Can AI Catch What Doctors Miss? | Eric Topol | TED
Summary
TLDRThe script explores groundbreaking advancements in biomedical and healthcare technology through the lens of Scripps Research's experiences, highlighting the transformative impact of AI, particularly AlphaFold by DeepMind, in protein structure prediction. It discusses how AI has revolutionized diagnostic accuracy, from identifying diseases through retinal images to improving medical imaging and pathology. The narrative also touches on the potential of transformer models and GPT-4 in enhancing healthcare delivery, reducing diagnostic errors, and liberating medical professionals from administrative tasks. The speaker shares compelling patient stories where AI significantly improved diagnostic outcomes, underscoring a future where AI empowers deeper patient-doctor connections and precision medicine.
Takeaways
- 💻 AlphaFold, developed by DeepMind, has significantly accelerated the process of determining the 3D structures of proteins from taking years to just minutes.
- 📈 The American Nobel Prize recognized the contributions of Demis Hassabis, John Jumper, and their team for AlphaFold, despite their admitted lack of understanding of the underlying transformer model.
- 🛠 AlphaFold's success has inspired advancements in predicting structures of not only proteins but also RNA, antibodies, and novel proteins not found in nature.
- 📱 Diagnostic errors in medicine are a major issue, with a Johns Hopkins study indicating they contribute to 800,000 American deaths or serious disabilities annually, a problem AI could help address.
- 👁 AI has shown remarkable accuracy in medical imaging, such as determining gender from retinal images with 97% accuracy, outperforming human experts in many areas.
- 🧪 Deep learning models have demonstrated the ability to detect health issues from retinal scans that are not visible to the human eye, predicting diseases such as Alzheimer's years in advance.
- 📊 Transformer models, like GPT-4, are setting new standards in AI's capability to process and understand complex data, including language, images, and speech.
- 📚 The implementation of AI in healthcare promises 'keyboard liberation,' improving patient-doctor interactions by reducing the clerical burden on healthcare providers.
- 📷 Moorfields Eye Hospital's use of a foundation model to predict various health outcomes from retinal images demonstrates the potential for comprehensive diagnostics from single AI models.
- 📝 ChatGPT and similar AI technologies have successfully diagnosed complex medical conditions, underscoring their potential to augment or even outperform human clinical judgment in challenging cases.
Q & A
What breakthrough allowed protein structure prediction to go from taking years to just minutes?
-The breakthrough was the work of AlphaFold, a derivative of DeepMind, which takes the one-dimensional amino acid sequence of a protein and can predict its 3D structure at the atomic level in just minutes. This used to take scientists years to determine experimentally.
What impact could more accurate protein structure prediction have on fields like RNA, antibodies, and genome analysis?
-More accurate protein structure prediction models like AlphaFold have inspired similar models for predicting RNA structures, antibody structures, and even detecting mutations across the genome. This could massively accelerate research and discovery in these fields.
Why does the speaker suggest the AlphaFold team should get an asterisk on their award?
-The speaker notes that the AlphaFold team of 30 scientists does not fully understand how their transformer model works. So he jokingly suggests they should get an asterisk on their award since the AI itself deserves some credit.
How could AI help reduce diagnostic medical errors?
-AI has shown it can analyze medical images as well as or better than experts, catching things human doctors miss. With enough accurate labeled training data, AI diagnostic tools could significantly reduce errors and improve health outcomes.
What remarkable diagnoses has AI made that stumped teams of human doctors?
-In one case, ChatGPT correctly diagnosed a boy with occulta spina bifida when 17 previous doctors missed it over 3 years. In another, it diagnosed limbic encephalitis in a patient incorrectly told she had long COVID. AI tools show real promise in augmenting human diagnostic capabilities.
How could transformer models like GPT help transform medicine?
-Transformer models can process huge datasets spanning text, images and speech. Applied to healthcare, they could help automate keyboard/data entry tasks, analyze patient history to assist diagnosis, generate notes from doctor-patient conversations, provide decision support, and more.
What is keyboard liberation in healthcare and why does it matter?
-Keyboard liberation refers to freeing doctors from having to do data entry and clerical work, letting them focus on patients. With AI handling notes, prescriptions, referrals etc. based on conversations, it improves doctor-patient relationships and outcomes.
How was the Moorfields eye disease prediction model different from previous disease detection AIs?
-Unlike previous models trained on images for single diseases, this open-source model was trained on 1.6 million eye images to predict likelihoods for 8 different eye conditions with one unified model. It demonstrates the expanded capabilities from transformer architectures.
What future positive impacts does the speaker foresee AI having on medicine?
-The speaker is excited about keyboard liberation freeing up doctor time, improved doctor-patient relationships as a result, AI assistance making diagnosis faster and more accurate, and transformed medical education so students learn up-to-date best practices augmented by AI.
What advice does the speaker give to a medical student about embracing the coming age of AI in medicine?
-He tells the student doctor that he's lucky to be practicing in an era with keyboard liberation, more time with patients, AI diagnostic assistance, and better patient care. But he notes AI still needs extensive validation before being deployed widely.
Outlines
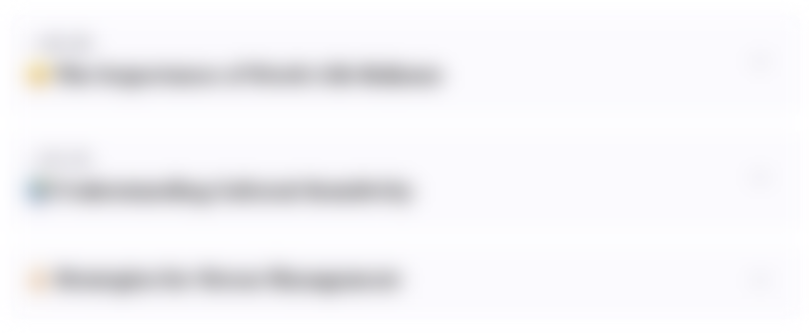
Этот раздел доступен только подписчикам платных тарифов. Пожалуйста, перейдите на платный тариф для доступа.
Перейти на платный тарифMindmap
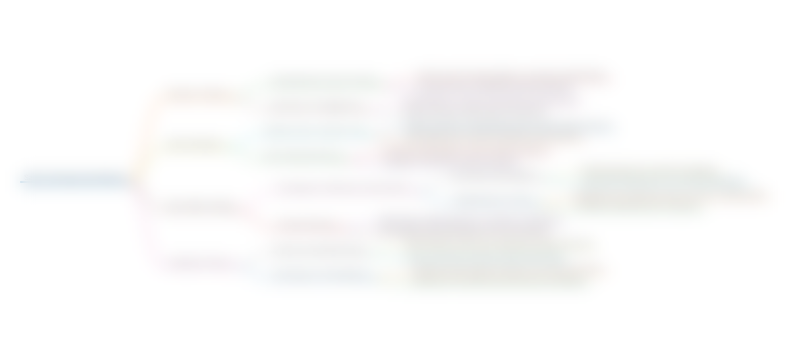
Этот раздел доступен только подписчикам платных тарифов. Пожалуйста, перейдите на платный тариф для доступа.
Перейти на платный тарифKeywords
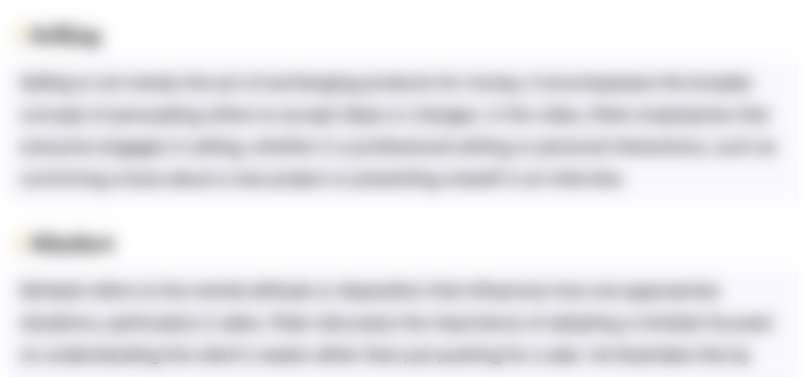
Этот раздел доступен только подписчикам платных тарифов. Пожалуйста, перейдите на платный тариф для доступа.
Перейти на платный тарифHighlights
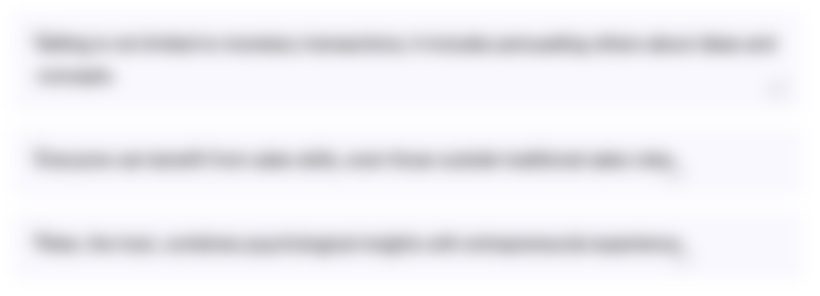
Этот раздел доступен только подписчикам платных тарифов. Пожалуйста, перейдите на платный тариф для доступа.
Перейти на платный тарифTranscripts
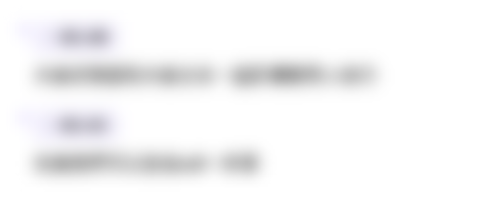
Этот раздел доступен только подписчикам платных тарифов. Пожалуйста, перейдите на платный тариф для доступа.
Перейти на платный тарифПосмотреть больше похожих видео
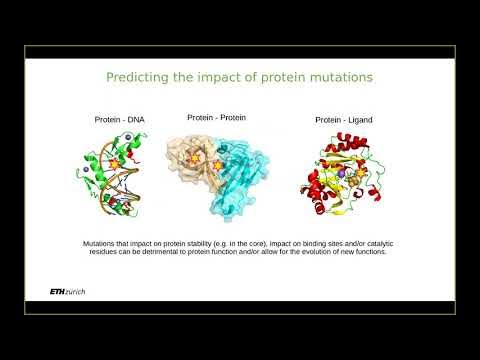
PTP - Structural Bioinformatics 3
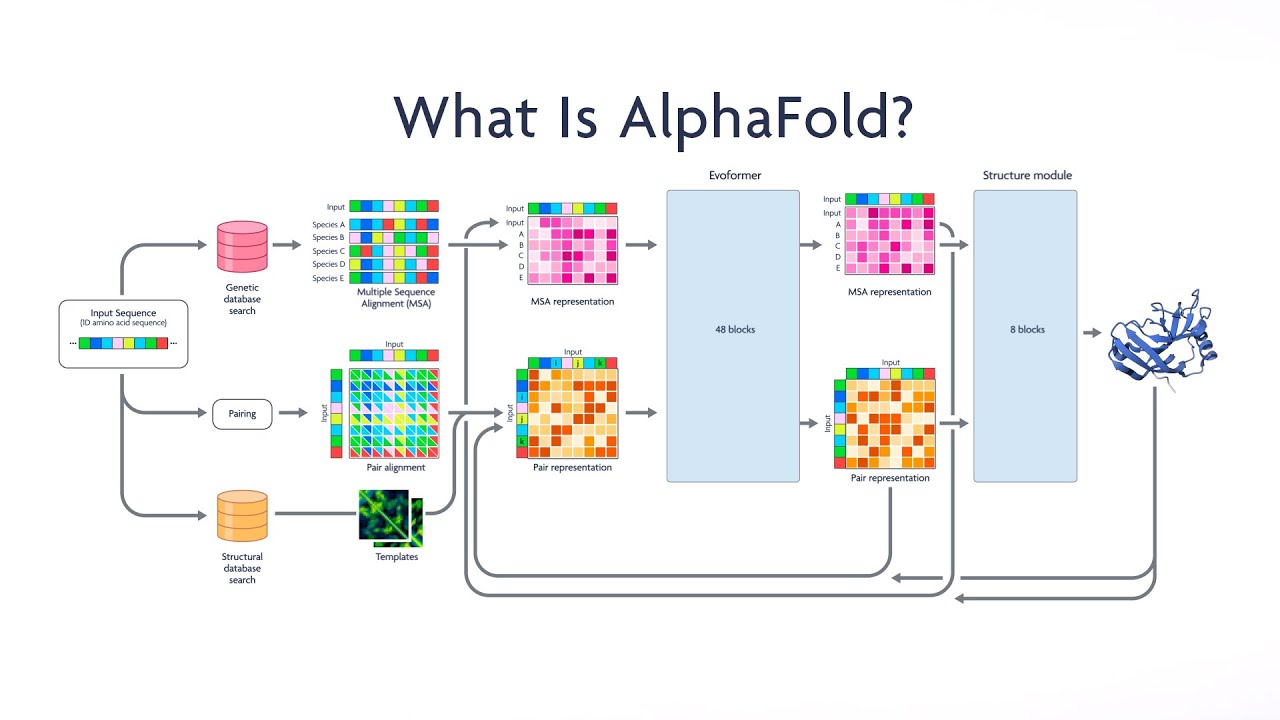
What Is AlphaFold? | NEJM
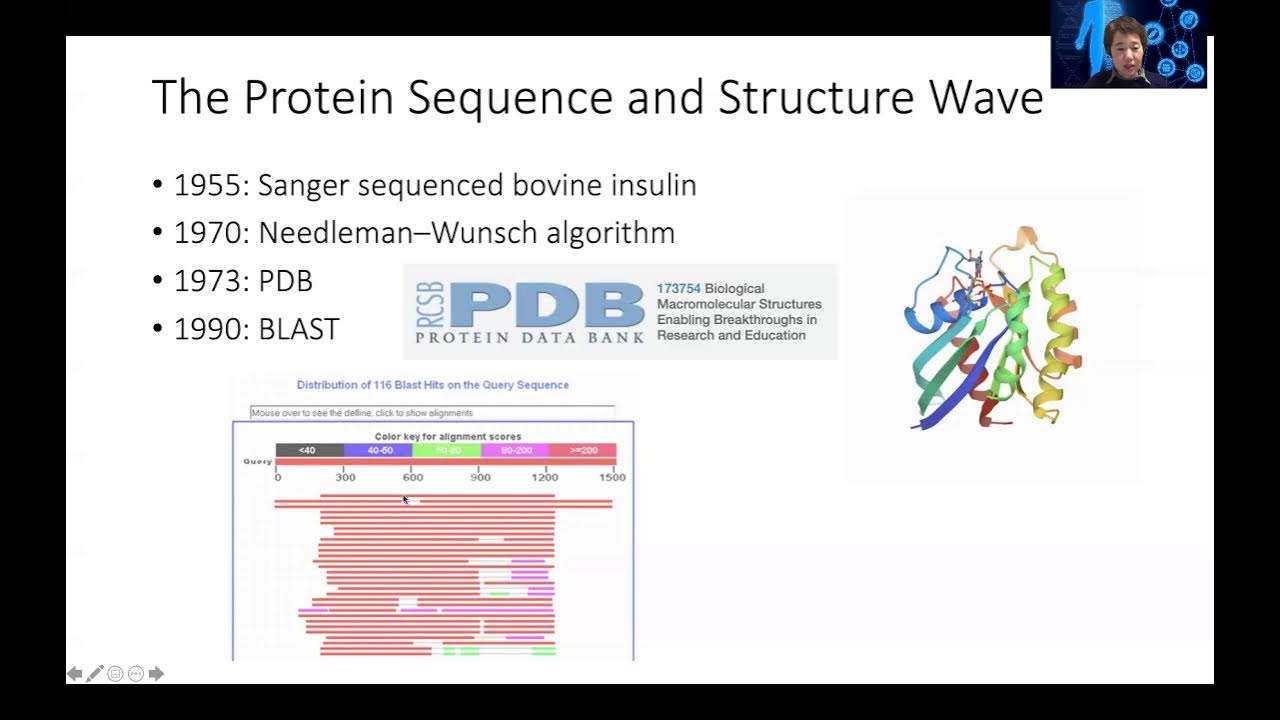
STAT115 Chapter 2.1 Protein Wave
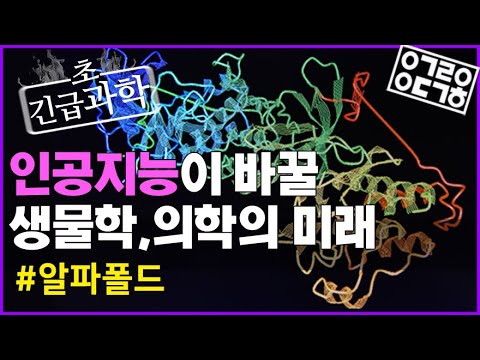
생물학 50년 난제 해결한 인공지능 '알파폴드', 생물학,의학 패러다임의 대전환?! [안될과학-초긴급과학]

How AI Cracked the Protein Folding Code and Won a Nobel Prize
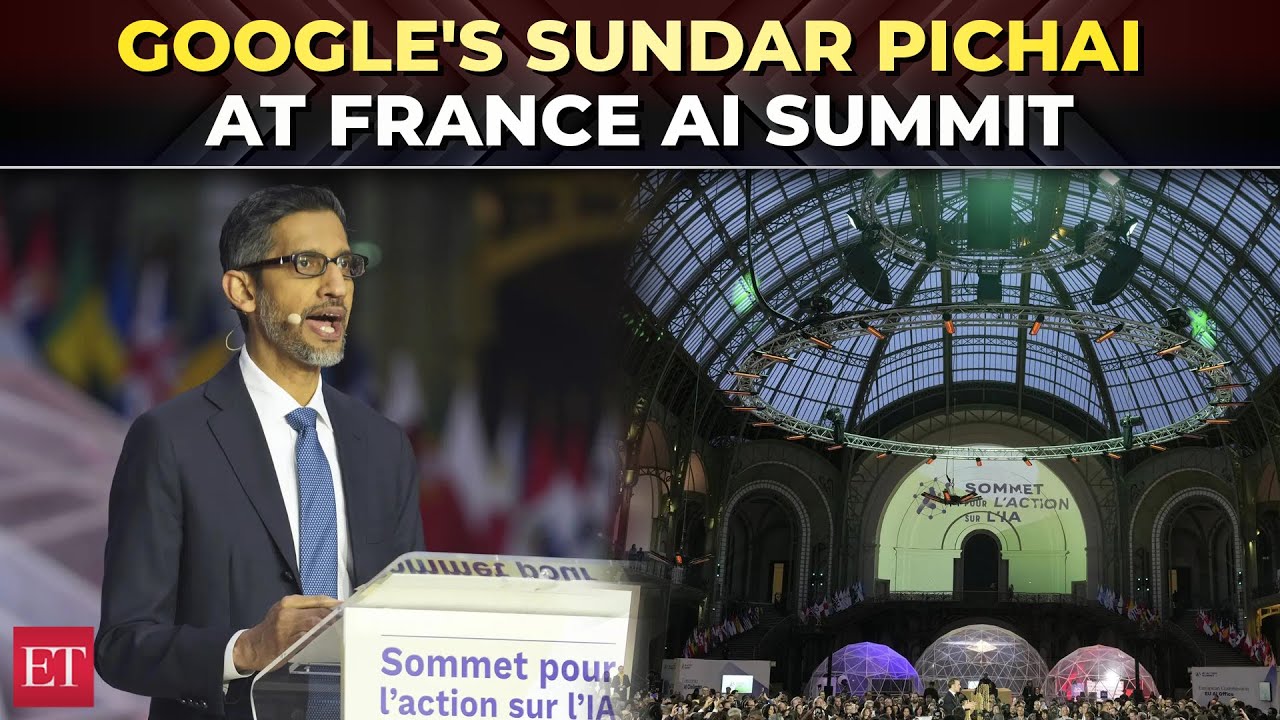
Google's Sundar Pichai at France AI Summit: 'AI rewiring technology, accelerating human ingenuity'
5.0 / 5 (0 votes)