Why Fine Tuning is Dead w/Emmanuel Ameisen
Summary
TLDRIn this talk, Emmanuel discusses the evolution and diminishing importance of fine-tuning in machine learning. He shares his insights on trends, performance observations, and the challenges associated with fine-tuning. He suggests that focusing on data work, infrastructure, and effective prompting might be more beneficial than fine-tuning, especially as language models continue to improve.
Takeaways
- 😀 The speaker, Emmanuel, believes that fine-tuning may be less important in the field of machine learning than it once was, and he aims to defend this stance in his talk.
- 🔧 Emmanuel has a background in machine learning, including roles in data science, ML education, and working as a staff engineer at Stripe, and currently at Anthropic.
- 📚 He mentions mlpower.com, where his book, considered a classic in applied machine learning, can be found, and suggests it may be updated with Large Language Model (LLM) specific tips in the future.
- 💡 The talk emphasizes that the 'cool' trends in machine learning are not always the most impactful or useful, advocating for a focus on fundamentals like data cleaning and SQL queries over chasing the latest techniques.
- 📉 Emmanuel presents a chart illustrating the shift in machine learning practices from training models to fine-tuning and then to using models without any backward pass, suggesting a potential decrease in the prevalence of fine-tuning.
- 🚫 He argues against the idea of fine-tuning as a default solution, especially for adding knowledge to models, proposing that often a simple prompt or context injection can be more effective.
- 📈 The speaker shares research and data indicating that Retrieval-Augmented Generation (RAG) often provides greater performance improvements than fine-tuning alone, especially with larger models.
- 🔑 The importance of understanding the type of knowledge one wants to add to a model is highlighted, with the suggestion that some knowledge may be better suited to prompting rather than fine-tuning.
- 🛠️ Emmanuel stresses that the work of machine learning involves much more than just model training or fine-tuning, including data work, engineering, and infrastructure setup.
- 💼 He discusses the practical considerations of fine-tuning, such as cost and the moving target of model improvements, suggesting that fine-tuning may not always be the most efficient use of resources.
- ⏱️ The talk concludes with a focus on the importance of keeping an eye on trends in machine learning, such as decreasing model costs and increasing context sizes, which may influence the necessity of fine-tuning in the future.
Q & A
What is the main topic of the talk?
-The main topic of the talk is the diminishing importance of fine-tuning in machine learning, particularly in the context of large language models (LLMs).
Who is Emmanuel, and what is his background in machine learning?
-Emmanuel is a speaker with almost 10 years of experience in machine learning. He started as a data scientist, worked in ML education, authored a practical guide on training ML models, and has worked as a staff engineer at Stripe. Currently, he works at Anthropic, where he fine-tunes models and helps understand how they work.
What is mlpower.com, and why is it mentioned in the talk?
-Mlpower.com is a website where you can find Emmanuel's book, which is considered a classic in machine learning and applied machine learning. It is mentioned to promote his work and provide resources for those interested in the subject.
What are the key trends Emmanuel has observed in machine learning over the past 10 years?
-Emmanuel has observed a shift from training models from scratch to fine-tuning pre-trained models, and more recently, to using models that can perform tasks without any fine-tuning. He also notes that the 'cool' trends in machine learning often turn out to be less impactful than the more mundane, foundational work.
What is the main argument against fine-tuning presented in the talk?
-The main argument against fine-tuning is that it is often not the most efficient or effective approach. Emmanuel suggests that fine-tuning is less important than it once was and that other methods, such as prompting and retrieval (RAG), can be more beneficial.
What is RAG, and how does it compare to fine-tuning in the context of the talk?
-RAG (Retrieval-Augmented Generation) is a technique that uses retrieved information to enhance the generation process in language models. In the talk, RAG is often more effective than fine-tuning, especially for larger models, as it leverages context to improve performance.
What is the role of data in machine learning, according to Emmanuel's experience?
-Data plays a crucial role in machine learning. Emmanuel emphasizes that a significant portion of time should be spent on data work, including collecting, labeling, enriching, cleaning, and analyzing data. This is more important than focusing on model training or fine-tuning.
What are some examples of tasks where fine-tuning might still be beneficial?
-Fine-tuning might still be beneficial in specific cases, such as improving performance on a small dataset or when dealing with domain-specific knowledge that is not well-represented in the pre-trained model's training data.
What is the future of fine-tuning according to Emmanuel's 'hot take'?
-Emmanuel's 'hot take' is that fine-tuning is becoming less relevant as models become more capable of handling tasks without fine-tuning. He suggests that the trend might continue, with fewer applications requiring fine-tuning in the future.
How does Emmanuel suggest evaluating whether fine-tuning is necessary for a specific task?
-Emmanuel suggests evaluating the performance of a model with and without fine-tuning, and considering the cost and effort involved. He also recommends focusing on improving prompts and using retrieval techniques like RAG before resorting to fine-tuning.
Outlines
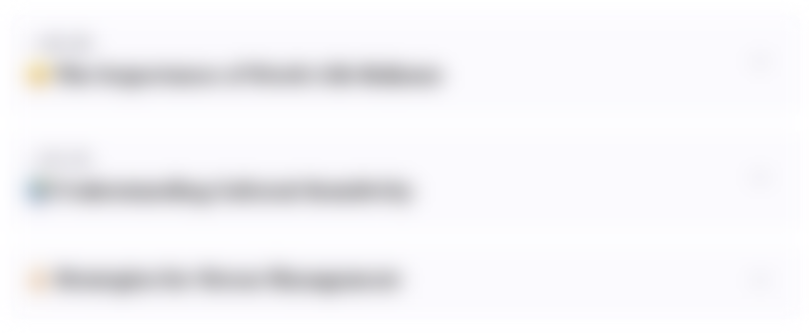
このセクションは有料ユーザー限定です。 アクセスするには、アップグレードをお願いします。
今すぐアップグレードMindmap
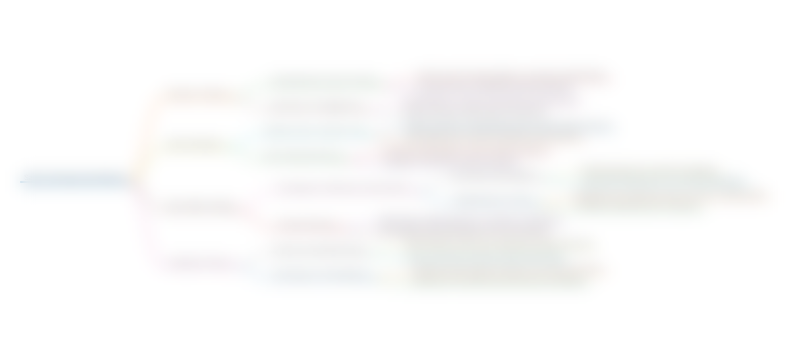
このセクションは有料ユーザー限定です。 アクセスするには、アップグレードをお願いします。
今すぐアップグレードKeywords
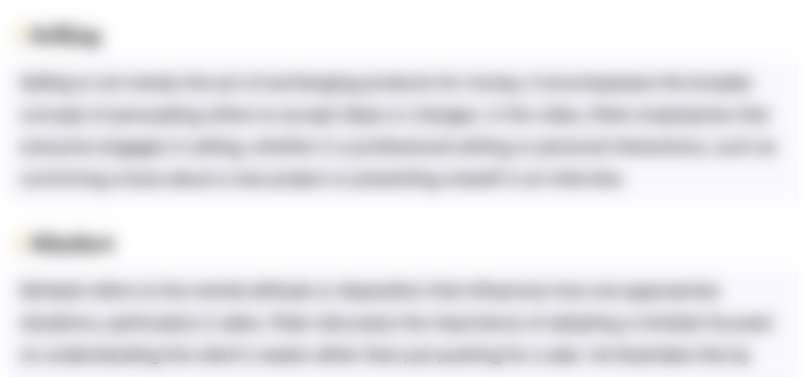
このセクションは有料ユーザー限定です。 アクセスするには、アップグレードをお願いします。
今すぐアップグレードHighlights
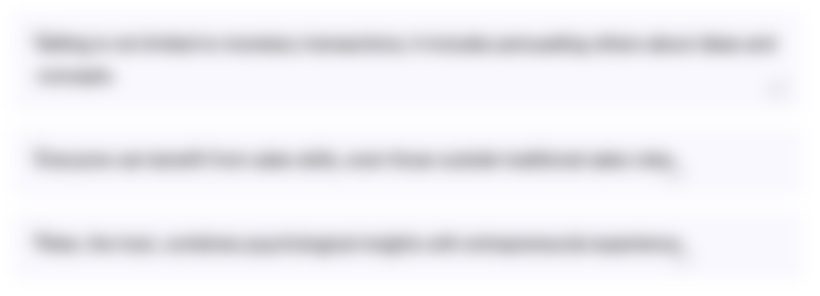
このセクションは有料ユーザー限定です。 アクセスするには、アップグレードをお願いします。
今すぐアップグレードTranscripts
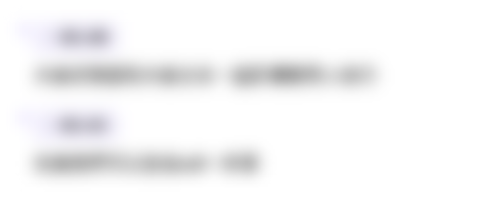
このセクションは有料ユーザー限定です。 アクセスするには、アップグレードをお願いします。
今すぐアップグレード関連動画をさらに表示

LLM Foundations (LLM Bootcamp)
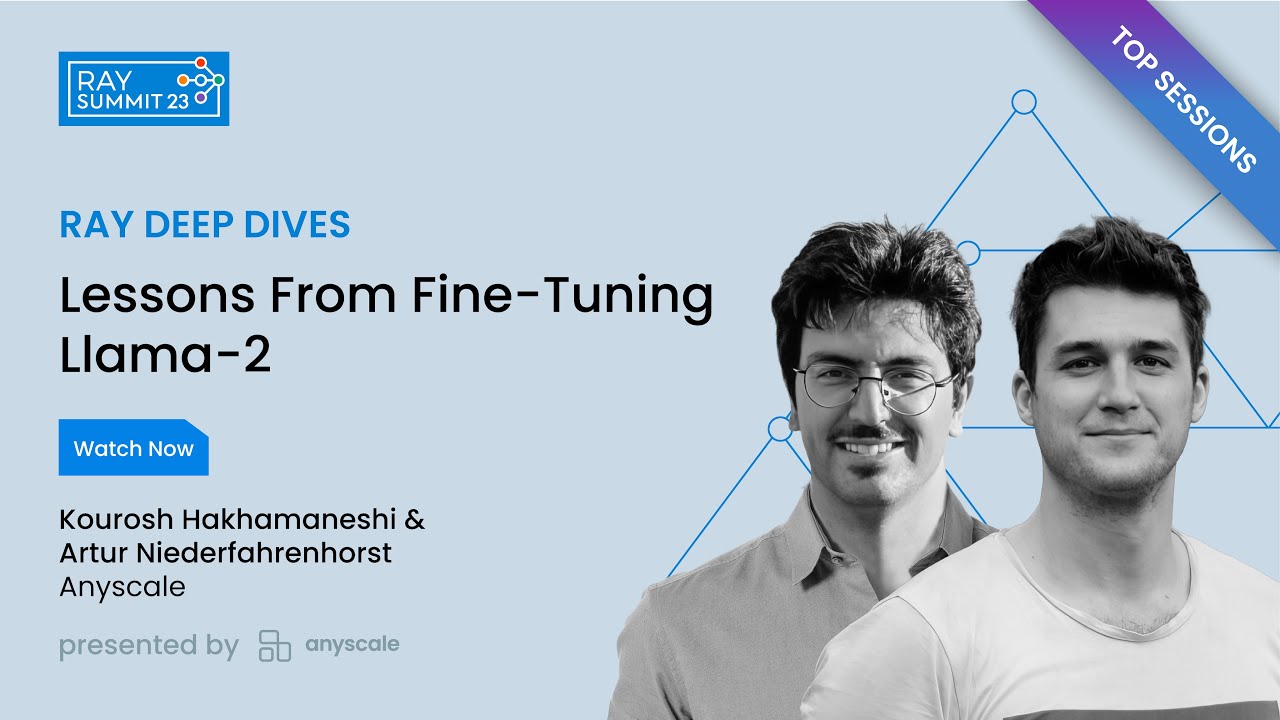
Lessons From Fine-Tuning Llama-2
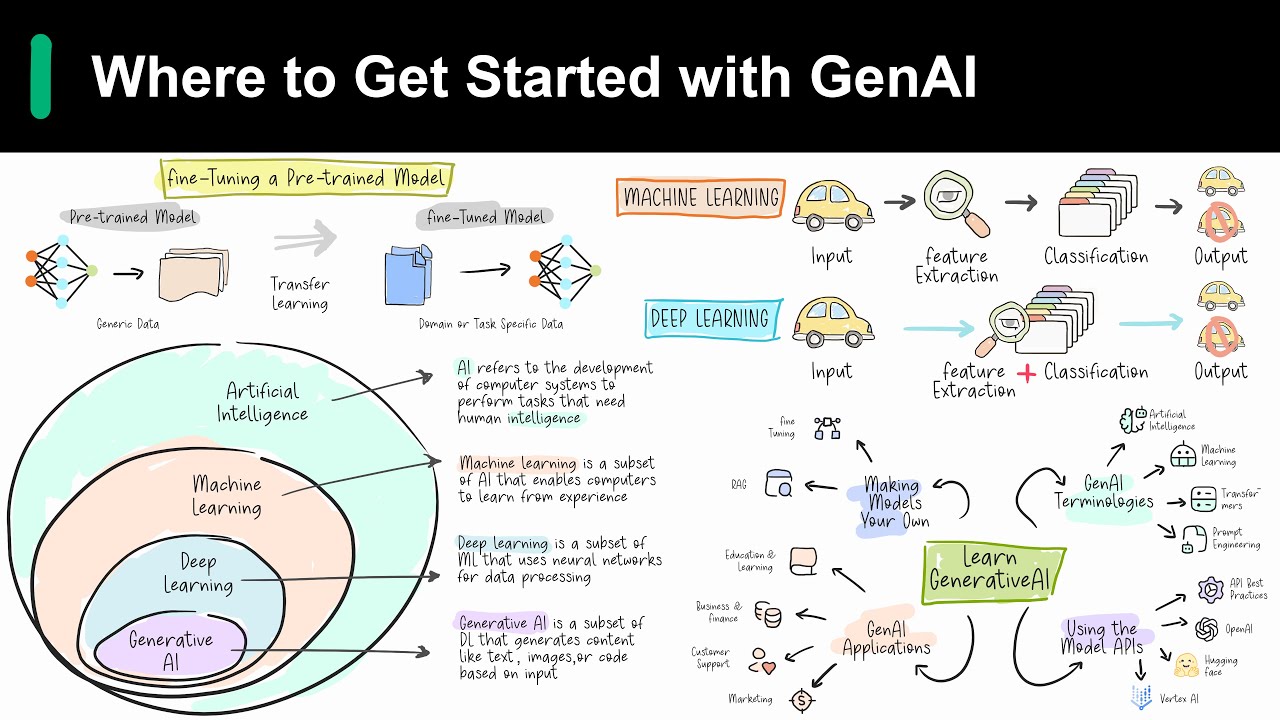
Introduction to Generative AI
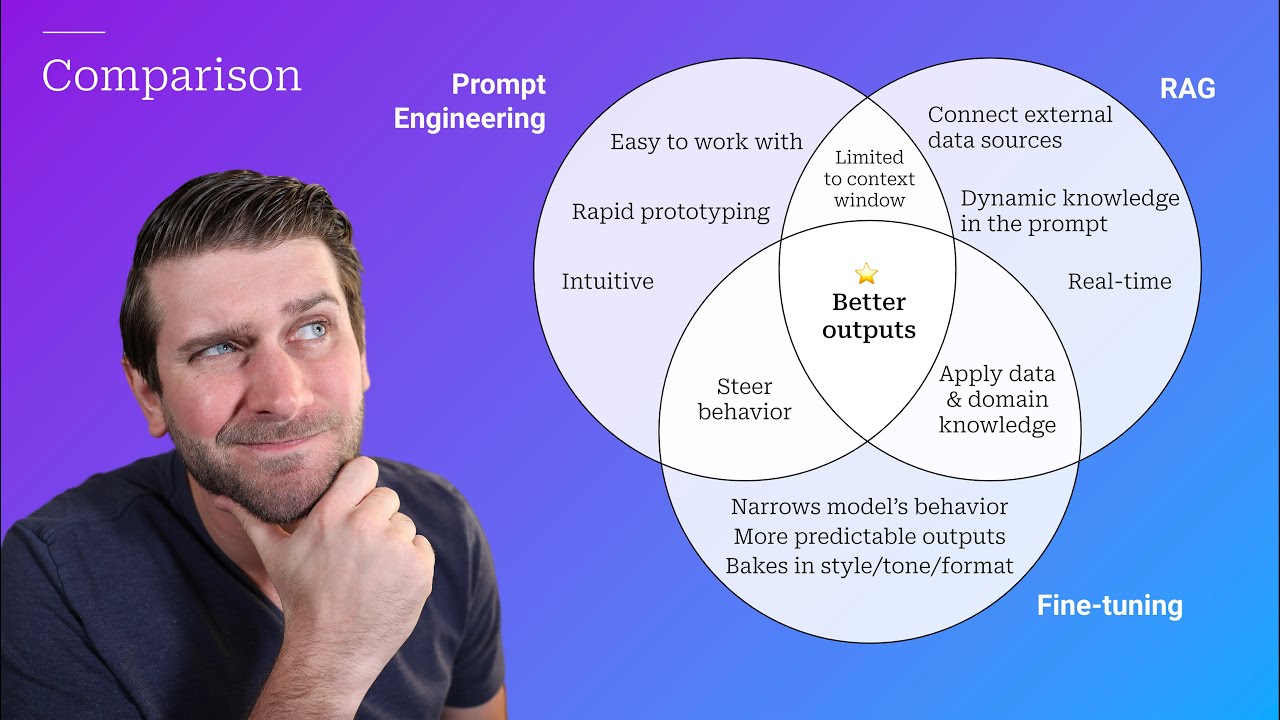
Prompt Engineering, RAG, and Fine-tuning: Benefits and When to Use

Fine-Tuning BERT for Text Classification (Python Code)
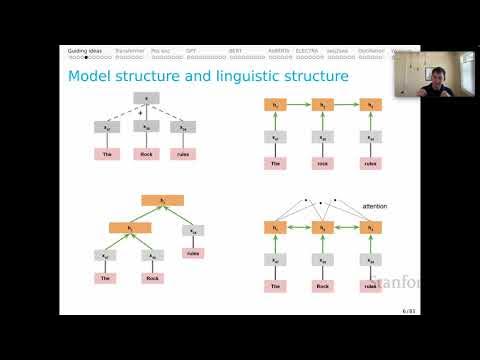
Stanford XCS224U: NLU I Contextual Word Representations, Part 1: Guiding Ideas I Spring 2023
5.0 / 5 (0 votes)