Augmentation of Data Governance with ChatGPT and Large LLMs
Summary
TLDR在这期《数据之光》节目中,我们邀请到了RecordPoint的联合创始人兼CEO安东尼·伍德沃德,深入探讨了数据治理如何通过ChatGPT和其他大型语言模型得到增强。安东尼分享了他对于大型语言模型定义的见解,以及它们如何帮助企业更有效地管理数据,降低风险,并提高合规性。此外,他还讨论了大型语言模型在数据分类、总结、问题回答、实体识别和情感分析等方面的应用,以及使用这些模型时可能遇到的风险和挑战。通过此次对话,观众能够对如何利用这些前沿技术优化数据治理过程获得更深入的理解。
Takeaways
- 😀 Anthony Woodward是Record Point的联合创始人和CEO,这是一个快速发展的软件服务解决方案,旨在帮助组织发现、治理和控制他们的数据,以便更严格的合规、更高效和更低的风险。
- 📊 大型语言模型(LLMs),如ChatGPT和其他,被讨论用于数据治理增强,它们通过神经网络,能够生成文本,进行对话,写作文,等等。
- ☕ Anthony分享了他对咖啡的热情,以及搬到西雅图后,如何继续他的咖啡爱好和学习雪板作为新爱好。
- 🧠 LLMs是基于数学模型,能够模拟人脑的连接神经元方式来处理和生成语言,这让它们在处理语言方面表现出色。
- 🔍 LLMs在数据治理中的应用包括数据分类、数据摘要、问答系统,以及实体识别和情感分析,这对于管理风险和合规性至关重要。
- 🤖 尽管LLMs表现强大,但它们也容易受到偏见的影响,需要管理和调整以确保准确性和公正性。
- 💡 LLMs能够在不需要预先定义的本体论的情况下生成分类和本体论,但通过细化可以提高它们的准确性。
- 🚀 Record Point使用LLMs来提高数据分类和标记的效率,同时也提供了一个反馈循环,允许人工校验和改善分类结果。
- 📈 LLMs预计将极大加速数据治理和其他商业流程的能力,预示着人工智能技术将成为未来技术的一个核心组成部分。
- 🔑 Anthony提醒,使用免费版本的LLMs(如ChatGPT)可能会与数据隐私政策冲突,推荐在商业应用中考虑使用付费版本或API。
- 🌐 LLMs的未来发展将进一步深化它们在数据治理、隐私保护和业务决策支持中的作用,但同时也需要注意数据的安全性和隐私保护。
Q & A
安东尼·伍德沃德是谁?
-安东尼·伍德沃德是Record Point的联合创始人兼首席执行官,这是一家快速增长的软件即服务解决方案公司,专注于帮助组织发现、管理和控制他们的数据,以实现更严格的合规性、更高效率和更低风险。
什么是大型语言模型(LLMs)?
-大型语言模型是一种人工智能或机器学习的变种,特别是使用神经网络,它们是非常擅长生成文本的数学模型。这意味着它们能够与人进行对话、生成论文,并且能以与问题上下文相关的方式进行,而且语法正确。
大型语言模型如何帮助数据治理?
-大型语言模型可以帮助数据治理通过:1. 分类数据,2. 汇总数据,3. 提供问答服务,4. 实体识别,5. 情感分析。这些功能可以用于标记数据、简化信息,并根据风险和治理要求采取相应的行动。
大型语言模型能否自动生成本体论?
-大型语言模型位于监督学习和无监督学习之间,它们可以在一定程度上自动生成本体论,但通过微调模型可以提高准确性。尽管它们能够生成英语基础的本体论,但提高特定本体论的精确度需要进行定制化训练。
大型语言模型在处理个人数据时可能面临的风险是什么?
-大型语言模型在处理个人数据时可能面临的风险包括偏见、数学幻觉、误导信息等。这些模型可能会反映它们所代表的数据的偏见,或者因为模型混淆而产生错误的联系和输出。
如何确保使用大型语言模型时考虑到批判性思维?
-使用大型语言模型时,需要培养用户的批判性思维能力,以判断模型提供的结果是否可信。这包括理解模型的局限性、识别可能的偏见,并在决策过程中综合考虑多个因素。
Record Point是如何利用大型语言模型改进数据治理的?
-Record Point利用大型语言模型通过分类和标记数据,理解数据的隐私背景,以及提供数据的控制和处理能力来改进数据治理。他们专注于数据清理、分类和企业内不同系统的数据控制。
大型语言模型能否直接应用GDPR本体论来构建政策和规则?
-大型语言模型本身不能直接应用GDPR本体论来构建政策和规则。它们可以提供关于如何应用GDPR的建议,但实际上实施特定的数据保护和隐私管理措施需要更专业的工具和流程。
大型语言模型的未来发展方向是什么?
-大型语言模型的未来发展方向预计将深入整合到各种技术和业务流程中,提高信息汇总、决策支持和数据处理的效率。它们将在未来的技术解决方案中扮演关键角色,影响从数据治理到客户服务等广泛领域。
安东尼·伍德沃德平时更倾向于使用哪个大型语言模型?
-安东尼·伍德沃德在使用大型语言模型时没有特定偏好,他在不同的情况下会根据需要选择使用Chat GPT或Bard。他认为这两个模型各有优缺点,并且随着模型的不断更新和进化,他的选择也可能会改变。
Outlines
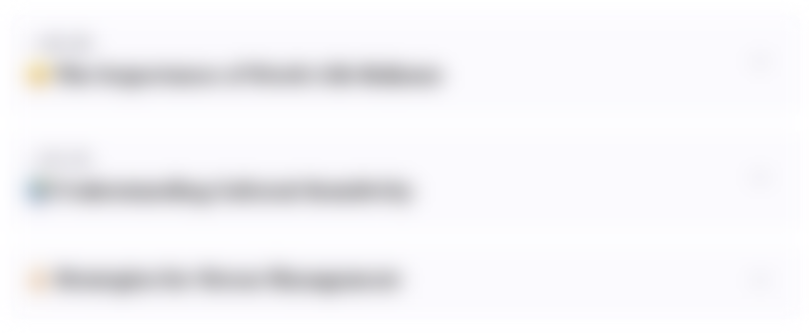
This section is available to paid users only. Please upgrade to access this part.
Upgrade NowMindmap
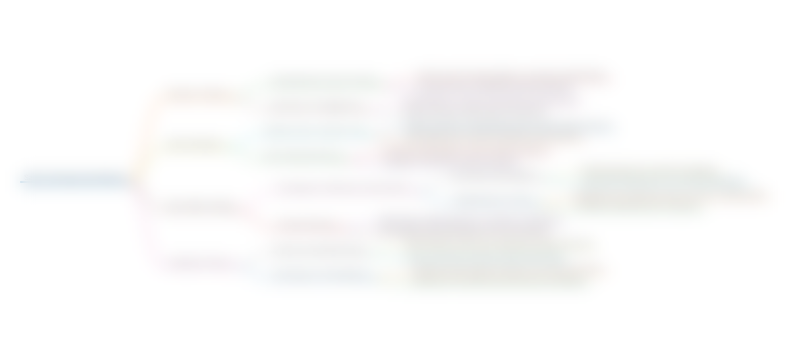
This section is available to paid users only. Please upgrade to access this part.
Upgrade NowKeywords
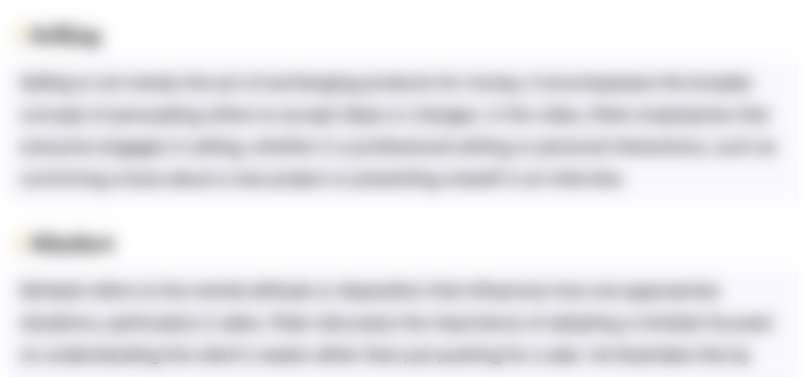
This section is available to paid users only. Please upgrade to access this part.
Upgrade NowHighlights
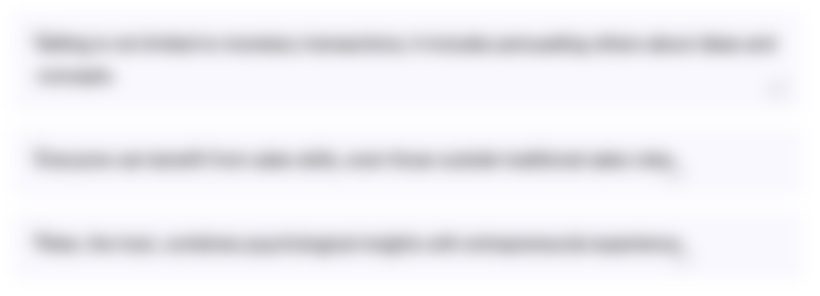
This section is available to paid users only. Please upgrade to access this part.
Upgrade NowTranscripts
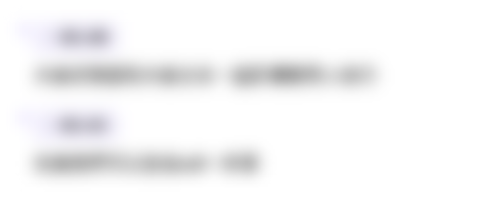
This section is available to paid users only. Please upgrade to access this part.
Upgrade NowBrowse More Related Video
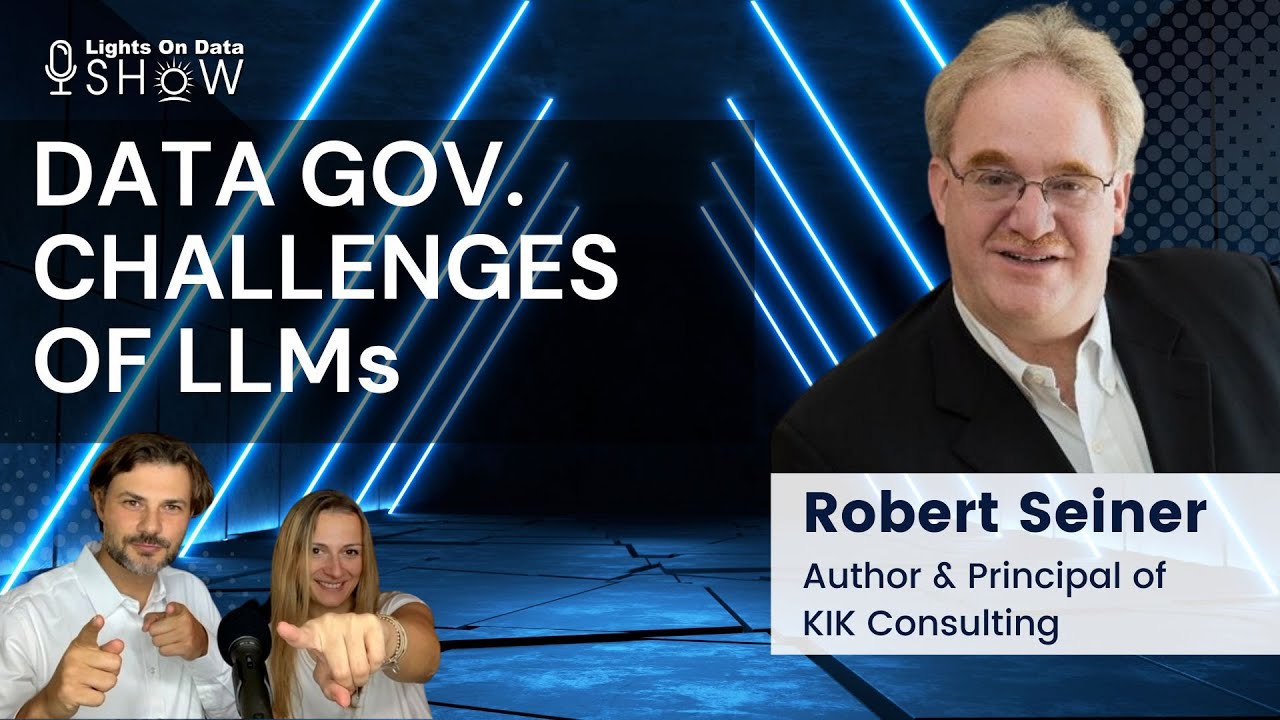
The Data Governance Challenges of LLMs
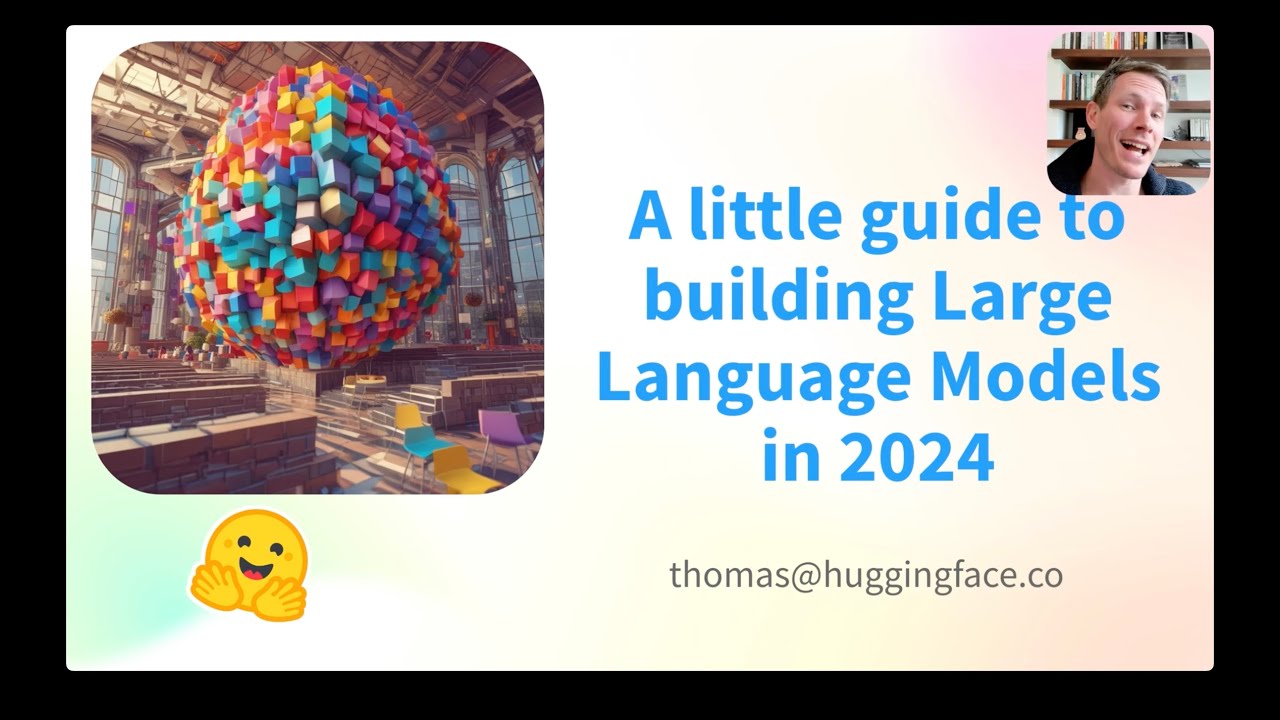
A little guide to building Large Language Models in 2024

How Did Dario & Ilya Know LLMs Could Lead to AGI?
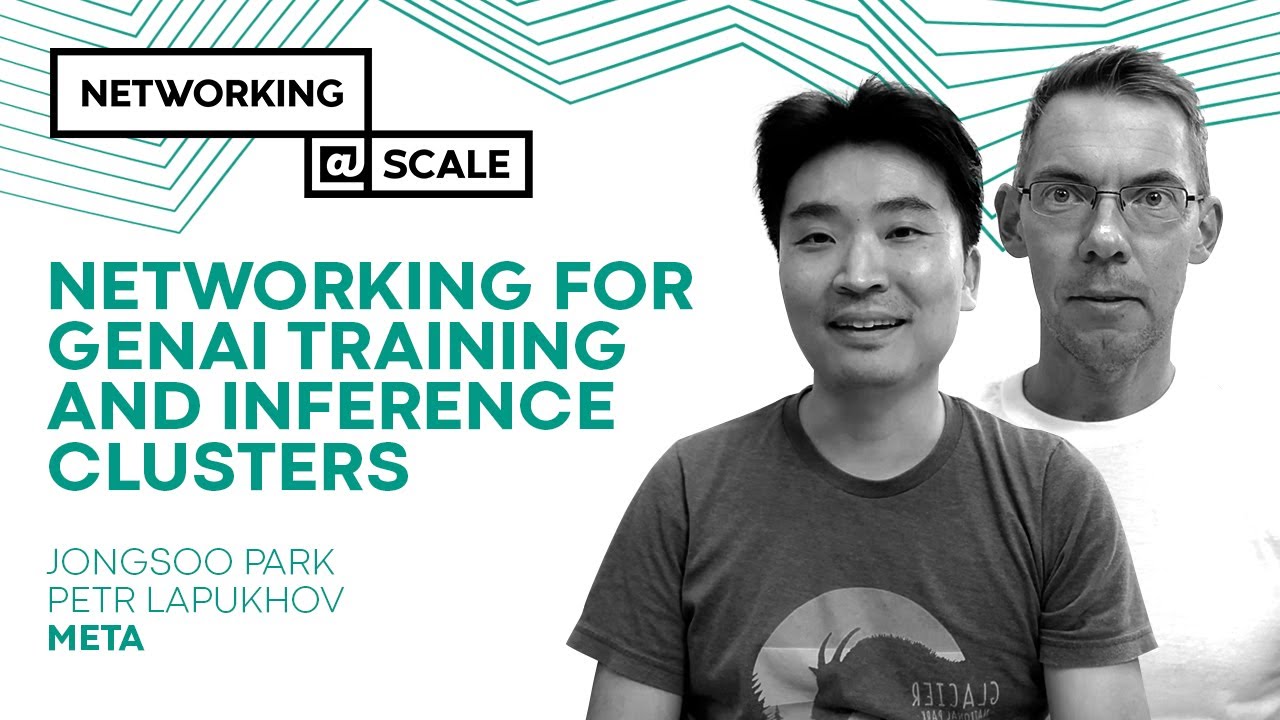
Networking for GenAI Training and Inference Clusters | Jongsoo Park & Petr Lapukhov
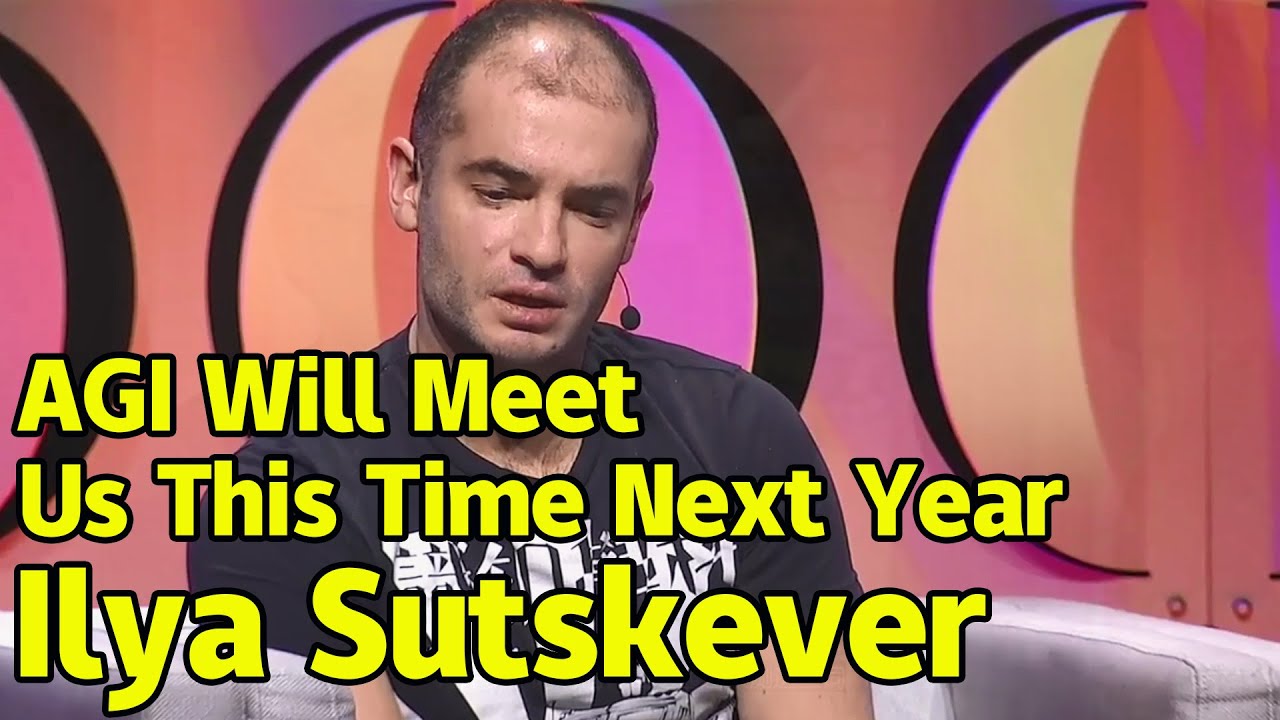
Ilya Sutskever | The birth of AGI will subvert everything |AI can help humans but also cause trouble

Stream of Search (SoS): Learning to Search in Language
5.0 / 5 (0 votes)