Key Machine Learning terminology like Label, Features, Examples, Models, Regression, Classification
Summary
TLDRThis video introduces the topic of using machine learning for predictive modeling and data analysis. It covers concepts like regression, classification, and label prediction. The speaker highlights practical examples, such as using machine learning to predict product prices based on features and data trends. The video also discusses the relationships between data features, model training, and validation. The content touches on fundamental machine learning terms and encourages viewers to subscribe for more content on data science, prediction models, and related topics.
Takeaways
- ๐ The video introduces the concept of using machine learning for people and positive west, starting with a basic example of machine learning recognizing bird species from pencil drawings.
- ๐ The script emphasizes the importance of labeling data correctly, which is crucial for the machine learning model to learn effectively.
- ๐ It discusses the use of models to predict the future forms of products based on their features, and how different features can influence the prediction of product prices.
- ๐ The video mentions the creation of a new model and the process of training and production, highlighting the role of data in building these models.
- ๐ฌ There's a focus on the relationship between teacher and label, suggesting that understanding this relationship can help in improving the model's predictions.
- ๐ The script talks about the process of feature engineering, which involves transforming raw data into features that better represent the underlying patterns.
- ๐ It briefly touches on regression models, which are used for predicting continuous outcomes, and the importance of training these models with the right data.
- ๐ The video script includes an invitation for viewers to subscribe to the channel for more content on machine learning and related topics.
- ๐ There's a mention of using data to build relationships and make predictions, such as predicting the price of a product based on its features.
- ๐ The script also covers the idea of using machine learning to categorize and reduce features, which can help in simplifying the data for better model performance.
Q & A
What is the main topic of the video?
-The main topic of the video is about machine learning, specifically using machine learning for people and positive west, with a focus on machine learning reminders, drawing, and art.
What does the speaker encourage the audience to do at the beginning of the video?
-The speaker encourages the audience to subscribe to the channel, press the notification bell, and engage with the content by liking and commenting.
What is the purpose of the 'subscribe' and 'subscribe the Channel' phrases mentioned in the script?
-The phrases are used to remind viewers to subscribe to the YouTube channel to receive updates and notifications about new content.
What is the significance of 'labels' and 'cross-validation' in the context of the video?
-Labels are used to categorize data, and cross-validation is a technique for assessing the performance of machine learning models, ensuring that the model is robust and generalizes well to new data.
What does the speaker mean by 'simple machine learning problem'?
-A 'simple machine learning problem' refers to a basic task in machine learning that involves training an algorithm to make predictions or decisions based on input data.
How does the speaker discuss the future forms of product features in the video?
-The speaker talks about the future forms of product features by mentioning the use of machine learning to predict the future price of products and the factors that influence it.
What is the role of 'data' in building new models as mentioned in the script?
-Data plays a crucial role in building new models. It is used to train and validate the models, helping to improve their accuracy and effectiveness in making predictions.
What does the speaker suggest about the importance of understanding the relationship between features and labels in machine learning?
-The speaker emphasizes the importance of understanding the relationship between features and labels because it helps in building more accurate models that can make better predictions.
How does the speaker approach the topic of 'regression' and 'classification' models?
-The speaker discusses regression and classification models as types of machine learning models used for different types of predictions. Regression models predict continuous values, while classification models predict discrete categories.
What is the significance of the 'volume feature' mentioned in the script?
-The 'volume feature' is significant as it is one of the factors that can be used to train machine learning models, helping them to make predictions based on the volume of data or products.
What does the speaker mean by 'transitive verb' and 'building relationship between what is' in the context of machine learning?
-The speaker uses 'transitive verb' to refer to verbs that require an object to complete their meaning, and 'building relationship between what is' likely refers to establishing connections between different data points or features within a dataset.
Outlines
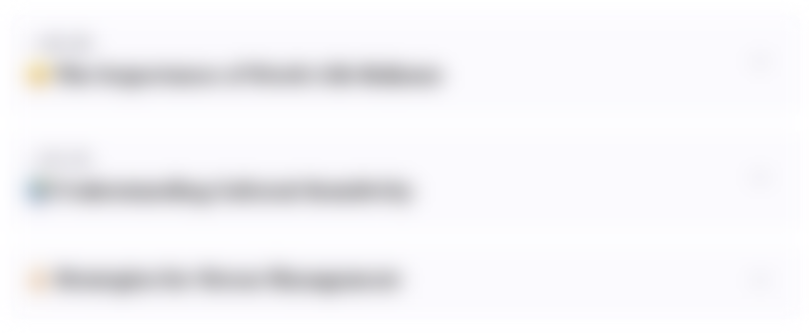
This section is available to paid users only. Please upgrade to access this part.
Upgrade NowMindmap
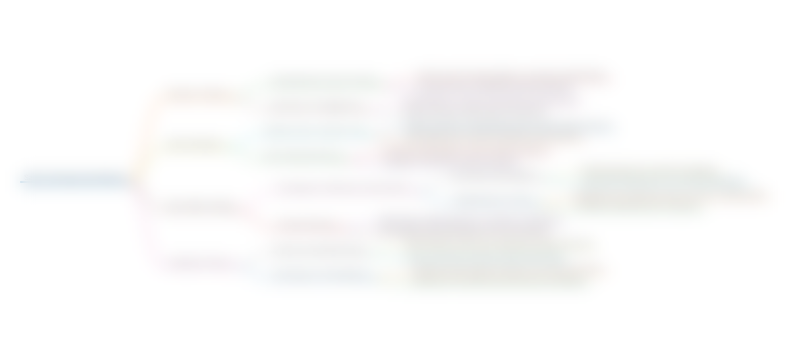
This section is available to paid users only. Please upgrade to access this part.
Upgrade NowKeywords
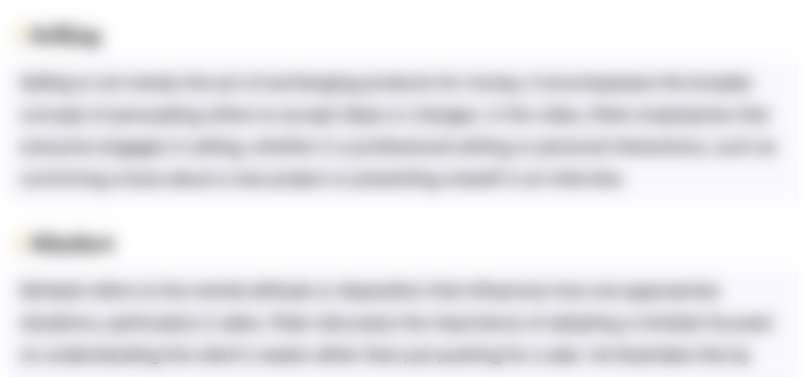
This section is available to paid users only. Please upgrade to access this part.
Upgrade NowHighlights
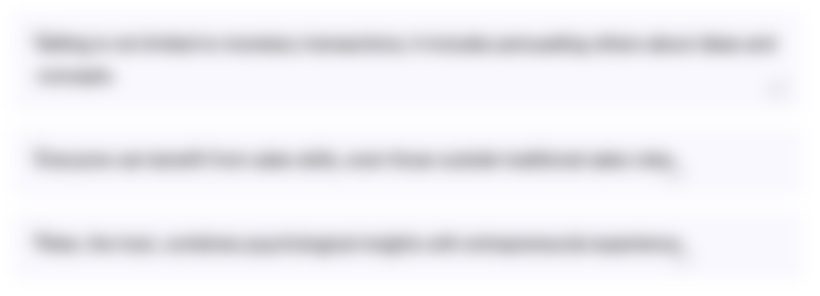
This section is available to paid users only. Please upgrade to access this part.
Upgrade NowTranscripts
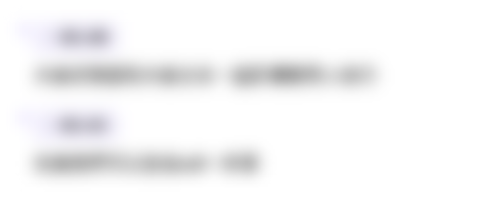
This section is available to paid users only. Please upgrade to access this part.
Upgrade NowBrowse More Related Video
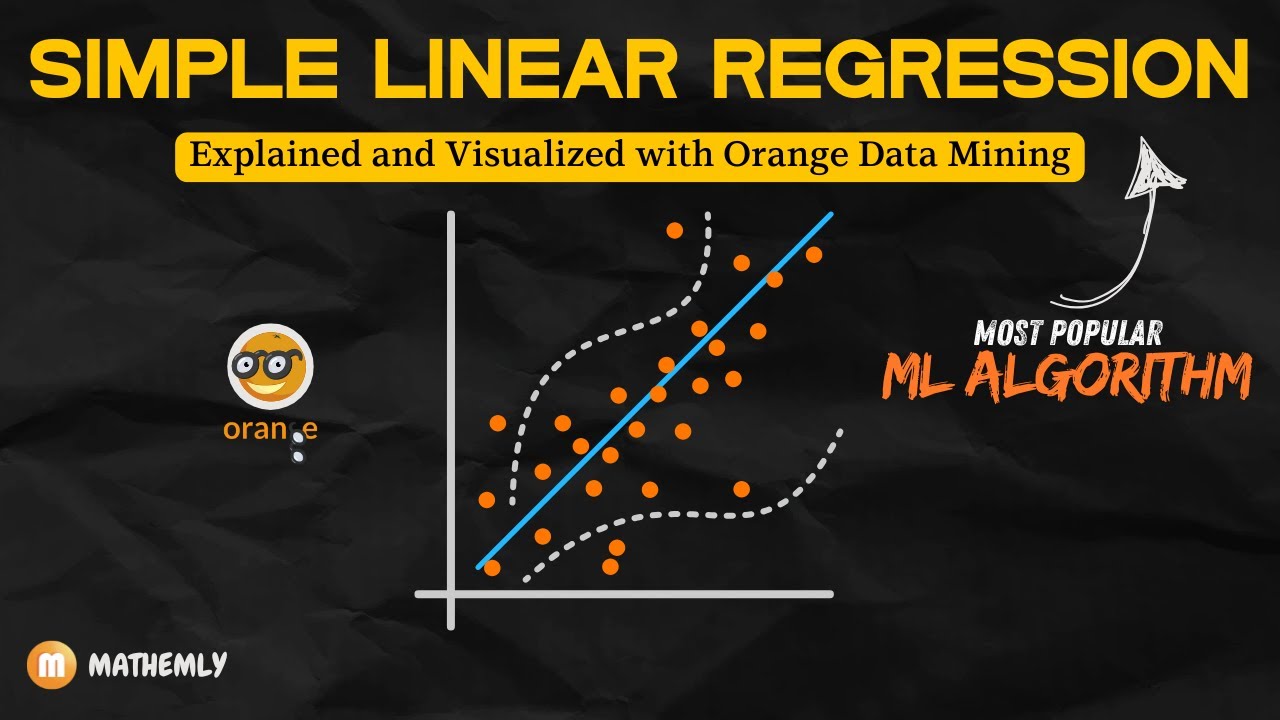
Simple Linear Regression Simplified | Orange Data Mining Tutorial
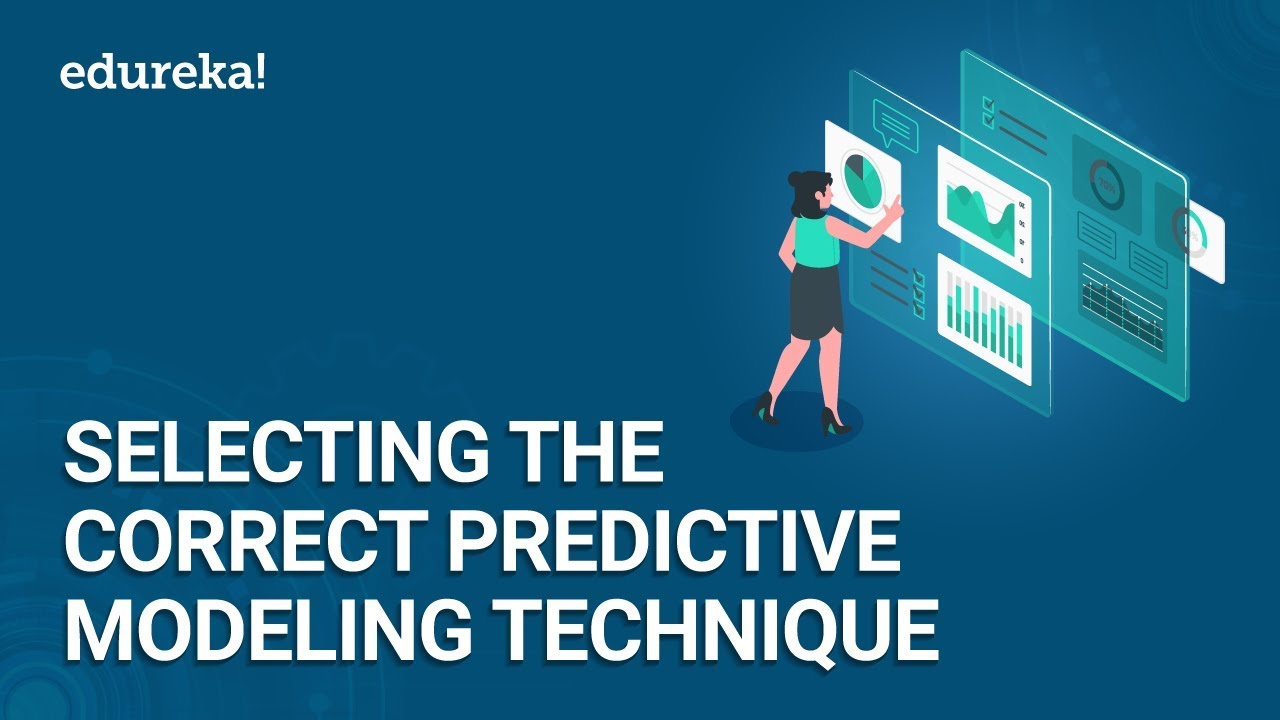
How to Select the Correct Predictive Modeling Technique | Machine Learning Training | Edureka
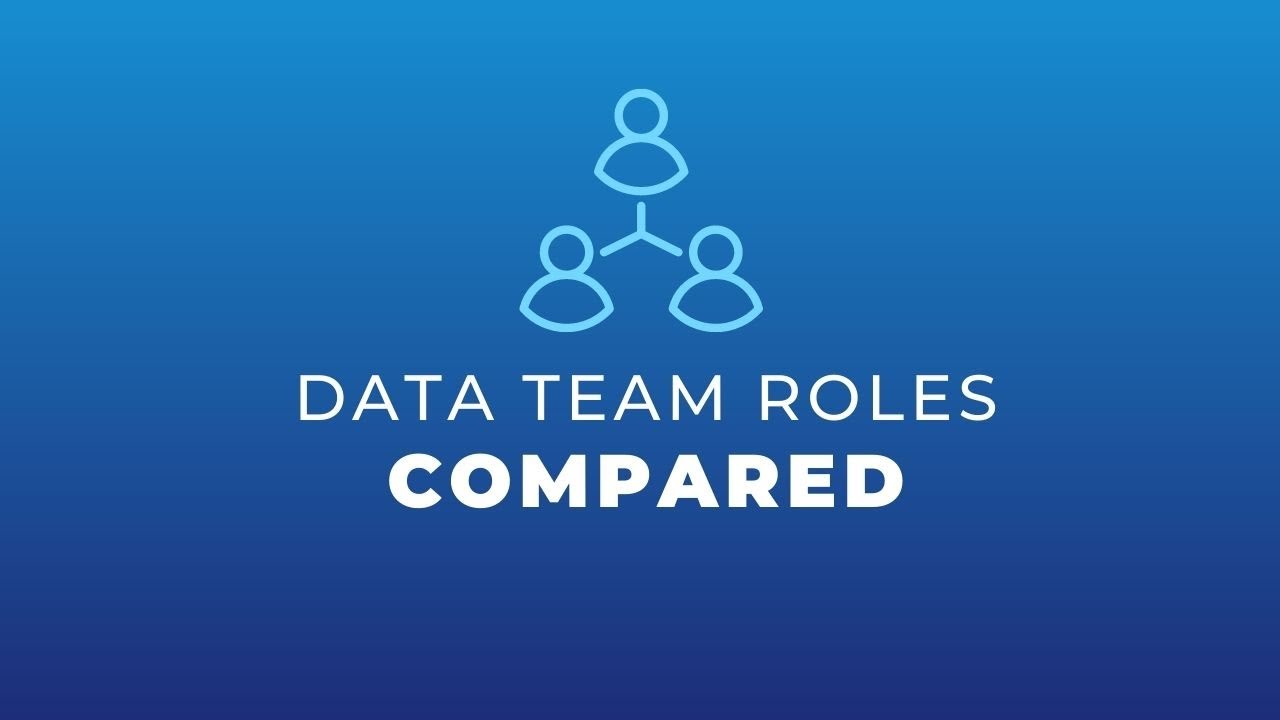
Common Data Team Structures (Engineer vs Analyst vs Scientist)
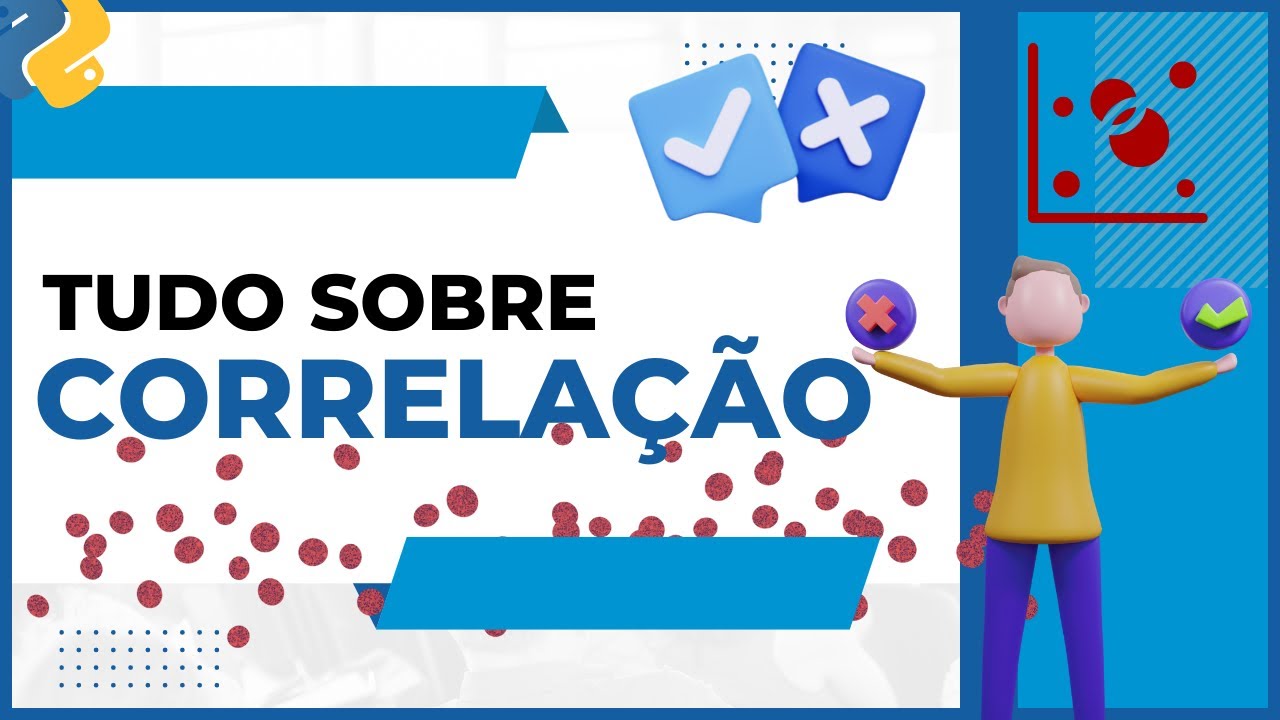
O que รฉ CORRELAรรO e como analisar os Grรกficos
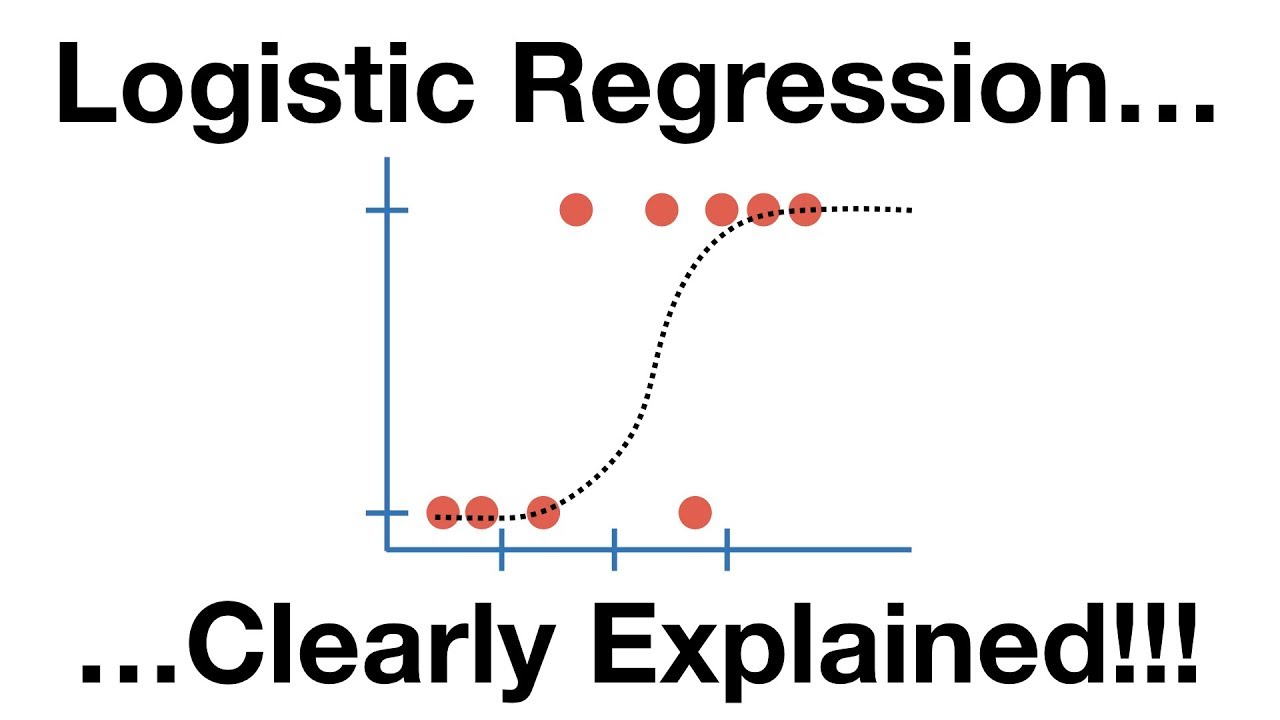
StatQuest: Logistic Regression
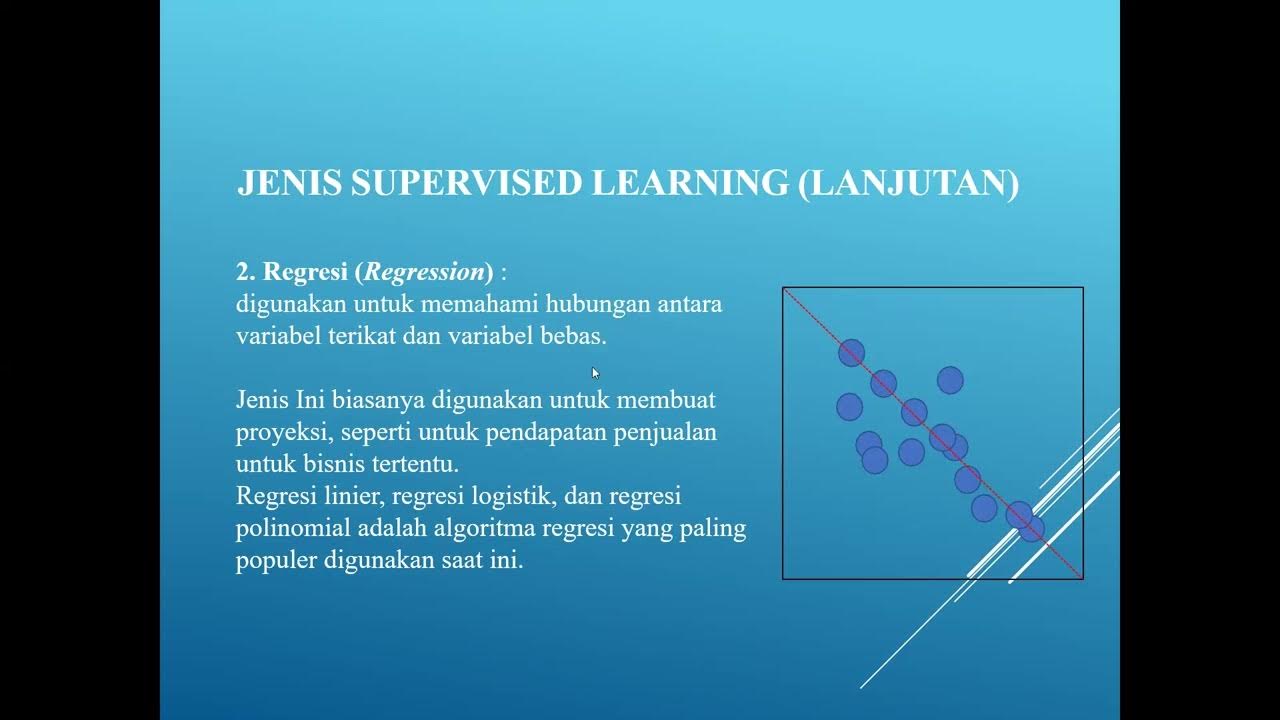
Pengantar Data sains Pert. 4
5.0 / 5 (0 votes)