Toward a Deeper Understanding of Our Climate System Through Data Science | Emily Gordon
Summary
TLDR在这段视频中,斯坦福大学的数据科学家Emily Gordon讨论了气候变化这一关键的可持续性领域。她展示了全球海表面温度的年度平均值,并讨论了自1950年以来全球变暖的趋势。Gordon强调了气候模型在理解气候系统和预测温室气体排放影响方面的重要性。她介绍了气候模型如何帮助科学家模拟不同的气候情景,并预测未来气候变化对地区性极端天气事件的影响。此外,她还提到了使用数据科学方法来分析大量气候模型数据,以及如何通过改进模型本身,例如利用神经网络和人工智能技术,来提高气候模型的准确性和效率。
Takeaways
- 🌡️ 全球海表面温度自1950年代以来呈现上升趋势,显示了全球变暖的现象。
- 📊 确定温室气体排放对气候变暖的贡献是一个复杂的问题,需要依赖气候模型来辅助理解。
- 🌐 气候模型是理解气候系统的强大工具,它们通过不同的假设和参数化方法模拟地球系统。
- 🔮 气候模型可以进行各种实验,比如模拟地球升温、降温或模拟火山爆发等极端事件的影响。
- 📉 气候模型预测显示,二氧化碳浓度加倍可能导致2到4摄氏度的全球变暖。
- 📈 气候模型的预测能力在过去几十年中得到了验证,1979年的预测与当前的模型结果相符合。
- 🌍 气候模型可以预测不同气候敏感度下的全球变暖情况,并预测未来可能超过1.5摄氏度的阈值。
- 🌡️ 气候模型还可以用来预测区域尺度上的温度变化,如热浪和极端降雨事件的增加。
- 🔬 构建气候模型需要将地球系统划分为网格盒,并使用参数化方法来处理小于网格盒尺度的物理过程。
- 💾 气候模型产生的数据量巨大,需要先进的数据科学方法来分析和理解这些数据。
- 🌐 未来的工作包括使用现有数据源进行气候预测、训练AI模型快速查询气候影响、以及改进气候模型本身。
Q & A
全球海表面温度的变化趋势是什么?
-从1950年代开始,全球海表面温度呈现上升趋势,这表明了全球变暖的现象。
温室气体排放对全球变暖的贡献有多大?
-温室气体排放是导致全球变暖的主要原因之一,但实际观测到的变暖幅度略低于气候模型预测的仅由温室气体排放引起的变暖。
气候模型是如何帮助我们理解气候变化的?
-气候模型通过模拟地球系统的不同假设和过程,使我们能够进行实验,比如增加温室气体浓度,观察地球系统的响应,从而帮助我们理解气候变化。
气候模型的预测准确性如何?
-气候模型的预测在历史上显示出相当的准确性。例如,1979年的预测与后来的观测结果相比,预测的变暖范围与实际观测相当接近。
气候模型中的“敏感性”是什么意思?
-气候模型的敏感性指的是模型对二氧化碳浓度变化的反应程度,高敏感性模型预测的变暖幅度更大。
气候模型如何帮助我们理解区域气候变化的影响?
-气候模型可以链接到区域尺度,帮助我们理解不同水平的全球变暖对热浪和极端降雨事件等区域气候影响。
构建气候模型需要考虑哪些因素?
-构建气候模型需要考虑地球系统的网格划分、子网格尺度的过程(如云和地形)、辐射方案的准确性,以及通过参数化来解决小尺度过程。
气候模型产生的数据量有多大?
-最新的气候模型实验产生了约20拍字节(petabytes)的数据,这显示了气候模型产生的数据量是巨大的。
如何使用现有的气候模型数据进行科学研究?
-可以使用现有的气候模型数据来训练仿真器和AI,快速有效地查询特定年份或变量的区域气候变化影响。
气候模型中存在哪些挑战和改进方向?
-气候模型中的挑战包括处理大量数据、理解内部气候过程、减少系统性偏差以及提高模型的可信度和稳健性。改进方向包括使用AI和神经网络来改进子网格尺度的参数化。
气候模型如何帮助我们应对未来的气候变化?
-气候模型不仅可以帮助我们预测未来的气候变化,还可以通过数据驱动的方法进行科学发现,提高模型的准确性和效率。
Outlines
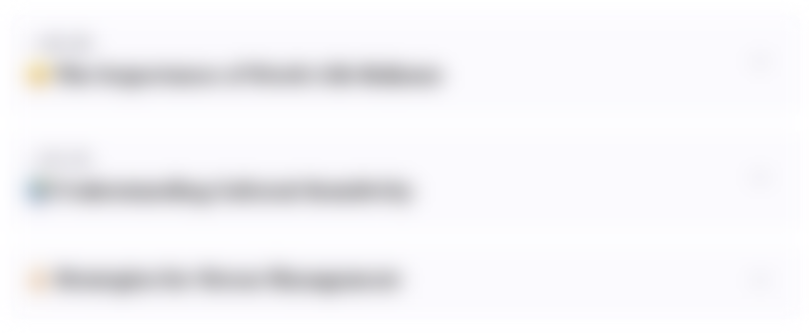
このセクションは有料ユーザー限定です。 アクセスするには、アップグレードをお願いします。
今すぐアップグレードMindmap
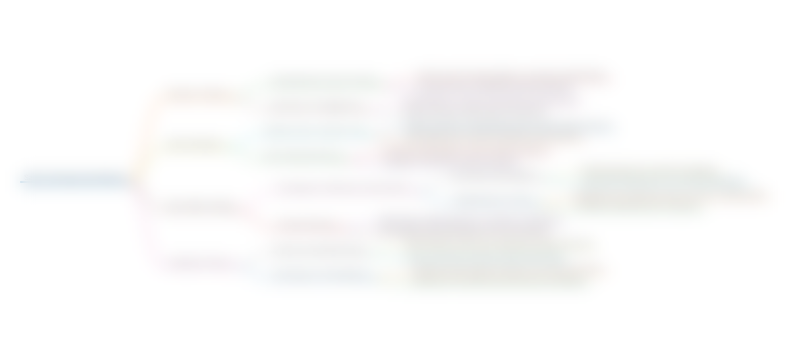
このセクションは有料ユーザー限定です。 アクセスするには、アップグレードをお願いします。
今すぐアップグレードKeywords
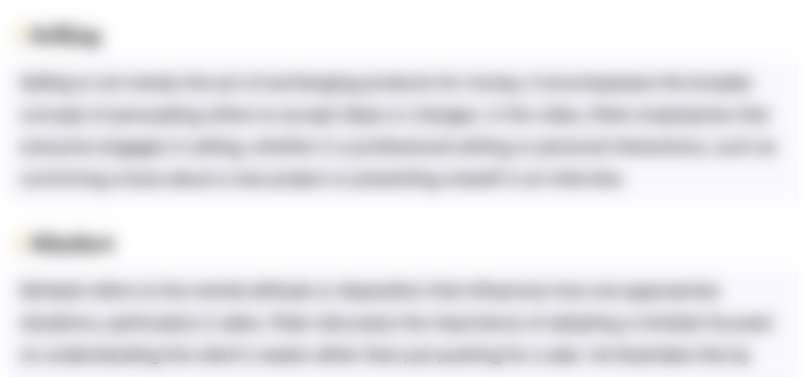
このセクションは有料ユーザー限定です。 アクセスするには、アップグレードをお願いします。
今すぐアップグレードHighlights
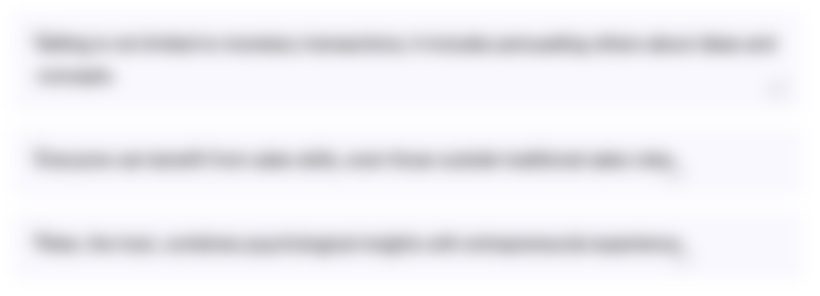
このセクションは有料ユーザー限定です。 アクセスするには、アップグレードをお願いします。
今すぐアップグレードTranscripts
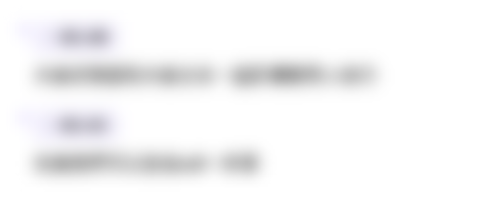
このセクションは有料ユーザー限定です。 アクセスするには、アップグレードをお願いします。
今すぐアップグレード5.0 / 5 (0 votes)