How OpenAI Strawberry Works ― "ONE TEXTBOOK TO RULE THEM ALL" ― My Educated Guess
Summary
TLDRThe speaker discusses the capabilities of 'Strawberry', a rumored AI project from OpenAI, which may utilize synthetic data and latent space activation to solve complex problems. They share their expertise in synthetic data and their previous work on similar concepts, suggesting that 'Strawberry' could be generating comprehensive textbooks by recursively interrogating and activating AI models, grading the output for quality. The video speculates on how this might be applied to train future AI models like GPT 5 or 6, with a focus on knowledge synthesis and refinement.
Takeaways
- 🍓 Strawberry, a rumored AI project from OpenAI, is speculated to solve complex math problems and utilize synthetic data for training.
- 📈 The speaker has been working on synthetic data generation for over two years and is considered a world-leading expert in the field.
- 🧠 Latent Space Activation is likened to how human brains connect dots during conversation, applying this concept to large language models.
- 🔍 The concept of 'Surface Plate' involves a combination of three models: an expert, an interrogator, and a grader, to extract and refine knowledge.
- 📚 The idea of recursively generating a 'textbook of everything' by using AI models to extract and synthesize human knowledge is introduced.
- 🤖 The process involves an expert model providing base information, an interrogator model asking for deeper insights, and a grader model assessing the output.
- 📈 Synthetic data, when highly curated, is found to be more efficient for training AI models, which is a key aspect of the 'Strawberry' project.
- 🔑 The grading process is crucial for refining the synthesized data, ensuring that only the highest quality information is retained.
- 🔄 The iterative process of questioning and answering can lead to the creation of comprehensive textbooks on various topics by AI.
- 🤝 The potential of multiple fine-tuned models working in parallel to unpack every domain of human knowledge is highlighted.
- 🤔 The speaker expresses uncertainty about how the 'Strawberry' project handles complex math, suggesting it might involve generating LaTeX formulas and logical unpacking.
Q & A
What is 'Strawberry' as mentioned in the script?
-Strawberry is a rumored feature or capability from OpenAI that is said to involve solving complex math problems and generating synthetic data for training models like Project Oion.
What does the speaker claim to be an expert in?
-The speaker claims to be a world-leading expert in synthetic data, having worked on it for a long time and shared insights openly.
What is Latent Space Activation?
-Latent Space Activation is a concept where a model's embedded knowledge is assembled and crystallized upon being asked a question it hasn't thought of before, similar to how human brains make new connections during conversation.
What is the speaker's previous work related to 'Strawberry'?
-The speaker had been working on a startup 18 months ago that involved developing a concept called 'Surface Plate,' which combined three models: a generator, an interrogator, and a grader, similar to the rumored workings of 'Strawberry'.
How does the speaker describe the process of creating synthetic data?
-The speaker describes the process as one where a model is iteratively drilled into topics to generate comprehensive information, which is then graded for quality and used to refine the data set.
What role does the 'interrogator' play in the process described?
-The 'interrogator' is a model that extracts all the information from the 'expert' model, prompting it to crystallize its knowledge on a given topic.
What is the purpose of the 'grader' in the process?
-The 'grader' evaluates the quality of the generated text based on a rubric, helping to ensure that only the highest quality information is retained in the data set.
How does the speaker relate the process to writing a textbook?
-The speaker likens the process to recursively writing a textbook of everything humans know, with models working in parallel to unpack every domain of human knowledge.
What is the speaker's speculation about how 'Strawberry' might handle math?
-The speaker is unsure about the specifics but suggests that 'Strawberry' might use a generator to write out LaTeX formulas and logically unpack them, similar to its approach with other types of knowledge.
What is the potential outcome of the process described by the speaker?
-The potential outcome is the creation of a comprehensive, high-quality data set that encapsulates a vast amount of human knowledge, which could be used for fine-tuning models like GPT-5 or GPT-6.
What is the speaker's final thought on the process and its implications?
-The speaker believes that if their understanding of the process is correct, it could represent a significant advancement in AI training and knowledge synthesis, potentially leading to the creation of a 'one data set to rule them all.'
Outlines
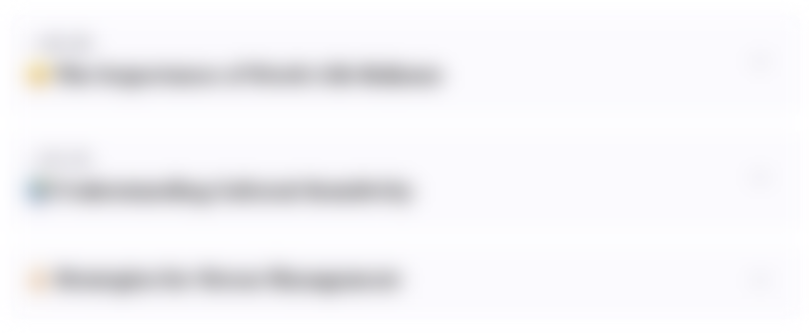
This section is available to paid users only. Please upgrade to access this part.
Upgrade NowMindmap
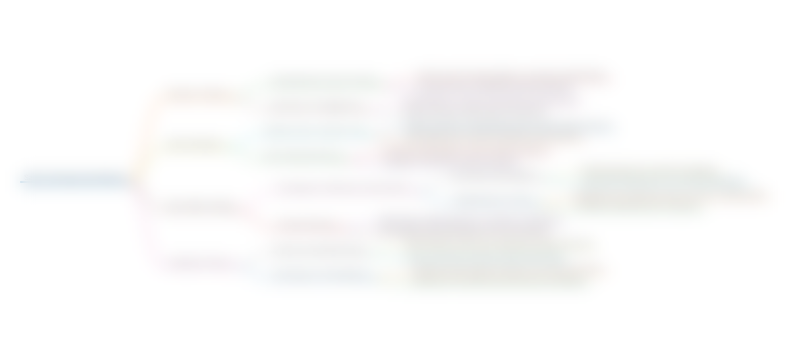
This section is available to paid users only. Please upgrade to access this part.
Upgrade NowKeywords
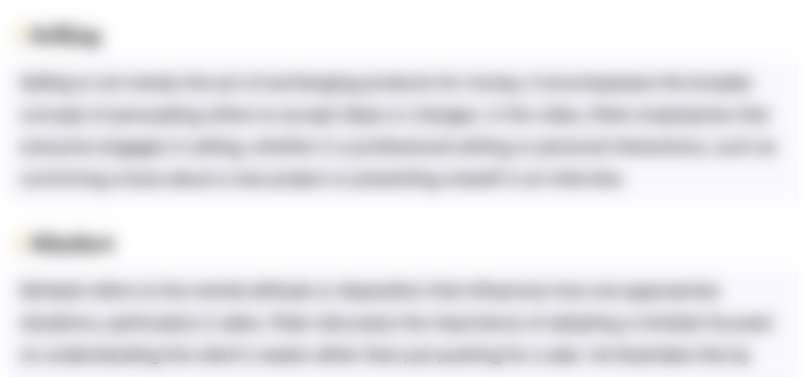
This section is available to paid users only. Please upgrade to access this part.
Upgrade NowHighlights
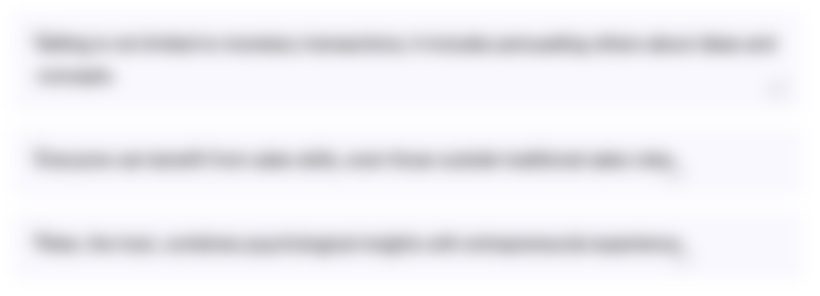
This section is available to paid users only. Please upgrade to access this part.
Upgrade NowTranscripts
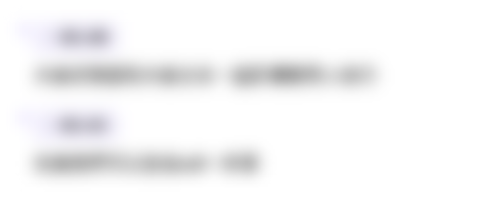
This section is available to paid users only. Please upgrade to access this part.
Upgrade NowBrowse More Related Video
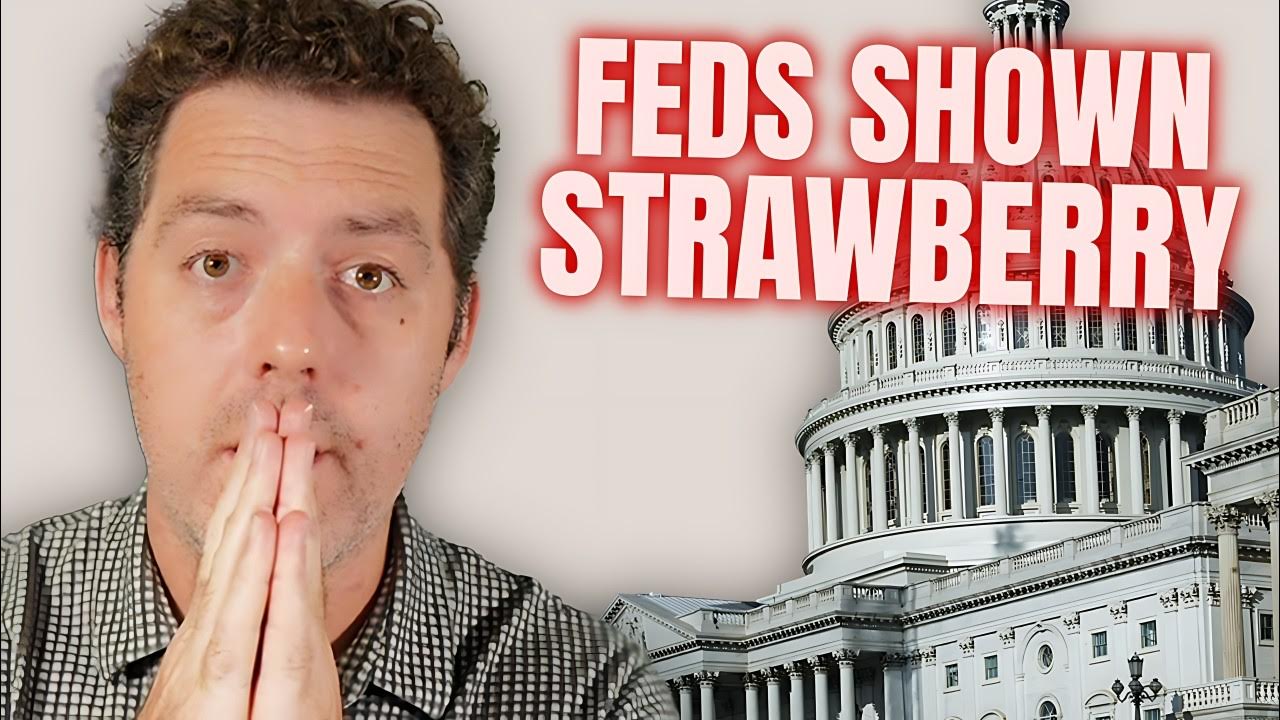
Project Orion (GPT-5 Strawberry) Imminent, Already Shown To FEDS!
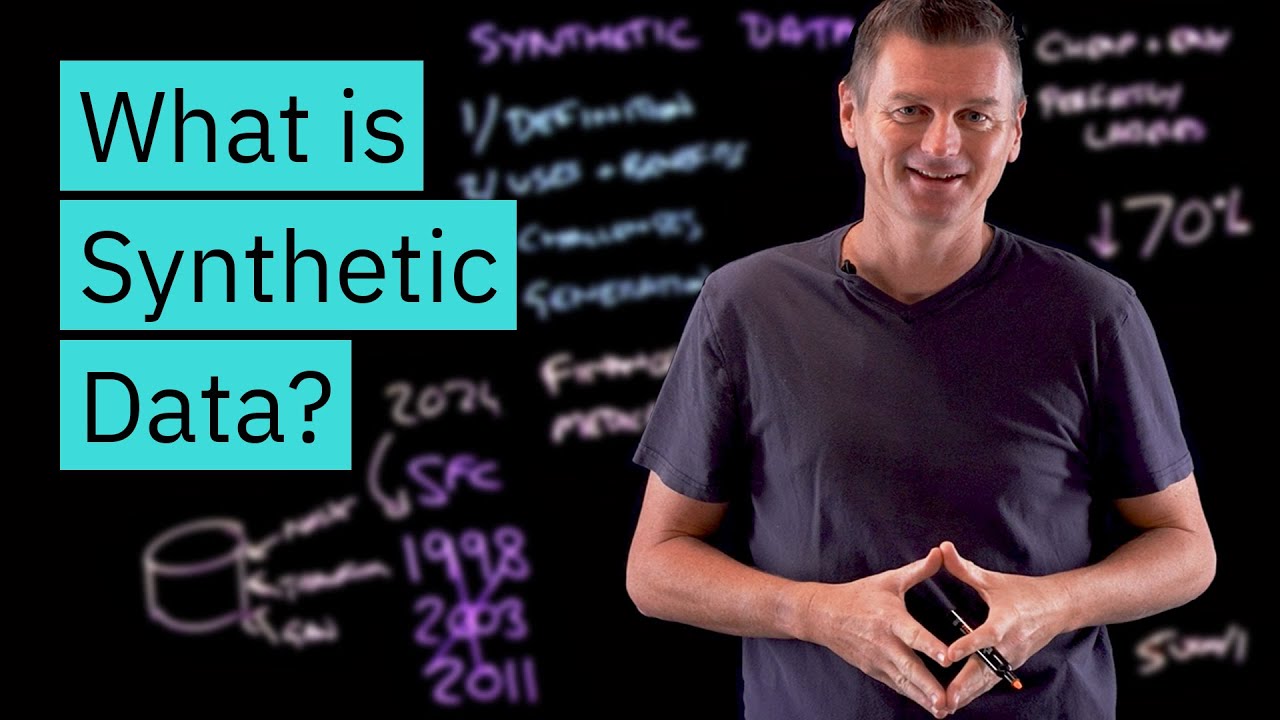
What is Synthetic Data? No, It's Not "Fake" Data
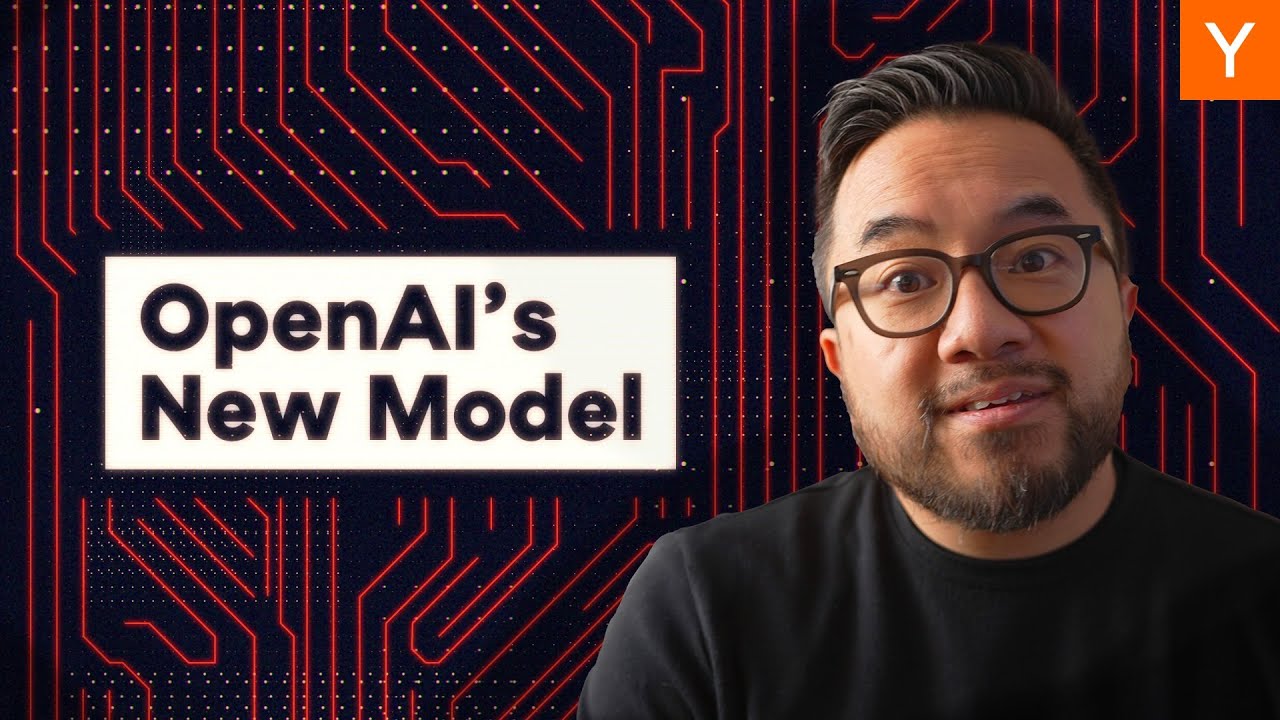
Why OpenAI's o1 Is A Huge Deal | YC Decoded
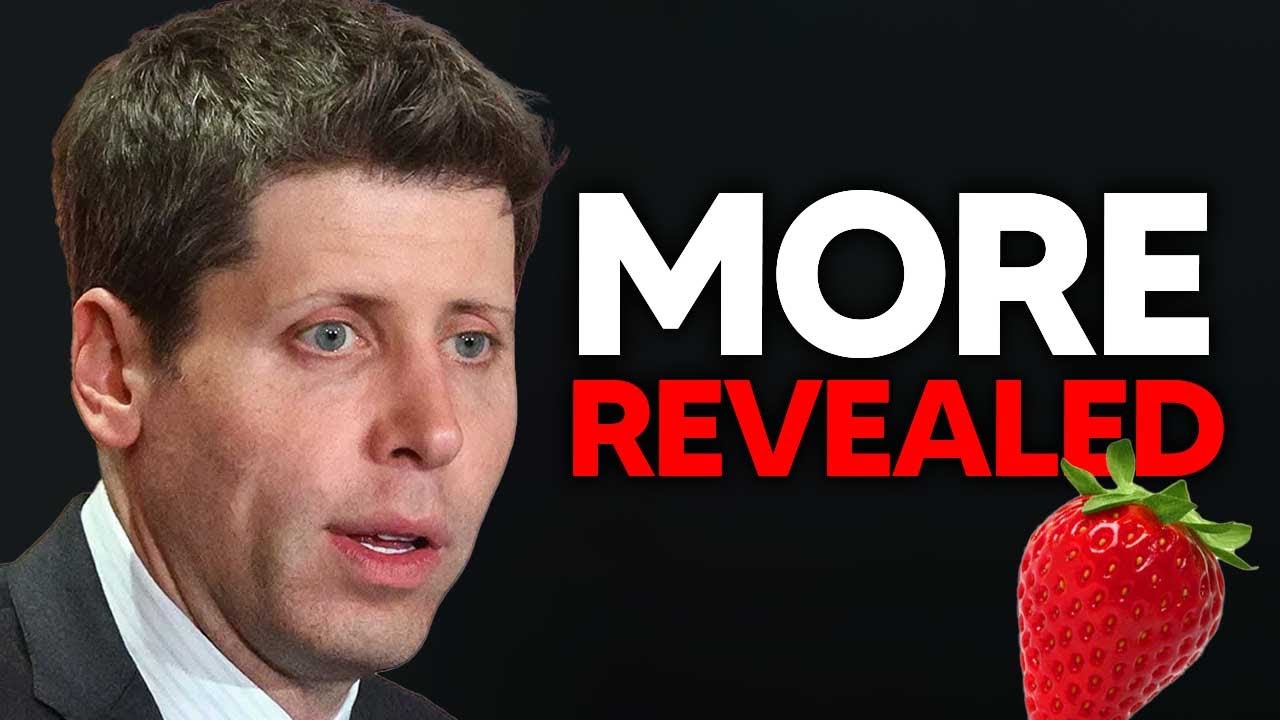
Stunning New OpenAI Details Reveal MORE! (Project Strawberry/Q* Star)
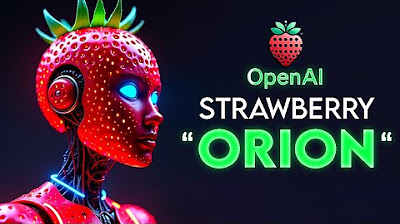
OpenAI ORION (GPT-5) Arrives with Strawberry AI This Fall: AGI Soon!
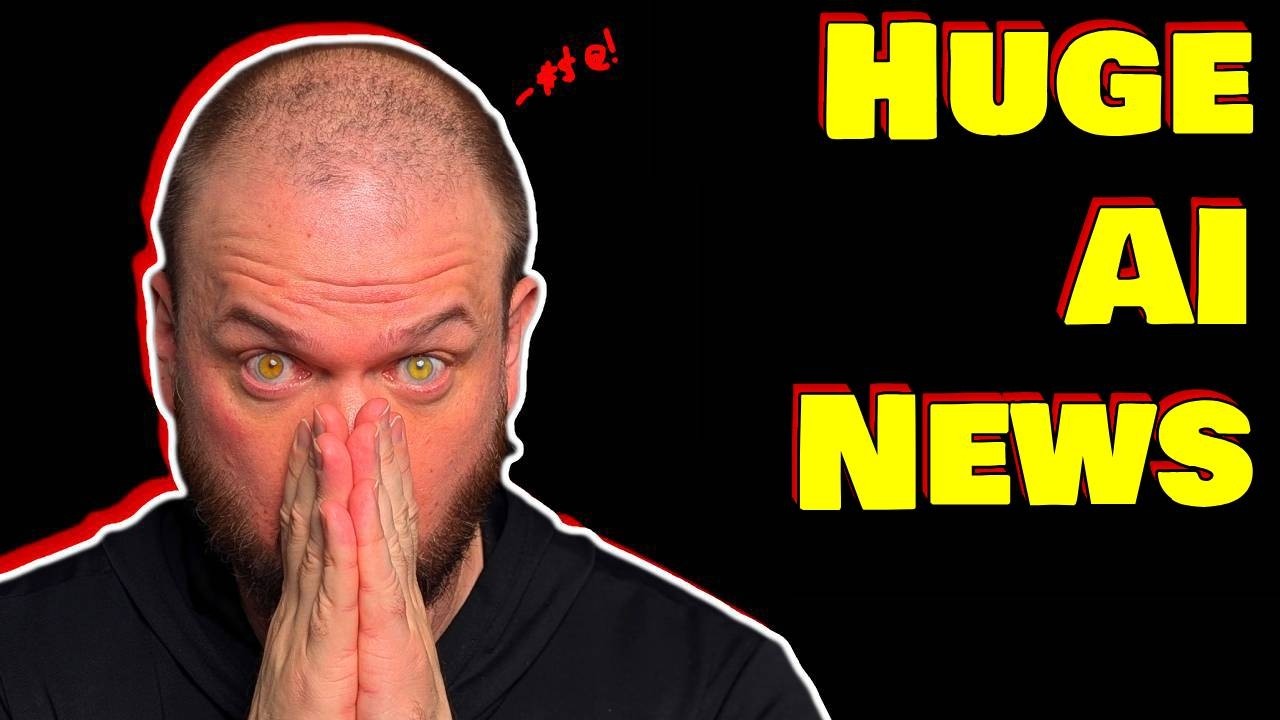
Sam Altman Teases Orion (GPT-5) 🍓 o1 tests at 120 IQ 🍓 1 year of PHD work done in 1 hour...
5.0 / 5 (0 votes)