The EDA framework data hiring managers love (use it in your portfolio projects)
Summary
TLDRIn this video, the speaker provides a practical framework for conducting Exploratory Data Analysis (EDA), emphasizing its importance for data analysts, especially in early career stages. The speaker walks through a real-world scenario involving an e-commerce company's sales trends, showing how to break down large business questions into manageable smaller ones. Key topics include identifying the type of analysis required, using simple tools like pivot tables and conditional formatting, and generating actionable insights. The speaker encourages analysts to use structured approaches to stand out in the job market and highlights how EDA is crucial for both daily tasks and interviews.
Takeaways
- 😀 Networking and in-person interactions with colleagues and students can be very rewarding and provide valuable insights, especially when discussing challenges like Exploratory Data Analysis (EDA).
- 😀 EDA is a crucial skill for early-career data analysts, accounting for 30-40% of their job in the first few years.
- 😀 A structured approach to EDA helps data analysts translate their findings into valuable insights for stakeholders, setting standout analysts apart.
- 😀 Confidence in EDA comes from mastering the skill, which directly translates to real-world value for businesses, an essential trait for both analysts and hiring managers.
- 😀 The framework for EDA involves identifying the question type, breaking down big questions into smaller ones, and translating these into specific techniques.
- 😀 There are four main categories of exploratory analysis questions: overall trends, growth rates, performance measurement, and KPI reporting.
- 😀 For overall trends in sales, smaller questions include seasonality (e.g., sales by time/month), dimensionality (e.g., sales by region/product), summary stats (e.g., highest/lowest sales), and distribution (e.g., countries/products contributing to a high percentage of sales).
- 😀 In growth rates, seasonality, dimensionality, and summary stats also play key roles, helping to calculate and analyze growth trends across different timeframes and segments.
- 😀 As an analyst, applying these smaller questions (seasonality, dimensionality, summary stats, and distribution) helps answer broader business questions and leads to actionable insights.
- 😀 The first few years of EDA in a data analyst role can be done effectively with basic tools like pivot tables, conditional formatting, and simple charts, which help uncover patterns without requiring complex algorithms or programming skills.
Q & A
What is the main focus of the speaker in the video?
-The speaker discusses the importance of exploratory data analysis (EDA) in the early stages of a data analyst's career, emphasizing a structured approach to EDA that translates directly into insights for stakeholders.
Why is EDA a crucial skill for early career data analysts?
-EDA accounts for about 30-40% of a data analyst's job in the early years. It is a skill that allows analysts to identify patterns, trends, and insights in data, which is highly valued by stakeholders and businesses.
What is the first step the speaker suggests in approaching an EDA request?
-The first step is to clarify the request with the stakeholder by asking questions such as what needs to be delivered, understanding the business context, and ensuring clarity about the necessary metrics and data sources.
How does the speaker suggest breaking down large business questions in EDA?
-The speaker proposes a framework where the big business questions are broken down into smaller, more manageable questions that focus on specific analysis techniques like seasonality, dimensionality, summary stats, and distributions.
What are the four main categories of exploratory analysis questions?
-The four main categories are: 1) Overall trends, 2) Growth rates, 3) Performance measurement, and 4) KPI reporting.
What smaller questions are associated with the analysis of overall trends in sales?
-Smaller questions include seasonality (e.g., sales by month), dimensionality (e.g., sales by region or product), summary stats (e.g., highest and lowest sales), and distributions (e.g., percentage of sales from specific regions or products).
Why is dimensional segmentation important in EDA?
-Dimensional segmentation helps break down a metric (like sales) by different categories, such as region or product, which allows analysts to understand how various factors contribute to overall performance.
What is the purpose of summary statistics in EDA?
-Summary statistics help summarize key aspects of the data, such as the minimum, maximum, average, and mode, to give a clearer understanding of the overall trends and outliers in the dataset.
What is the significance of conditional formatting in EDA?
-Conditional formatting is used to visually highlight important patterns or anomalies in the data, such as sales spikes or dips, making it easier for analysts to spot trends at a glance.
What is the role of communication in the EDA process, according to the speaker?
-Effective communication is crucial. Once analysis is done, the findings need to be clearly communicated to stakeholders. The speaker also mentions creating an executive summary or email that highlights key insights and recommendations.
Outlines
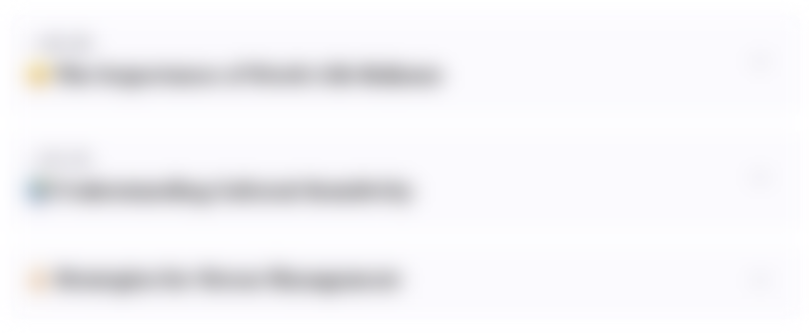
Этот раздел доступен только подписчикам платных тарифов. Пожалуйста, перейдите на платный тариф для доступа.
Перейти на платный тарифMindmap
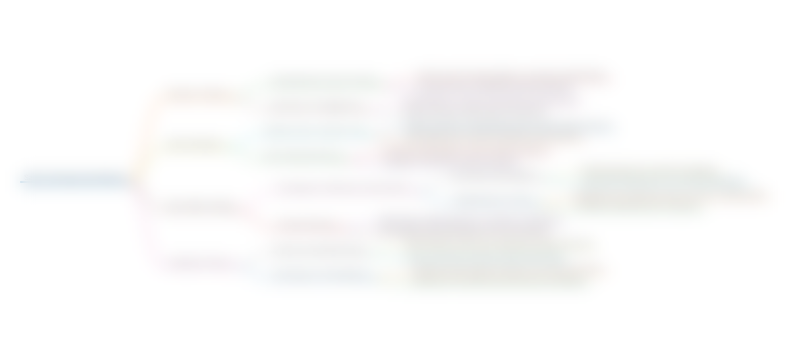
Этот раздел доступен только подписчикам платных тарифов. Пожалуйста, перейдите на платный тариф для доступа.
Перейти на платный тарифKeywords
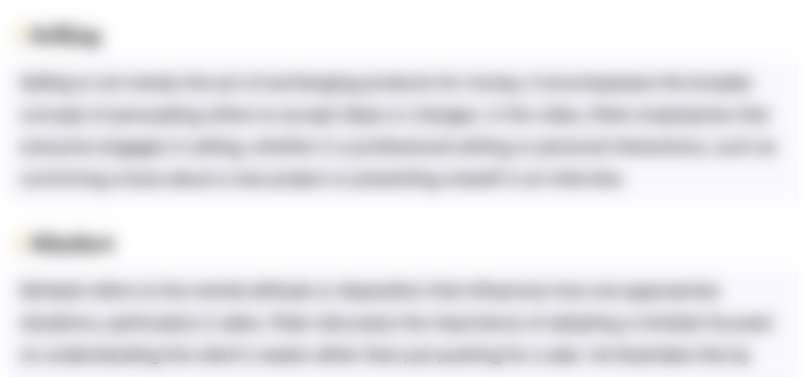
Этот раздел доступен только подписчикам платных тарифов. Пожалуйста, перейдите на платный тариф для доступа.
Перейти на платный тарифHighlights
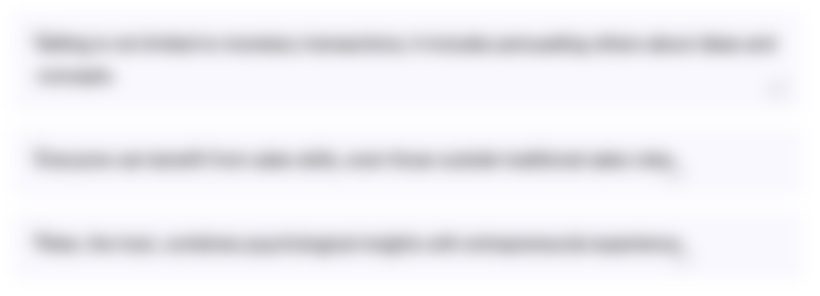
Этот раздел доступен только подписчикам платных тарифов. Пожалуйста, перейдите на платный тариф для доступа.
Перейти на платный тарифTranscripts
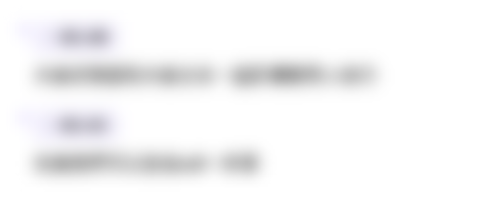
Этот раздел доступен только подписчикам платных тарифов. Пожалуйста, перейдите на платный тариф для доступа.
Перейти на платный тарифПосмотреть больше похожих видео
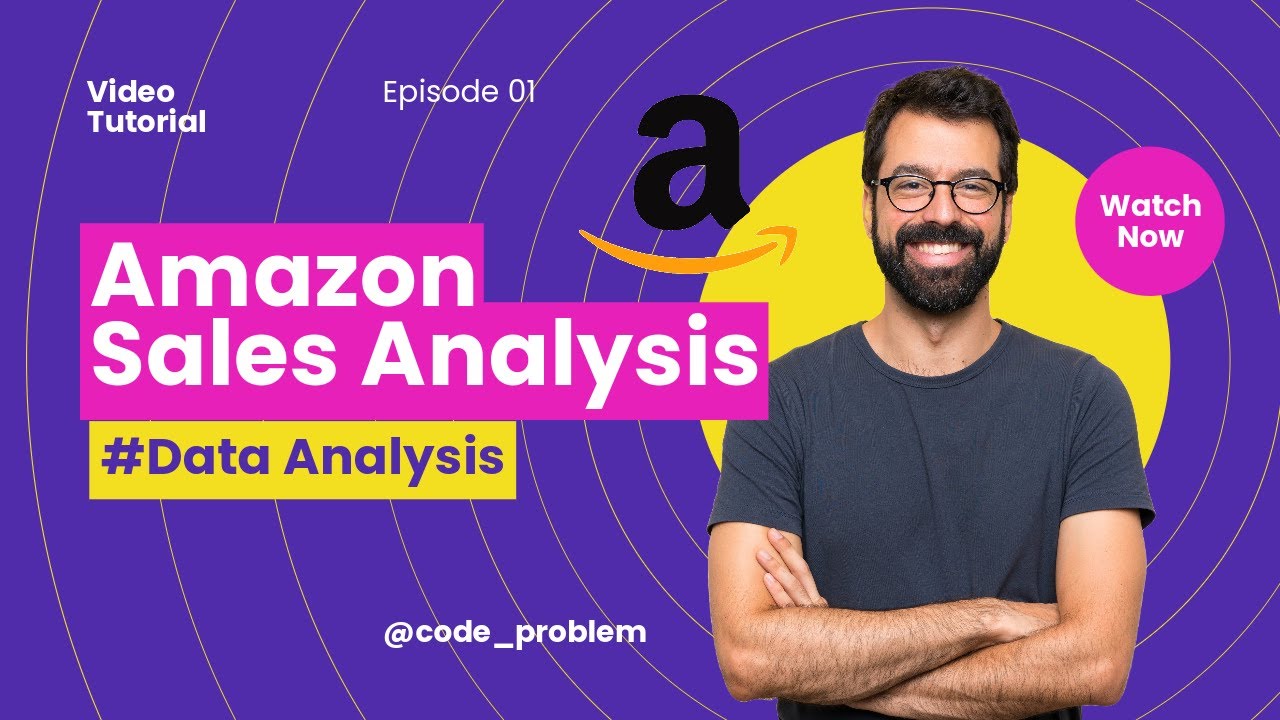
#1 Unlock The Secrets Of Data Analysis: A Comprehensive Tutorial On The Data Analysis Lifecycle
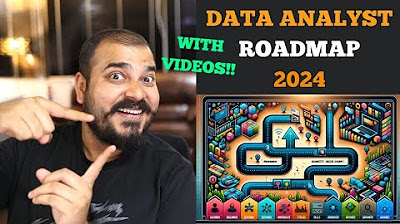
Complete Roadmap To Become Data Analyst In 2024 With Videos And Materials
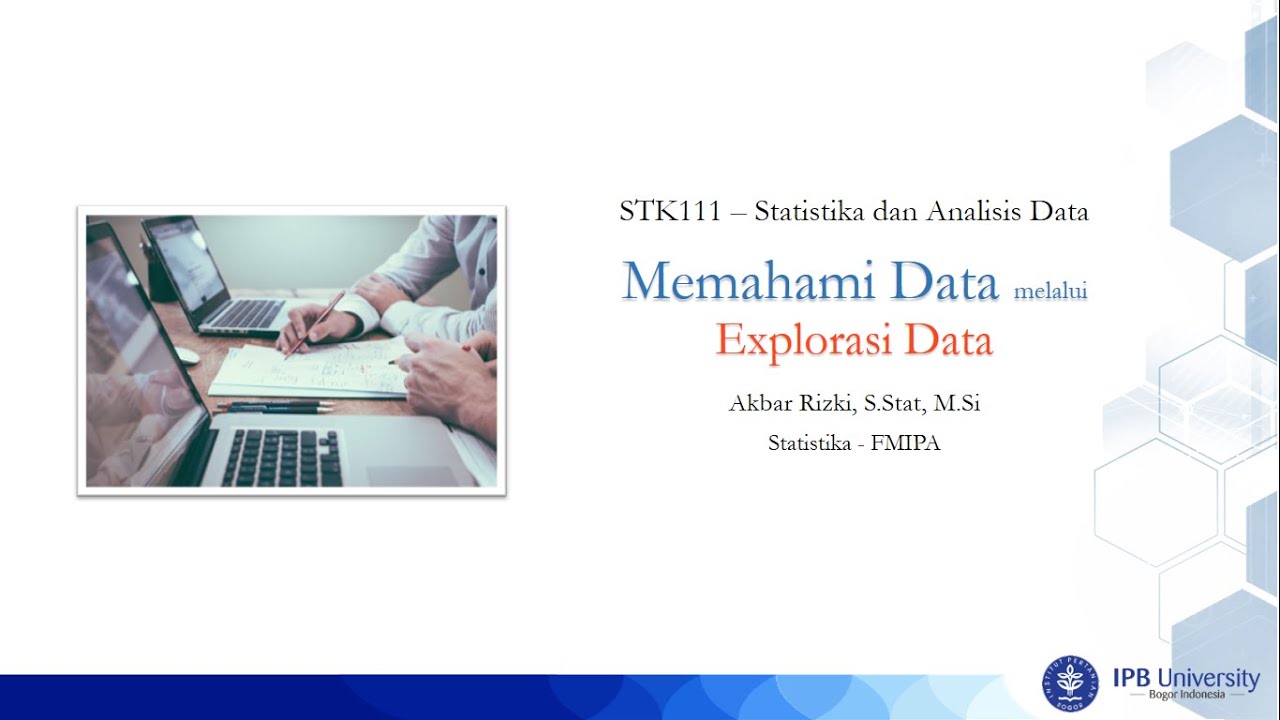
04-1 Memahami Data Melalui Eksplorasi Data
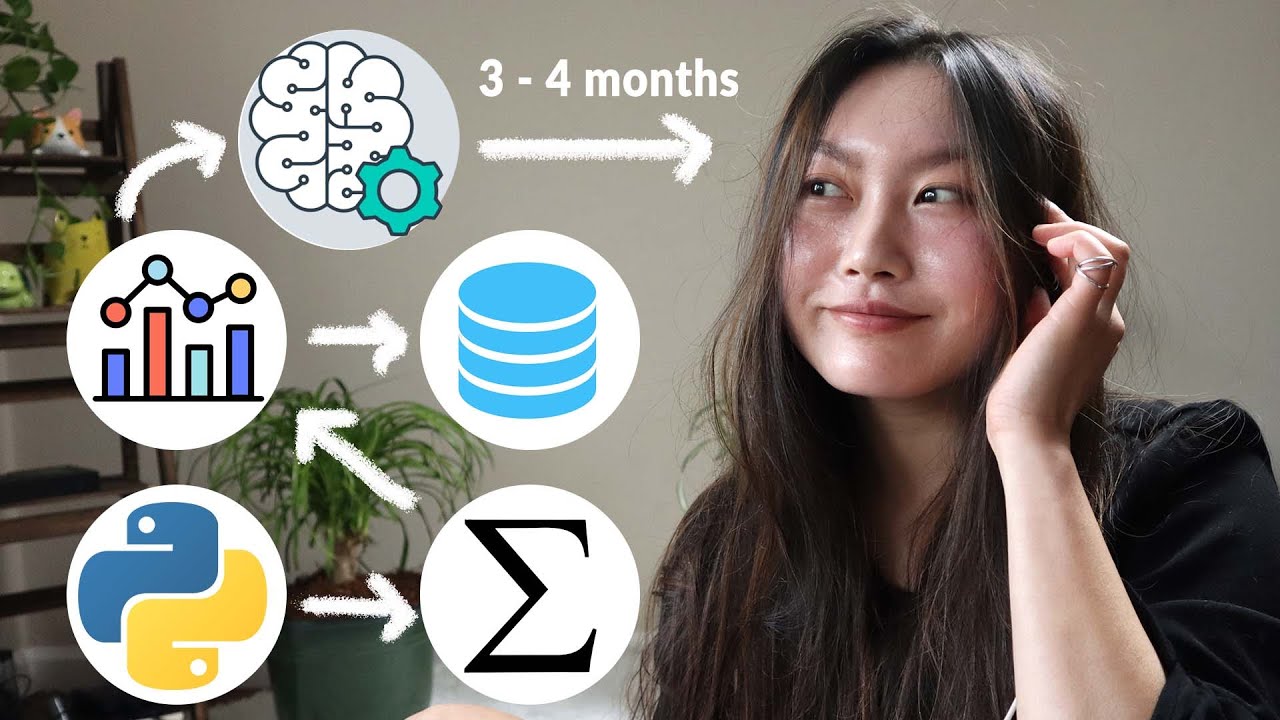
How I Would Learn Data Science in 2022
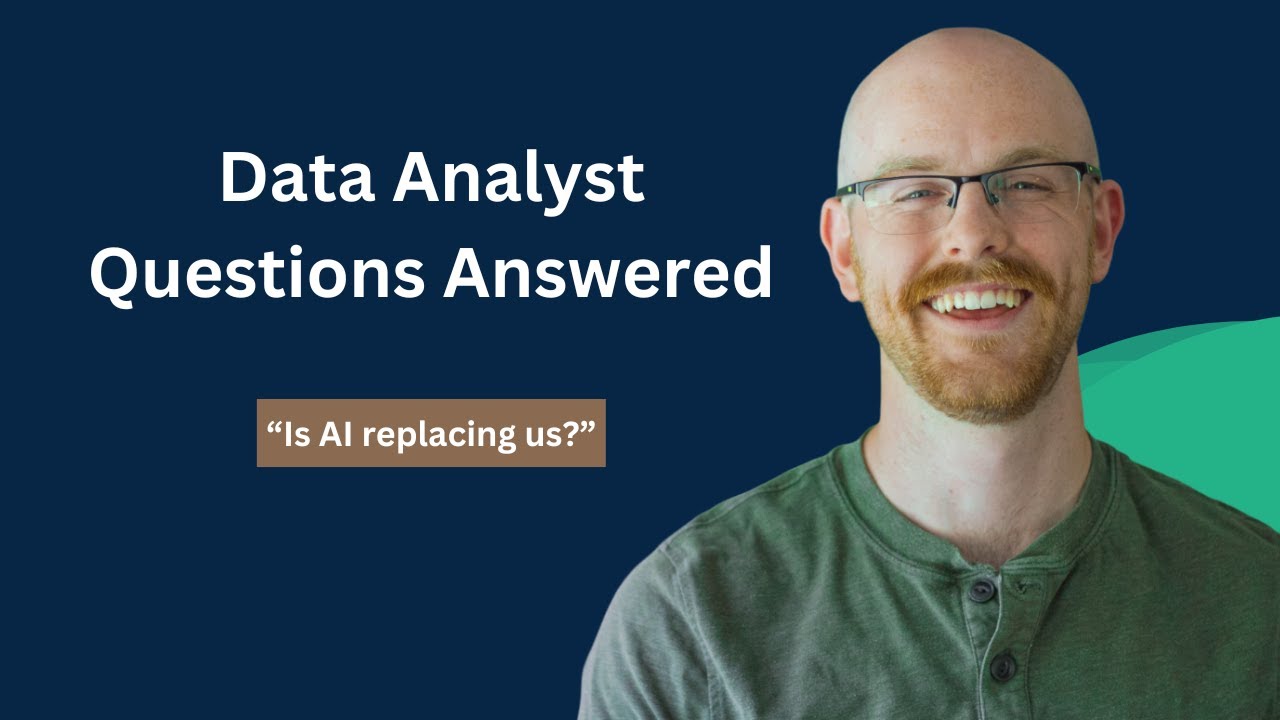
Most Commonly Asked Questions by Aspiring Data Analysts

What is exploratory data analysis
5.0 / 5 (0 votes)