Why Don't AI Agents Work?
Summary
TLDRIn this video, DJ from Truth Theta discusses the growing excitement around AI agents and the scientific hurdle that currently limits their effectiveness. He highlights the need for AI agents to learn causal models to adapt to shifting environments, citing a key DeepMind paper that proves this necessity. While AI agents require this capability for robust functionality, current technology is not capable of scaling causal modeling at the level required. DJ suggests that breakthroughs in causal modeling and reasoning models may be the key to unlocking agentic AI in the future, emphasizing the need for focused innovation.
Takeaways
- 😀 AI agents are receiving increasing attention from major tech companies like Google and Microsoft, with a notable rise in AI agent development as tracked by the AI agent index.
- 😀 Despite the excitement, AI agents face a fundamental scientific challenge that prevents them from working effectively with current technology: the need for causal modeling at scale.
- 😀 A clear definition of AI agents includes four key traits: autonomy (acting without human intervention), social ability (interacting with other agents), reactivity (perceiving and responding to the environment), and proactivity (pursuing goals independently).
- 😀 True AI agents require robustness in adapting to distributional shifts—changes in environments beyond their training data, like the difference between sunny roads and icy roads in driving tasks.
- 😀 DeepMind’s paper argues that any agent capable of adapting to large environmental changes must have learned a causal model of the world, going beyond simple associations and understanding cause and effect relationships.
- 😀 Causal modeling, essential for developing robust AI agents, is currently not feasible at scale. The existing causal models are narrow and slow, requiring assumptions and cannot be fully automated.
- 😀 Modern causal modeling relies on techniques like causal inference, which is slow and manually intensive. It’s often constrained by the inability to know exact cause-and-effect relationships from the data.
- 😀 Causal inference, even in commercial settings, is highly focused and requires expert analysis, as seen in the example of user engagement management with AMP where AI-powered experimentation guides decision-making.
- 😀 AI agents must incorporate causal reasoning to achieve agentic capabilities, and current AI models lack the necessary depth to do so effectively due to the limited scope of existing causal models.
- 😀 The breakthrough required to achieve agentic AI involves solving the issues of causal modeling and improving experimentation techniques. There may be progress through reasoning models that could be adapted to causal domains.
Q & A
What is the main focus of this video?
-The video focuses on the scientific challenge that makes AI agents, as imagined in the future, not possible with current technology. Specifically, it discusses the necessity of causal modeling for AI agents to function effectively, which remains an unsolved problem.
What are AI agents and why is there a lot of interest in them?
-AI agents are systems that operate autonomously, interact socially, perceive their environment, and pursue goals proactively. The interest in AI agents arises because they can automate mundane tasks and cooperate to solve complex real-world problems, unlike reactive AI systems like ChatGPT.
What are the four key factors that define an AI agent?
-The four key factors are: Autonomy (acts without human intervention), Social Ability (interacts with other agents), Reactivity (perceives its environment and responds), and Proactivity (forms and pursues its own goals).
Why is causal modeling crucial for AI agents?
-Causal modeling is essential for AI agents because it helps them understand cause-and-effect relationships in their environment. This is needed for agents to adapt and perform well in various situations and environments, which is a fundamental requirement for agentic systems.
What does the DeepMind paper prove regarding AI agents?
-The DeepMind paper proves that any AI agent capable of adapting to a broad range of distributional shifts must have learned a causal model of its environment. This means that agents need to understand cause and effect in their environment to perform effectively in diverse scenarios.
What is the difference between causal models and associative models?
-Causal models capture actual cause-and-effect relationships, while associative models only identify correlations between variables. Causal models are more complex and provide a deeper understanding of how actions influence outcomes, whereas associative models only predict outcomes based on observed data.
What is a Bayesian network, and how is it used in causal modeling?
-A Bayesian network is a probabilistic graphical model that represents variables and their relationships. In causal modeling, a causal Bayesian network is used to represent cause-and-effect relationships, helping to understand the dynamics of an environment or system.
Why don't current AI agents work as expected?
-Current AI agents don't work as expected because they lack the capability to model causal relationships at the necessary level. They rely on associative data rather than causal understanding, limiting their ability to adapt effectively to new environments and tasks.
How does causal inference work in practice, and why is it slow?
-Causal inference works by analyzing experimental or observational data to predict the effects of actions. It's slow because it requires making assumptions about data and testing those assumptions carefully, which is time-consuming and cannot be easily automated.
What is the potential future breakthrough that could speed up the development of AI agents?
-A potential future breakthrough could involve reasoning models that have been successful in mathematical tasks. These reasoning models could potentially be applied to causal modeling, bridging the gap between theoretical reasoning and real-world causal understanding, although this would require substantial advancements in the field.
Outlines
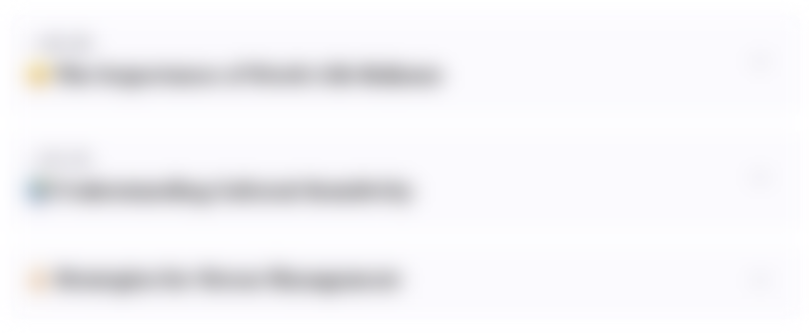
This section is available to paid users only. Please upgrade to access this part.
Upgrade NowMindmap
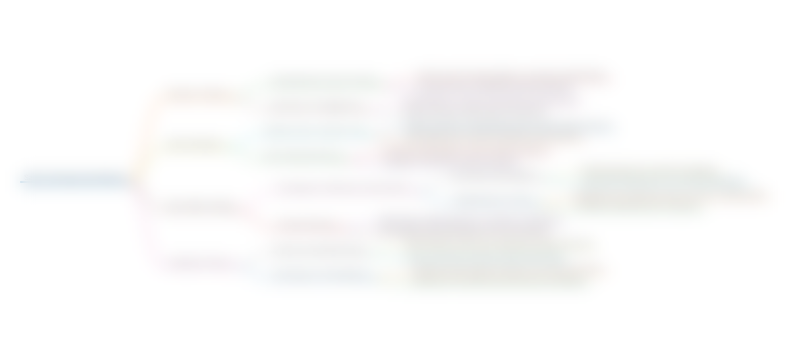
This section is available to paid users only. Please upgrade to access this part.
Upgrade NowKeywords
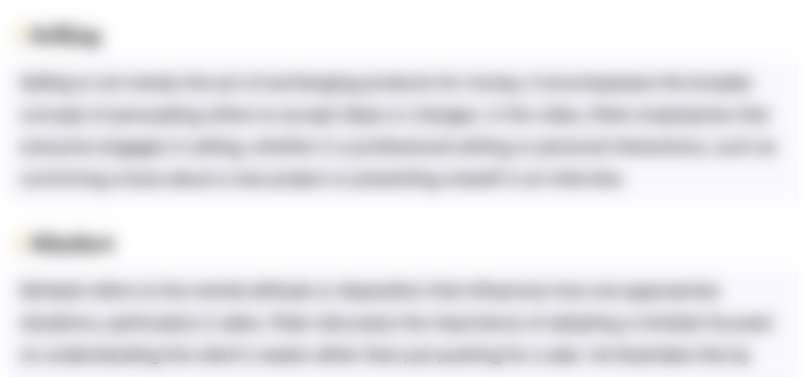
This section is available to paid users only. Please upgrade to access this part.
Upgrade NowHighlights
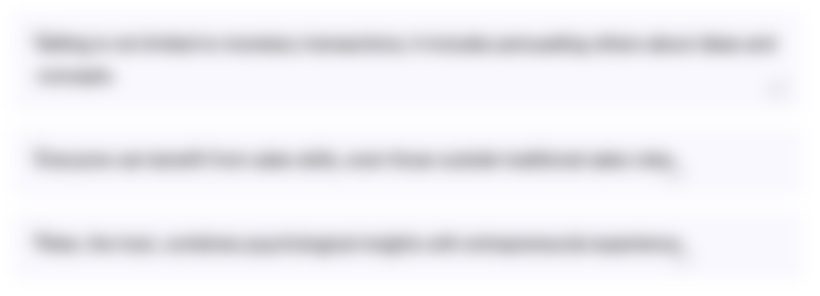
This section is available to paid users only. Please upgrade to access this part.
Upgrade NowTranscripts
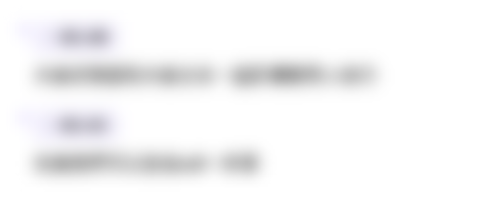
This section is available to paid users only. Please upgrade to access this part.
Upgrade NowBrowse More Related Video

Respons Pengecer Gas dan Warga soal Aturan Penjualan Gas LPG 3 Kg: Kasihan Rakyat Kecil
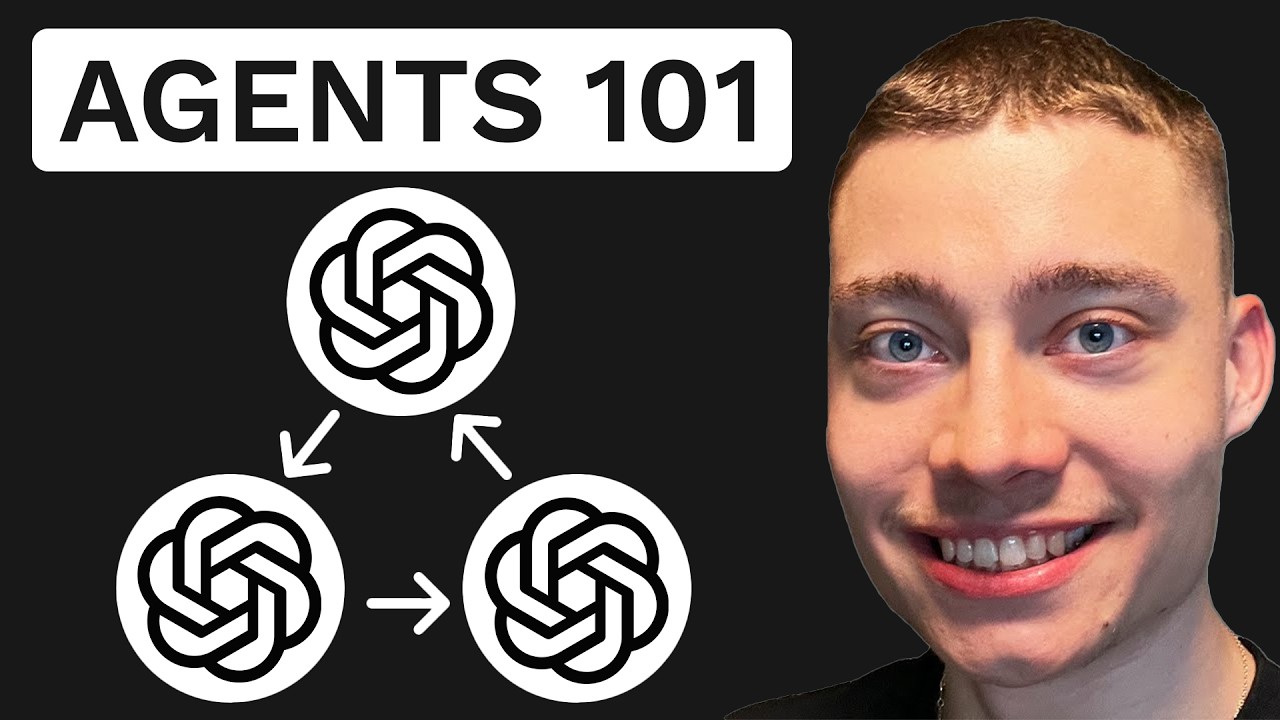
OpenAI’s 7 Hour AI Agents Course in 15 Minutes

Giải Thích AI Agent Là Gì Như Thể Bạn Mới 5 Tuổi (Cơ Hội Mới 2025)
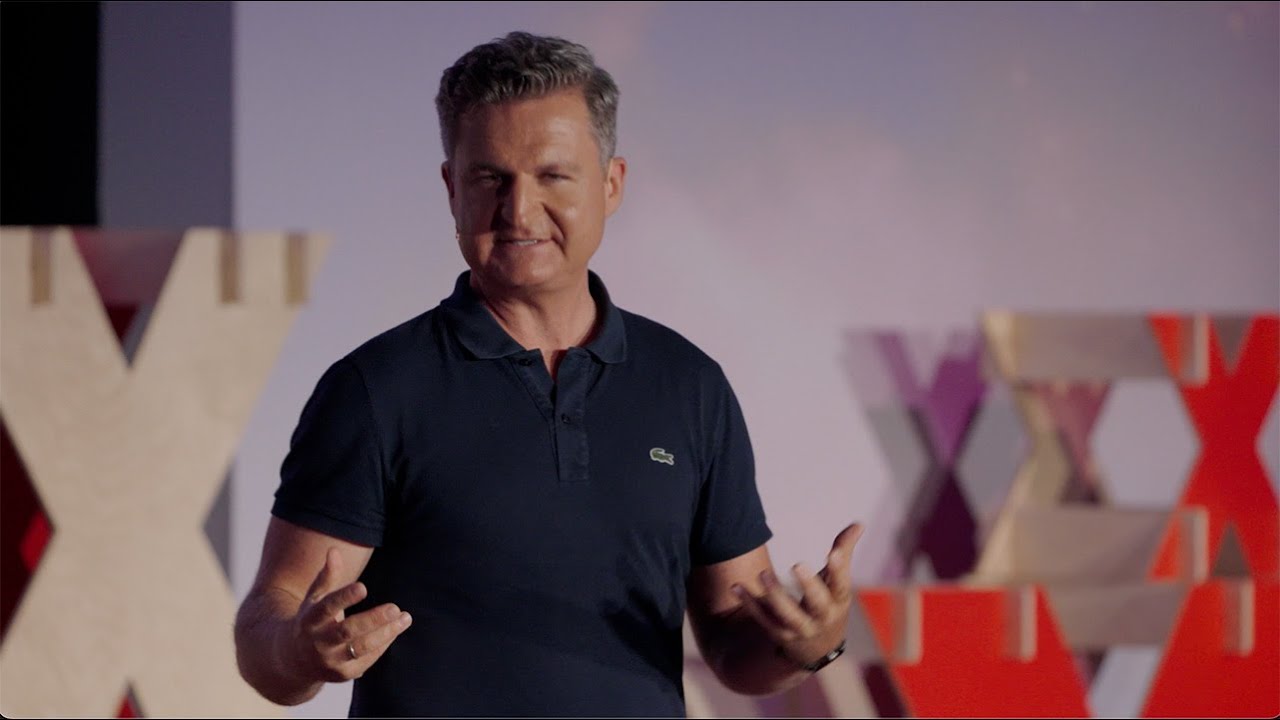
AI agents: The scientist's new superpower | Stefan Harrer | TEDxSydney Salon
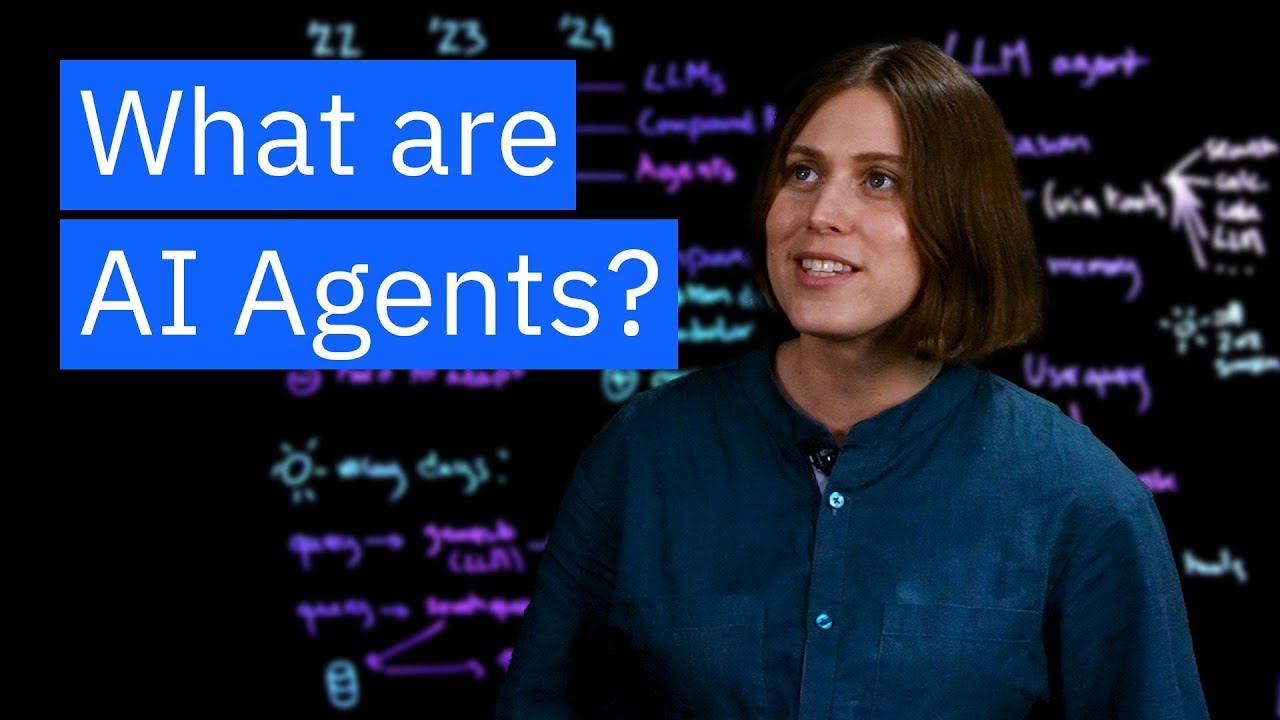
What are AI Agents?
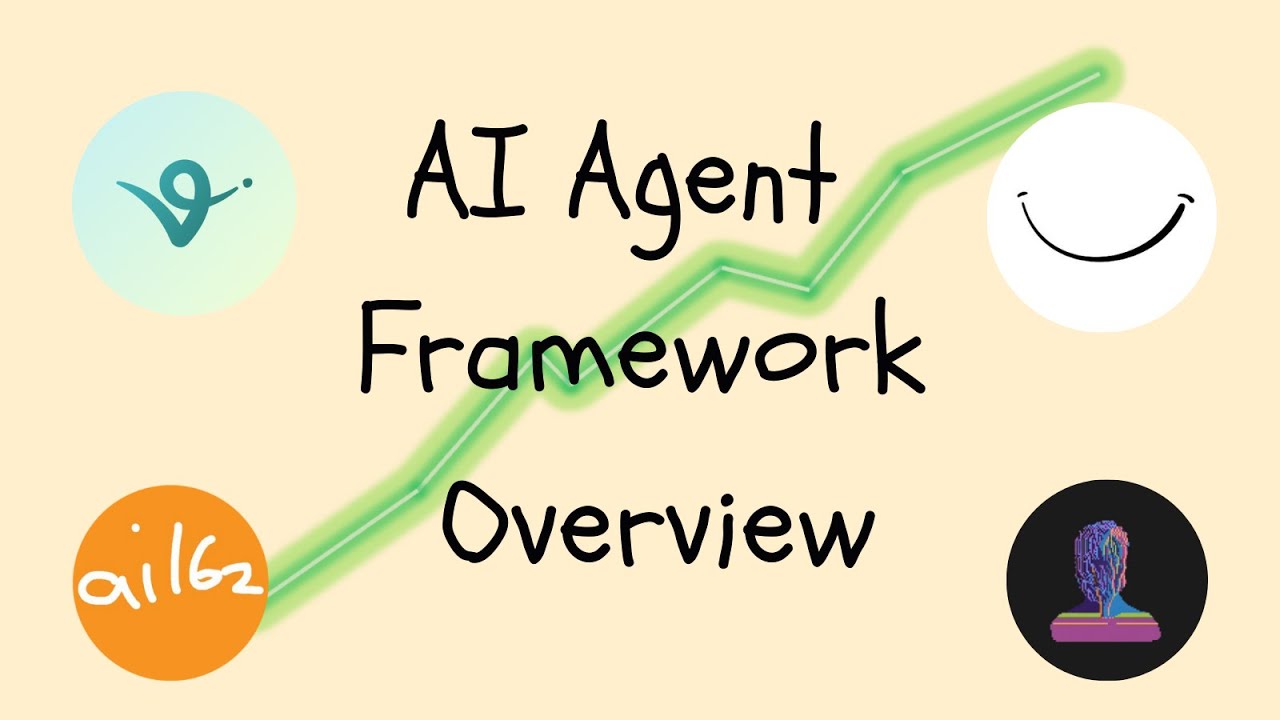
Everything You Need To Know About Crypto AI Agent Frameworks
5.0 / 5 (0 votes)