporating an iterative agent workflow with GPT 3.5?
-Incorporating an iterative agent workflow with GPT 3.5 can achieve up to a 95.1% improvement rate, which is significantly better than the 48% rate of GPT 3.5 alone and even surpasses the performance of GPT 4.
What is the potential application of AI agents in the stock market according to the script?
-AI agents can be used to analyze financial data, news, sentiment, and industry trends for stocks within a given industry, rank them by investment potential, and provide price targets, although it's emphasized that these are for educational or informational use only.
Outlines
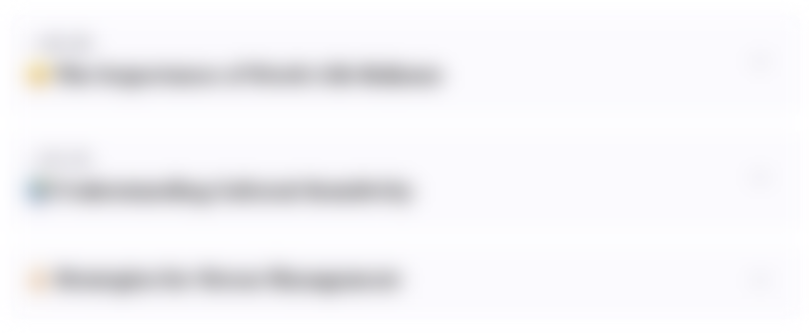
This section is available to paid users only. Please upgrade to access this part.
Upgrade NowMindmap
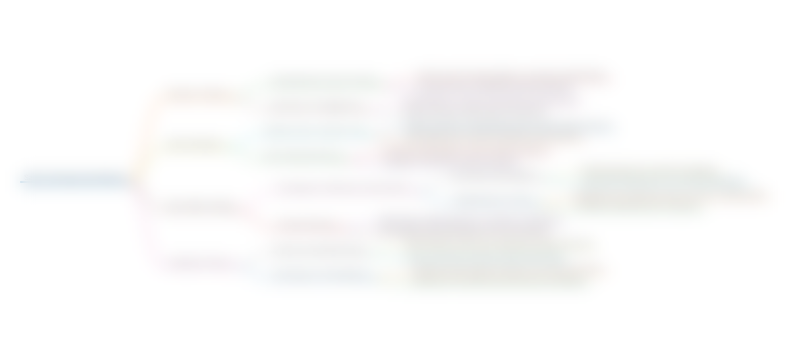
This section is available to paid users only. Please upgrade to access this part.
Upgrade NowKeywords
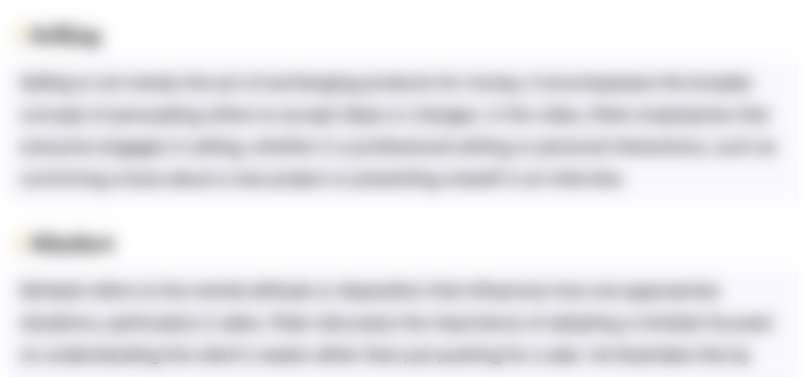
This section is available to paid users only. Please upgrade to access this part.
Upgrade NowHighlights
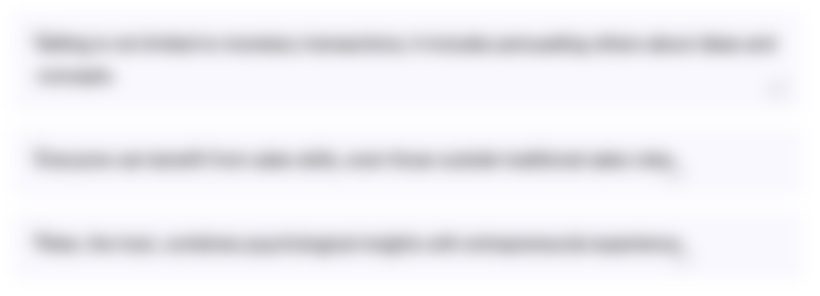
This section is available to paid users only. Please upgrade to access this part.
Upgrade NowTranscripts
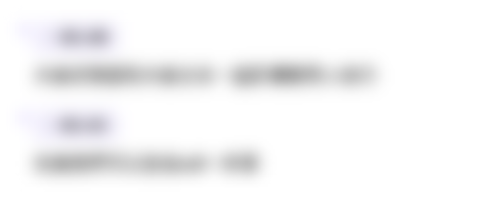
This section is available to paid users only. Please upgrade to access this part.
Upgrade NowBrowse More Related Video
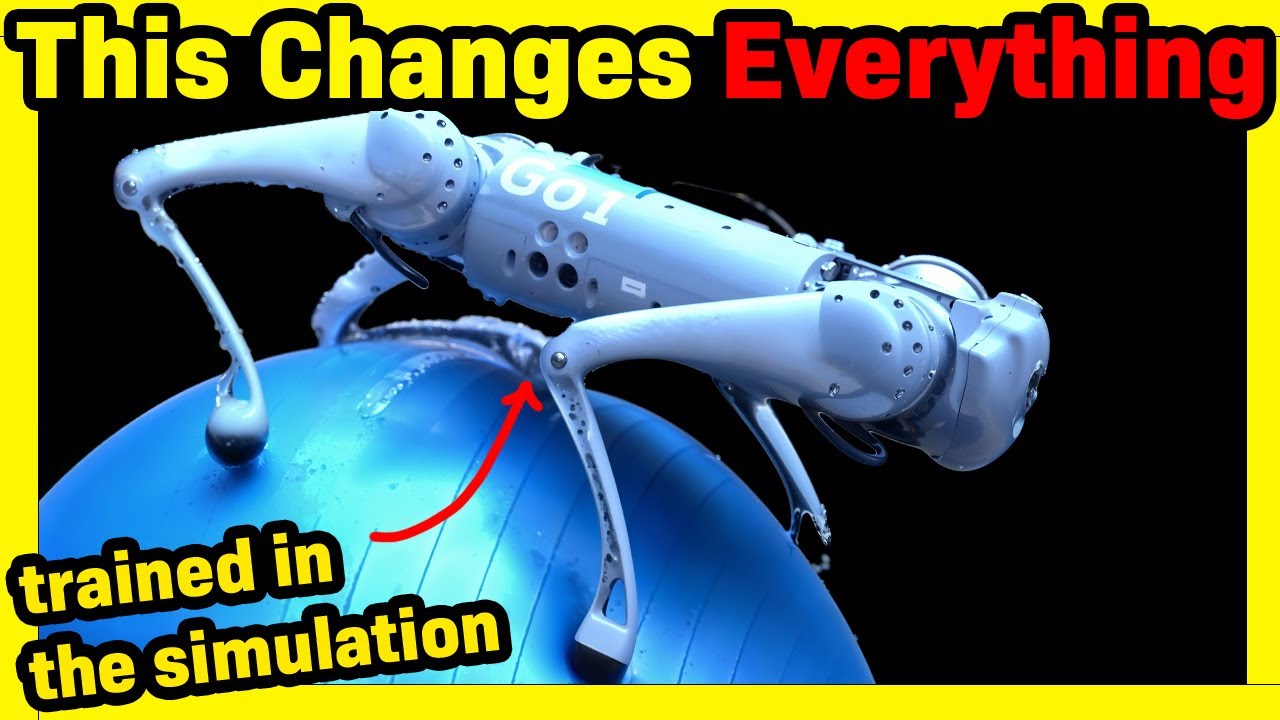
SHOCKING Robots EVOLVE in the SIMULATION plus OpenAI Leadership Just... LEAVES?
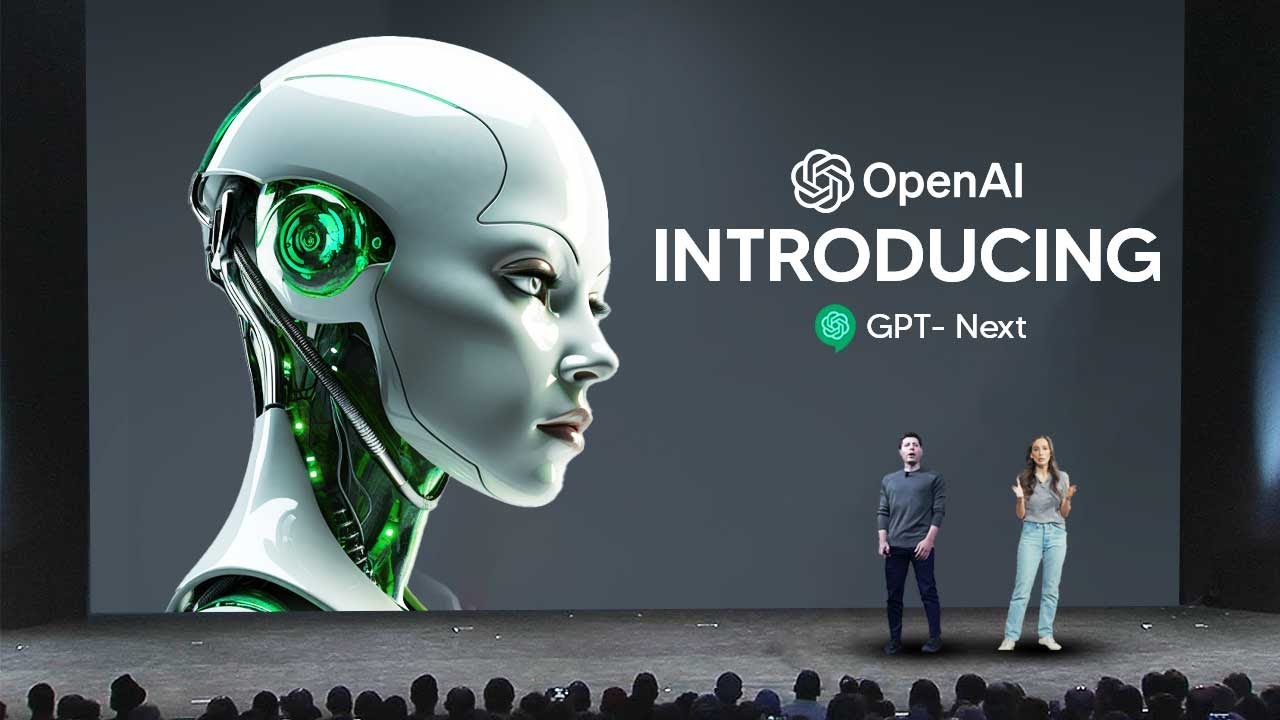
OpenAI'S "SECRET MODEL" Just LEAKED! (GPT-5 Release Date, Agents And More)
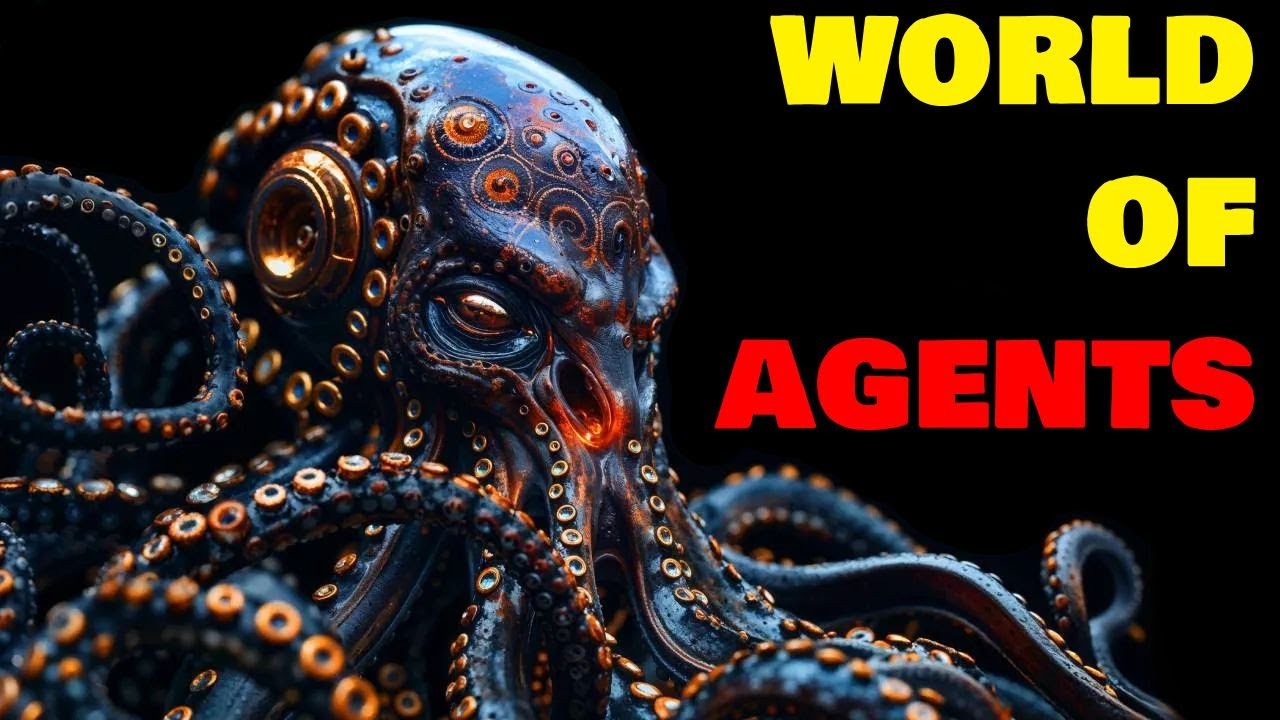
STUNNING Step for Autonomous AI Agents PLUS OpenAI Defense Against JAILBROKEN Agents
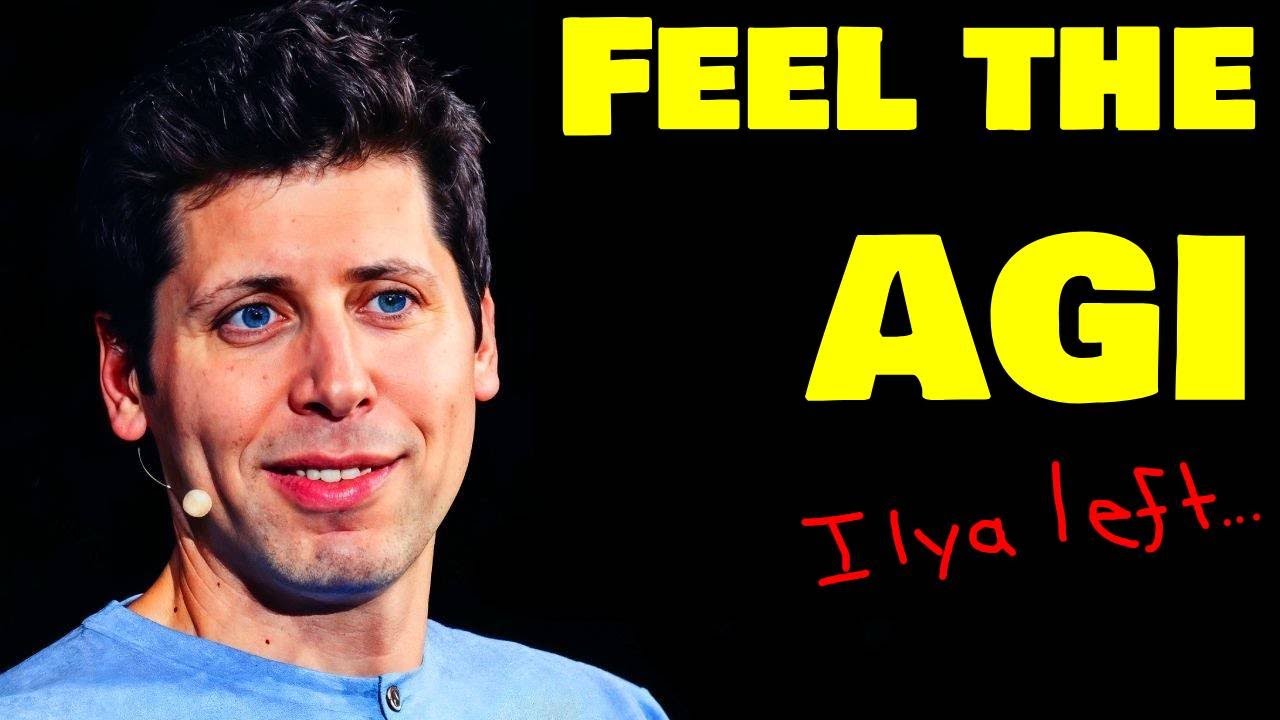
AI News: The AI Arms Race is Getting INSANE
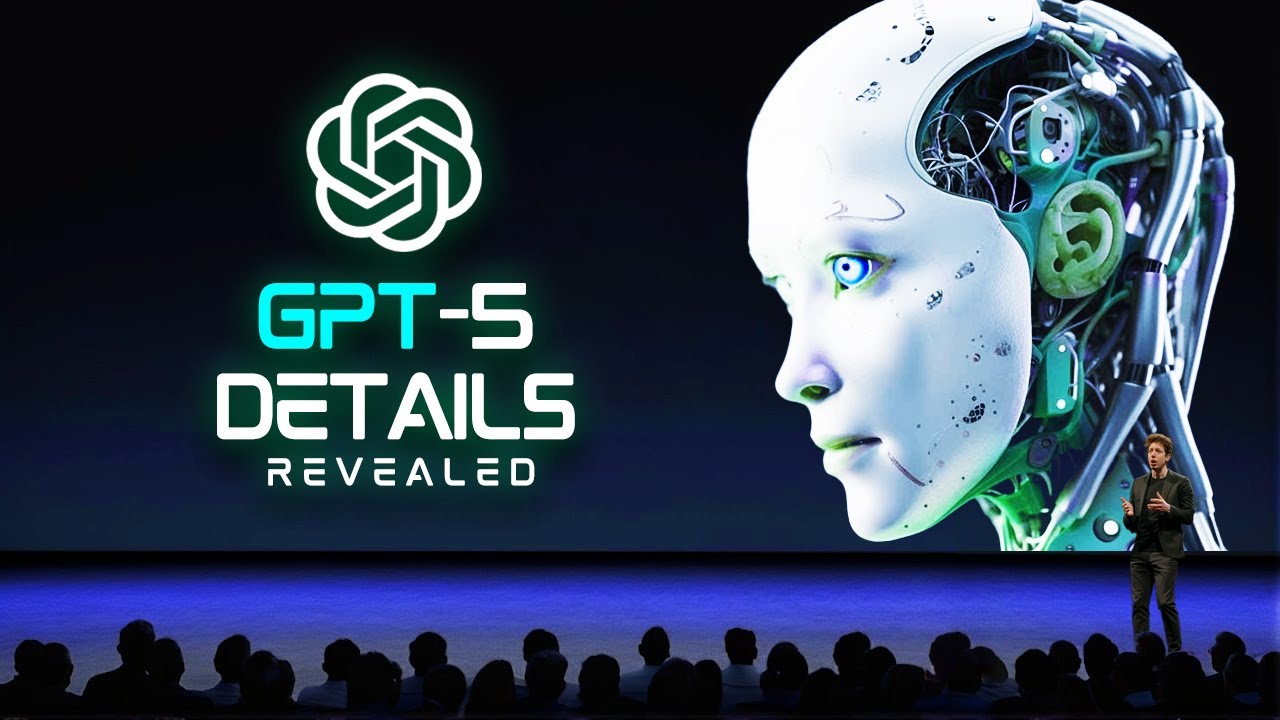
OpenAI Reveals New ChatGPT-5 Details

Digital Transformations and AI - Video 3
5.0 / 5 (0 votes)