Algebra 5.1 (Version A) - Exponential Growth
Summary
TLDRIn this educational video, Aiden Gonzalez explores the exponential growth of diseases, using COVID-19 as a case study. He explains how the virus spread rapidly due to its ability to infect multiple people, leading to a significant increase in cases over a short period. The video delves into the mathematics of exponential growth, illustrating the concept with a simple multiplication model and real-world data. It also discusses the importance of public health measures like 'flattening the curve' to reduce infection rates and prevent healthcare systems from being overwhelmed. The video concludes with a discussion on the impact of various government mandates on infection rates and their potential to save lives, encouraging viewers to consider the trade-offs of different public health strategies.
Takeaways
- 📚 The video script is an educational piece discussing exponential growth in the context of disease spread, specifically COVID-19.
- 📈 The script uses a simple mathematical model to illustrate how diseases can spread exponentially, doubling the number of cases with each passing day.
- 🗓 It outlines the early timeline of COVID-19 in the United States, highlighting key dates and milestones in the pandemic's escalation.
- 📊 The script includes a graph to visually represent the exponential growth of COVID-19 cases over time, showing an initial slow growth that accelerates.
- 🧮 Mathematical modeling is used to predict the spread of the virus, with the formula y = a * b^x where x is the number of days, and b is the growth rate.
- 🌐 The script references real-world data from 'Our World in Data' to demonstrate the exponential growth pattern observed in the early stages of the pandemic.
- 🔢 The concept of 'flattening the curve' is introduced as a public health strategy to slow the spread of the virus and prevent healthcare systems from becoming overwhelmed.
- 🏥 The script discusses the impact of public health measures such as mask mandates and social distancing on reducing the infection rate and saving lives.
- 🤔 It poses thought-provoking questions about the trade-offs between public health measures and their economic and social implications, encouraging critical thinking about policy decisions.
- 🌟 The script concludes by emphasizing the importance of mathematical models in predicting and managing the spread of diseases like COVID-19.
Q & A
What is the main topic discussed in Aiden Gonzalez's video?
-The main topic discussed is the spread of disease, specifically COVID-19, and how exponential growth models can be used to understand and predict the spread.
What is the significance of the timeline of COVID-19 in the United States presented in the video?
-The timeline is significant as it shows the rapid escalation of cases from the first reported case to widespread infection, illustrating the concept of exponential growth.
How does the video explain the concept of exponential growth in relation to virus spread?
-The video explains exponential growth by demonstrating how an infected individual can spread the virus to two new people, who then each spread it to two more, and so on, doubling the number of new infections each day.
What is the mathematical representation of exponential growth used in the video?
-The mathematical representation used is y = a * b^x, where 'x' represents days, 'y' represents the number of new infections, 'a' is the starting value (y-intercept), and 'b' is the growth rate multiplier.
What was the first case of COVID-19 in the United States according to the video?
-The first case of COVID-19 in the United States was reported in Washington state on January 21st.
How does the video demonstrate the rate of change in the number of new infections over time?
-The video shows that initially, the rate of change is slow, but as time progresses, the rate of new infections accelerates, highlighting the exponential nature of the spread.
What role do public health measures play in the video's discussion on disease spread?
-Public health measures are discussed as a way to 'flatten the curve' by reducing the infection rate, thereby slowing the spread of the disease and preventing healthcare systems from becoming overwhelmed.
How does the video use the concept of 'flattening the curve' in relation to COVID-19?
-The video uses 'flattening the curve' to illustrate the importance of reducing the rate of infection to keep the number of cases below hospital capacity, thus saving lives and reducing the strain on healthcare systems.
What is the significance of the death rate in the context of the video's discussion on COVID-19?
-The death rate is significant as it helps to estimate the potential loss of life from the disease. The video uses this rate to predict the number of deaths based on the number of new infections.
How does the video address the balance between public health measures and economic impact?
-The video addresses this balance by presenting hypothetical scenarios where stricter measures like outdoor dining bans could further reduce infection rates but also have significant economic consequences, prompting viewers to consider the trade-offs.
Outlines
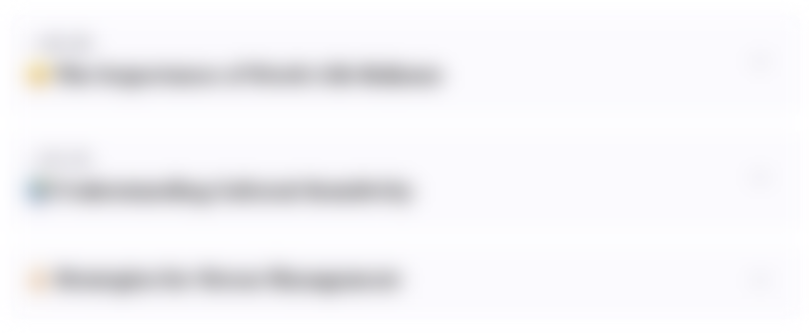
This section is available to paid users only. Please upgrade to access this part.
Upgrade NowMindmap
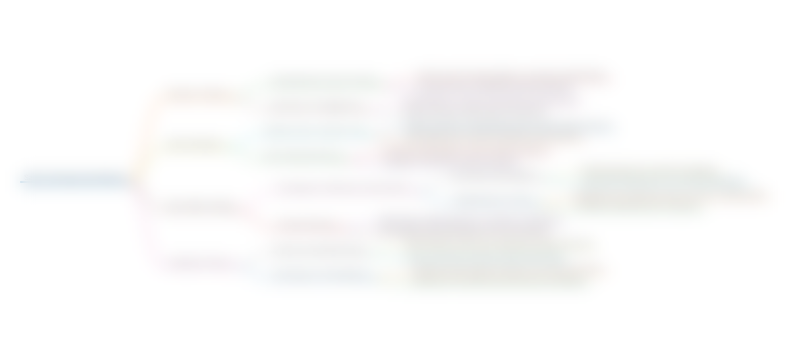
This section is available to paid users only. Please upgrade to access this part.
Upgrade NowKeywords
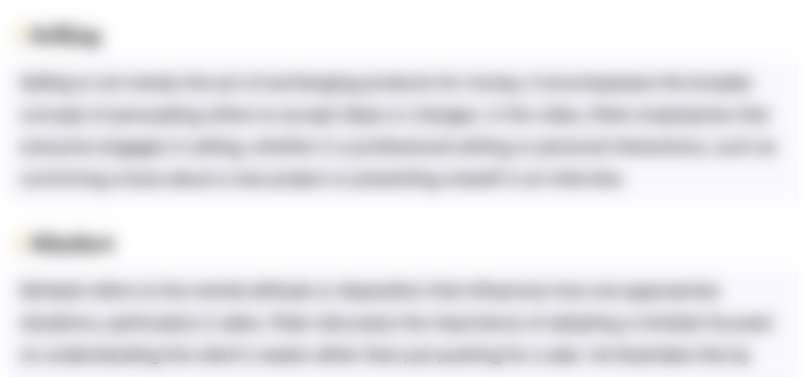
This section is available to paid users only. Please upgrade to access this part.
Upgrade NowHighlights
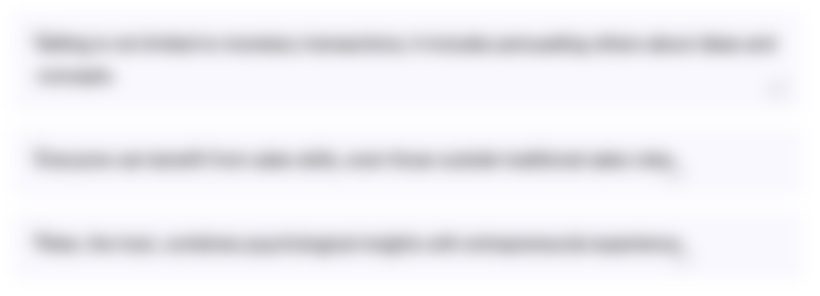
This section is available to paid users only. Please upgrade to access this part.
Upgrade NowTranscripts
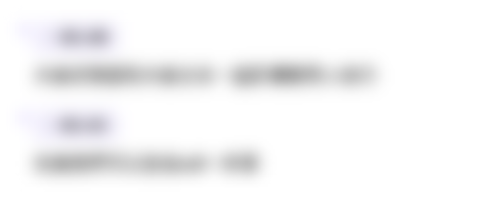
This section is available to paid users only. Please upgrade to access this part.
Upgrade NowBrowse More Related Video

Fungsi Eksponen Matematika Peminatan Kelas X - Apersepsi Masalah COVID-19
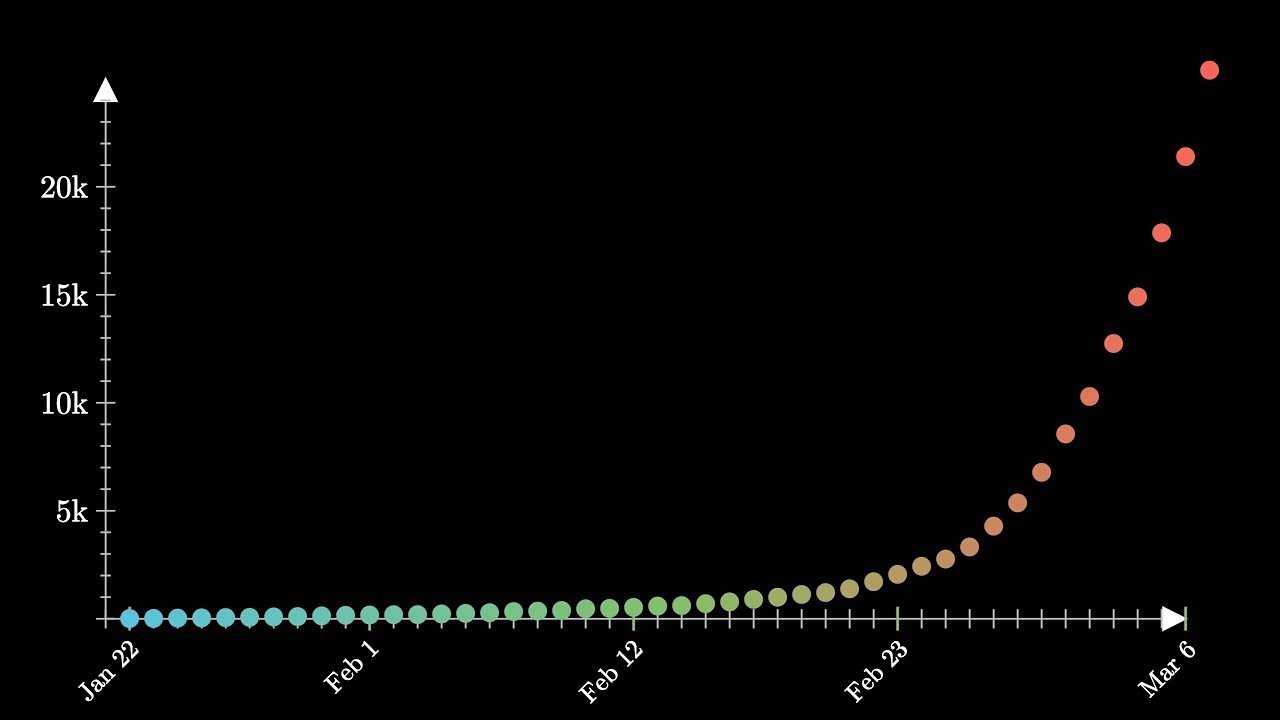
Exponential growth and epidemics

Are there Challenges to Monetary Policies?

China’s Covid Crime and Sinister Cover-Up Exposed | Shocking New Report | Gravitas | World News
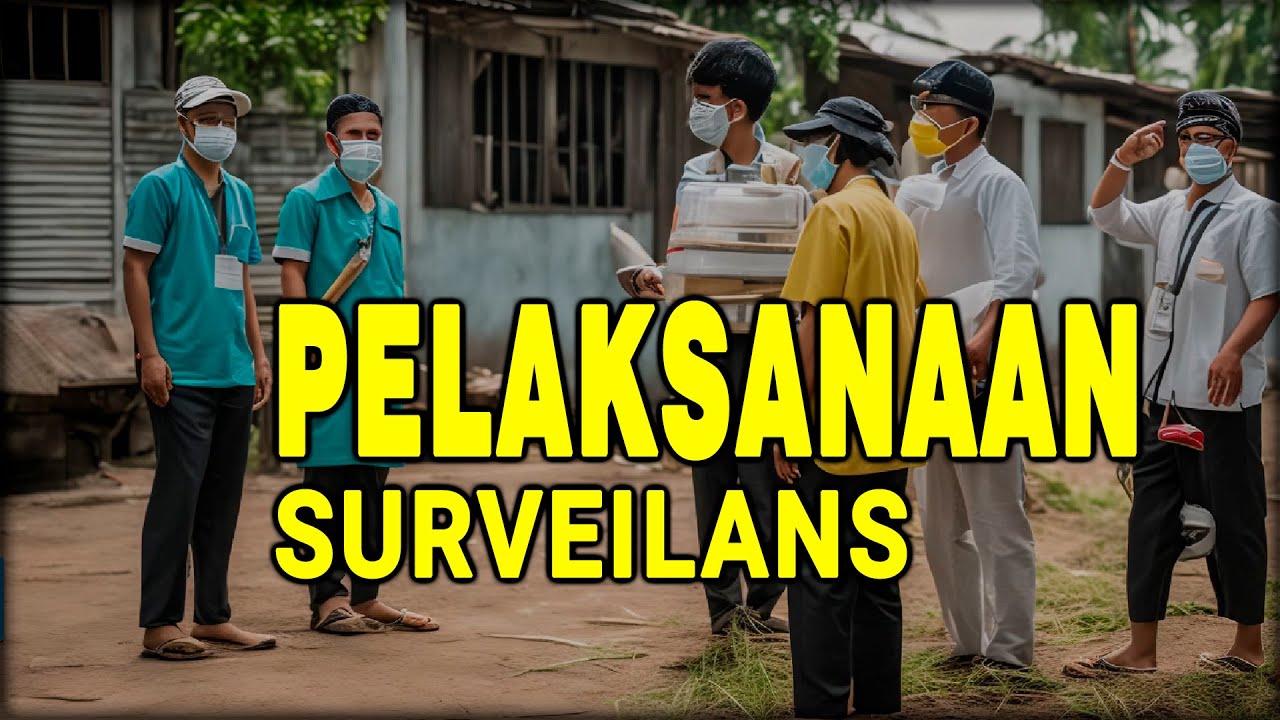
PELAKSANAAN SURVEILANS

Yuk Jaga kebersihan diri dan lingkungan
5.0 / 5 (0 votes)