The Population Bag Example
Summary
TLDRThis video script delves into a hypothetical 'population bag' example to illustrate the concepts of Type 1 and Type 2 errors in statistical hypothesis testing. It explains the scenario of sampling from two bags, one with and one without removed chips, to demonstrate how researchers might incorrectly reject or fail to reject a null hypothesis. The script clarifies why in real-world applications, managing Type 2 errors is more critical than Type 1 errors, as the null hypothesis of identical population means is never exactly true, unlike in the classroom example where it can be.
Takeaways
- 📚 The video discusses the 'population bag example', which is a hypothetical scenario used to illustrate concepts in hypothesis testing.
- 🎓 The example involves two population bags, each containing chips with numbers from one to five, and the task is to determine if the bags are the same or different based on samples.
- 🔍 The video introduces a null hypothesis that the two bags have the same mean, and an alternative hypothesis that they do not.
- 🧐 The decision rule is set with an alpha level of 0.1, meaning the null hypothesis will be rejected if the observed sample mean difference is unlikely (p < 0.1).
- 🔢 The video explains the process of taking samples from each bag, calculating the mean difference, and then determining a T value and p-value to test the hypothesis.
- 🔄 The process is repeated 16 times to simulate multiple experiments, highlighting the variability in outcomes and the potential for errors in hypothesis testing.
- 🚫 Type 1 errors occur when the null hypothesis is incorrectly rejected (false positive), and Type 2 errors occur when the null hypothesis is incorrectly accepted (false negative).
- 🤔 The video emphasizes that in real-world scenarios, the null hypothesis is never exactly true, making Type 1 errors impossible and Type 2 errors more critical to manage.
- 🌐 The 'population bag example' contrasts with real-world situations where the effect size (difference between population means) is often small, increasing the likelihood of Type 2 errors.
- 📉 The video concludes by answering a specific question about why beta (Type 2 error rate) is more important than alpha (Type 1 error rate) in real-world applications, highlighting the practical implications of hypothesis testing.
Q & A
What is the 'population bag example' described in the video?
-The 'population bag example' is a hypothetical scenario used to illustrate the concept of hypothesis testing. It involves two bags, each containing chips with numbers on them. The bags can either have the same chips or one bag has some chips removed, altering the mean value. The task is to determine, through sampling and statistical analysis, whether the two bags have the same or different chips.
What is the significance of the mean and standard deviation in the population bag example?
-In the population bag example, the mean and standard deviation of the chips in the bags are crucial. The mean represents the average value of the chips, and the standard deviation measures the spread of the values. These statistical measures help in understanding the distribution of the chips and are used in hypothesis testing to determine if the samples are drawn from bags with the same or different chips.
What are the two situations described in the population bag example?
-The two situations in the population bag example are: 1) Both bags have the same chips (e.g., six ones, twos, threes, fours, and fives), and 2) One bag has the same chips as the population bag, while the other has some chips removed (e.g., three ones, six twos, six threes, six fours, and six fives), resulting in a different mean.
Why is it important to calculate the mean difference between the samples in the population bag example?
-Calculating the mean difference between the samples is important because it helps in making a judgment about whether chips have been removed from the bag. If the two bags have the same chips, the means of the samples should be similar. However, if chips have been removed, the mean of the bag with removed chips will be higher, and the sample means will likely differ.
What is the null hypothesis in the hypothesis testing procedure described in the video?
-The null hypothesis in the hypothesis testing procedure is that the two bags are exactly the same, meaning the mean of the chips in bag one is the same as the mean of the chips in bag two. This assumption of no difference is what is being tested against the alternative hypothesis.
What is the decision rule used in the hypothesis testing procedure, and what is its significance?
-The decision rule used in the hypothesis testing procedure is that if the p-value is less than alpha (set at 0.1 in the example), the null hypothesis is rejected. This rule is significant because it determines whether the observed sample mean difference is statistically significant enough to conclude that the bags are different.
What are type 1 and type 2 errors in the context of hypothesis testing?
-In hypothesis testing, a type 1 error occurs when the null hypothesis is rejected when it is actually true. A type 2 error occurs when the null hypothesis is not rejected when it is actually false. In the population bag example, type 1 errors would be incorrectly concluding that the bags are different when they are the same, while type 2 errors would be incorrectly concluding that the bags are the same when they are different.
Why is beta more important than alpha in real-world situations but not in the population bag example?
-In real-world situations, the means of two populations are never exactly the same, so the null hypothesis is never strictly true. This means that the only kind of error that can be made is a type 2 error (failing to reject a false null hypothesis). Therefore, managing the probability of a type 2 error (beta) is more important. In the population bag example, however, the null hypothesis can be true, making both type 1 and type 2 errors possible.
What is the effect of sample size on the probability of making a type 2 error?
-The sample size has a significant impact on the probability of making a type 2 error. A smaller sample size reduces the power of the test, making it more likely to fail to detect a true effect (i.e., make a type 2 error). In the population bag example, the small sample size of 10 chips from each bag contributes to the high probability of type 2 errors.
How does the effect size influence the probability of making a type 2 error in the population bag example?
-The effect size, which is the difference between the population means, plays a crucial role in the probability of making a type 2 error. If the effect size is small, the test is less likely to detect a difference even if one exists, increasing the likelihood of a type 2 error. In the population bag example, the small difference in means between the bags with and without removed chips contributes to the high probability of type 2 errors.
Outlines
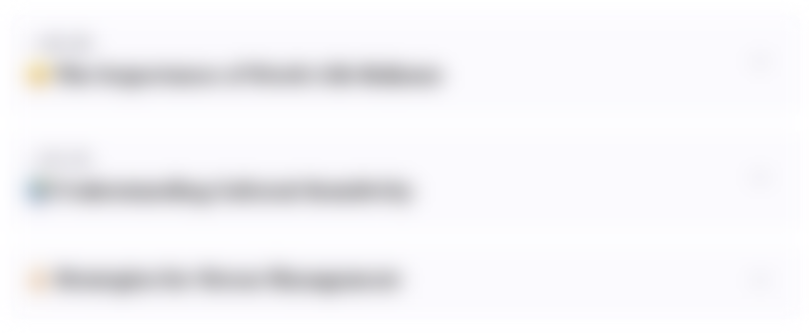
Этот раздел доступен только подписчикам платных тарифов. Пожалуйста, перейдите на платный тариф для доступа.
Перейти на платный тарифMindmap
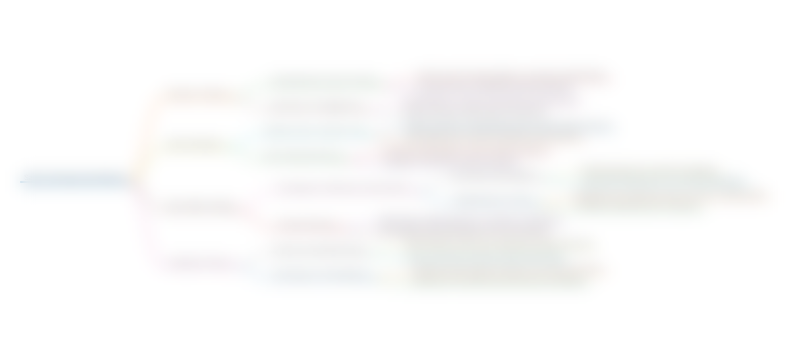
Этот раздел доступен только подписчикам платных тарифов. Пожалуйста, перейдите на платный тариф для доступа.
Перейти на платный тарифKeywords
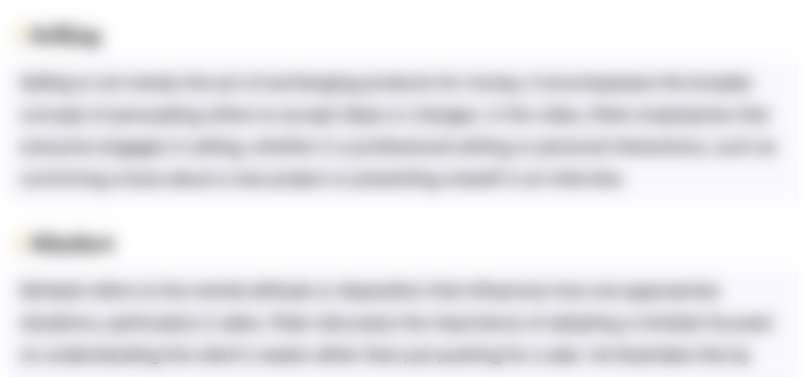
Этот раздел доступен только подписчикам платных тарифов. Пожалуйста, перейдите на платный тариф для доступа.
Перейти на платный тарифHighlights
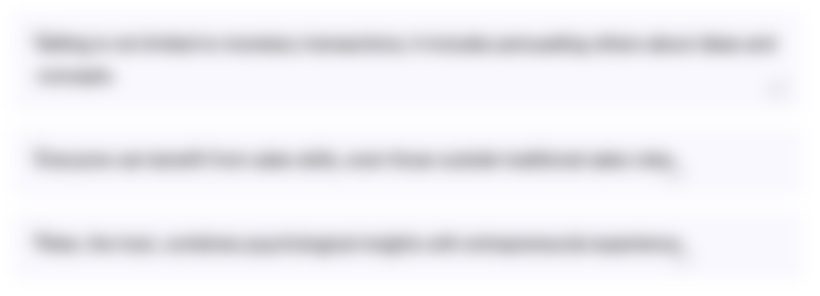
Этот раздел доступен только подписчикам платных тарифов. Пожалуйста, перейдите на платный тариф для доступа.
Перейти на платный тарифTranscripts
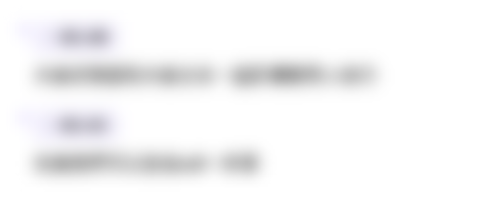
Этот раздел доступен только подписчикам платных тарифов. Пожалуйста, перейдите на платный тариф для доступа.
Перейти на платный тариф5.0 / 5 (0 votes)