How to Use LangSmith to Achieve a 30% Accuracy Improvement with No Prompt Engineering
Summary
TLDRビデオの要約を以下のようにまとめます。ハリソン氏は、Lang chainのチームメンバーであるdosuが、プロンプトエンジニアリングを行わずにアプリケーションのパフォーマンスを30%向上させた方法について、ブログをリリースしたと語ります。彼らが使用したのは、Lang Smithというプラットフォームで、これはLang chainとは別であり、オープンソースで作動します。Lang Smithは、アプリケーションのデータフローを改善するためのツール群を組み合わせています。これには、アプリケーションを通過するデータのログ記録、トレース、テスト、バリデーション、プロンプトハブ、人間による注釈などが含まれます。彼らは、分類タスクに取り組み、OpenAIクライアントを直接使用して、問題のタイプを分類しました。また、Lang Smithを使用して、実行に関連付けられたフィードバックを残す方法を説明し、その後、そのフィードバックを使用してアプリケーションのパフォーマンスを向上させるプロセスを紹介します。さらに、彼らは、セマンティック検索を介して、類似した例を通じて入力に応じた出力を提供する手法も使用しました。この方法は、分類タスクにおいては単純な例ですが、より複雑なタスクにも適用可能です。
Takeaways
- 🚀 ハリソンからランチェーンのブログを紹介。dosuがアプリケーションのパフォーマンスを30%向上させた方法について解説します。
- 🛠️ dosuが使用したのはLang Smithというプラットフォームで、ロギング、トレース、テスト、バリデーションを統合したツールを使用しました。
- 🔍 Lang Smithは、アプリケーションのデータフローを改善するための機能を組み合わせたものです。
- 📈 dosuは分類タスクを30%向上させるために、Lang Smithの機能を活用してフィードバックを収集し、アプリケーションを改善しました。
- ⚙️ 環境変数の設定から始め、Lang Smithプロジェクトにデータをログする方法を学びます。
- 📝 アプリケーションはOpenAIクライアントを使用して分類タスクを実行し、Lang Smithで追跡します。
- 🔁 フィードバック機能を使って、実行結果に問題がある場合に修正を提案できます。
- 🔢 Lang Smithで自動化ルールを設定し、フィードバックを持つデータをデータセットに移動させます。
- 🔧 データセットから良い例を引き出し、アプリケーションに取り入れることでパフォーマンスを向上させます。
- 🤖 モデルは以前のパターンから学習し、新しい入力に対しても適切な分類を行えるようになります。
- 🔍 dosuは、類似した例を用いたセマンティック検索を行い、入力に最も関連性のある例を適用しました。
- 📚 フィードバックループを構築し、アプリケーションのパフォーマンスを継続的に改善していく方法が示されています。
Q & A
dosuがアプリケーションのパフォーマンスをどのように改善しましたか?
-dosuは、プロンプトエンジニアリングなしでアプリケーションのパフォーマンスを30%向上させました。これは、Lang chainが過去数ヶ月間に構築したツールを大幅に使用して行われました。
Lang Smithとは何ですか?
-Lang Smithは、Lang chainとは別個のプラットフォームで、Lang chainと一緒に使用したり、独立して使用したりすることができます。アプリケーションのデータフローを改善するための機能の組み合わせを提供しています。
Lang Smithの強力な点は何ですか?
-Lang Smithの強力な点は、ロギング、トレース、テスト、バリデーション、プロンプトハブ、人間アノテーションキューなどの機能がすべて別々のものではなく、一つのプラットフォームに統合されていることです。
チュートリアルでdosuが30%の増加を実現するために行ったタスクは何ですか?
-チュートリアルでdosuが行ったタスクは分類です。これは、LLM(大規模言語モデル)の標準で比較的簡単なタスクです。
アプリケーションで使用されているプロンプトテンプレートとは何ですか?
-アプリケーションで使用されているプロンプトテンプレートは、問題のタイプを以下のトピックの1つとして分類するというものです。トピックには、バグ、改善、新機能、ドキュメンテーション、または統合が含まれます。
Lang Smithでフィードバックを残す方法とは何ですか?
-Lang Smithでは、実行に関連付けられたフィードバックを残すことができます。これは、特定の実行IDを使用して行われ、後からその実行に関連付けられたフィードバックを残すことができるように設計されています。
データセットにデータを移動するために設定されたルールは何ですか?
-データセットにデータを移動するために設定されたルールは、フィードバックが関連付けられたデータポイントをデータセットに移動させるものです。正しいフィードバックは「user score is one」で、これは正しい分類を意味し、修正されたフィードバックは「correction」を使用して行われます。
ルールがトリガーされるまで待つ必要がありますか?
-はい、ルールはデフォルトで5分ごとに実行されます。ルールが設定された後には、同じデータポイントを再実行し、ルールが適用されるまで待つ必要があります。
アプリケーションのパフォーマンスを向上させるために使用されるデータポイントは何ですか?
-アプリケーションのパフォーマンスを向上させるために使用されるデータポイントは、Lang Smithのデータセットから取得される正しい値のデータセットです。これには、フィードバックに基づく修正された値が含まれます。
アプリケーションで使用されるプロンプトテンプレートはどのように変更されましたか?
-アプリケーションで使用されるプロンプトテンプレートは、新しい方法で変更されました。具体的には、2行の例が追加され、その後に例のプレースホルダーが置かれています。これは、以前のパターンから学習し、新しい入力に対して応じて応答をカスタマイズするようにモデルに学習させます。
dosuが行った意味のあるセマンティックサーチとは何ですか?
-dosuが行ったセマンティックサーチは、数百のデータポイントの良いフィードバックと修正済みフィードバックをlsmithに記録し、その中から5つまたは10つの例をランダムに選択するのではなく、現在の入力に最も類似している例を選択することでした。これは、類似した入力を持つ場合、出力を同じまたは似たものにするべきであり、新しい入力に適用されるべきロジックが、それらの入力に適用されるロジックに似ていることを意味します。
このチュートリアルで説明されているプロセスはどのようにして他の複雑なタスクにも適用できますか?
-このチュートリアルで説明されているプロセスは、分類タスクに適用されており、比較的単純な例です。しかし、Lang chainのチームは、これらの同じ概念がより複雑なタスクにも関連性があると信じており、それらを試すことに興味を持っています。
Outlines
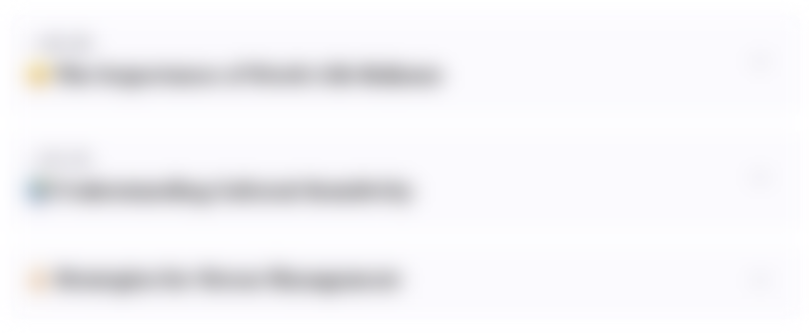
このセクションは有料ユーザー限定です。 アクセスするには、アップグレードをお願いします。
今すぐアップグレードMindmap
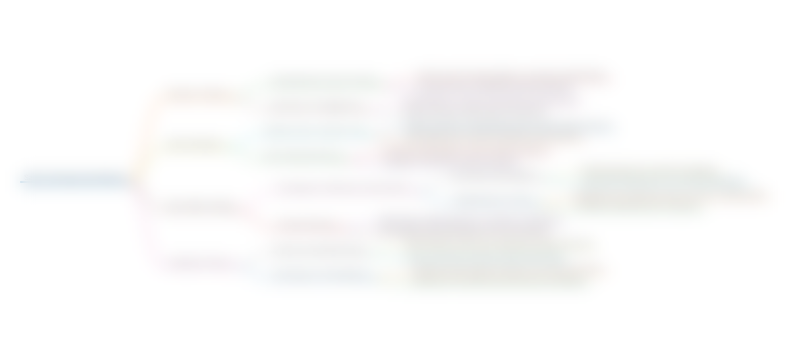
このセクションは有料ユーザー限定です。 アクセスするには、アップグレードをお願いします。
今すぐアップグレードKeywords
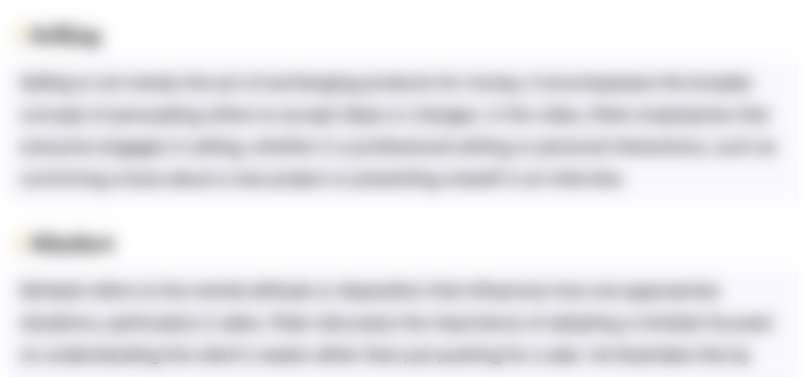
このセクションは有料ユーザー限定です。 アクセスするには、アップグレードをお願いします。
今すぐアップグレードHighlights
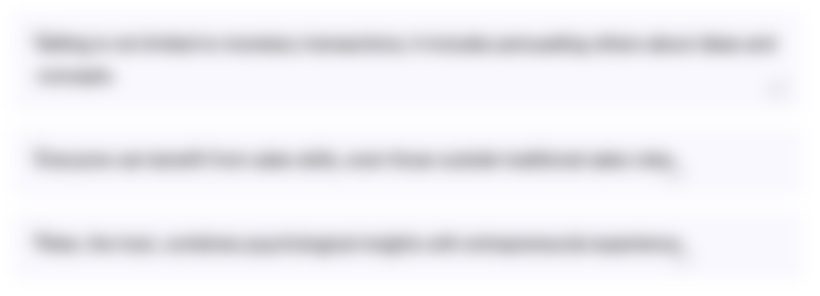
このセクションは有料ユーザー限定です。 アクセスするには、アップグレードをお願いします。
今すぐアップグレードTranscripts
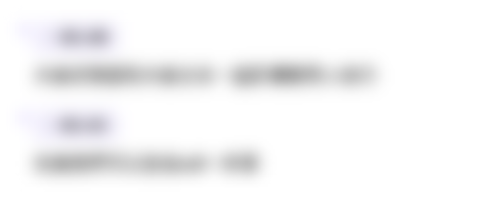
このセクションは有料ユーザー限定です。 アクセスするには、アップグレードをお願いします。
今すぐアップグレード関連動画をさらに表示
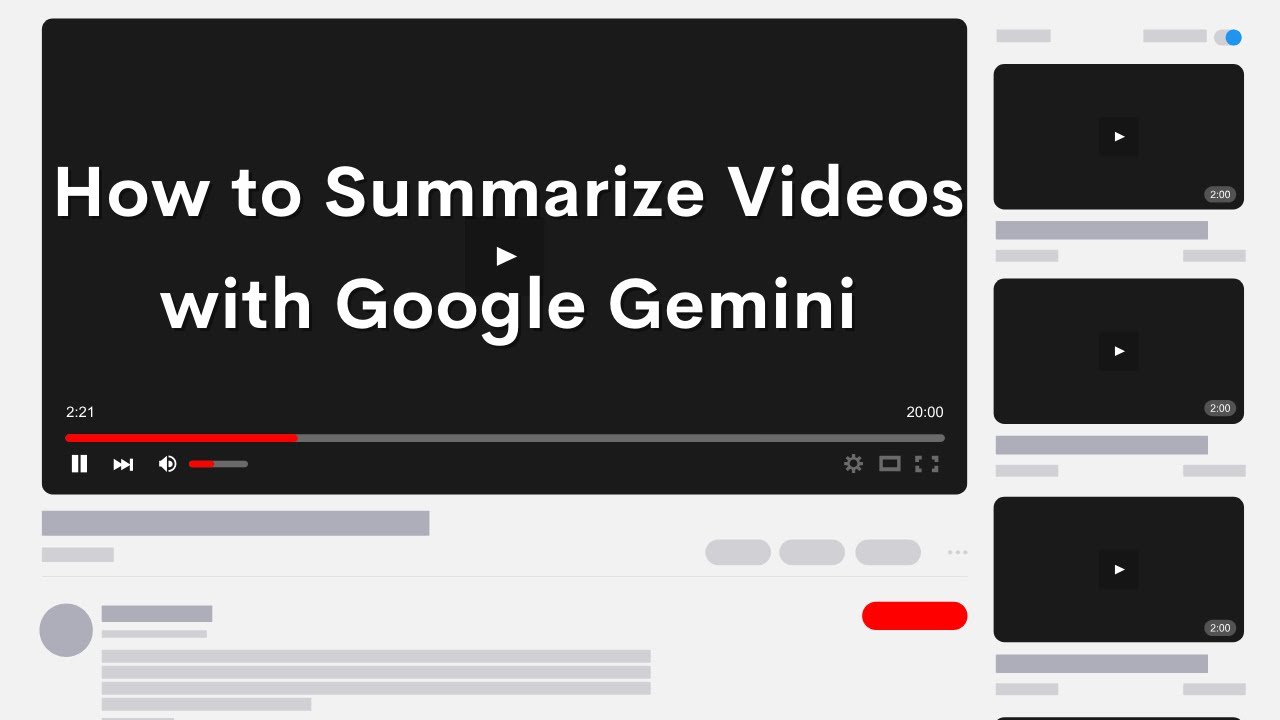
How to Use Google Gemini to Summarize a Video

ちーにゃチャンネル使用BGM
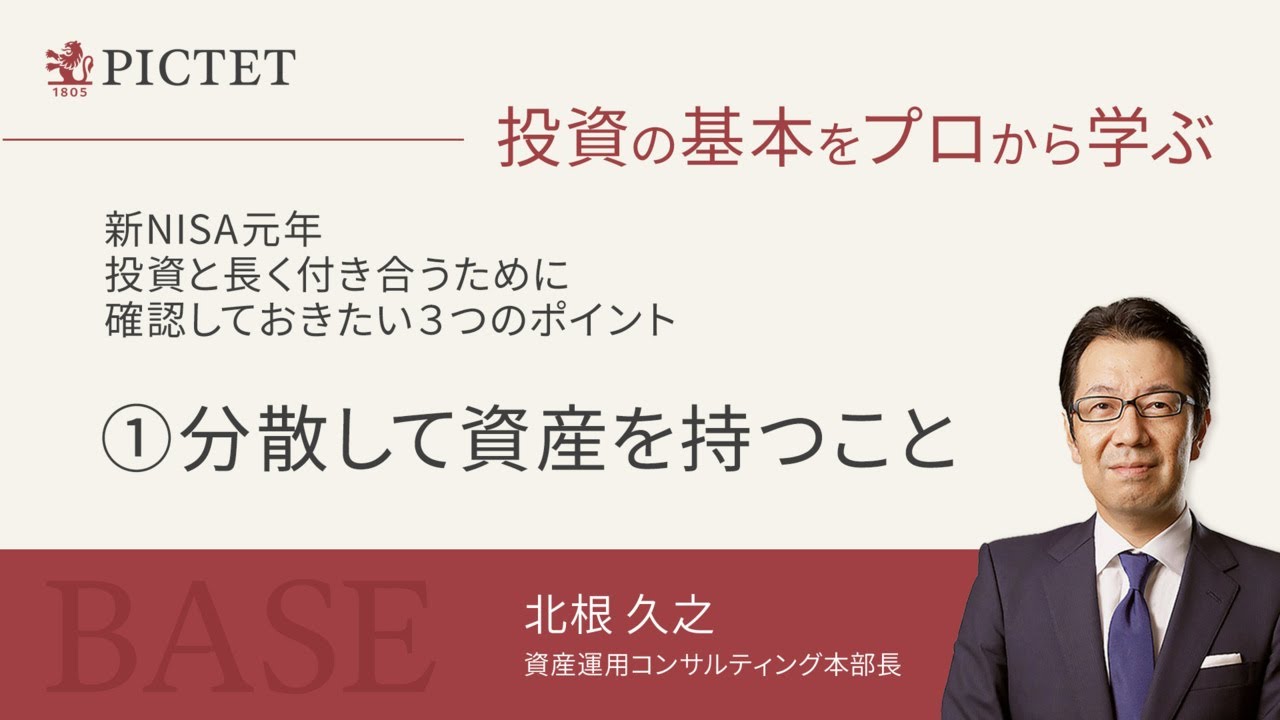
①分散して資産を持つこと「 新NISA元年 投資と長く付き合うために確認しておきたい3つのポイント」<北根久之>|投資の基本をプロか学ぶBASE 2024.2

青色専従者給与は、いくらがお得?節税効果が最大になる金額設定を解説します

Sewage scandal: how will dirty water affect the election?
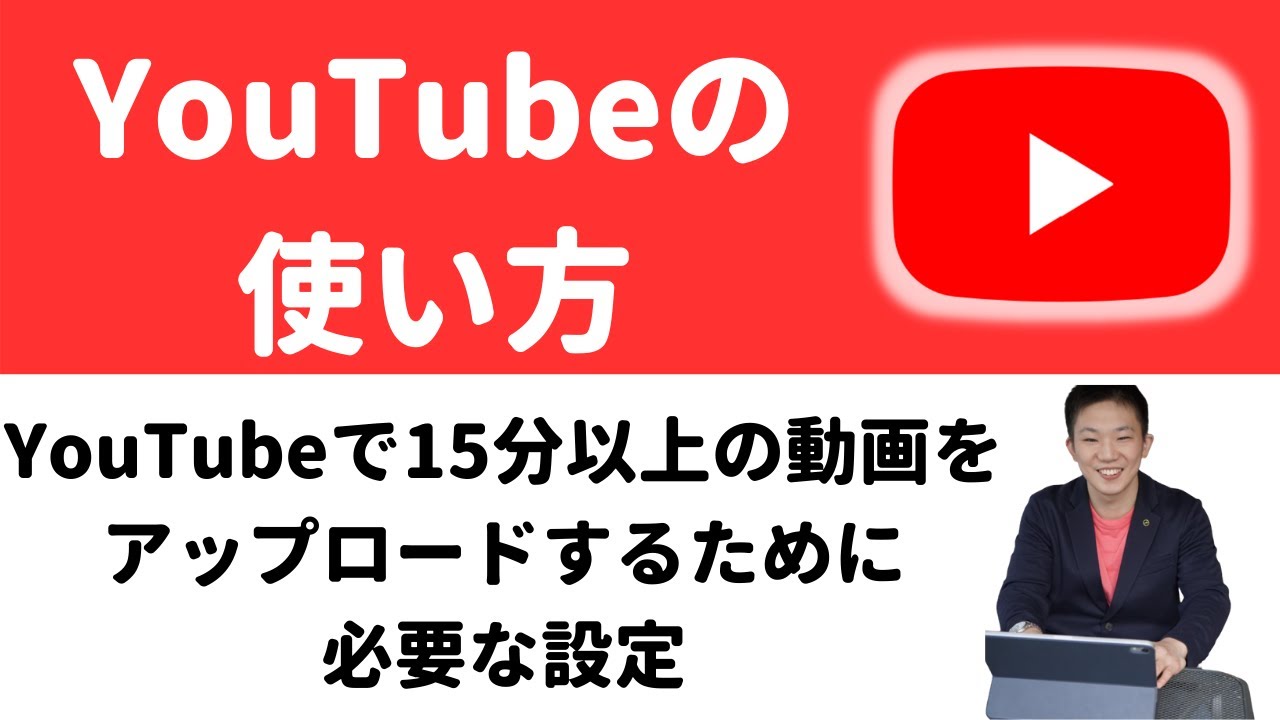
【必見!】Youtubeで15分以上の動画をアップロードするために必要な設定

I Was WRONG: CHANGE YOUR ROCKET LEAGUE SETTINGS...
5.0 / 5 (0 votes)