2023A Kelompok 5 Statistik Non Parametrik
Summary
TLDRGroup 5 presents a comprehensive overview of non-parametric statistics, highlighting its significance in analyzing data without strict distribution assumptions. The discussion contrasts non-parametric with parametric methods, emphasizing their flexibility, especially for small sample sizes and ordinal data. Key tests like the sign test and Mann-Whitney U test are introduced, along with their respective applications. The advantages include fewer assumptions and resilience to outliers, while limitations include lower statistical power. Overall, the presentation underscores the importance of non-parametric methods in various research contexts, providing valuable insights for effective data analysis.
Takeaways
- 📊 Non-parametric statistics do not rely on assumptions about data distribution, making them useful for small sample sizes.
- 🔍 Unlike parametric statistics, non-parametric methods can analyze ordinal and nominal data effectively.
- 📏 Key differences: Parametric requires normal distribution; non-parametric is more flexible with data types.
- 📉 Non-parametric tests are ideal for situations with limited data or when normality cannot be assumed.
- ⚖️ Examples of non-parametric tests include the Sign Test, Median Test, and Kruskal-Wallis Test.
- 🛠️ Advantages of non-parametric statistics include fewer assumptions and robustness against outliers.
- ⚠️ Disadvantages include lower power compared to parametric tests when the latter's assumptions are met.
- 📝 Steps in non-parametric analysis include data collection, understanding data types, and selecting appropriate tests.
- 🔄 Non-parametric tests can be applied to paired samples, independent samples, or multiple groups.
- 💡 Understanding when to use non-parametric methods enhances research validity and reliability.
Q & A
What is non-parametric statistics?
-Non-parametric statistics refers to statistical methods that do not assume a specific distribution for the data, making them useful for analyzing data that is nominal or ordinal, particularly when sample sizes are small.
When should non-parametric methods be used?
-Non-parametric methods are used when the sample size is very small, when data is ordinal, or when the data does not meet the assumptions required for parametric tests, such as normal distribution.
What are the main differences between parametric and non-parametric statistics?
-Parametric statistics assume that samples come from populations with normal distributions and have similar variances, while non-parametric statistics do not require these assumptions and can analyze smaller samples or non-normal data.
Can you give examples of non-parametric tests?
-Examples of non-parametric tests include the sign test, Wilcoxon signed-rank test, and Kruskal-Wallis test, which are used to compare medians and ranks of data.
What are the advantages of using non-parametric statistics?
-Advantages include fewer assumptions, robustness against outliers, and applicability to ordinal and nominal data, as well as ease of manual calculation without specialized software.
What are some disadvantages of non-parametric statistics?
-Disadvantages include lower statistical power compared to parametric methods when the assumptions for parametric tests are met, and they may not effectively analyze larger datasets.
What types of data are suitable for non-parametric methods?
-Non-parametric methods are suitable for nominal data, ordinal data, and situations where the assumptions of parametric methods cannot be satisfied.
What steps are involved in conducting non-parametric statistical analysis?
-Key steps include collecting relevant data, understanding the type of data, conducting assumption tests, selecting appropriate methods, preparing the data for analysis, executing statistical tests, and interpreting the results.
What is the significance of choosing the correct non-parametric test?
-Choosing the correct non-parametric test is crucial for valid results, as different tests are designed for specific types of data relationships, such as paired or independent samples.
How does one interpret the results from non-parametric tests?
-Results from non-parametric tests are interpreted based on p-values, which indicate whether there is a statistically significant difference between groups, along with the context of the research question.
Outlines
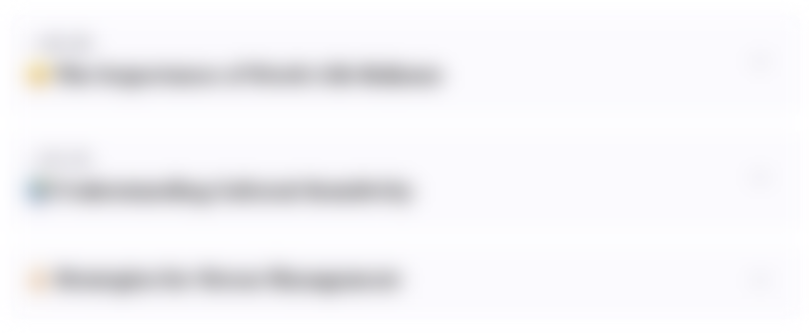
このセクションは有料ユーザー限定です。 アクセスするには、アップグレードをお願いします。
今すぐアップグレードMindmap
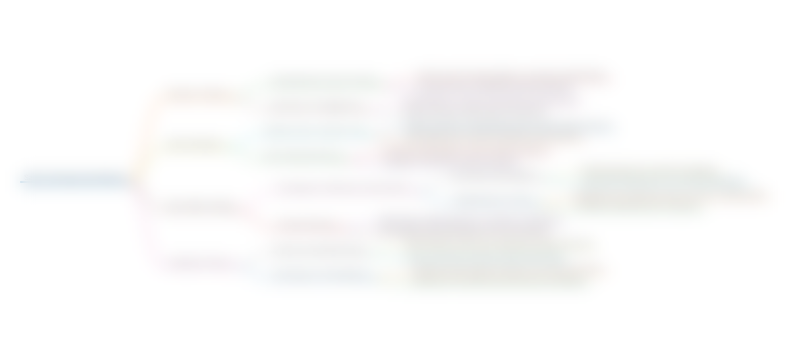
このセクションは有料ユーザー限定です。 アクセスするには、アップグレードをお願いします。
今すぐアップグレードKeywords
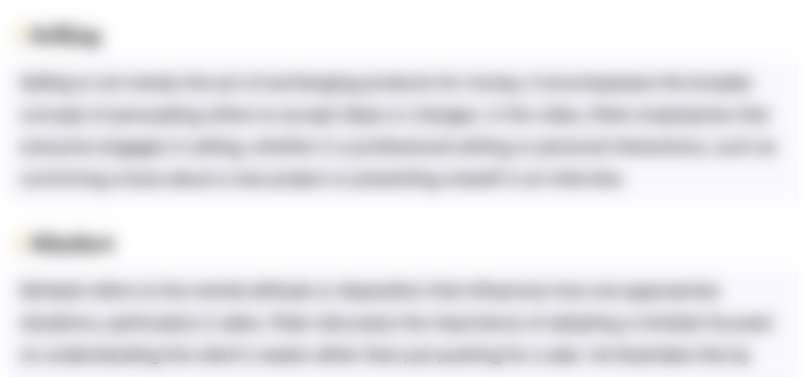
このセクションは有料ユーザー限定です。 アクセスするには、アップグレードをお願いします。
今すぐアップグレードHighlights
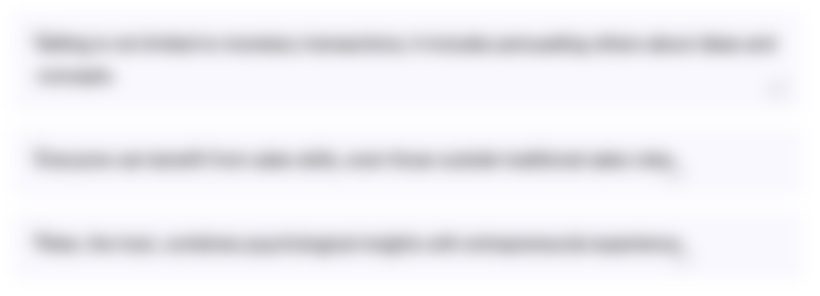
このセクションは有料ユーザー限定です。 アクセスするには、アップグレードをお願いします。
今すぐアップグレードTranscripts
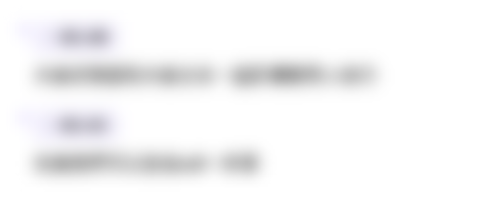
このセクションは有料ユーザー限定です。 アクセスするには、アップグレードをお願いします。
今すぐアップグレード関連動画をさらに表示
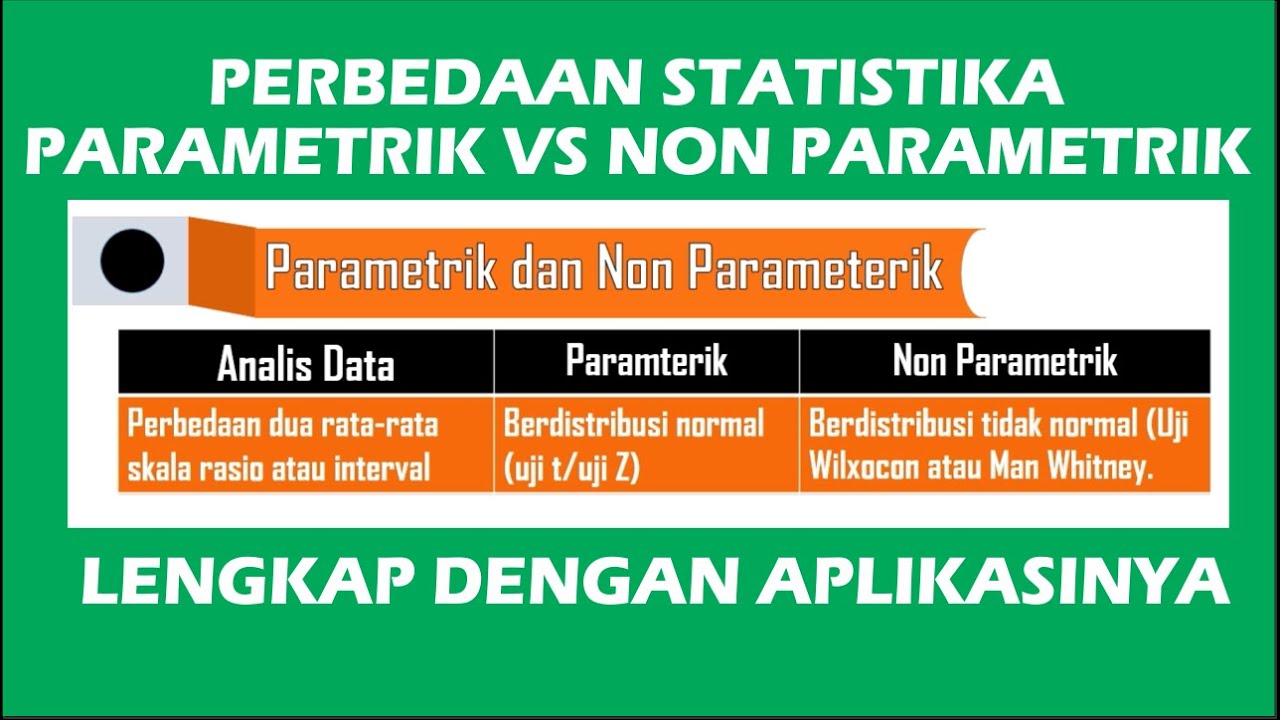
Perbedaan Statistika Parametrik dan Non Parametrik
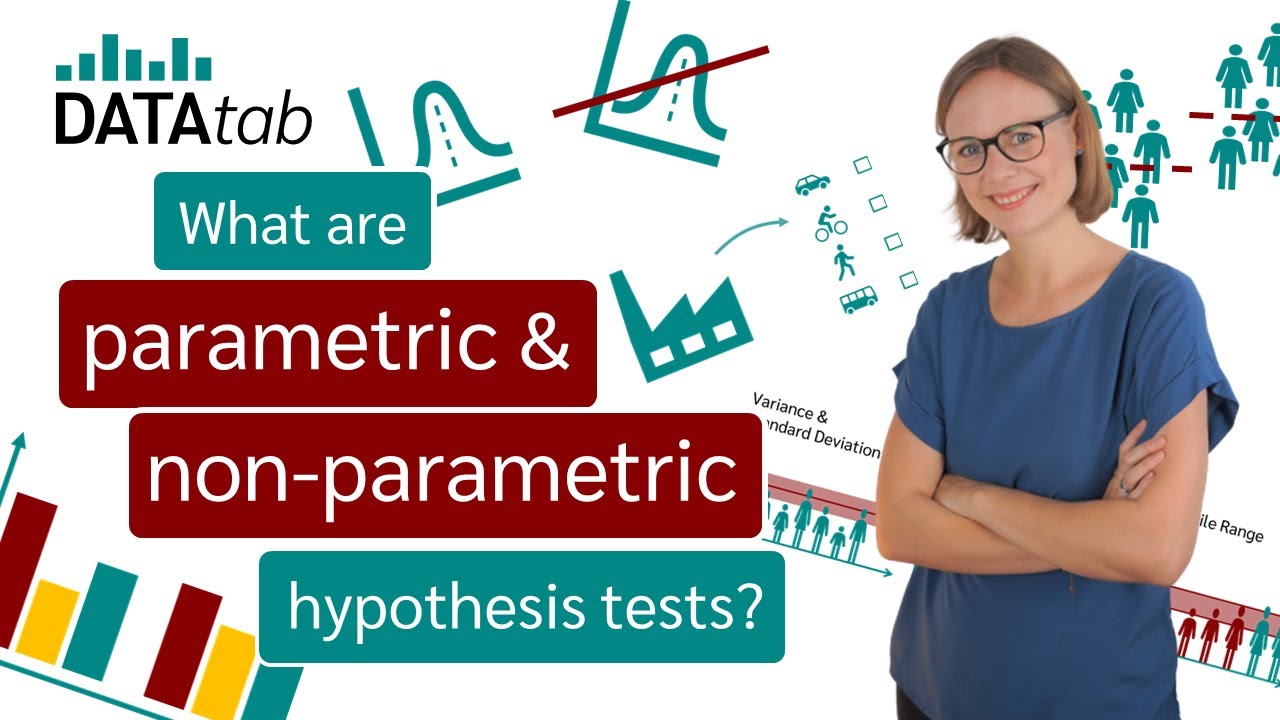
What is the difference between parametric and nonparametric hypothesis testing?
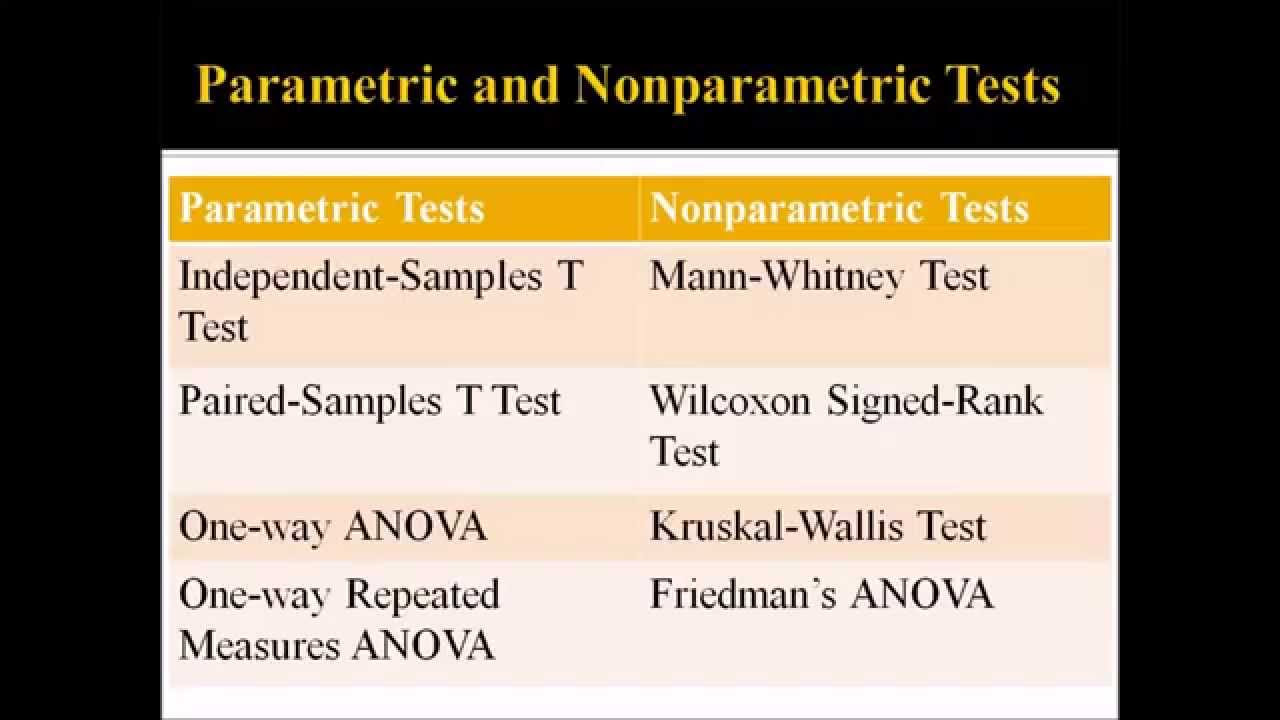
Parametric and Nonparametric Statistical Tests
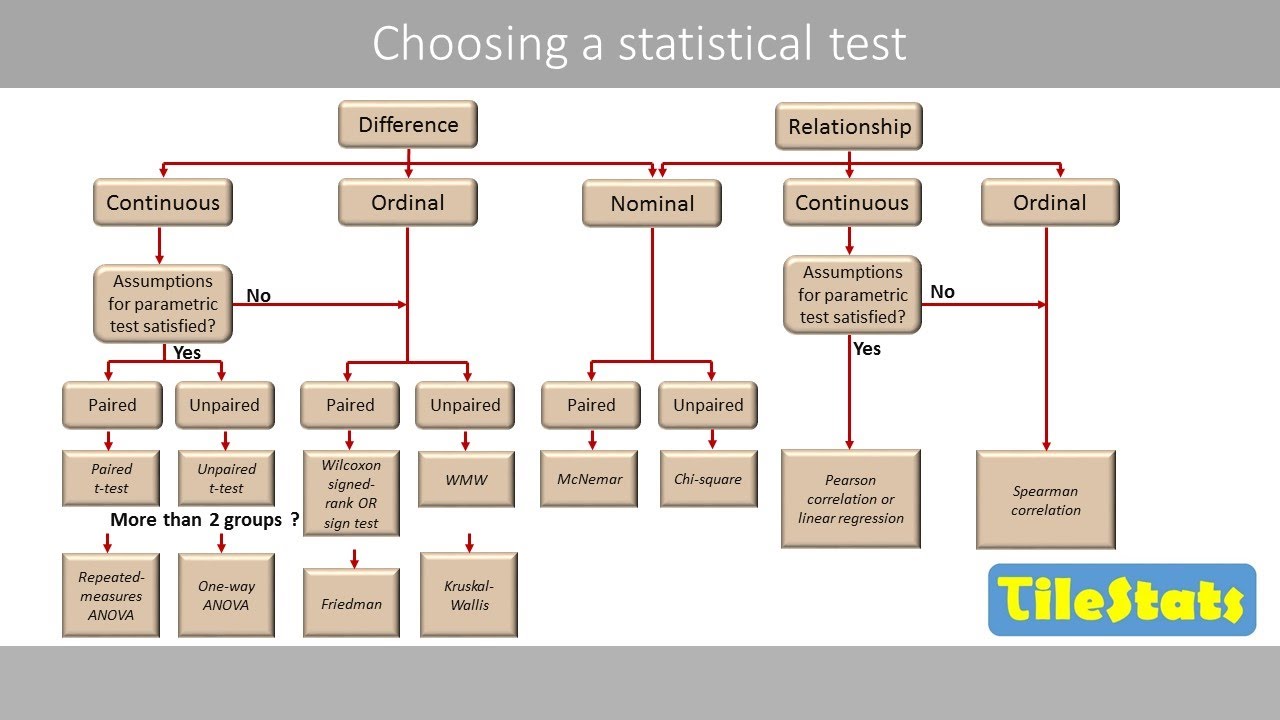
How to choose an appropriate statistical test
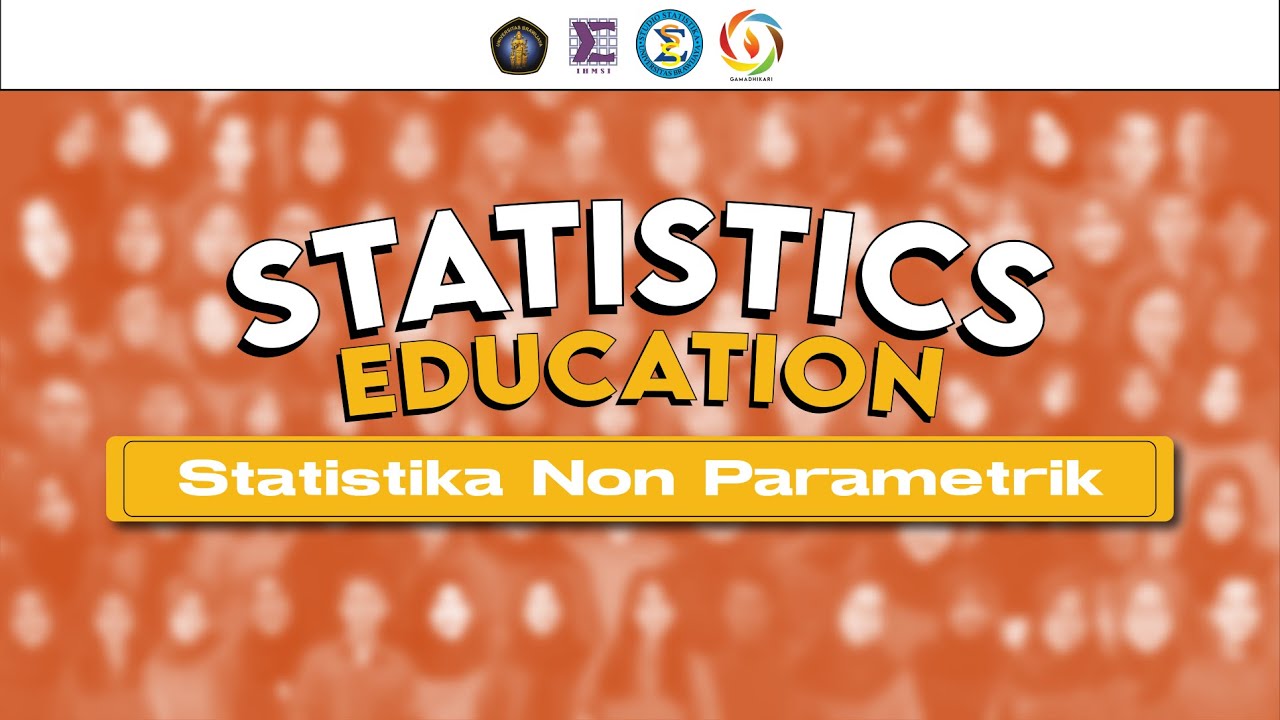
Statistika Non Parametrik
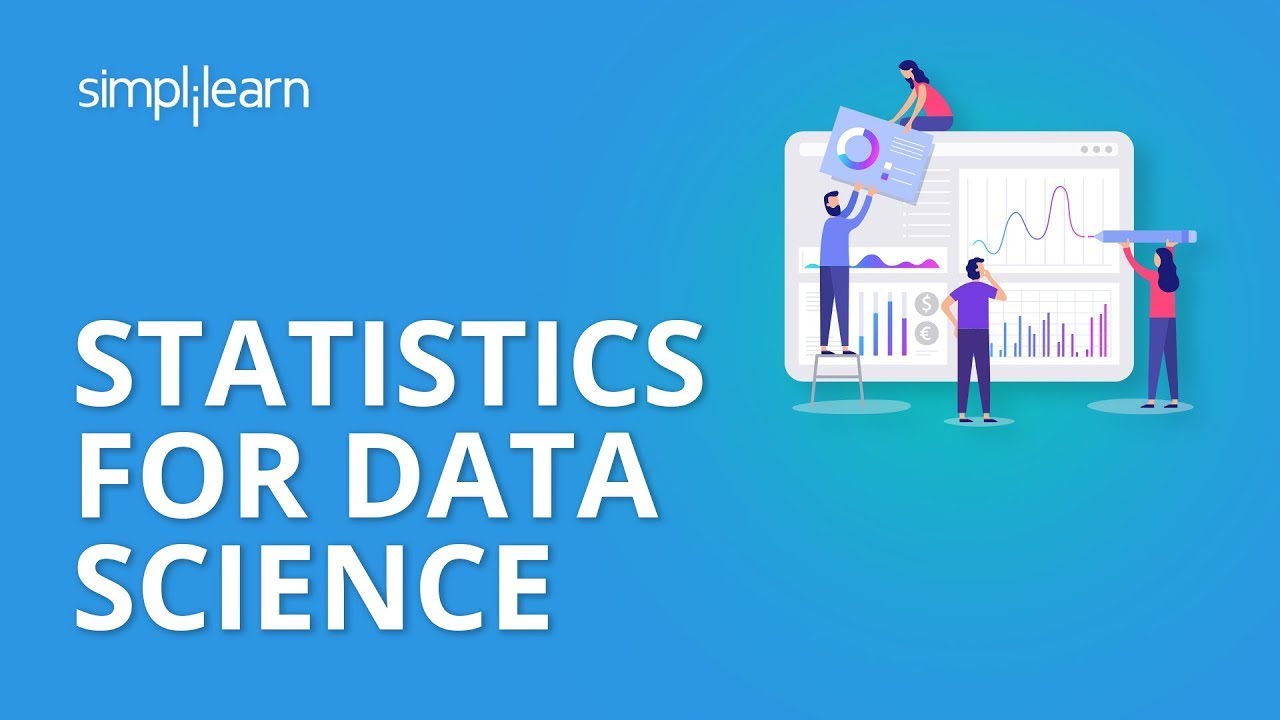
Statistics For Data Science | Data Science Tutorial | Simplilearn
5.0 / 5 (0 votes)