How Perplexity works | Aravind Srinivas and Lex Fridman
Summary
TLDRThe transcript discusses 'Perplexity,' an AI-driven answer engine that functions by combining search engine capabilities with a large language model (LLM). It emphasizes the importance of sourcing, akin to academic research, to ensure accuracy. Perplexity is designed to provide direct answers with citations, inspired by the need for reliable information in a world of abundant but often misleading online content. The conversation also touches on the limitations of Perplexity compared to traditional search engines like Google, highlighting the ongoing challenges in AI integration for real-time information and user experience.
Takeaways
- 🧠 Perplexity is described as an 'answer engine' that provides answers backed by sources, similar to academic referencing.
- 🔍 The search engine component of Perplexity extracts relevant results from the internet, which are then fed into a large language model (LLM).
- 🤖 The LLM processes the information and generates a well-formatted answer with citations, adhering to the academic principle of sourcing every statement.
- 📚 Perplexity's approach is inspired by the need for accuracy in academic writing, aiming to ensure that chatbots provide reliable and citable information.
- 💡 The founders of Perplexity, with academic backgrounds, sought to apply the rigor of academic research to chatbot technology to enhance accuracy.
- 🌐 Perplexity is not just a search engine but a 'knowledge discovery engine' that encourages users to explore further after receiving an answer.
- 📊 A comparison between Perplexity and traditional search engines like Google highlights Perplexity's strengths in direct answers and synthesized information.
- 🚀 Perplexity's AI, while impressive, is not yet a full replacement for Google due to limitations in accuracy, speed, and real-time information provision.
- 🔗 The platform generates related searches and questions to spark curiosity and continue the knowledge discovery process.
- 🌟 The potential for personalization and localization in Perplexity's responses could significantly enhance the user experience by anticipating needs and providing contextually relevant information.
Q & A
What is the primary function of Perplexity?
-Perplexity is primarily described as an answer engine that provides direct answers to questions by synthesizing information from various sources, backed by citations.
How does Perplexity combine the search engine and LLM functionalities?
-Perplexity uses a search engine to find relevant sources, extracts information, and then feeds it into a large language model (LLM) to formulate a well-formatted answer with appropriate citations.
What is the significance of sourcing in Perplexity's operation?
-Sourcing is crucial as it ensures that every statement made by Perplexity is backed by credible sources, similar to academic writing, which enhances the accuracy and reliability of the answers provided.
How does Perplexity's approach differ from traditional search engines?
-Unlike traditional search engines that provide a list of links, Perplexity focuses on delivering direct answers synthesized from multiple sources, aiming to be more like an academic paper with citations.
What inspired the creation of Perplexity?
-The creators' academic roots and the need for accurate information led to the development of Perplexity. They wanted a system that would only provide information that could be cited from the internet, ensuring accuracy.
Why did the founders feel the need to build Perplexity?
-The founders felt the need to build Perplexity due to their own experiences with the inadequacy of existing search engines in providing clear and accurate answers, especially in areas like insurance where they were novices.
How does Perplexity ensure the accuracy of its answers?
-Perplexity ensures accuracy by mandating that every piece of information provided is backed by sources found on the internet, following the academic principle of citing every statement made in a paper.
What is the role of user experience in Perplexity's design?
-User experience plays a significant role in Perplexity's design as it aims to provide direct answers and synthesize information in a way that is easy for users to understand and appreciate, much like Wikipedia.
How does Perplexity handle real-time information?
-Perplexity is working on integrating real-time information, but it is a complex task that requires prioritizing recent information and presenting it in a user-friendly manner.
What are some of the challenges Perplexity faces in providing accurate answers?
-Some challenges include ensuring accuracy and speed, integrating real-time information, and designing custom UIs for specific sets of questions to present information in the best possible way.
How does Perplexity's approach compare to Google for everyday searches?
-While Perplexity provides direct answers and summaries, Google is faster and more efficient for simple navigational queries and real-time information. Perplexity is not yet a full replacement for Google for everyday searches.
Outlines
🔍 The Concept of Perplexity as an Answer Engine
The first paragraph introduces Perplexity as an innovative answer engine that functions by combining the capabilities of a traditional search engine with a large language model (LLM). It emphasizes that Perplexity is designed to provide answers backed by sources, akin to academic research. The process involves extracting relevant information from search results, feeding it into the LLM, and generating a well-formatted answer with citations. The goal is to ensure accuracy and coherence in the responses, inspired by the rigorous standards of academic writing where every statement is supported by a citation. The narrative also touches upon the challenges faced by the founders, who, despite being new to building a startup, sought to create a product that could answer questions with the same reliability and depth as academic research.
🌐 Perplexity as a Knowledge Discovery Engine
The second paragraph delves into the distinction between Perplexity and traditional search engines. It positions Perplexity as a knowledge discovery engine, emphasizing the journey of learning that begins after receiving an answer. The speaker highlights the importance of related questions and the continuous pursuit of knowledge, which is central to Perplexity's design. The paragraph contrasts Perplexity's approach of providing direct answers with the link-based results of search engines like Google. It also discusses the user experience and technological integration that sets Perplexity apart, including its ability to handle poorly phrased questions and generate related searches to spark curiosity and further exploration. The speaker acknowledges that while Perplexity is impressive, it is not yet a full replacement for Google for everyday searches, citing differences in speed, accuracy, and the ability to provide real-time information.
🛠️ Challenges in Building a User-Centric Knowledge Engine
The third paragraph addresses the complexities involved in creating a user-centric knowledge engine like Perplexity. It discusses the need for custom user interfaces (UIs) tailored to specific queries and the importance of presenting information in a way that anticipates user needs. The speaker reflects on the limitations of relying solely on advanced models and emphasizes the importance of product design in delivering a personalized and anticipatory user experience. The paragraph also touches on the potential for personalization based on user habits and interests, suggesting that a combination of location, frequented sites, and topics of interest can significantly enhance the personalization of search results. The discussion concludes with a hypothetical scenario where Perplexity could provide not just the weather but also practical advice like what to wear, based on the user's activities and preferences.
Mindmap
Keywords
💡Perplexity
💡Search Engine
💡LLM (Large Language Model)
💡Citations
💡Academic Writing
💡Coherence
💡Knowledge Discovery
💡Real-time Information
💡User Experience (UX)
💡Personalization
Highlights
Perplexity is described as an answer engine that provides answers backed by sources, similar to academic referencing.
The search engine component of Perplexity extracts relevant results from the internet to feed into the large language model (LLM).
The LLM processes the extracted information and generates a well-formatted answer with citations.
Perplexity is instructed to write answers with academic rigor, ensuring each statement is supported by a citation.
The concept of Perplexity was born out of the need for accurate information, inspired by the founders' academic backgrounds.
The founders aimed to create a chatbot that only states facts it can source from the internet, mirroring academic practices.
Perplexity's approach is compared to Wikipedia, which requires sources for edits, emphasizing the importance of notable sources.
The product's development was driven by the need to answer questions accurately, especially in areas like insurance where the founders were inexperienced.
Perplexity is positioned as a knowledge discovery engine, going beyond just providing answers to spark curiosity and further inquiry.
The user experience with Perplexity includes AI-generated summaries and a focus on direct answers rather than a list of links.
Perplexity generates related searches to continue the knowledge discovery process, encouraging users to explore more.
The comparison between Perplexity and Google highlights Perplexity's strengths in direct answers and summarization.
Google's speed and efficiency in providing real-time information and direct navigation are noted as advantages over Perplexity.
Perplexity's challenge in accuracy and speed is discussed, with a focus on the need for improvement in these areas.
The importance of UI design in presenting information effectively and anticipating user needs is emphasized.
The potential for personalization in Perplexity is discussed, suggesting that it could enhance the user experience.
The concept of localization and personalization based on user habits and preferences is explored as a way to improve the product.
The idea of providing additional context beyond the direct answer, such as suggesting what to wear based on weather, is presented as a future enhancement.
Transcripts
perplexity is part search engine part
llm so how does it work and what role
does each part of that the search and
the llm play in uh serving the final
result perplexity is best described as
an answer engine so you ask it a
question you get an answer except the
difference is all the answers are backed
by
sources this is like how an academic
writes a paper now that referencing part
the sourcing part is where the search
engine part comes in so you combine
traditional search extract results
relevant to the query the user asked you
read those links extract the relevant
paragraphs feed it into an llm llm means
large language model and that llm takes
the relevant paragraphs looks at the
query and comes up with a well formatted
answer with appropriate footnote to
every sentence it says because it's been
instructed to do so it's been instructed
with that one particular instruction of
given a bunch of links and paragraphs
write a concise answer for the user with
the appropriate citation so the magic is
all of this working together in one
single orchestrated product and that's
what we build perplexity for so it was
explicitly instructed to uh write like
an academic essentially you found a
bunch of stuff on the internet and now
you generate something called coherent
and uh something that humans will
appreciate and cite the things you found
on the internet in the narrative you
create for the human correct when I
wrote my first paper uh the senior
people who are working with me on the
paper told me this one profound thing
which is that every sentence you write
in a
paper should be backed with a citation
with a with a citation from another
peer-reviewed paper or an experimental
result in your on paper anything else
that you say in the paper is more like
an
opinion that's it's it's a very simple
statement but pretty profound and how
much it forces you to say things that
are only
right and we took this principle and
asked
ourselves what is the best way to make
chat
Bots
accurate is force it to only say things
that it can find on the
internet right and find from multiple
sources so
this kind of came out of a need rather
than oh let's try this idea when we
started the startup there were like so
many questions all of us had because we
were complete
noobs never built a product before never
built like a startup before of course we
had worked on like a lot of cool
engineering and research problems but
doing something from scratch is the
ultimate
test and there were like lots of
questions you know what is the health
insur like the first employee we had
hired he came and asked us for health
insurance normal need I didn't care I
was like why do I need a health
insurance this company dies like who
cares um my other two co-founders had
were married so they had health
insurance to their spouses but this guy
was like looking for health
insurance and I didn't even know
anything who are the providers what is
co insurance or deductible or like none
of these made any sense to me and you go
to Google insurance is a category where
like a major ad spend category so even
if you ask for something you're not
Google has no incentive to give you
clear answers they want you to click on
all these links and read for yourself
because all these insurance providers
are biding to get your attention so we
integrated a slackbot that just PS GPD
3.5 and answered a
question now sounds like problem solve
except we didn't even know whether what
it said was correct or not and in fact
was saying incorrect things we were like
okay how do we address this problem and
we remembered our academic Roots um you
know Dennis and myself were both
academics then is my
co-founder and we said okay what is one
way we stop ourselves from saying
nonsense in a peerreview paper we're
always making sure we can cite what it
says what what we what we write every
sentence now what if we ask the chatbot
to do that and then we realized that's
literally how Wikipedia works in
Wikipedia if you do a random edit people
expect you to actually have a source for
that and not just any random Source they
expect you to make sure that the source
is
notable you know there are so many
standards for like what counts is
notable and not so you decide this is
worth working on it's not just a problem
that will be solved by an smarter model
because there's so many other things to
do on the search layer and the sources
layer and making sure like how well the
answer is formatted and presented to the
user so that's why the product exists
well there's a lot of questions to ask
there but first zoom out once again so
fundamentally it's about search so you
said first there's a search element mhm
and then there's an storytelling element
via
llm and the citation element but it's
about search first so you think of
perplexity as a search engine mhm I
think of perplexity as a knowledge
discovery engine neither a search engine
I mean of course we call it an answer
engine but everything matters here uh
the journey doesn't end once you get an
answer in my opinion the Journey Begins
after you get an answer you see related
questions at the bottom suggested
questions to ask why because maybe the
answer was not good enough or the answer
was good enough but you probably want to
dig deeper and ask
more
and that's why in in the search bar we
say where knowledge begins
cuz there's no end to knowledge you can
only expand and grow like that's the
whole concept of the beginning of
infinity book by David do you always
seek new knowledge so I see this as sort
of a discovery process you start you
know let's say you literally whatever
you ask me to right now you could have
asked perplexity too MH hey perplexity
is it a search engine or is it an answer
oral what is it and then like you see
some questions at the bottom right we're
going to straight up ask this right now
I I don't know how it's going to work is
a perplexity a search engine or an
answer
engine that's a poorly phrased question
but one of the things I love about
perplexity the poorly phrased questions
will nevertheless lead to interesting
directions perplexity is primarily
described as an answer engine rather
than a traditional search engine key
points showing the difference between
answer Engine versus search engine uh
this is so nice and it compares
perplexity versus a traditional search
engine like Google so Google provides a
a list of links to websites perplexity
focuses on providing direct answers
synthesizing information from various
sources user experience technological
approach uh so there's AI integration
with Wikipedia like responses this is
really well done and you look at the
bottom right right so you you were not
intending to ask those
questions but they're relevant like can
perplexity replace Google for everyday
searches all right let's click on that
but the really interesting generation
that task that step of generating
related searches so the next step of the
Curiosity journey of expanding your
knowledge is really interesting exactly
so that's what David do shes in this
book which is for creation of new
knowledge starts from the spark of
curiosity to seek
explanations and then you find new
phenomenon or you get more depth on
whatever knowledge you already have I
really love the steps that the pro
search is doing compare perplexity and
Google for everyday searches step two
evaluate strengths and weaknesses of
perplexity evaluate strengths and
weaknesses of Google it's like a
procedure yeah complete okay answer
perplexity AI while impressive is not
yet a full replacement for Google for
everyday searches yes here are the key
points based on the provided sources
strength of perplexity AI direct answers
AI part summaries Focus search user
experience we can dig into the details
of a lot of these weaknesses of
perplexity AI accuracy and speed
interesting I don't know if that's
accurate well Google Google is faster
than perplexity because you instantly
render the links the latency is it's
like you get 200 300 to 400 milliseconds
results here it's like you know still
not about like th000 milliseconds here
right for simple navigational queries
such as finding a specific website
Google is more efficient and reliable so
if you actually want to get straight to
the source yeah you just want to go to
kayak yeah just want to go fill up a
form like you want to go like pay your
credit card dues real time information
Google excels in providing real time
information like sports SC so like while
I think perplexity is trying to
integrate real time like recent
information put Priority on recent
information that require that's like a
lot of work to integrate exactly because
that's not just about throwing an
llm uh you like when you're asking oh
like what what dress should I wear out
today in Austin um you you do want to
get the weather across the time of the
day even though you didn't ask for it
and then Google presents this
information in like cool
widgets um and I think that is where
this is a very different problem from
just building another
chatbot and and and the information
needs to be presented well and and the
user intent like for example if you ask
for a stock price uh you might even be
interested in looking at the historic
stock price even though you never asked
for it you might be interested in
today's price these are the kind of
things that like you have to build as
custom uis for every query and why I
think this is a hard problem it's not
just like the Next Generation model will
solve the previous generation models
problems here the next Generation model
will be smarter you can do these amazing
things like planning like query breaking
it down into pieces collecting
information aggregating from sources
using different tools those kind of
things you can do you can keep answering
in harder and harder queries but there's
still a lot of work to do on the product
layer in terms of how the information is
best presented to the user and how you
think backwards from what the user
really wanted and might want as a next
step and give it to them before they
even ask for it but I don't know how
much of that is a UI problem of
Designing custom UI for a specific set
of questions I think at the end of the
day Wikipedia
looking uh UI is good enough if the raw
content that's provided the text content
is is powerful so if I want to know the
weather m in Austin if it like gives
me five little pieces of information
around that maybe the weather today and
maybe uh other links to say do you want
hourly and maybe it gives a little extra
information about rain and temperature
all that kind of stuff yeah exactly but
you would like the product when you as
for weather uh let's say it localizes
you to Austin automatically and not just
tell you it's hot not just tell you it's
humid but also tells you what to
wear you wouldn't ask for what to wear
but it would be amazing If the product
came and told you what to wear how much
of that could be made much more powerful
with some memory with some
personalization a lot more definitely I
mean but the personalization there's an
8020 here the 8020 is
achieved uh
with your
location let's say your
Jer and then you know like like sites
you typically go to like a rough sense
of topics of what you're interested in
all that can already give you a great
personalized experience mhm it doesn't
have to like have infinite
memory infinite context Windows have
access to every single activity you've
done that's an Overkill yeah yeah I mean
humans are creatures of habit most of
the time we do the same thing and yeah
it's like first few principal
vectors first few principal first like
most most important I vectors yes yeah
thank you for reducing humans to that to
the most important IG vectors right but
like for me usually I check the weather
if I'm going running so it's important
for the system to know that running is
an activity that I do but also depends
on like you know when you when you run
like if you're asking night maybe you're
not looking for running but right but
then that that starts to get into
details really I never ask a night cuz I
don't care so like usually it's always
going to be a running about running and
even at night it's going to be about
running because I love running at night
Посмотреть больше похожих видео
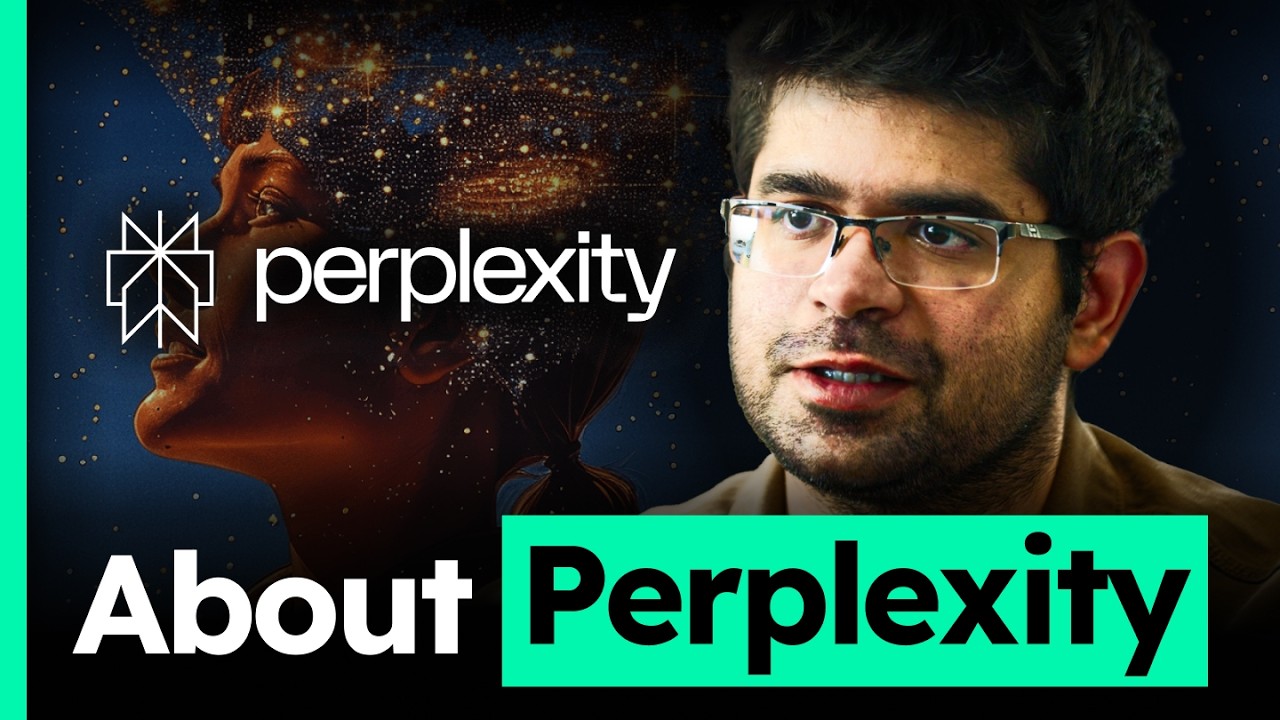
How we built $1B Startup in 2 Years | Perplexity AI, Aravind Srinivas
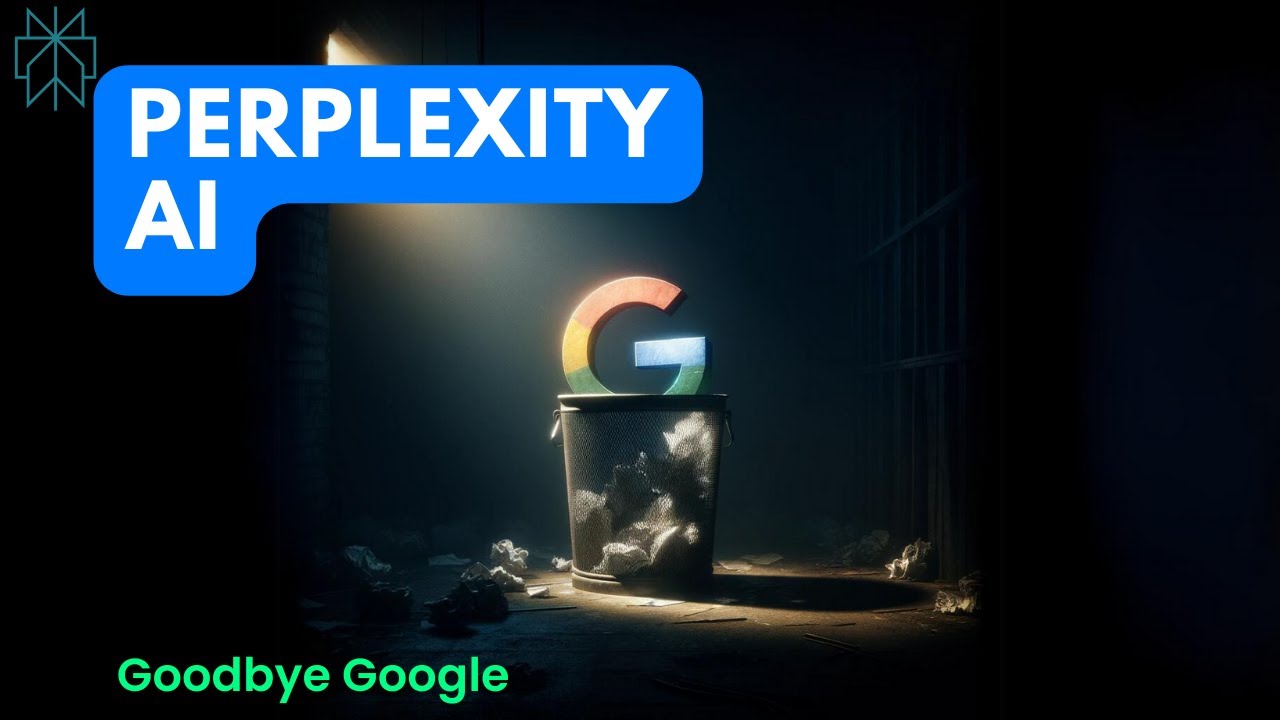
Perplexity AI Masterclass: Search Will Never Be the Same
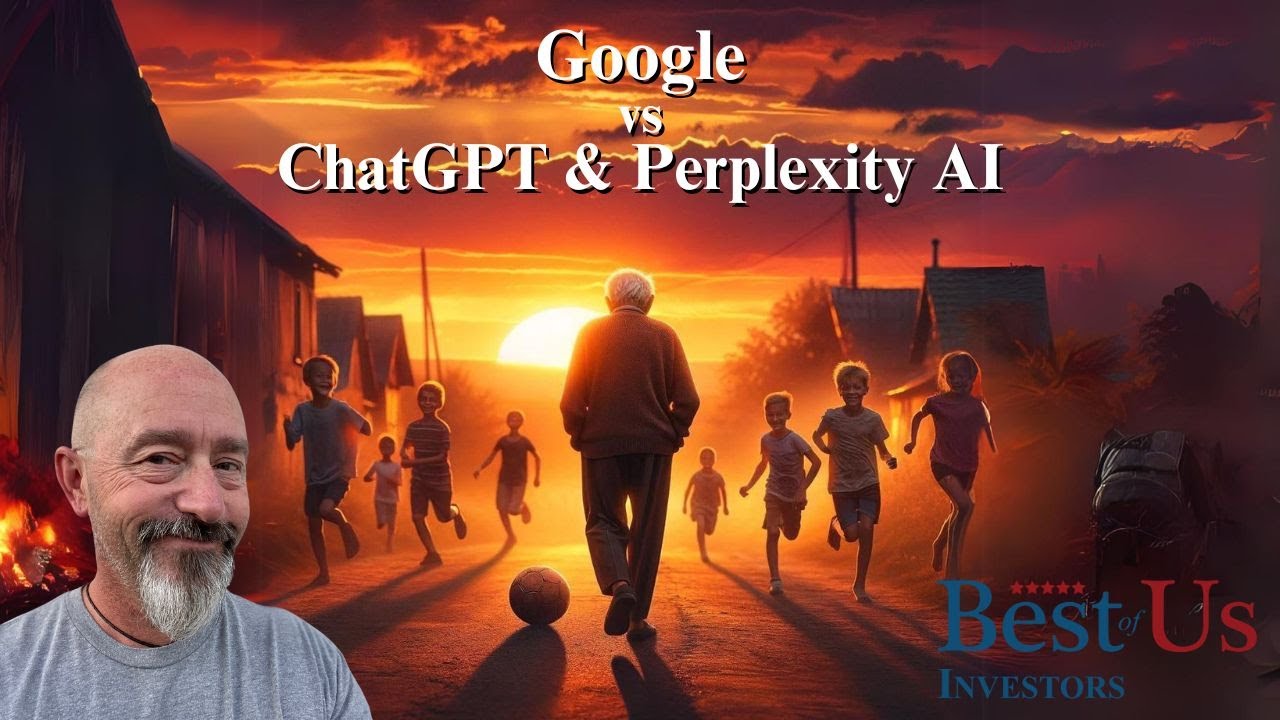
Has Google Met its Match | ChatGPT and Perplexity AI
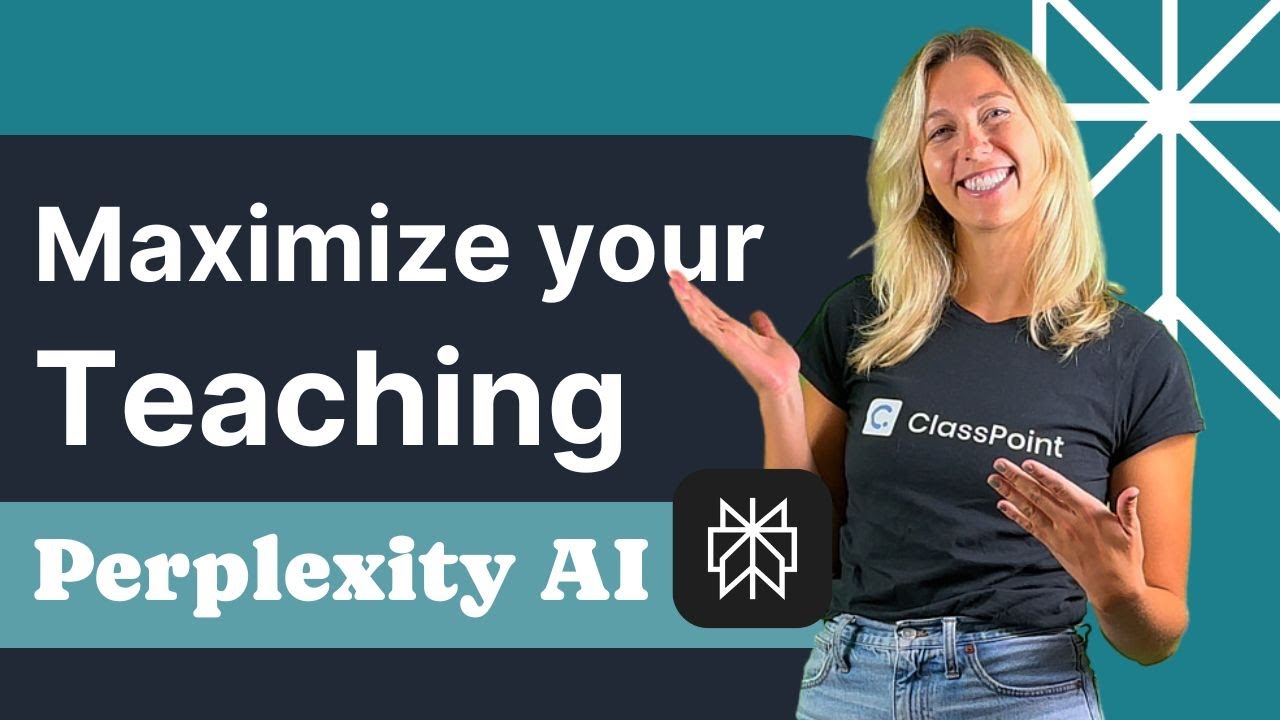
Maximize Teaching with Perplexity AI [ How to Use Perplexity for Teachers ]
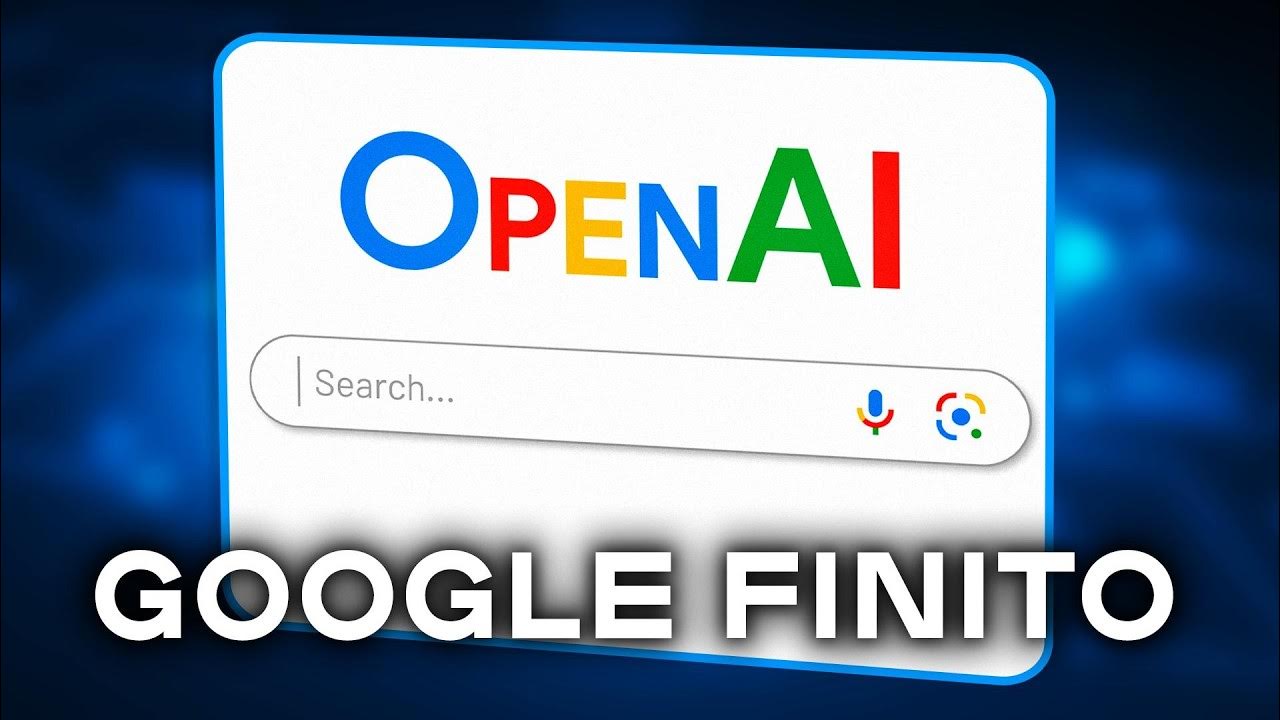
La fin de Google ?
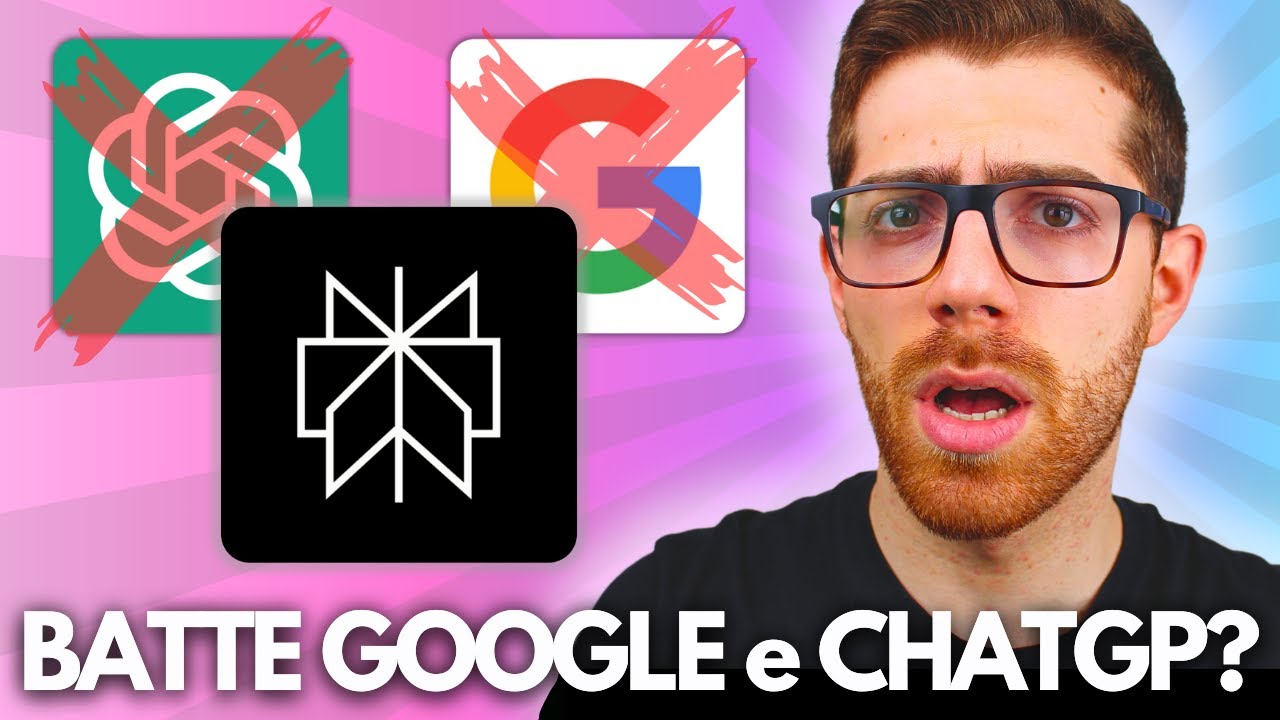
MEGLIO di GOOGLE e CHATGPT? Perplexity AI mi ha STUPITO! 🤯
5.0 / 5 (0 votes)