🔴 10 AI Agent Systems That ACTUALLY Work! (The last one is a gem!) | Agentic Workflows + Results
Summary
TLDRThis video explores the concept of agentic AI workflows, where AI systems improve their output iteratively through self-refinement, feedback, and the use of tools. It discusses recent research like self-refine, reflection methods, and multi-agent collaborations in AI, such as Autogen and the Internet of Agents (IOA). These systems demonstrate how AI can plan, reason, and collaborate to perform complex tasks more effectively. The video also highlights the emerging field of autonomous systems like Adala for data labeling. With advancements in AI workflows, the potential for efficient, scalable, and specialized AI applications is growing rapidly.
Takeaways
- 😀 **Agentic AI Workflow** refers to AI systems that can refine their outputs through iterative self-feedback and reflections, improving accuracy and performance.
- 😀 **Self-Reflection and Iterative Learning** are key components of agentic AI, with models like GPT-4 demonstrating the ability to refine results through reflection, as shown in the **Reflection Language Agents** framework.
- 😀 **Tool Usage** in agentic AI allows models to interact with external APIs, enhancing their functionality beyond generating text, enabling tasks like document retrieval and more.
- 😀 **Chain of Thought Prompting** helps AI systems break down complex tasks into smaller steps, facilitating structured reasoning and planning in task completion.
- 😀 **Multi-Agent Collaboration** utilizes different agents working together, often with human oversight, to achieve better outcomes, as explored in projects like **Autogen** and **Internet of Agents (IOA)**.
- 😀 The **Mixture of Agents (MOA)** method improves task accuracy by using specialized models for distinct tasks and combining them for enhanced results, outperforming single models like GPT-4 in certain benchmarks.
- 😀 **Autonomous Data Labeling (Adala System)** leverages AI agents to independently label data with high accuracy, reducing the need for human intervention in data processing tasks.
- 😀 The **Adala System** can apply labels such as sentiment analysis, question answering, and text classification autonomously by learning from a provided ground truth dataset.
- 😀 AI agents in systems like **Adala** continuously improve their skillset through iterative learning, allowing them to adapt to new tasks without additional human input.
- 😀 The future of AI involves more **agentic AI workflows**, offering a dynamic and autonomous approach to completing complex tasks with minimal human intervention.
Q & A
What is the concept of agentic AI, and how does it differ from traditional AI usage?
-Agentic AI refers to a system where an AI, such as a large language model (LLM), is asked to refine its outputs iteratively by providing self-feedback and revising its work. Unlike traditional AI, which often produces a final output based on a single prompt, agentic AI uses a feedback loop to continually improve the results, much like how humans work through drafts and revisions.
How does the 'self-refine' method work in agentic AI systems?
-The 'self-refine' method involves generating an initial output using an LLM, then having the model provide feedback on its own output. This feedback is used for iterative improvement, without the need for additional training or reinforcement learning. This process continues, with each iteration refining the previous result.
What is the role of reflection in agentic AI, and how is it applied?
-Reflection in agentic AI refers to the model's ability to review and adjust its outputs based on feedback. The reflection process, seen in frameworks like Reflection Language Agents with Verbal Reinforcement Learning, allows the AI to improve its reasoning and decision-making abilities by using verbal feedback rather than traditional reinforcement learning methods.
How do agentic AI systems use tools to perform tasks, and why is this important?
-Agentic AI systems can access various tools via API calls, allowing them to perform tasks like document retrieval or code generation. This tool usage enables the AI to expand its capabilities beyond text generation, making it more versatile and efficient in solving complex tasks. For example, Gorilla is a system that can write API calls effectively and improve on traditional models like GPT-4.
What is 'chain of thought prompting,' and how does it benefit agentic AI models?
-Chain of thought prompting involves providing an AI model with a series of intermediate reasoning steps that help it solve complex problems. This method allows the AI to break down tasks into smaller, more manageable steps, improving its reasoning ability and performance on challenging tasks, as seen in tests like GSM8K.
Can multiple agentic AI systems collaborate on tasks? How does this work?
-Yes, multiple agentic AI systems can collaborate on tasks, and systems like Autogen enable collaboration between both human users and multiple AI agents. This collaboration allows for more complex and nuanced problem-solving, where each agent can specialize in different aspects of the task, like code generation or reasoning.
What is the 'Internet of Agents' (IOA) system, and what problem does it address?
-The Internet of Agents (IOA) system is a decentralized, collaborative platform that connects multiple AI agents across different environments, allowing them to collaborate on complex tasks. Inspired by the internet's decentralized nature, IOA allows agents to form teams autonomously, adapt to tasks, and learn from each other's experience, tackling issues that single models cannot handle alone.
How does the 'Mixture of Agents' (MOA) framework improve AI performance?
-The Mixture of Agents (MOA) framework uses multiple LLM agents to collaboratively solve a task. Each agent in the system generates responses independently, and those responses are refined by agents in subsequent layers. This iterative refinement process ensures higher accuracy and robustness, especially in complex tasks, by leveraging the diversity and expertise of various models.
What is the significance of agentic AI in reducing the cost of software development?
-Agentic AI can significantly reduce the cost of software development by automating tasks traditionally handled by human engineers, such as designing, coding, testing, and documentation. Systems like Chad Dev employ multiple agents with different roles, mimicking the collaborative nature of a real-world software development team, thereby lowering costs and enhancing efficiency.
How does the Adala system contribute to data labeling tasks?
-Adala is a framework for autonomous data labeling using agentic AI. It allows AI agents to independently learn and apply skills such as sentiment analysis and text classification to a set of data. With the ability to refine their understanding iteratively, Adala provides a high level of accuracy in data labeling, making it a valuable tool for automating the data processing pipeline.
Outlines
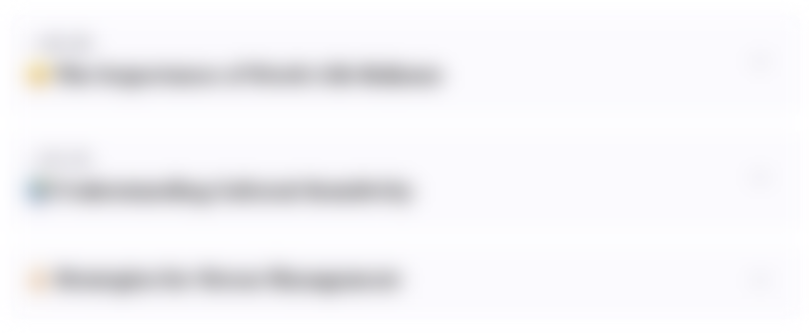
This section is available to paid users only. Please upgrade to access this part.
Upgrade NowMindmap
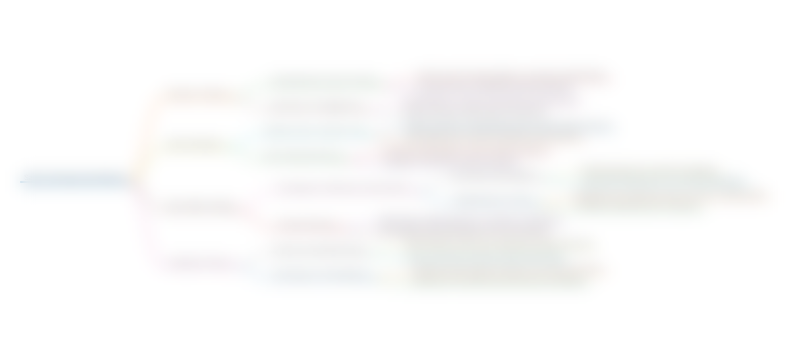
This section is available to paid users only. Please upgrade to access this part.
Upgrade NowKeywords
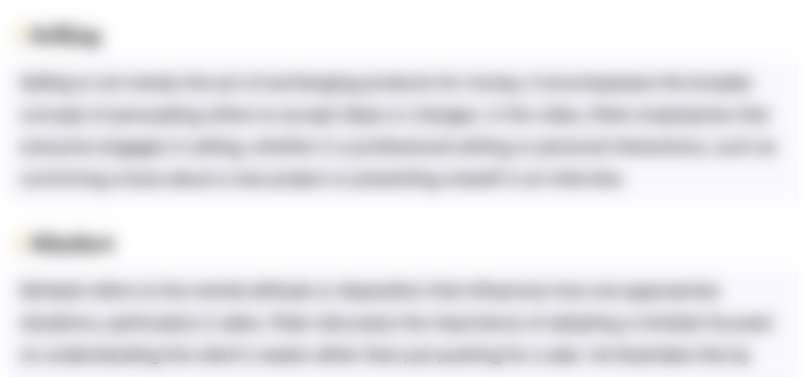
This section is available to paid users only. Please upgrade to access this part.
Upgrade NowHighlights
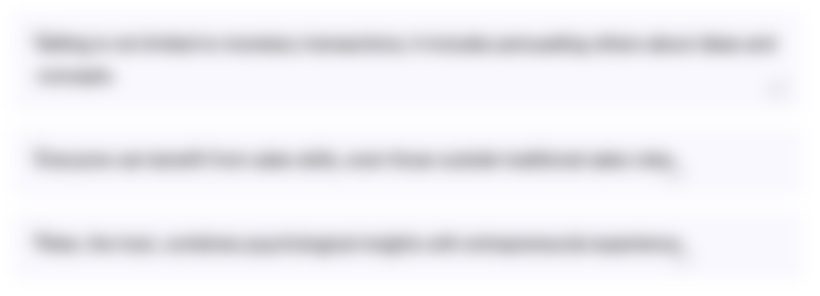
This section is available to paid users only. Please upgrade to access this part.
Upgrade NowTranscripts
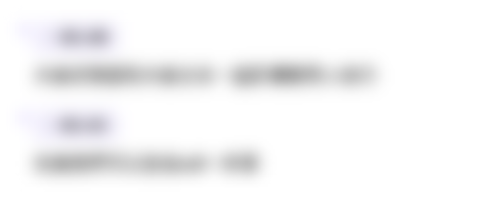
This section is available to paid users only. Please upgrade to access this part.
Upgrade NowBrowse More Related Video
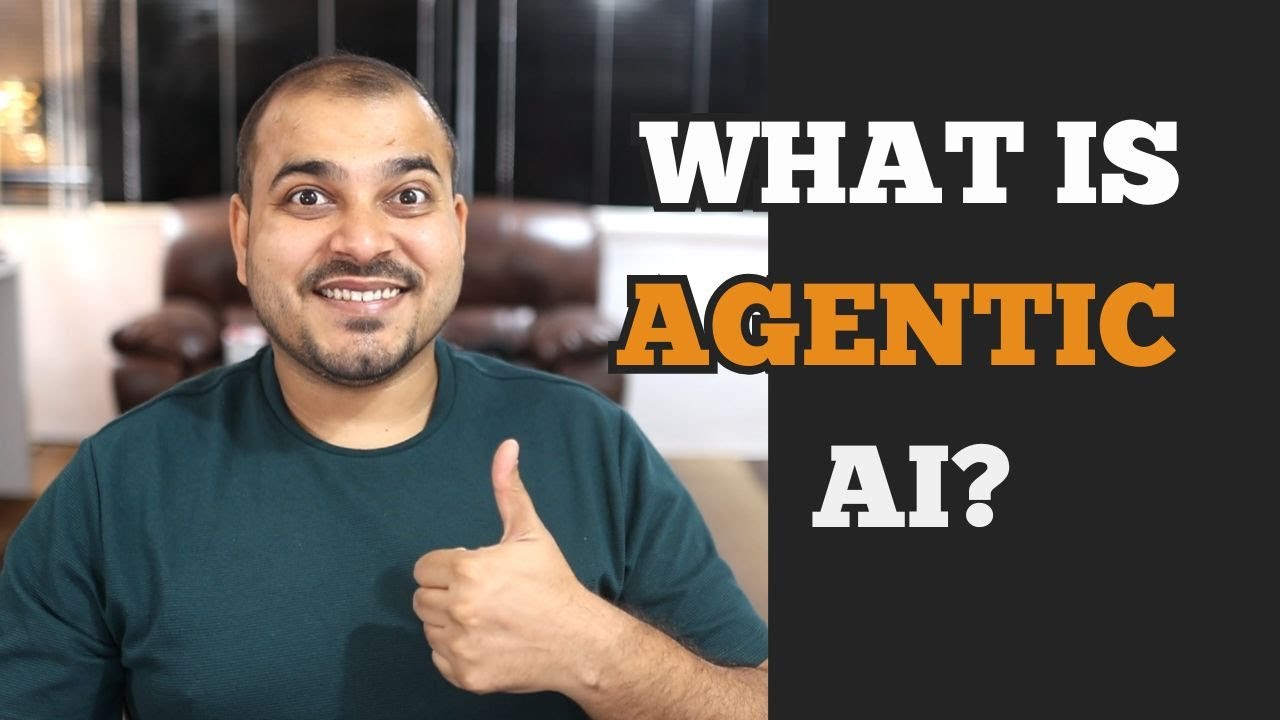
What is Agentic AI? Important For GEN AI In 2025
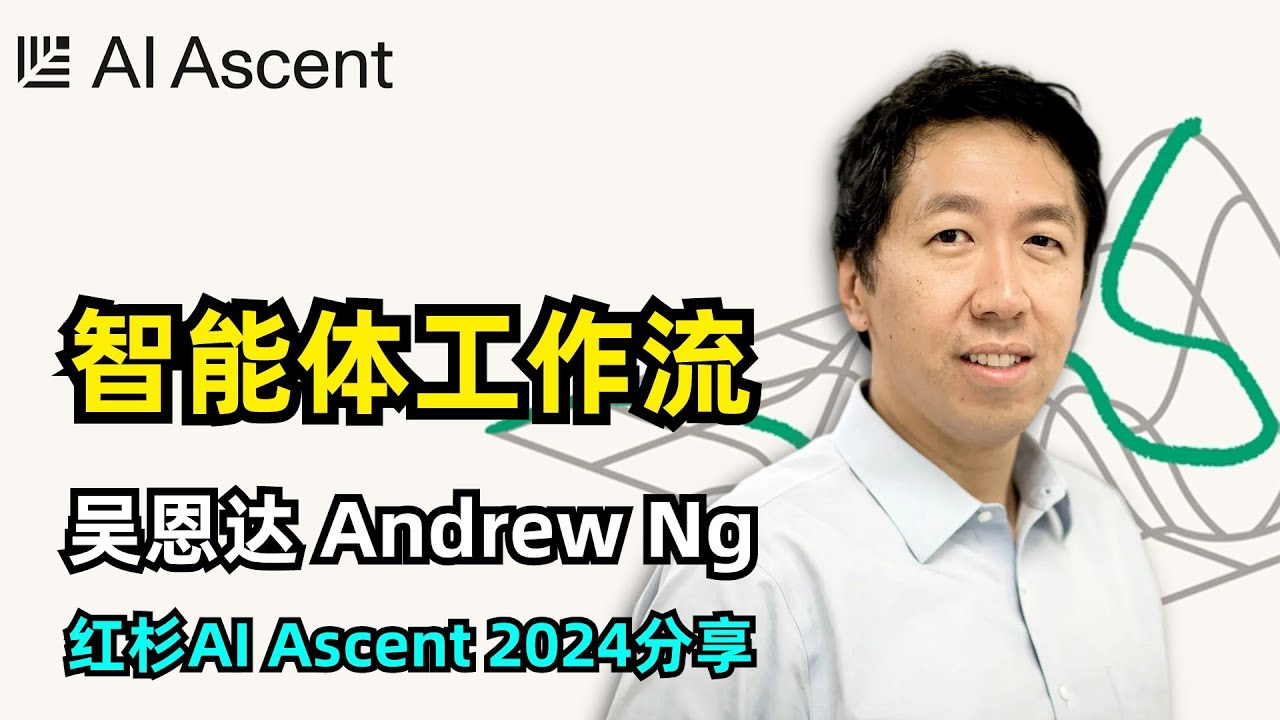
【人工智能】AI智能体工作流 | Agentic Reasoning | 吴恩达Andrew Ng | 红杉AI Ascent 2024分享 | Agent 4大设计模式
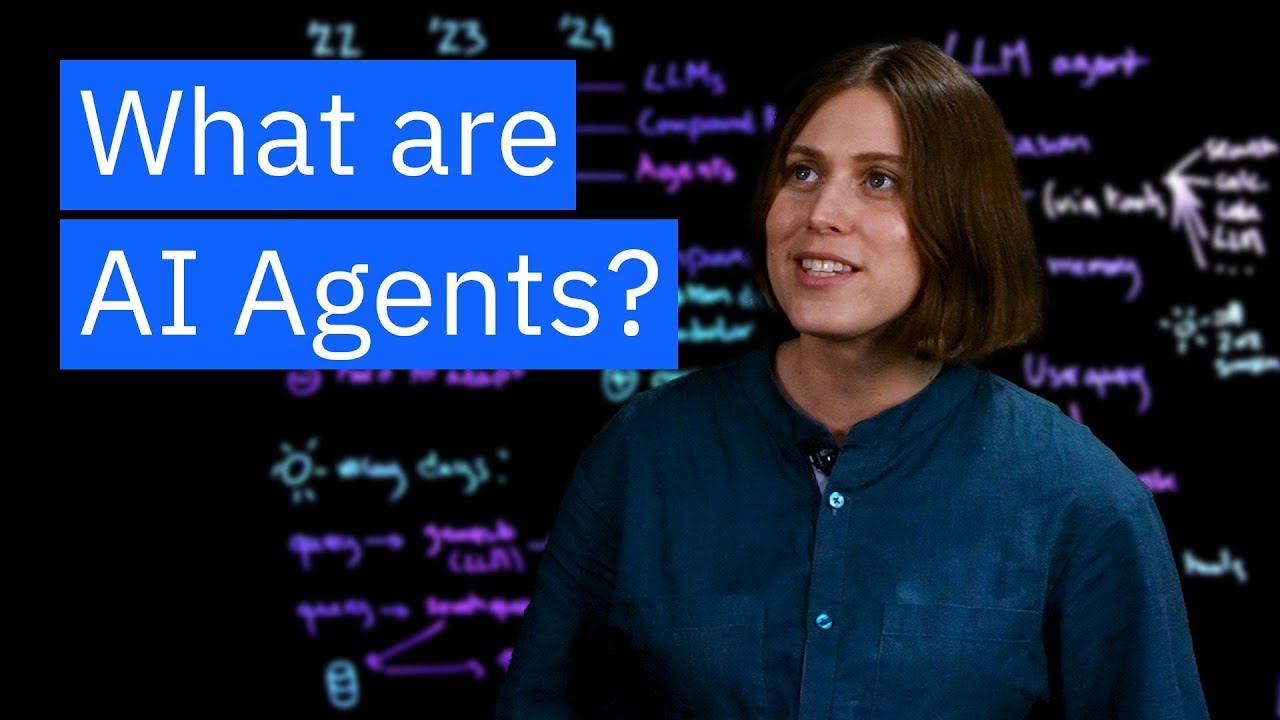
What are AI Agents?
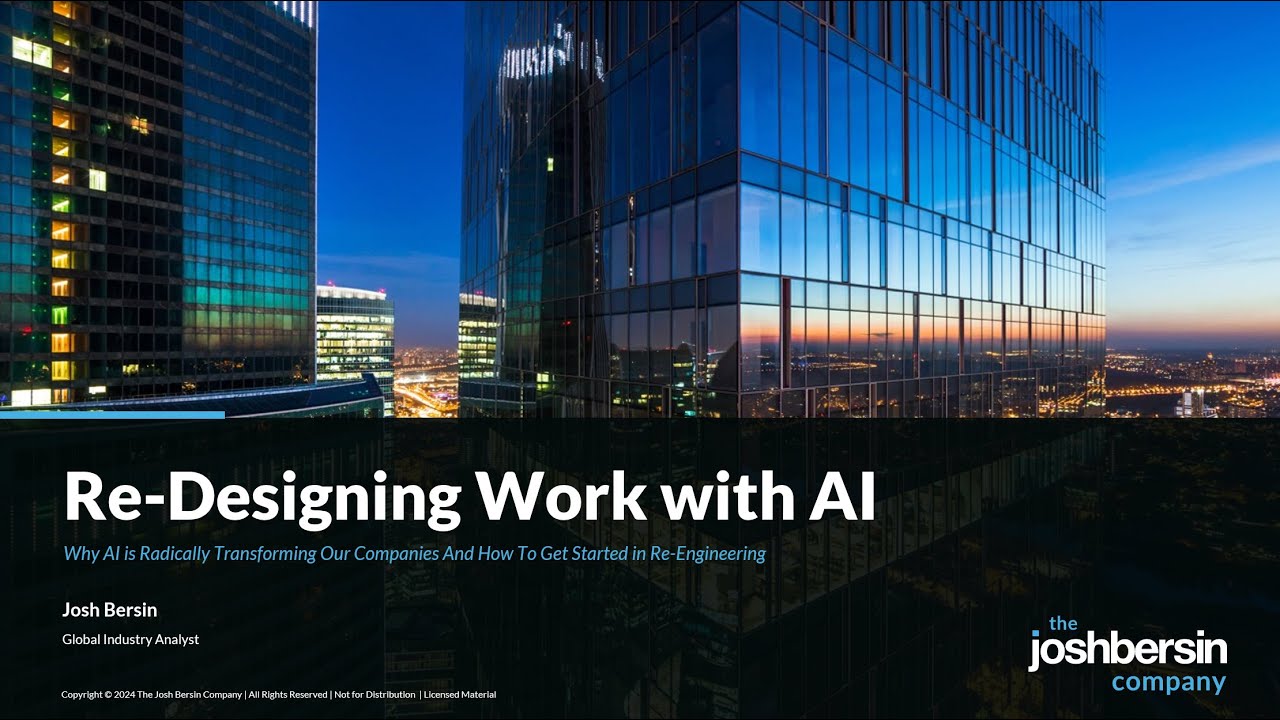
How To Redesign Your Work And Your Business With AI
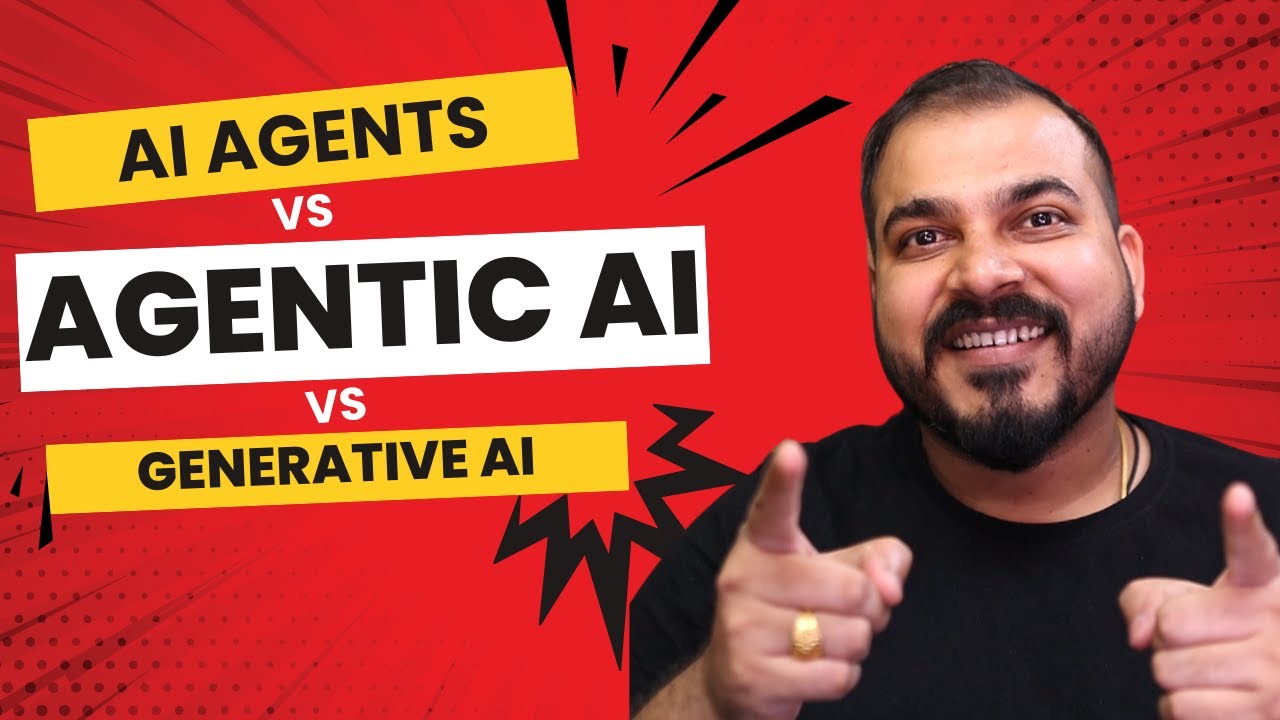
Generative AI Vs Agentic AI Vs AI Agents
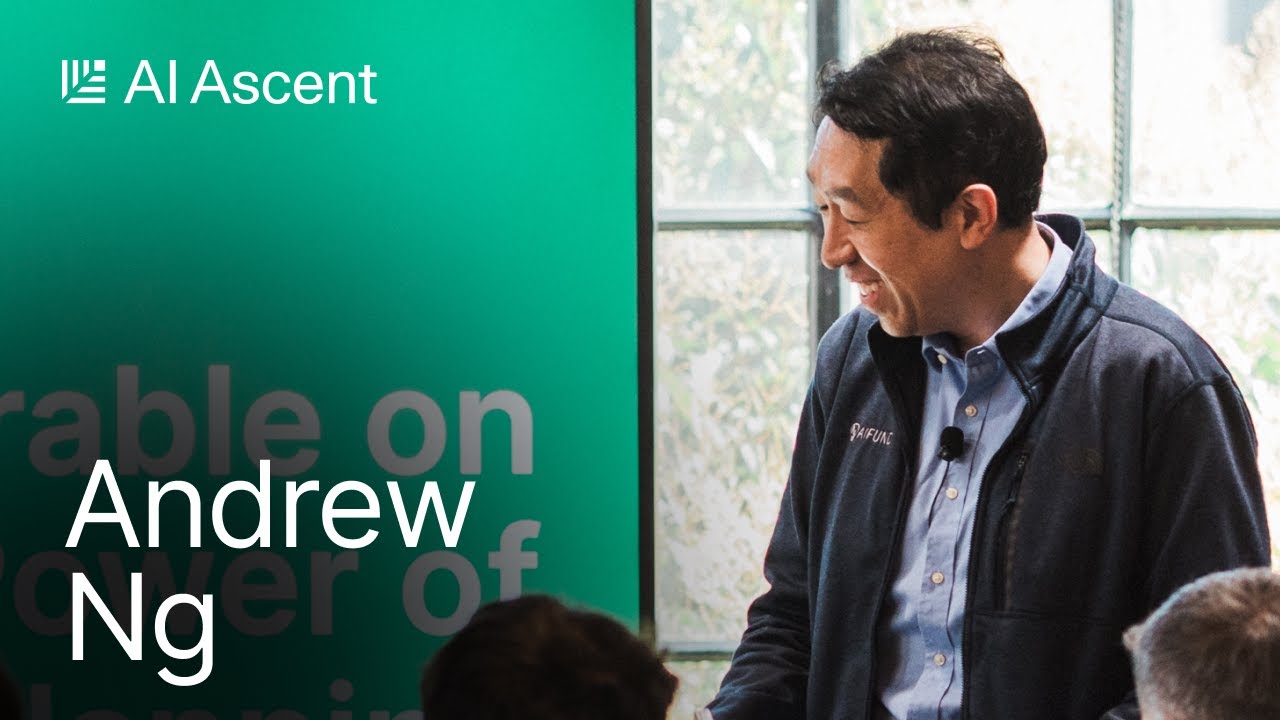
What's next for AI agentic workflows ft. Andrew Ng of AI Fund
5.0 / 5 (0 votes)