AI Pioneer Shows The Power of AI AGENTS - "The Future Is Agentic"
Summary
Please replace the link and try again.
Takeaways
- 🧠 Dr. Andrew Ngは人工知能のエージェントに非常に前向きで、GPT 3.5やGPT 4を動力源としたエージェントが論理的思考を行えるレベルに達する可能性があると述べています。
- 🎓 Dr. Andrew Ngはコンピューターサイエンティストであり、Google Brainの共同創設者、かつBuuの前チーフサイエンティストとして人工知能の分野で先駆的な存在です。
- 🌐 Ngが共同創設したCourseraは、コンピューターサイエンスや数学など多岐にわたる分野について無料で学ぶことができます。
- 💡 Sequoia Capitalはシリコンバレーで最も伝説的なベンチャーキャピタルの一つであり、彼らのポートフォリオはNASDAQ市場資本額の25%を占めています。
- 🔍 非代理的ワークフローでは、プロンプトを入力すると生成された回答がすぐに返されますが、代理的ワークフローでは、AIがイテレーションを通じて回答を改善することが可能です。
- 🤖 代理的ワークフローでは、複数のエージェントが異なるロールやツールを使ってタスクを共同でイテレートし、最終的な最良の結果を生み出すことができます。
- 📈 ゼロショットプロンプティングでは、AIにコードを生成させると48%の正解率だった一方、代理的ワークフローを用いたGPT 3.5はGPT 4を上回る性能を発揮しました。
- 🛠 反射(reflection)は、言語モデルに対して生成した出力を改善する方法を見つけ出させるプロセスであり、これによりモデルのパフォーマンスが向上します。
- 🔧 ツール使用(tool use)は、AIに特定のツールを用いさせて特定のタスクを遂行させる方法で、これは言語モデルが新しい機能を獲得する手段です。
- 📝 計画(planning)と多代理協作(multi-agent collaboration)は、AIがより良い結果を生み出すために、ステップを計画し、複数のエージェントが協力するワークフローです。
- 🔑 Andrew Ngの講演では、これらの代理的設計パターンが今年AIが遂行できるタスクのセットを劇的に拡大する可能性があると示唆しています。
- 🚀 代理的ロジックとワークフローは、人工知能の将来において重要な役割を果たし、AGI(人工一般知能)への道のりにおいて一歩進む助けとなるでしょう。
Q & A
Dr. Andrew Ngはどのような人物ですか?
-Dr. Andrew Ngはコンピューターサイエンティストで、Google Brainの共同創設者で元チーフサイエンティスト、またオンライン学習プラットフォームCourseraの創設者であり、人工知能の分野で重要な影響を与えている主要な思想家です。
Sequoia Capitalはどのような企業ですか?
-Sequoia Capitalはシリコンバレーの伝説的なベンチャーキャピタル企業で、彼らのポートフォリオ企業はナスダック市場資本総額の25%を占めています。
Dr. Andrew Ngが語るエージェントとは何を指していますか?
-Dr. Andrew Ngが語るエージェントとは、人工知能の未来を形作るであろう、自己完結的なAIシステムを指しており、GPT 3.5やGPT 4などの大きな言語モデルを駆使して、高度な思考や反復的な作業を実行できる能力を持つものを指します。
エージェントのワークフローとはどのようなものでしょうか?
-エージェントのワークフローとは、AIが特定のタスクを遂行するために、複数のエージェントが異なるロールやツールを使って共同で反復的に作業を進めるプロセスを指します。
Dr. Andrew Ngが示すエージェントの強みは何ですか?
-Dr. Andrew Ngが示すエージェントの強みは、複数のエージェントが異なる役割を分担し、反復的に作業を進めることにより、最終的な結果をより良いものにする能力にあります。
エージェントが使用するツールの利点は何ですか?
-ツールの利点は、AIが特定のタスクを遂行するために必要な機能を持つコードを利用できることで、結果の予測可能性が高まり、AIの能力を拡張することができる点にあります。
Dr. Andrew Ngが語る「自己反映」とはどのようなプロセスですか?
-「自己反映」とは、AIが生成した出力を自己が評価し、改善点を探してより良い結果を生成するプロセスを指します。
マルチエージェントコラボレーションの利点は何ですか?
-マルチエージェントコラボレーションの利点は、異なるエージェントが異なる視点や専門知識を持ち、協力して問題を解決することで、より高品質な結果を出すことができる点にあります。
Dr. Andrew Ngが示すエージェントのワークフローはどのようにして実際のアプリケーションに適用されるでしょうか?
-Dr. Andrew Ngが示すエージェントのワークフローは、開発者や研究者がAIエージェントを用いてタスクを遂行する際に、より効率的で高品質な結果を得るために、計画、反復、ツール使用などのステップを組み合わせて適用されます。
Dr. Andrew Ngが語るエージェントの未来についてどう思われていますか?
-Dr. Andrew Ngはエージェントの未来について非常に前向きであり、人工知能の進化において重要な役割を果たすことを信じています。彼はエージェントのワークフローがタスクのセットを大幅に拡大し、AIの能力をさらに高めると予想しています。
Outlines
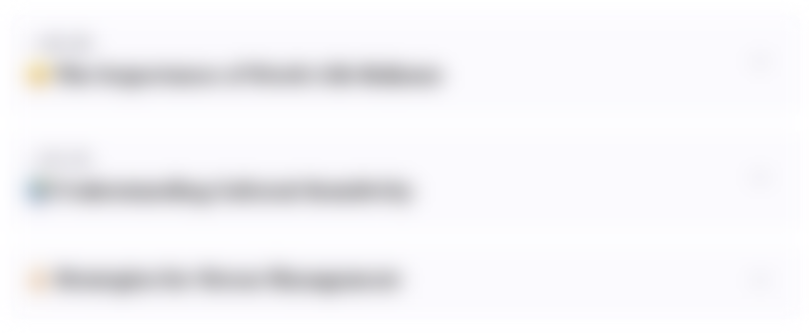
このセクションは有料ユーザー限定です。 アクセスするには、アップグレードをお願いします。
今すぐアップグレードMindmap
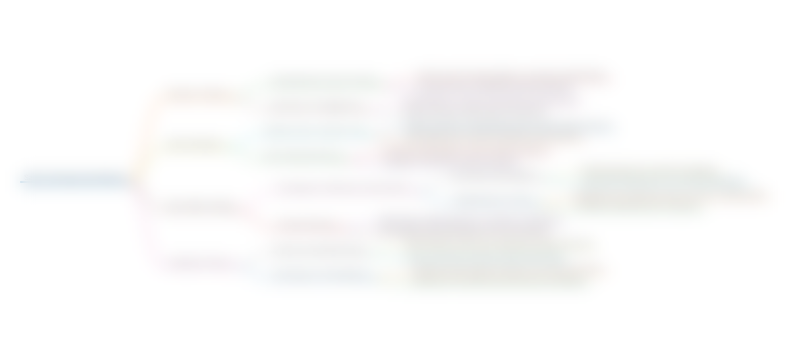
このセクションは有料ユーザー限定です。 アクセスするには、アップグレードをお願いします。
今すぐアップグレードKeywords
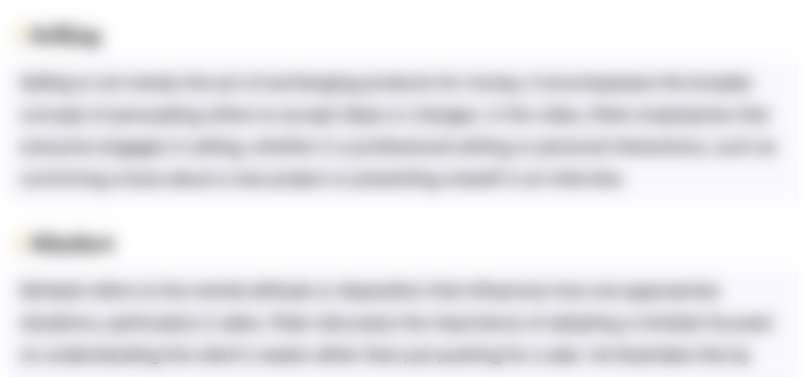
このセクションは有料ユーザー限定です。 アクセスするには、アップグレードをお願いします。
今すぐアップグレードHighlights
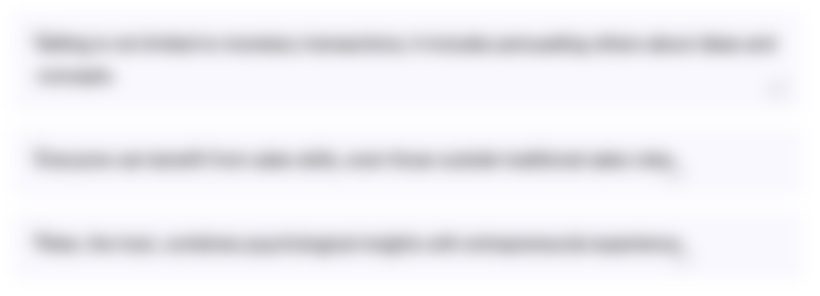
このセクションは有料ユーザー限定です。 アクセスするには、アップグレードをお願いします。
今すぐアップグレードTranscripts
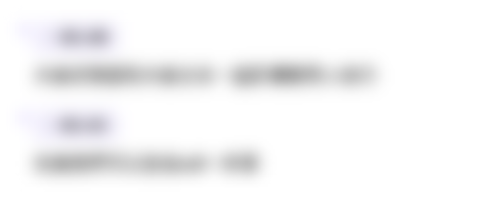
このセクションは有料ユーザー限定です。 アクセスするには、アップグレードをお願いします。
今すぐアップグレード関連動画をさらに表示
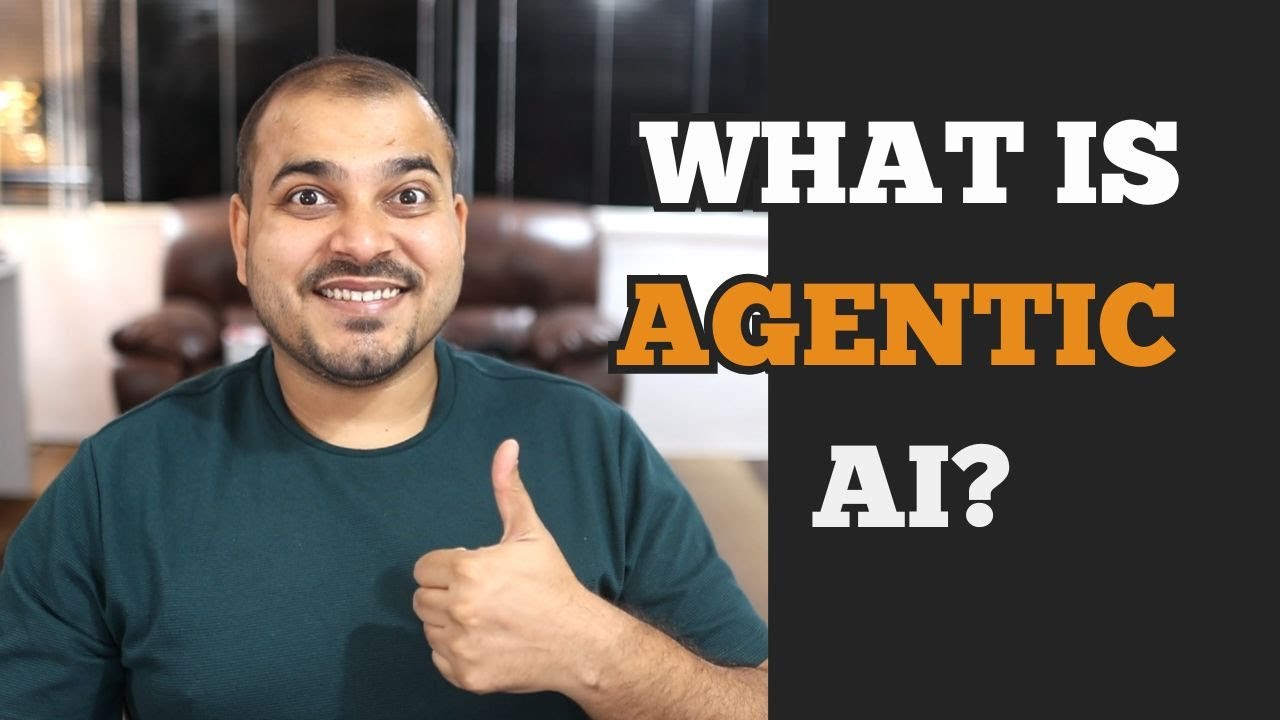
What is Agentic AI? Important For GEN AI In 2025
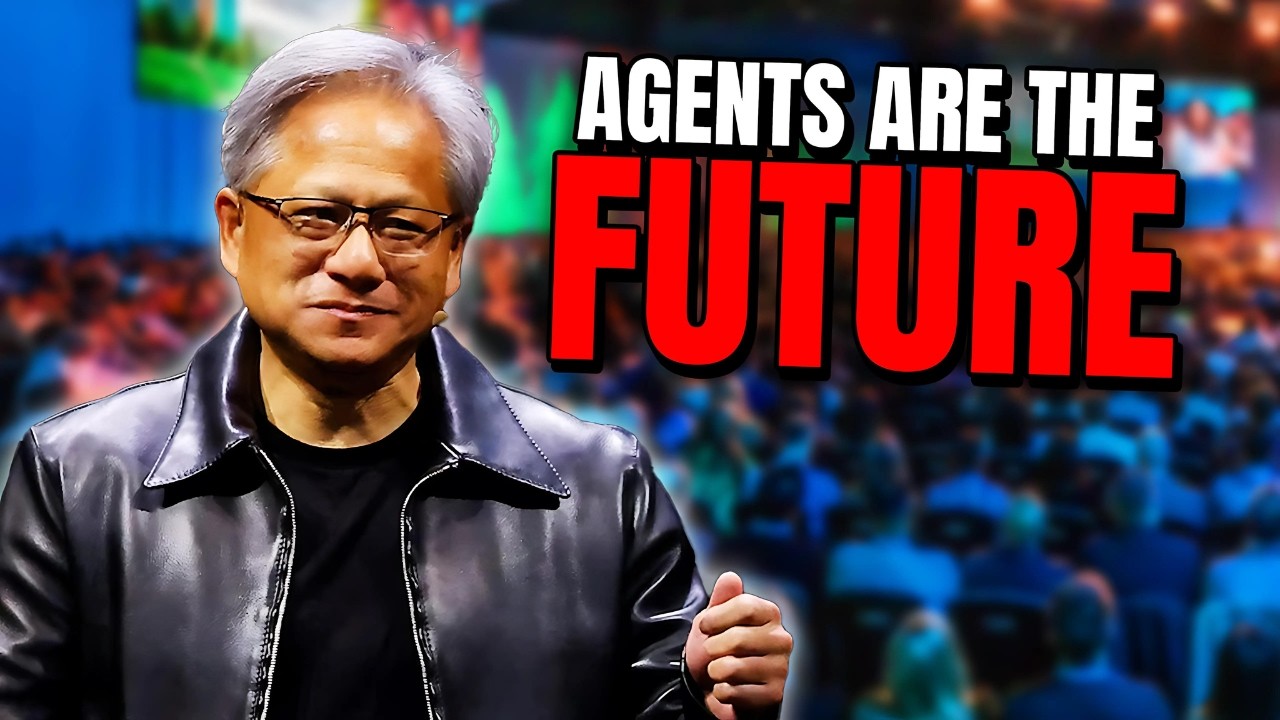
NVIDIA CEO on Agents Being the Future of AI
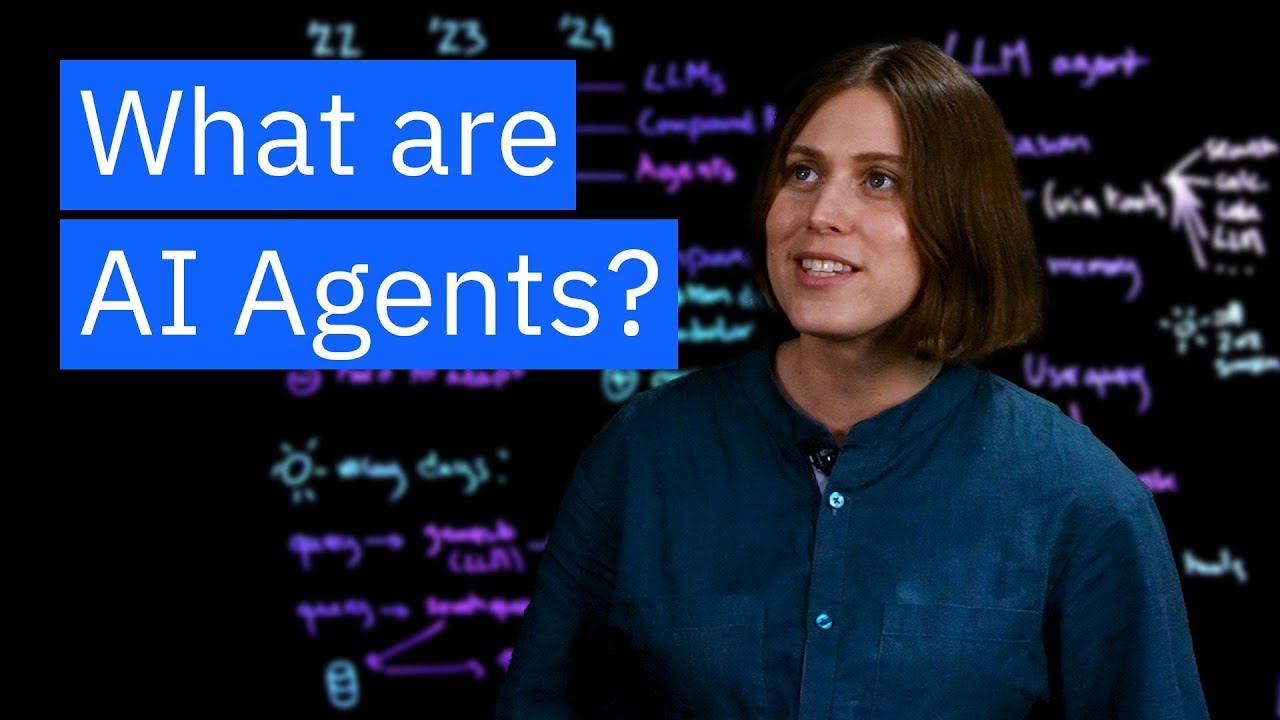
What are AI Agents?
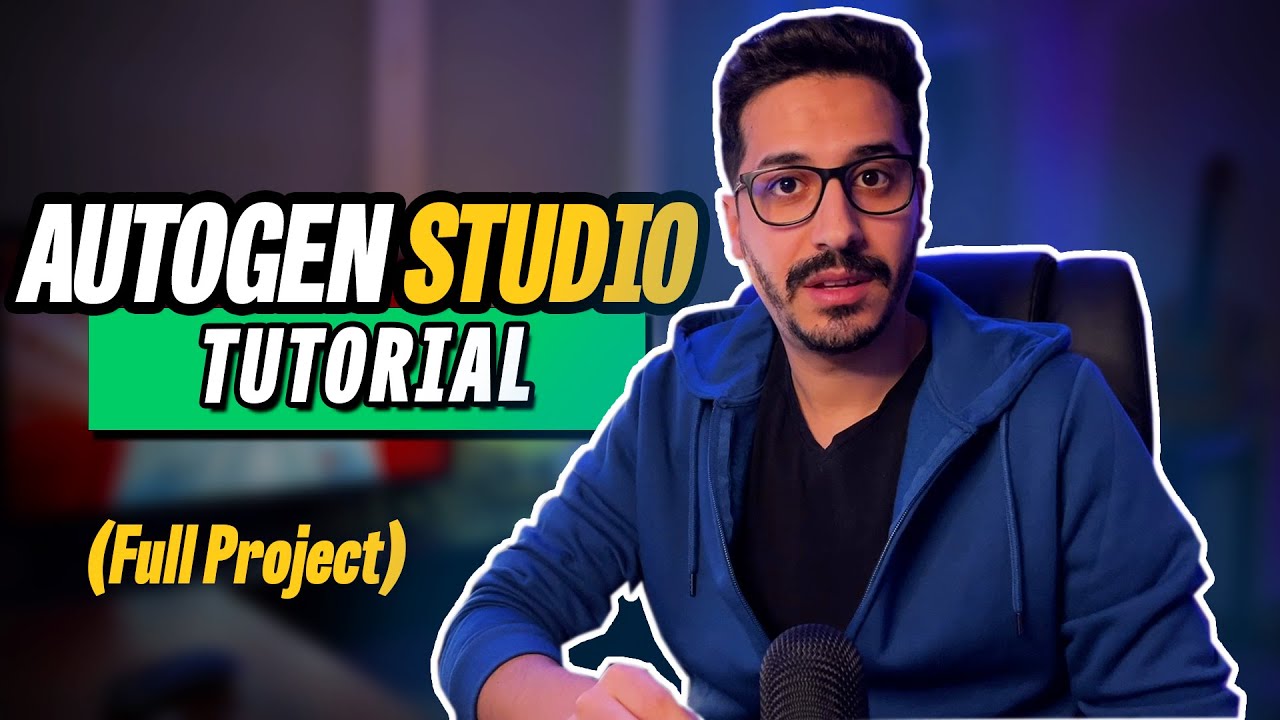
AUTOGEN STUDIO : The Complete GUIDE (Build AI AGENTS in minutes)
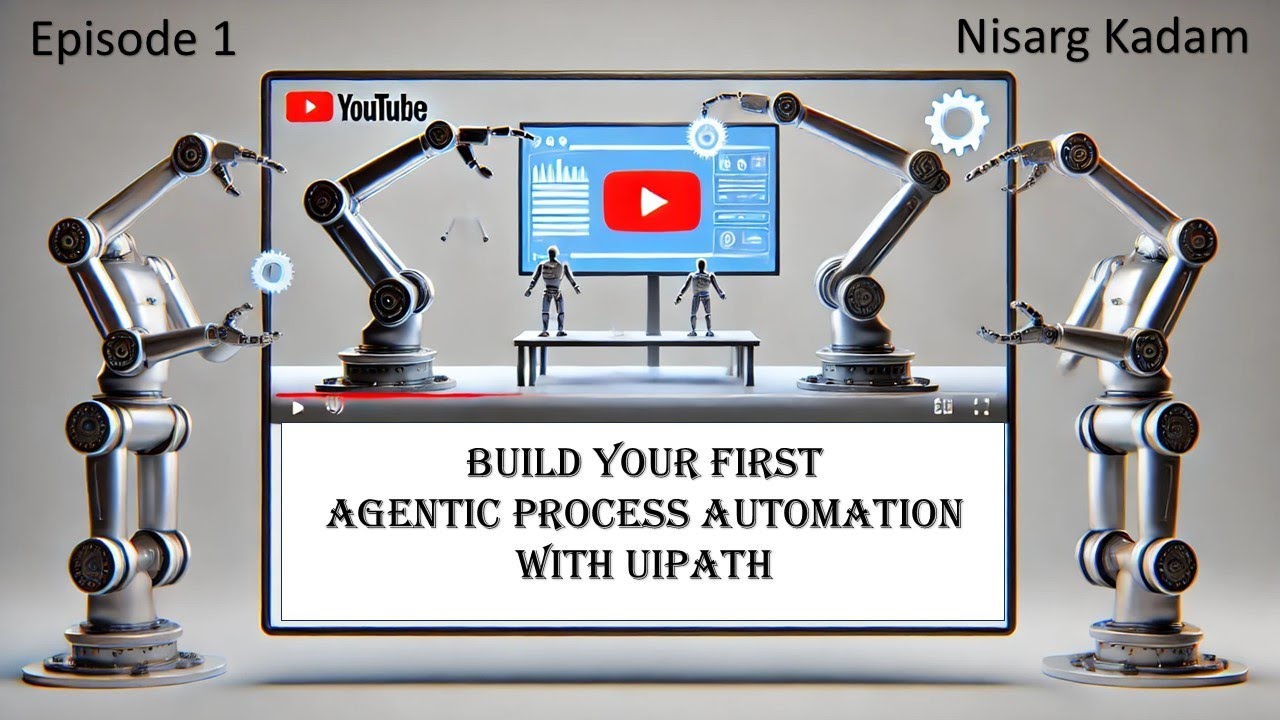
Build your first Agentic Process Automation with UiPath: Episode 1 - Autopilot for Everyone
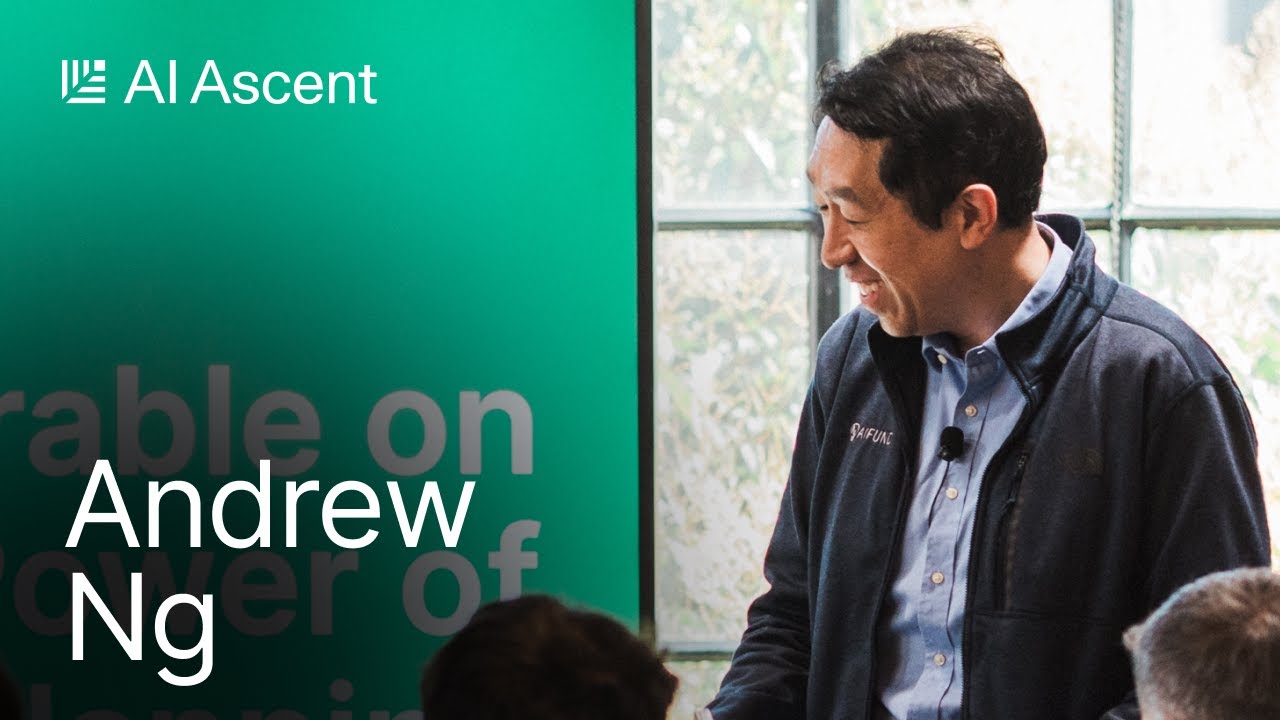
What's next for AI agentic workflows ft. Andrew Ng of AI Fund
5.0 / 5 (0 votes)