Optimization of LLM Systems with DSPy and LangChain/LangSmith
Summary
TLDRこのトークは、Harrison ChaseとOmarが共同で行った、DSP(宣言的自己改善言語プログラム)フレームワークの紹介です。DSPは、複雑な問題を解決するための言語モデルを使わずに、問題を分解し、標準的なプログラミングツールを使用して構築するフレームワークです。Omarは、DSPがどのように問題を解決し、どのように使用されるかについて説明し、また、DSPが持つ最適化の問題やフローエンジニアリングの重要性についても触れています。
Takeaways
- 🌟 DSP (Declarative Self-Improving Language Programs) は、言語モデルをより効果的に使用するためのフレームワークで、プログラムの構造を考慮する方法を提供します。
- 🔍 DSP は、問題を分解し、標準的なプログラミングツールを使用可能にすることで、困難な問題を解決する方法を考えるフレームワークです。
- 📈 DSP は、標準的なコードではなく、モデルがどのようにタスクを実行するかを宣言する「自然言語サイン」を使用して、プログラムを最適化します。
- 🔧 DSP は、問題の構造を理解し、ループ、再帰、アサーションなどの標準的なプログラミングツールを使用可能にすることで、AIモデルに特に適した曖昧なステップを解決します。
- 🚀 DSP は、言語プログラムの開発と最適化に焦点を当てており、GPT-4などの言語モデルを使用してプログラムを実行する際のプロンプトを考案する方法を改善しています。
- 🧠 DSP は、言語モデルにタスクを教える「デモンストレーション」の段階を自動化し、最適化のためのオプティマイザを使用して、プログラムを改善します。
- 🛠️ DSP は、プログラミングの抽象化を提供し、問題を解決するためのプログラムを宣言する「サブモジュール」を定義します。
- 🔄 DSP は、プログラムをPythonクラスのように宣言し、フォワード関数を使用して問題を解決する方法を提供します。
- 📊 DSP は、プログラムを「コンパイル」し、より低いレベルの言語(多くの場合、英語のような自然言語)に変換することで、より効果的なシステムを作成することを目的としています。
- 🤖 DSP は、言語モデルの「インストラクション」を生成し、各サブモジュールが使用する方法を最適化するための「モジュール」を使用します。
- 🌐 DSP は、言語モデルに関連する「signatures」を定義し、プログラムの「signatures」を最適化するための「optimizers」を提供します。
Q & A
DSPフレームワークの主な目的は何ですか?
-DSPフレームワークの主な目的是言語プログラムを構築するための考え方を提供し、問題を分解して標準的なツールやプログラミング概念を使用できるようにし、AIモデル尤其是に言語モデルに最適化された曖昧なステップを表現する方法を考えます。
DSPの利点は何ですか?
-DSPの利点は、プログラミング概念を標準化し、曖昧なステップを解決するための標準的なツールと抽象化を提供することです。これにより、言語モデルに特定の問題を解決させるために最適化されたプロンプトを生成することができます。
DSPフレームワークはどのように問題を解決するのに役立ちますか?
-DSPフレームワークは、問題を部品に分解し、問題構造を理解することを助けます。標準的なプログラミングツールを使用して、ループ、再帰、アサーションなどの標準的なプログラミングツールを使用できます。
DSPフレームワークはどのようにしてプログラムを最適化しますか?
-DSPフレームワークは、宣言型のプログラムを用いて、プログラムの各ステップを最適化します。これには、各ステップに対応するプロンプトを生成し、最適な方法でモデルをトレーニングまたは微調整することが必要です。
DSPフレームワークはどのような種類の問題に適用できますか?
-DSPフレームワークは、検索、予測、カテゴリ化、要約などの様々なタスクに適用できます。言語モデルを効果的に使用するための問題を解決するため、DSPフレームワークを使用できます。
DSPフレームワークはどのようにして新しい言語モデルに適応するのですか?
-DSPフレームワークは、最適化されたプログラムを生成することで、新しい言語モデルに適応します。これには、新しいモデルに対してプログラムを再び最適化することが含まれます。これにより、新しいモデルでも効果的なプロンプトが生成されます。
DSPフレームワークはどの程度の柔軟性を持っていますか?
-DSPフレームワークは、問題を分解し、各ステップを個別に最適化できるため、非常に柔軟性があります。これにより、さまざまなタスクに適応し、新しい言語モデルにも簡単に適応できます。
DSPフレームワークを使用する際に考慮すべき事項は何ですか?
-DSPフレームワークを使用する際には、問題の構造を正確に理解し、各ステップを適切に宣言することが重要です。また、最適化プロセスに必要なデータセットやメトリックスを準備することも重要です。
DSPフレームワークはどのようにしてプログラムの品質を向上させますか?
-DSPフレームワークは、プログラムの各ステップを個別に最適化することで、全体的なプログラムの品質を向上させます。これにより、言語モデルが効果的にタスクを実行し、正確な結果を生成することができます。
DSPフレームワークを使用する際に直面する可能性がある課題は何ですか?
-DSPフレームワークを使用する際に直面する可能性がある課題は、言語モデルのブラックボックス性です。モデルがどのように動作するかを完全に理解できないことがあるため、プログラムの正確性を保証することが難しい場合があります。
Outlines
📌 はじめに
ビデオスクリプトの最初の段落では、Harrison Chase と Omar が自己紹介を行い、DSP(Declarative Self-Improving Language Programs)というフレームワークについて話し始めています。Harrison は Lang chain の CEO であり、DSP は LM アプリケーションを構築するための開発者ツールキットを提供することを述べています。Omar はスタンフォードの博士課程の学生であり、K bear という名前の NLP プロジェクトに携わっています。DSP フレームワークは、言語プログラムを開発し最適化するための仕組みで、標準的なプログラミングツールや抽象化を利用して、AI モデルに解決を委ねる代わりに問題を分解し、構造化することを目的としています。
🔍 DSP フレームワークの目的と機能
DSP フレームワークは、問題を分解し、問題構造を理解するための標準的なツールとプログラミングツールを使用できるようにすることで、言語プログラムの概念を考慮するフレームワークです。DSP は、標準的なコードでは表現できない不確定なステップを扱うために AI モデル、特に言語モデルによって解決されるステップを考慮に入れたプログラムを構築するための枠組みを提供します。DSP は、これらの不確定なステップをどのように表現するか、そしてこれらのステップがどのように組み合わさってより大きなプログラムに変換されるかを定義する高水準な宣言から、最適化されたプログラムのバージョンに至るまでのプロセスを考慮しています。
🛠️ DSP フレームワークの応用と最適化
DSP フレームワークは、問題に対するプログラムの構築方法、言語モデルにどのようにプロンプトを提示するか、そして異なるタスクに適切なプロンプトを設計する方法を考慮するだけでなく、gp4 などの言語モデルを使用する際のプロンプトの最適化にも注目しています。DSP は、プログラムの各段階を最適化するための最適なプロンプトを生成する方法を学ぶことで、言語モデルを使用する際のパフォーマンスを向上させることができます。また、DSP は、モデルの変更や微調整など、言語モデルの変更に関連する問題を解決するために、プログラムのロジックを言語モデルに依存しない表現で定義することを目的としています。
🔧 DSP フレームワークのフローと最適化
DSP フレームワークは、フローエンジニアリングの概念を導入し、システムの各段階を宣言的に定義する方法を提供しています。これにより、問題を解決するための一連のステップを明確にし、プログラムの構造を理解することができます。DSP は、プログラムを Python クラスのように書くことで、内部的な制御フローを宣言します。これにより、プログラムをコンパイルして、モデルに提示するための自然言語プロンプトを生成することができます。DSP の主な問題は、これらのプログラムを最適化するためのアルゴリズムを提供することです。これには、プログラムの各段階に対して最適なプロンプトを生成するためのディスクリプティブ検索を含みます。
🤖 DSP フレームワークと言語モデルの統合
DSP フレームワークは、言語モデルを統合し、プログラムのロジックを最適化するためのモジュールと呼ばれる中間層を提供します。これにより、言語モデルにどのように各ステップを実行するかを教えるかを定義することができます。DSP は、Chain of Thought などの戦略をモジュールとして提供し、これにより、言語モデルに対して特定のパターンを学習させることができます。DSP は、これらのモジュールを通じて、プログラムの各段階に対する最適なプロンプトを自動的に学習することができます。
📈 最適化と評価
DSP フレームワークでは、最適化と評価の概念が重要です。最適化は、プログラムの各段階に対して最適なプロンプトを生成することで、言語モデルのパフォーマンスを向上させることができます。評価は、プログラムのパフォーマンスを測定し、最適化プロセスを指导するために使用されます。DSP は、プログラムを評価するためのメトリックスを提供し、これにより、プログラムの品質を測定し、最適化の方向性を決定することができます。
🔄 データセットとトレースの使用
DSP フレームワークでは、データセットとトレースの使用が重要です。データセットは、プログラムをトレーニングと評価するために使用されます。トレースは、プログラムの実行時に生成されるデータであり、プログラムの各段階を理解し、最適化に役立ちます。DSP は、トレースの収集と使用を通じて、プログラムのパフォーマンスを向上させることができます。
🌐 Lang chain と DSP の統合
Lang chain と DSP の統合は、より高度な言語モデルアプリケーションを構築するための重要なステップです。Lang chain は、高水準の抽象化と統一された抽象化を提供し、DSP は、プログラムのロジックを最適化するためのフレームワークを提供します。Lang chain と DSP の統合により、開発者は、より効率的にプログラムを構築し、最適化することができます。
🚀 将来の展望
DSP フレームワークの将来の展望では、プログラムのフロー自体を最適化することを検討しています。これには、プログラムの構造を改善し、言語モデルのパフォーマンスを向上させることが含まれます。DSP は、プログラムの最適化とフローエンジニアリングの両方を考慮し、より高度な言語モデルアプリケーションを構築するための道を開いています。
Mindmap
Keywords
💡DSP
💡Language Model
💡Flow Engineering
💡Optimization
💡Control Flow
💡Abstractions
💡Chain of Thought
💡Prompts
💡Tracing
💡Metrics
Highlights
Harrison Chase, CEO and co-founder of Lang chain, introduces a developer toolkit for building LM applications.
Omar, a PhD candidate at Stanford, discusses his work on K bear, a framework for developing and optimizing language programs.
DSP (Declarative Self-Improving Language Programs) is a framework that allows for the expression of fuzzy steps in programming that are best tackled by an AI model.
DSP aims to tackle the problem of building programs for different types of tasks and generating effective prompts for GPT.
DSP is designed to treat the use of language models for specific tasks as an extended machine learning problem.
Flow engineering is a concept that involves creating a system that operates like a flow with different stages.
DSP was originally a backronym for Demonstrate, Search, Predict, focusing on retrieval-focused tasks.
DSP evolves from DSP by automating the demonstration stage, allowing users to write Python classes and use optimizers for effective program flows.
Lang chain has been utilized in building language programs since 2020, with advancements in prompting and fine-tuning.
The challenge with language model pipelines is that they are non-transferable and tied to specific systems.
DSP aims to make working with language programs more systematic, focusing on programming rather than prompting language models.
The process of optimizing a language program involves separating the program logic from teaching the language model each step.
DSP introduces the concept of natural language signatures and modules for controlling the flow of signatures.
Optimization in DSP involves using language models to convert high-level task descriptions into effective instructions or examples.
Lang chain and DSP can be combined, with Lang chain providing higher-level pieces and lower-level integration, while DSP focuses on optimization and control flow.
Tracing is crucial for observability, example collection, and learning from data sets in both Lang chain and DSP.
The potential integration of Lang chain and DSP could lead to streamlined data set export, improved control flow expressions, and optimization of Lang graph syntax.
Transcripts
before that we can do brief
introductions and then jump right into
it so so my name is Harrison chase a CEO
co-founder of Lang chain um a a
developer toolkit making it easy to
build LM
applications um thank you Harrison and
uh thanks everyone for joining me looks
like we have a a lot of folks um I'm
Omar um I'm a PhD candidate at Stanford
um I work in NLP Broadley you might know
about my work on K bear uh in retrieval
um and um you know excited to talk about
dspi today which is a framework for
developing and optimizing uh language
programs and I'll tell you all about
language programs
today all right so DSP that's how it's
pronounced that puts all the questions
to bed yes like like numpy or pytorch
DSP all right so yeah I mean let's jump
right into it what what is DSP DSP um
why did you build it how how are people
using it yeah so this is a framework for
really thinking about those like this
notion of language programs you're
writing a piece of code and you know
you're trying to solve a hard problem
and the way you're going to solve that
hard problem is not by working really
hard at you know teaching that model to
um understand your task it's going to be
by you know breaking this problem down
into pieces and thinking about the
problem structure that you have you
could employ you know um all standard
sorts of tools and we have't programming
so you could have um you know Loops or
uh recursion or you know um assertions
or all other sorts of um standard
programming tools um and abstractions
but the key thing is that in this
program there are these steps that you
don't know how to express um in standard
code so there are these kind of fuzzy
steps that are generally best tackled by
an AI model by a language model in
particular um and so DSP is basically a
framework for thinking about that layer
how do we express this type of thing and
then how do we actually go from you know
a high level Declaration of what these
you know fuzzy steps are going to do and
how how they sort of compose together
into a bigger program um into an
optimized version of the program that
runs effectively given any particular
language model in any particular program
um so one one of the main problems we're
trying to tackle is well thinking about
you know how do you build a program this
kind like what should you do for
different types of problems um and then
the one that is sort of I think DSi is
better known for is now that you've
written this program and you want to run
it for
gp4 what should the prompts look like um
to gp4 so that it does all of these
stages in your program um effectively so
you have a you're solving a you know
you're doing rag for example and let's
say you don't want um your rag component
to rely entirely on this retrieval uh
system because in many cases retrievers
are kind of optimized to be fast and and
and cheap and scalable but you really
want it to be very accurate so maybe
your program is given the user's
question um I want the language model to
generate a bunch of search queries um
that will you know be optimized one way
or another to find as many of the
relevant facts as possible maybe this is
like patent search or something and so
you really really care about Recall now
that you've uh generated those queries
you want to basically
um find the documents that
um um that match those sties and then
you want the last stage of your system
maybe to summarize the information there
with
citations in principle you could just
ask a language model to do these steps
but as we're all aware these models are
very very sensitive and they're
sensitive in different ways so over time
these models get updated or you want to
switch from gp4 to to clad or you want
to sort of go to a smaller mod mod to
save costs over time or because you're
struggling with the learn with the with
the rate limits or some other challenges
um in the general problem that you face
is
that even if you manage to get prompts
that more or
less declare the behavior that you're
interested in and in and enforce it
they're very very um they're
non-transferable right they're just tied
to the particular system that you built
with a particular language model that
you built so the SP is basically adding
this layer in between where you can
express those stages in terms of what
you want to do rather than how you want
the particular language model to do it
um and um it basically gives you the
rest of the pieces of the puzzle to
treat this almost like a machine
learning extended machine learning
problem where you're trying to learn to
fill in the blanks of how to use a
language model for a particular task and
so so one of the terms that I've heard a
bunch on Twitter recently is is flow
engineering and this idea of like yeah
creating or your system being kind of
like a flow and there's different stages
and is that essentially what you're
talking about with the first part of it
like this way of declaring kind of like
that system right so dspi stands for
declarative self-improving language
programs pythonic which is a which is
which is a complex kind of backronym
because originally DSP was demonstrate
search predict and uh so that's a 2022
paper um we wrote so demonstrate search
predict is a particular way um to think
about designing these these flows if you
will um so basically search is this
notion of to solve a task you basically
want to be able to break down how your
language
system takes the input maybe a question
or whatever just trying to solve and
gathers information and you know if you
read if you read the DSP paper not the
DSP paper it basically includes a number
of design patterns what should how
should you build a search stage with
language models and you know there's a
lot of literature on stuff of this kind
now like hide and other stuff um that
for example use language models to
generate documents and then search
search for them or U multihop retrieval
or other sorts of um types of that kind
of things so let me see if I
can hopefully you don't get a lot of
that um and predict is like well now
that you've gathered those stuff how do
you you know design the system
architecture so that you um
output grounded answers you know
potentially uh with citations and other
other uh this type of constraint so DSP
was very kind of like retrieval focused
right DP is more General and can be used
for kind of like anything things or
would you say that it's still fairly
retrieval focused no the dpy is not
retrieval focused um DSP but the bigger
difference the bigger difference was
that DSP required you to think about
this demonstrate stage so I said
demonstrate search predict and I didn't
describe what demonstrate was you had to
manually declare the or Define the logic
of how your language model will be
taught to do the task so you wouldn't
write you wouldn't be writing the
prompts but you would be writing the
code that that essentially searched over
prompts to to Define how the program
should work and in dsy that's kind of we
automated that for for for people and we
created a bunch of optimizers so that
you know the the the standard flows that
we saw were very effective um could just
work so in DSP you basically just write
to python class um maybe this is a good
place to um to share screen I don't have
slides specifically for today but I have
some kind of things I could probably
just
show yeah I think that helpful because
one of the things that struck me looking
at and I think you've said this is it
looks very similar to P torch um and so
how about so what think what I could do
is I could share screen slides are
slightly not for today but
um let's see if we can make this
work I have the repo pulled up as well
if you want me to share that oh there we
go so um I said I started by saying that
these language programs are advancing
many tasks today and you know this is
part of the in the context of a talk
where I discuss language programs I
built in like 2020 and 2021 which was
not with prompting it was with
fine-tuning and it was you know you
didn't couldn't really kind of it was
hard to figure out how to generate data
for them but now it's much easier
because you could prompt these language
models to build stuff like storm people
might be familiar with this cool work um
from y Stanford um and and and folks uh
with us um basically writes whole
Wikipedia articles and the interesting
thing about something like storm is not
really the language model itself the
language model is more of a device in
the background
the interesting thing is how these parts
are are are composed together to um to
solve this hard task and to ensure that
you know outputs which in this case are
entire Wikipedia articles are planned
properly and um are citing their sources
properly another one is AA um with Carl
and and folks um so this is basically a
system for classifying um long documents
but not classifying them into five or 10
classes it's classif classifying them
into up to I think 15,000 classes and
the challenge is in general you don't
have labels for all these classes and so
you're basically bringing in a language
model and in this case a retrieval model
um in an interesting way to solve this
and this sort of thing is obviously very
very popular in Lang chain you know you
this is basically um all the sorts of
chains you guys U you guys have
popularized dramatically um but lots of
folks are building these you know text
tosql stuff um really cool one from
Google rar um that revises um you know
tax generation from Models um Alpha
codium right this is the um flow
engineering uh uh paper um so this is
stuff this stuff is is super popular and
then basically the problem that dsy is
solving is that these diagrams are
really cool like you look at these
diagrams and they're very modular
they're very attractive there slot like
about them but the problem is because
language models are very sensitive to
how to how you prompt them if you think
of them as like as as like basically um
CPUs it's like their instruction sets
are just very different and ad hoc um so
under the hood this stuff looks more
like as as we're all familiar looks like
like this looks more of um you know
here's a long and and very carefully
crafted prompt that teaches the model
how each of these stages should work and
we really hope that the output of each
stage looks good enough to the input of
the next stage because if they don't
we're kind of going to have a Sy that
doesn't work and so people are sort of
writing these and and I'm guilty of that
as well you know we're all kind of
writing these um you know tens of
kilobytes of raw text files and just
kind of trying to do our best with that
and and this basically leaves us very
dissatisfied in in DSP because we're
thinking these language model pipelines
that people are building are so modular
in principle and there's lots to love
about them and there's something really
attractive and moving beyond the world
where language models are you know are
are so important into a model where they
are basically devices you know you just
buy or rent the best one you can get and
then you're building the software on top
um and you know we have all these large
design spaces but in reality this is
extremely messy and hog and it's you
know that's that's really limiting what
you can do so in particular you take any
of the pipelines or some of the
pipelines we looked at and you want to
change your language model to a cheaper
one or you want to switch from prompting
to fine tuning um and you're sure of
stuck like do you have to rewrite the
prompts do you have to like redesign
this whole thing so that it could find
you in the model um that doesn't feel
like you know the right way to go about
this especially given how powerful these
language models are supposed to be um so
the goal of dspi is to sort of make um
working with these language programs
more like systematic machine learning um
and we have this sort of um theme of
programming and not prompting language
models um so basically you're not going
to be writing these Long messy uh
prompts which again we're all guilty of
writing and in in many cases basically
you know you write those like thousand
words or 2,000 words and maybe you're
not even satisfied with your results at
that at the end of this um instead
you're going to write a piece of code um
as you said Harrison that looks like a
pytor
program um so you're basically going to
declare a dsy module and in your dsy
module you're going to Define you're
going to sort of um Define one or more
subm modules and these sub modules um
allow you to express internal sort of
control flow um that declares how uh
signature is going to work so I'll say
more about these terms but basically
you're going to declare a bunch of
modules here and then you have a forward
function that's just using these modules
it's using generate quy and using
generate answer um uh you know in a loop
or whatever to solve an actual problem
and you notice here that we're not
writing any prompts anywhere or we're
not writing you know like how the models
should be used um again the slides are
not really meant for today so they're uh
the ordering might not be ideal but
we'll try to make it work um the idea
then is that we want to take this
program and essentially compile it down
and compiling in general in in the
general sense I'm using here means
taking a high level program and sort of
generating a lower level language and
something that is strikes people as
interesting or odd or whatever at first
when they see DSP is we're actually
compiling computer code in Python to the
lowlevel language which in many cases is
like English for example um for the
promise so we're actually treating the
natural language that we use to speak to
the models as a low level as a low as a
lower level abstraction and that's not a
mistake that's intentional because the
language these modelist speak is not
necessarily the English that you or I
understand it's it's really you know
it's a much more subtle thing where very
small differences to us you know or or
in some cases even very strange uh
changes to us um may impact model
quality dramatically and and maybe I can
share some examples of that that like
ended up getting really popular but idea
is that when you compile this this
program which is actually shorter than
the prompt we had in the last slide um
you can get better scores you can get a
whole lot of better scores with bigger
models but the same program can be find
can be um compiled into smaller open
models um or even tiny language models
through fine-tuning um and you're not
really changing your code or your
description of the problem in any way
you're just selecting different
optimizers um which are the um sort of
modules in thepi responsible for turning
this this thing as it being compiled
into a highly effective um into a highly
effective system and what makes this all
possible is two ideas um want to
separate the program logic so what you
just call like this information flow um
from how we're going to teach the
language model each of the
steps and we want to um optimize we want
to think about algorithms that can
optimize how we will prompt or fine-tune
the language models um and in general
the role the the the approach we'll take
here is some sort of discrete search
over modular language elements so we
don't want to be doing like you know
reinforcement learning or something
where we need a whole lot of data or um
other approaches we we actually want to
use the language models themselves to
think about hey how should we convert
this high level sort of description of
our task into uh into something that's
effective um and obviously language
models are not very good at this sort of
thing on their own so we want to give
them the U the support to um to make
this to make this
effective um so let's see what's a good
way to do this so let's yeah I think
this may be a decent
example um a decent example basically is
let's say you want to teach three
different language models to answer a
question after they do some Chain of
Thought how would you express that so
let's say you want to do this with gp4
I'd say one way to do this is you would
like think about how you would craft an
instruction and then you trust that GPT
for can answer questions and can do
Chain of Thought So you want to keep
this cheap and you don't want to like
make the prompt too long unnecessarily
but if you want to do this with like an
open model that's a bit smaller maybe
mistal or or llu um on the smaller side
of those models um then maybe you want
to start showing examples and this is
trickier than it sounds because your
examples should demonstrate the pattern
you're trying to uh to show so for
example if you want to do a Chain of
Thought well you need to think about how
do I write the right Chain of Thought to
teach this model how to do this for my
types of questions um and let's let's
say you write something that looks like
this and maybe you have several more
examples because more is usually more
effective um but in some cases you want
to solve the same task but with a much
smaller model and here you know with a
T5 model for example prompting is not
going to do much at all the model is not
you know um in context learning aware so
you're going to have to synthesize or
otherwise create maybe a thousand
examples of this pattern um so we have
questions the Chain of Thought and the
answers and you want to actually fine
tune the model on it but it's what's
dissatisfying here is that we're solving
the exact same problem and actually
we're expressing the exact same um
control flow um although this is just
one language model call um in three
vastly different ways and and the idea
is that like instructing models fop
prompting fine-tuning are just different
implementations of the idea that I have
a question and I want to generate some
reasoning and I want to generate an
answer and this is actually how you
would Express this in dsy you say I you
know this is like a function signature
if you will so this is my input and
these are my outputs and you could have
multiple inputs or multiple outputs um
and the key question that um dspi is to
answer automatically for you is what is
a question like how do I tell a model
this is what a question is and this is
what you should do to it you know to
generate a reasoning and this is what a
reasoning is and this is what an answer
is um but this is something that we want
uh to learn from the from the data so
you could have say things like document
to summary or context and question to
search query um or as we said question
to answer um and you can obviously
specify a lot more constraints you don't
have to it doesn't have to be a string
like this you could have a more like you
could say I want the output to be you
know integer and and I want you know I
want it to behave like this or that
there there are various ways to
constrain these things but the key thing
is that what we'll Define what a
document or search query or reasoning is
is something that we want to learn
within the context of this program that
we built um so um I guess maybe we I can
stop after this part and and and and
just make sure we're on uh you know we
we
we everything makes makes sense to folks
in the audience um but let's say you
want to express this simple kind of
pipeline so you have a system that takes
questions and it generates search quties
it searches with them and then it like
asks further questions it searches again
and then it uses a retriever to find
some documents and then uses these
documents to finally answer the question
so in DSi it would express it like this
um and initialization you think of this
almost like a neural network if you are
familiar with that stuff but now each of
these layers is actually a language
model call if you're not familiar with
neural networks and pyour and all that
it doesn't matter you don't you don't
need to know that but if you are
familiar it's a good analogy so now
you're going to declare um that you just
want a chain of thought that will
Express each of the blue uh Parts here
so both of them take a question and
generate the quity
um but you know they could also take
some context that was retrieved earlier
or they could take an empty context at
the beginning and the black sort of box
here uh takes this context that was
retrieved and takes the question and
generates an answer and this is the full
sort of description of of you know the
different blocks on the on the
right you can then sort of compose them
together in a forward function where
you're calling these modules so you're
generating query and then you're
retrieving um and then you're dumping
the stuff you're achieving in this
context and then you're generating an
answer at the end um so this is the the
forward function and and this is
basically nothing but like a very direct
translation of the diagram into a piece
of code um with the interesting piece
being that we have these signatures that
are telling the model what it's supposed
to be doing um so these are called
natural language signatures um and we
touched them we touched on them like a
couple minutes ago um how these are or
like you know the strateg or the control
flow of how these signatures will be
expressed are are through what we call
modules so there are several modules in
the5 or all the kind of prompting
techniques that people have um if you
want to do Chain of Thought in general
as we said earlier you kind of have to
think of how to teach your particular
model this particular reasoning strategy
but in thep it's just a module that you
give your signature and there's an
actual like module there that is going
to learn that particular pattern for us
um and I I think I might have a slide on
what it's what it does you know in the
case of Chain of Thought but the
interesting piece here is that
internally this Chain of Thought is not
like a template uh although it has a
template um it's actually a module that
maintains three things it basically is
going to remember what instructions it
should use and so the idea is it wants
to learn the right instructions for your
language model for this particular
signature um it also wants to learn what
input out out with examples it should
show to the model and um if you use fine
tuning then it also wants to know you
know what model fine unit should be
using and there are other Notions of
constraining the outputs like assertions
where you say well you know I don't just
want to generate a query I want this
query to have a particular property for
example I want it to be less than 100
words um and I want the program to learn
to generate queries that pass my
assertions uh um so I'll pause here for
a bit and then we can continue and again
apologies that the slides are not really
kind of purpose built I I wasn't
expecting to present them no this is
great um a bunch of questions around
optimizers and you mentioned this is
kind of like one of the the two big
things what exactly is being optimized
and and how how does that work yeah
that's do that um so I guess this is
another way of asking you know what
defines the context the question the quy
these are terms and if you go to a
language model and you say I want an
answer it kind of has some prior over
what that might mean but it might not be
exactly right
um this is we should go we should get
back to this so that
people understand actually let's go in
order so that we don't forget so
prompting strategies like Chain of
Thought they're just dsy modules too the
difference is that they're going to take
our signature and change it so they're
going to take our signature and say like
oh I just want to add a field um that's
going to be like let's think step by
step or or whatever um and then I'm
going to build kind of a another module
with the newer signature and then
basically I'm going to like forward all
my calls to that um to that extended
extended uh um uh signature and this is
nice because now this extended signature
is is what we would learn we would
actually going to learn like you
specified that you have questions you
want and you want answers but when you
have a Chain of Thought we're actually
going to learn this reasoning um uh
field as well uh when we're optimizing
this thing um yeah this is so the role
of of the of the language program
optimizers is this process of taking
each of your signatures and figuring out
how to construct those prompts um and
you know there could either be by
creating instructions or by creating
examples or by building data to find
tune models um for the same signature um
and the way this is going to work is not
blind you don't just go to the model and
say like give me good prompts which I'm
starting to see out there that doesn't
make sense because language models you
know they're not necessarily aware of
what will work as a good prompt they
give you a long prompt for sure and odds
are it's not completely random but it
might not actually be very effective um
instead what you want is some way to
have to get like to get feedback um so
you want to build a metric um and you
want to have a small number of examples
maybe you know 20 some people are
successful with as little as 20 usually
around 50 or 100 is is is good um
sometimes without any labels um you can
still U proceed and you know I don't
think we need to like go through the
formula but basically um the idea is
we're trying in these optimizers to
search over basically the design space
of these prompts um to maximize the
quality on average over your examples
and I say sometimes it's without labels
um
metrics can be something like the output
of my let's go back to the pipeline we
had here one metric one set of uh of
of Optimizer inputs here could be take
this program here are 20 questions and
here are their answers and the metric is
you know does the answer match what I
what I give you but a different uh type
of of metric could be here's here's set
of 20 questions um I don't know what the
right answer is but I do know that I
Want U my answers to be not based on
hallucinations I want them to be
grounded so my metric is going to be
another DSP program that checks for
hallucination um and it says when I get
an answer from my system the text that
I'm getting is actually grounded you
know is is based on the documents or the
context that was retrieved and the nice
thing about that being a DSi program is
that we can actually optimize that
metric so that it is reliable at
detecting hallucination it's just a
classification problem it's very easy to
optimize um and now we have a really
good way to optimize this program so
that it generates answers that we that
don't have hallucinations and we did not
even need labels for that but in many
cases you know if you have some labels
you should use them
um so the general idea of these
optimizers is we want to learn module
Behavior by generating examples so
you're giving us those inputs um let's
actually do this again on the same
diagram you're giving us
um you're giving us this program here
and you're giving us 20 questions and we
want to teach the model to generate
search queries but we don't know what
types of search queries will work for
this retriever like you know you just
switch from kber V2 to like I don't know
kber V3 when it exists or something or
to like a um u.com search or some other
thing um and the types of queries that
work for each of them is different so
how can you teach a particular language
model to generate good queries here well
one idea is what if we take this
question and we actually try to run it
through the pipeline in various ways and
actually we trace the um inputs and
outputs of this stage and see if they've
been effective in the past at you know
getting the retriever to do what we want
and we can in general detect that
because we're measuring you know um
we're measuring the the the metric uh
the end or because you're giving us
those assertions although um you know in
general you don't need them that sort of
give us a signal as to whether things
are going right or not and when we have
those examples well we could um you know
we could go back to the um optimizers
here and basically use them as generated
examples for each of these stages of our
system um so this is the notion of
bootstrap fuse shot and then there are
extensions of that that you should be
using if you want to treat this as an
actual optimization problem like boot
have F shot with random search um and so
that's basically saying um I'll create
few shot examples for St for the stages
of your pipeline starting from your
inputs um and to choose which
combination um you know I I should work
with I'll run this random search process
um trying to maximize your
metric um bootstrap Point tune is just
saying well since I can create examples
now and I have this potentially
optimized set of prompts I can create a
lot more data now pretty efficiently and
use it to actually update the weights of
each of these modules through my
pipeline assuming that you're working
with a language model that we have an
API to find tun it so not you know you
can't do this with gp4 for example and
in general you shouldn't um even if they
give you an API to find tun it but maybe
you can find tun in a small llama model
or a T5 model um or you could do things
that actually learn instructions and
examples uh like the recent Meo that we
released uh like a couple of months ago
um and several other other stuff and I
think um people look at optimization and
they kind of fear that this stuff is
expensive but in reality you know partly
because llms are so cheap if you're
doing like 3.5 level models um
optimization is something that generally
takes a few minutes and you know a few
dollars um for a new kind of setting
that you have um if you have a couple
hundred examples if you have less it's
even cheaper um but don't you know
generally start small and generally
start with smaller models so you
understand what's happening and can sort
of track the quality before adding
complexity or scale um so I can tell you
how the me Optimizer works this is you
know this you could also look at the
thread about Meo and and other resources
um but I'll also pause here and just
sort of make sure we're uh we're doing
well on time as well so so just to maybe
summarize some of that and correct me if
I'm wrong but it seems like there's
three main ways that you can kind of
like optimize some of these programs you
optimize the instructions the the
examples or the few shot that you're
giving or the weights of the underlying
model itself and sometimes combinations
of them is is that correct or is is that
yeah this is right this is exactly right
what's interesting though is um if you
take a
program and and this is not really
changing what you said Harrison it's
kind of just building on it um if you
take this program and you optimize It
generally the easiest way to get started
is to optimize over examples and this is
giving you like a pretty power powerful
boost very quickly um um and and the
idea isn't just adding examples it's
like the notion that they're actually
coming from your program and there're
things that worked before and then on
top of that if you want to do random
search or you want to do bean search or
like ban optimization um um to sort of
Select these things uh these things
together what's it can be really
powerful but what's really cool here is
that when you take this program and
let's say you optimize it for GPT 3.5
with bootstrap F shot with random search
for
example or with Meo so let's say use Meo
so this gives you a setup where each of
these modules now learns a new
instruction and learns like two or three
nice examples um in in the prompt you
can take this exact same um optimized
program and say well that's my teacher
program now I want to actually learn
again with bootstrap F shot or with Meo
um but now I want examples that are
being constructed to come from this
pre-optimized version and so you're
basically composing the optimization and
what's really powerful about that is in
some cases it means that you can get
much better examples um because now
you're sampling them from this better
program um the other thing is now that
you've optimized your prompts you can
take this really high quality version
and Nest Nest it again into optimizing
the weights so you don't need to start
each of these from scratch you can
actually compose the process of
optimizing them and when when I say
compos the process of optimizing them I
don't know if that sounds scary or not
but in in practice that's like two lines
you just pass an extra argument
somewhere I can try to find if I have a
an example of the um of the code for
optimizing it's like two lines but it's
not in this set of slides I I'll get it
at the end if we want um yeah uh that's
hope that answered that question H yeah
absolutely another thing I wanted to
highlight because I think it's um I
think it's a really interesting
similarity between kind of like Lang
chain and and and DSP is something you
said around kind of like tracing and
traces and just to emphasize why I think
this cool is like you could have a
program with like 30 different steps in
it and when you're talking about
creating these uh data set of examples
you're not talking about creating a data
set for each of those 30 steps you're
talking about creating one for the whole
end to end Pipeline and then from that
you can generate you can generate
synthetically examples for each of those
30 steps or instructions for each of
those 30 steps but you do it once for
this high level pip
and that that all and I you know I I dug
around in the dsy code based bit and as
far as I like tracing is really
important for that and and so um do you
maybe want to talk a little bit about
like what tracing is and why it's needed
for that and like what it actually
provides yeah sure um so the idea there
is you've written this program or you
know this could have been you know a l
chain chain we have we have done this
nice collab in the past where you know
you could take an LCL expression and
then basically run a dsy Optimizer on
top and we showed like a nice cool
example of I think it was
optimizing rag to generate engaging
tweets or something for anwers data set
um so basically you have this this
program or this chain with has all these
pieces and um as you said you have a
data set that's describing the task not
your pipeline and this is really
important because let's say you're you
know you're building a you're working on
a on a on a at a startup or building a
nice project um usually the task is not
going to change every day it's like I'm
writing this thing that's helping people
schedule on email or something that task
is fixed but your pipeline that you're
building is something you want to
explore all all all the time and what
that means is we want to enable you to
like go and break the pipeline add
pieces remove pieces um but also we
still want to enable some notion of
learning of what your task looks like so
in general as you said if if you have a
bunch of inputs and ideally a bunch of
good outputs at the end um if you don't
have that we could rely basically on
language model based evaluations um and
and in some cases that can take you far
in other cases it's harder
um now the notion of tracing is well
given those inputs if we just make an
educated guess about the initial setting
of each of your prompts of each of your
modules then we can simply add I know
set a set the high temperature and run
through your pipeline and just see what
happens right see if you we're getting
good outputs see if we're getting bad
outputs and we know that because we have
the metric at the end or because we have
these assertions but the metric is is
the primary thing here um and so let's
say it's you're answering you're
building a question answering setup well
what if I just tell the model please
generate search quties and you know it's
hit and miss right sometimes it's pretty
good as language models you know
sometimes they surp surprise us they're
pretty decent um other times well
doesn't really know what we're saying so
it generates I've seen things like it
generates SQL quties um or it generates
um you know um like quties in this in
the archaic kind of like um keyword
format with pluses and minuses and like
uh you know which many mod retrievers
are not really optimized for and it's
kind of Overkill or even even
distracting um for these models but like
you can't blame it it doesn't really
know like how would how how is it
supposed to know that it's not that it's
not to do that um but you know if you
sample many sufficiently many of these
um you know maybe a couple hundred um
you're bound to find some interesting
ones that perform really well um and we
can basically collect those traces so
that's what bootstrap fot does it it's
will store those traces and then we can
plug them back into the prompt and say
when I ask you to generate the search
quy here's actually a good example of me
doing that in the past and here's the
quy you gave me so how about do more of
that um and you know it's basically few
shot learning but on on this on these
traces um
and it becomes a discrete search over
those so assuming we can generate and
filter for good examples um optimizing
with having selected them is actually a
pretty simple um process from there on
the question just becomes like how do we
do this without like trying all possible
combinations or you know um other stuff
and sort of research on hyperparameter
optimization for NE networks is actually
very useful here because it's kind of a
similar type of problem where you have
the architecture and then you're trying
to plug in those hyper parameters but
you don't want to like try all
combinations in an expensive
way yeah that that's a that's a great
explanation um I see a bunch of
questions in the chat around how to
combine Lang chain and dspi um I think
that would be really interesting to
discuss before that I want to spend
maybe like three minutes um showing some
of the things in Lange chain and L Smith
that we've been working on because I
think they're exactly related to tracing
and uh uh testing and evaluation um and
data sets and so I I maybe want to set
the stage with that and then we can and
then I think the rest of the time would
be great to discuss okay like and maybe
brainstorm with the audience as well
what is the right kind of like way to
put these puzzle pieces together um so
let me share my screen really quick and
you were just talking about tracing um
and and that's one of the main things
that we've invested pretty heavily in at
Lan chain so so this is a this is a list
of chat Langan chain queries um so on on
we have a chat bot for Shadow over Lang
chain documentation and if I click into
one of these let me find one that has
multiple uh language model steps cuz uh
that would be interesting um so here you
can see that this this is a trace of
kind of what's happening and so this
this mimics um one of the uh examples
that uh Omar was talking about earlier
where you have kind of like this this
generation of a query um and uh then
there's this retrieval step and you get
back you get back uh uh uh documents and
then there's this generation of a
response um and so this this is a very
similar uh kind of like uh in this case
this is written in lell but it's it's a
very it's a very similar program uh
putting aside how it's written um and
again I think one of the key
importantance things here is the
importance of tracing and here we we use
it for observability we find that
there's huge value in just seeing what's
going on in this pipeline but as Omar
talked about you can also use it to
collect all these these examples um one
of the things that's interesting is when
you're collecting them in lsmith there's
actually a difference to how to what
we're collecting and it's a very
productive difference um faes we collect
are like they're kind of synthetic on
like you basically hit compile or you
run the code with compiling and it's
collecting them but the traces you're
collecting here are maybe like
interactions with users and they're kind
of in production and they increase over
time and they kind of get sustained the
traces in our case we build them we
optimize we throw them away essentially
or we cach them but we we're not you
know this is this is a different type of
tracing but it's you know very very uh
valuable yeah no that's a that's a great
point I think it comes down to like what
the data set is right like what's the
data you're running it over in in the
case of uh DSP it's a bunch of examples
that you've curated and that may and you
have some metrics for and maybe you have
some labels for in this case this is
like production traffic um and I think
there's pros and cons to each I'd say
the pros probably of this and and to be
clear we also have this concept of kind
of like data sets contesting and Lang
Smith which I can jump to but the pros
of that is that you generally have maybe
thought a little bit more about metrics
and you have maybe have labels as well
like you have ground truth labels so you
can create better metrics here the pros
of this are you you get to see questions
that people are ask actually asking kind
of like your chatbot in in real life and
so I think there's just a broader swath
of them for better or or for worse but
yeah you're absolutely right that it's
different different settings that that
this tracing is being done in um
and and on the concept of kind of like
data say one of the things that we
purposefully added since the beginning
is this really uh or is this concept of
adding um any part of this Trace to a
data set so you can add like the high
level thing you can add like this
individual kind of like component you
can click add the data set and you can
basically add it to to an existing data
set um data sets themselves uh just
showing them really quickly are nothing
more than kind of like examples um so
examp examples of inputs and optionally
outputs um so so as aror said there's a
bunch of cool things you can do if even
if you don't have outputs um and then
you can run tests over them and so I
think the tie in here is like just as
you would Define a metric to kind of
like optimize your system here you're
defining a very similar metric and a
very similar kind of like data set but
you're using it for this this kind of
just like evaluation of your system as a
whole um and so I think very very
similar Concepts kind of like across the
two we've also this is one thing we've
added recently is this way of kind of
like moving data um automatically from
production logs into data sets um and I
think uh one of the things that we
wanted to make sure if if you think
about what um and and tell me tell me if
this is a a bad understanding no more
but what you're what you're doing in the
optimization is you're basically saying
uh I'm going to run it over these high
LEL inputs and high level outputs I'm
going to look at places where it was
scored correctly at it was scored
correctly and then I'm going to take all
the like sub examples for each
individual node or thing associated with
that and create a data set of few shot
examples or a data set to find tune on
or or somehow update the prompt
instructions for those nodes but
basically there's this again there's
this this Trace which has the
association between highle metric
low-level actually what went into the
prompt what actually are the examples um
and and that's kind of like the data
sets that you want together um that's
exactly right that's exactly right and
when you said instructions you basically
are taking this small data set and
you're basically getting a language
model to say oh well the task that is
kind of um described by those examples
is the following instructions and you
want to generate several of these so
that you can run run a search over those
as well but it's really it's building
those mini data
sets yeah and so that's that's one of
the things that we put a lot of um
effort into and so when you have fil
filtering here it's not just filtering
on the top level that's the default but
you can do things you can add filter so
like okay give me uh all things where
the name is like find find docs or
something like this so this would be a
substep then you can add a filter on the
trace so you can say okay all places and
Trace we mean like high level parent
level Trace so give me like all all all
sub runs where there's this fine doc
where it has this highlevel user score
is one and you can filter for that and
then you can set up an automation um and
you can set up an automation where it
automatically adds all of these to a
data set over time or something like
that um and so kind of like
programmatically taking these production
data and building uh and then and this
is where this is where I think uh you
know it's maybe a good spot to
transition into like how to merge these
because we have no concept of
optimization um and and so you know
that's obviously one really big thing
that's missing from things in Lang chain
Lang Smith and and and DSP and the value
it
provides and and maybe just backtracking
you into the beginning I know you
mentioned in the beginning there's kind
of like two big components one's like
the way of of expressing this program
and then the other is kind of like this
optimization um how with with those
things as context how how can we think
about combining Lang chain and
dspt so I I um and and we've disc this a
couple times Harrison um I I like to
think of P of DSP as sort of P torsion
dis stack so if you I I like analogies
and if you think of deep Neer networks
as like this wonderfully successful um
Paradigm in in machine learning that you
know got us here with language
models it really only I think there was
a threshold of Crossing from very fairly
messy space to like something where we
can actually all talk about the same
thing precisely um or or and and sort of
run the same code with the introduction
of like Fano and you know Cafe and
eventually things like pytorch and
tensorflow and other and other cool
Frameworks um and byor is kind of really
neat in my opinion in a bunch of ways
but it's not unique so I like to think
here of like the stack we have in neon
networks there is something like pytorch
and then there's lower level things like
you know um kernels you know um you know
mkl for CPUs or you know various Cuda
stuff and then on top of that there are
higher level things like hugging face
Transformers which is you know most
people I'd argue a lot more people are
familiar with using using hugging face
Transformers than pytorch um because you
what you just want to get birth or gpt2
or one of these models and you just get
it ready from there byor is more of like
here are the here are kind of like the
modular building pieces good luck um
here are optimizers and here are like
layers and here's convolution layers
here's Dropout layers um do something
cool um and I kind of like to think of
DSP as this the byor of this stack of of
basically saying you know it's a small
framework we're going to give you these
layers here's a Chain of Thought layer
here is a program of thought layer here
is a react layer that um sort of is is
is also similarly abstract and here's
the notion of signatures and the notion
of optimizers here is how you build
stuff with them good luck sort of you
know um and and we can help you sort of
think about how to construct this these
programs um if you want but otherwise
here is a bunch of optimizers that we're
continually expanding to take these
programs and give you high quality
systems um in my conception obviously
you know please feel free to correct
that or otherwise like you
know describe your your your framing um
one of the things it's not necessarily
the only thing one of the things that
Lang chain is just outstanding at is
kind of how it it brings in higher level
pieces of the stack and also lower level
pieces of the stack so you have all
these like language models integrated
and all these like uniform abstraction
around all kinds of tools that's on the
lower level but on the higher level you
have all these you know various chains
and various agents and lots of sort of
um sort of tracing systems and other
things that that say like well um
assuming you know the right prompts or
assuming you're given the right prompts
in one way or another um or that you're
using prompts that are sort of pre
pre-built um either from The Hub or that
are sort of internal to L chain you know
or otherwise um here's here's what you
could do with it and here's how you
could sort of think about streaming and
think about um these types of these
types of of things in DSP we don't think
about almost any of that um except to
the extent that we strictly need um
where we're focused is um what are the
right declarative modules like Chain of
Thought um and what are the right um
sort of fundamental abstractions like
assertions or metrics um and what are
the right optimizers and this is really
where we spend most of the time it's
like how can we think about the
algorithms that optimize this so this
was a long way of saying um in in
principle any any L chain um uh system
any any chain um is um a class of of
these language programs um and as we've
shown together in the demo before that
that like integration we did earlier um
like kind of a beta level integration um
it's nothing preventing us from thinking
that sort of compiling it with bsp to
get like you know to get new prompts
plug them back into L chain um and then
you have a chain that has been optimized
with these prompts but what you showed
today with lsmith is also of the sort
where you could also have another
plug-in space which is well not just the
optimizers um building the data set so
you you know you're starting out you
have no data set you've written some
initial prompts you're they're good
enough to to create a demo um and
they're good enough to like put in front
of your friends and some users to get
some feedback and and that means you can
get you start getting a wealth of really
really realistic data into the lsmith
traces and if we could export that in a
smooth way into like here's the data set
for DSP and maybe here is like a way to
think about the metrics I'm not sure
what's the best way to to do that but
maybe there are like simple abstractions
that we could build in that space um
yeah so yeah here is the metric here is
the chain I have here is the traces from
the data you know from putting this in
front of some users or maybe I already
have it in production and I already have
the traces in some cas
um um yeah let's let's see how how much
better an Optimizer could do at this and
kind of like plug it back in the ways in
which this is this requires development
effort is Lang Lang chain gives you a
lot of cool things around like I think
the main reason you have an expression
language or like a graph abstraction is
because you want to be able to think
about streaming and think about um you
know um kind of production type um
deployment type things and so the
question is how do we take the you know
how do we take prompts that we are
producing and not lose that part in in
Lang chain so plug them back into the
chain that you've had and we've had
simple stuff you know in that stage with
with the stuff we built before but I'm
pretty sure we could kind of also make
it more General and more modular and
more robust and I'm personally very
excited about that type of
thing yeah maybe okay maybe maybe taking
some of that it see in my mind there's
maybe like three main things that are
interesting one which I think you you
said I hadn't thought of in until this
but I think is maybe the easiest is just
like um around like the the data sets
and evaluation like you can use those
for different things you can use them
for optimization you can use them for
testing and eval and one of the big
pushes we're doing is like you like
evals are so important and like they're
important not only for knowing how the
system's doing but also so you can
optimize it and so I think like having a
really seamless kind of like handoff
there is incredibly easy and like having
a way to export Lang chain data sets
into DS piy format um having a way to
use uh metrics in one or the other that
that seems like a pretty easy kind of
like just just compar just yeah just
straight up thing there um the other way
I I think like you know both uh if you
think about the two big things of DSP as
you said there's one which is like the
way of expressing the control flow and
then there's the optimizers um and lell
and lingraph are also ways of expressing
control flow with different with
different benefits right I think they're
focused on on kind of like different
things and so one I don't know how much
work this would be but like one thing is
definitely just a way of switching
between those two control flows so like
you know we we did a very simple version
of this um but yeah a much tighter
version would be like any program
written in kind of like dspi could could
be compiled to lell or or to lingraph um
and and I yeah and and that's that
probably a largish undertaking but if
anyone in the audience is you know I
think that's a really cool project that
would get you really familiar with the
guts of both and then a third and I
don't know how feasible this is you know
you
like the there's there's so many
optimizers in DSP could those be applied
to a different control flow kind of like
thing so is there a way of using
optimizers on like raw Lane graph syntax
I'd assume there need to be some kind of
like conditions met one of the big ones
I'm assuming is this concept of Trace
which again has been like such kind of
like an emphasis for it that's that's
the main reason I'd be bullish on this
but I don't I you know the technical
details of this way better than I do so
like is that even feable this is this is
easier Harrison so um
optimizing is easier I mean at least at
least like yeah intellectually for sure
probably also in an implementation sense
um then compiling let's say which I'm
very interested in both but compiling uh
or like I mean it's a different sense of
compiling so I don't want to confuse
people but like taking a a DSP program
and emitting an A Lang graph or
LCL LCL could be hard I don't know um
how to so so here's here's the here's
the thing the challenge is in principle
people could put put like exceptions or
like recur or like some really you know
like free form things and obviously as
you said there's a trade-off it's kind
of like if you think of um kasas versus
like pytorch
most Neal networks are expressible
pretty easily in both but if you do
something really crazy in by torch then
you're sort of like how do I and and it
can be done eventually it can be done
it's just a little harder especially
automating it might be even harder but
the notion of and and you know I mean
maybe maybe doing it for like the 90% of
applications is good enough I mean
that's that's going to be a pretty big
win in general for the community um but
optimizing Lang graph objects is
probably like conceptually very simple
and we just need to figure out and so if
somebody's interested to chat like you
know somebody in the community wants to
help with this I'm sure Harrison and I
certainly like on our end we're happy to
help you and you know uh think about how
to do
that yeah I would I would I mean I will
chat offline I want to explore what that
looks like because I do think yeah like
getting going from DSP to lell 100% is g
to be a pretty large undertaking so if
you think it's easier to to apply the
optimizations like you know
fantastic I we've got two minutes left
so I wanted to ask you uh one other like
interesting question we've talked about
optimizing prompts we've talked about
optimizing examples weights what about
the flow itself have you thought about
like what it would be like to optimize
the flow
itself yeah um I'm sometimes like I I
the the context of the stuff I show
today is I like to think about my big
issue with language models is that
they're are as as powerful as they are
they're bad at being they're bad at
control I like to control these things
when I'm building a system and they're
not really amendable to that lots of
things in Lang chain are helping with
that um and they are bad at sort of
planning or search and like optimization
in in the sense we have in thep um the
issue with optimizing the flow is it's
kind of also giving giving up control
over the part that's like grounding Us
in understanding what's supposed to
happen um in principle you could have a
program synthesis or even just code
generation Loop where you're asking gp4
like here are 10 examples of thei
programs please generate a new one for
this task and it will work like it does
generate valid programs um but the
question is like do we want to do that
like at this point at this point I think
language models are so blackbox and the
contribution of things like the supp and
length chain is that hey you could
actually think about like could focus on
this program or this chain and focus on
the composition and focus on like
understanding those pieces together and
so I'm I'm very reluctant to already
move into the world where it's like more
black box more magic and nobody knows
like what the actual program is going to
do and I suspect it will not help
quality I think there's a lot of value
in designing those things um which which
is which is back to this notion of like
flow engineering like bringing in these
pieces being careful about
them yeah yeah that's that's a great way
to end it as well we're actually doing a
webinar uh with itamar uh from from the
alpha codium paper on Flow Engineering
in a few weeks so that's a great way to
end it as well and yeah optimization and
flow engineering are definitely two of
the most interesting things that I think
are are people are talking about in the
space right now so thank you Omar for
coming on this was a lot of fun thank
you everyone for tuning in I hope I hope
you enjoyed it awesome thanks so much
all right
cheers
関連動画をさらに表示
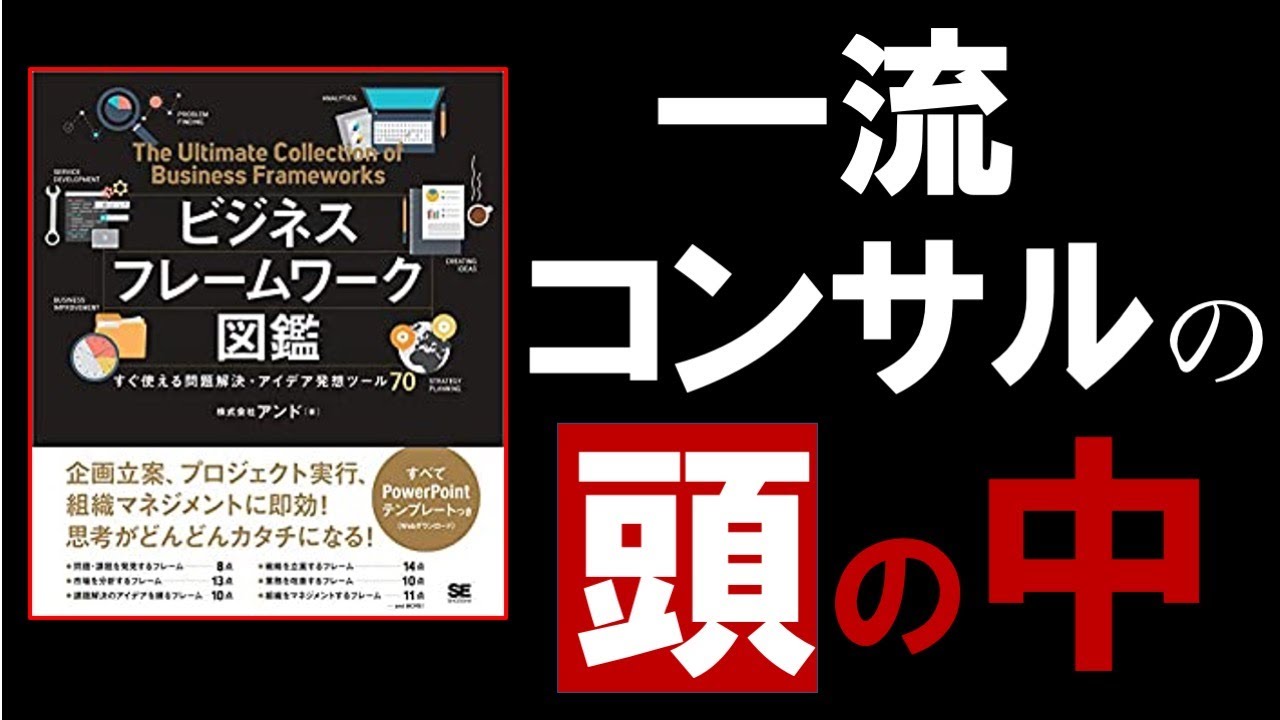
【13分で解説】ビジネスフレームワーク図鑑 すぐ使える問題解決・アイデア発想ツール
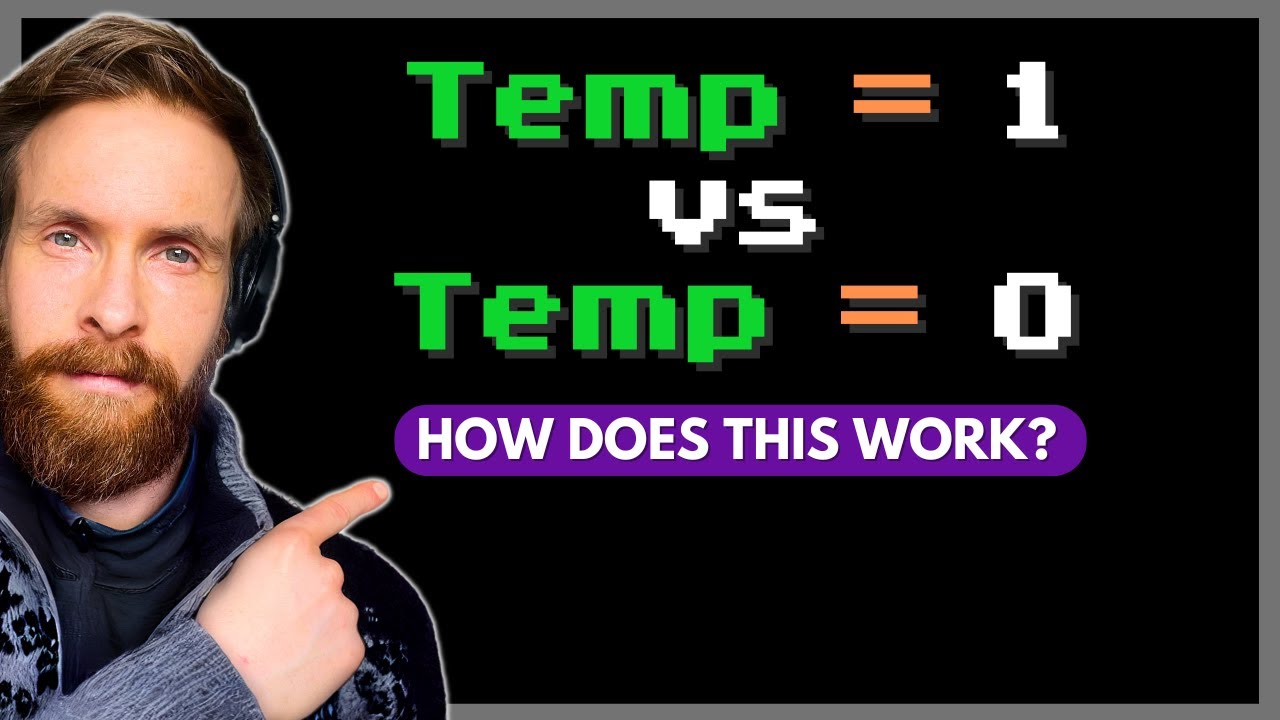
Determinism in the AI Tech Stack (LLMs): Temperature, Seeds, and Tools
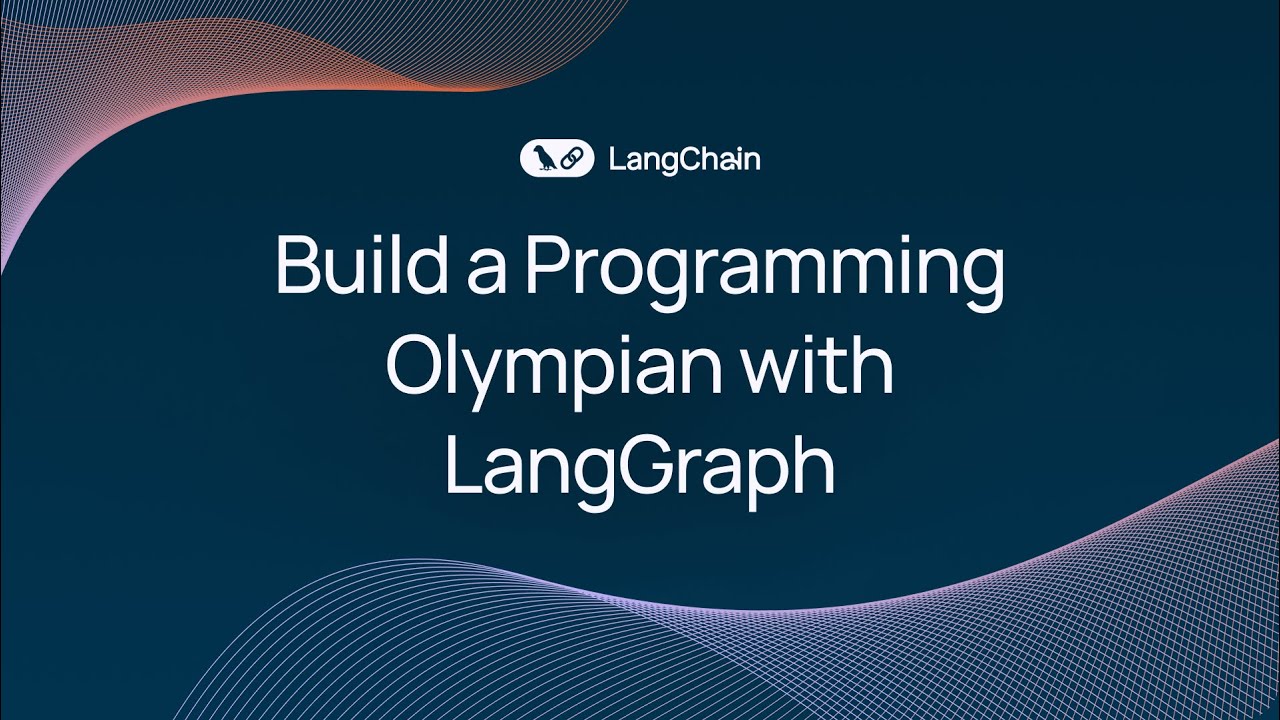
Build Computing Olympiad Agents with LangGraph
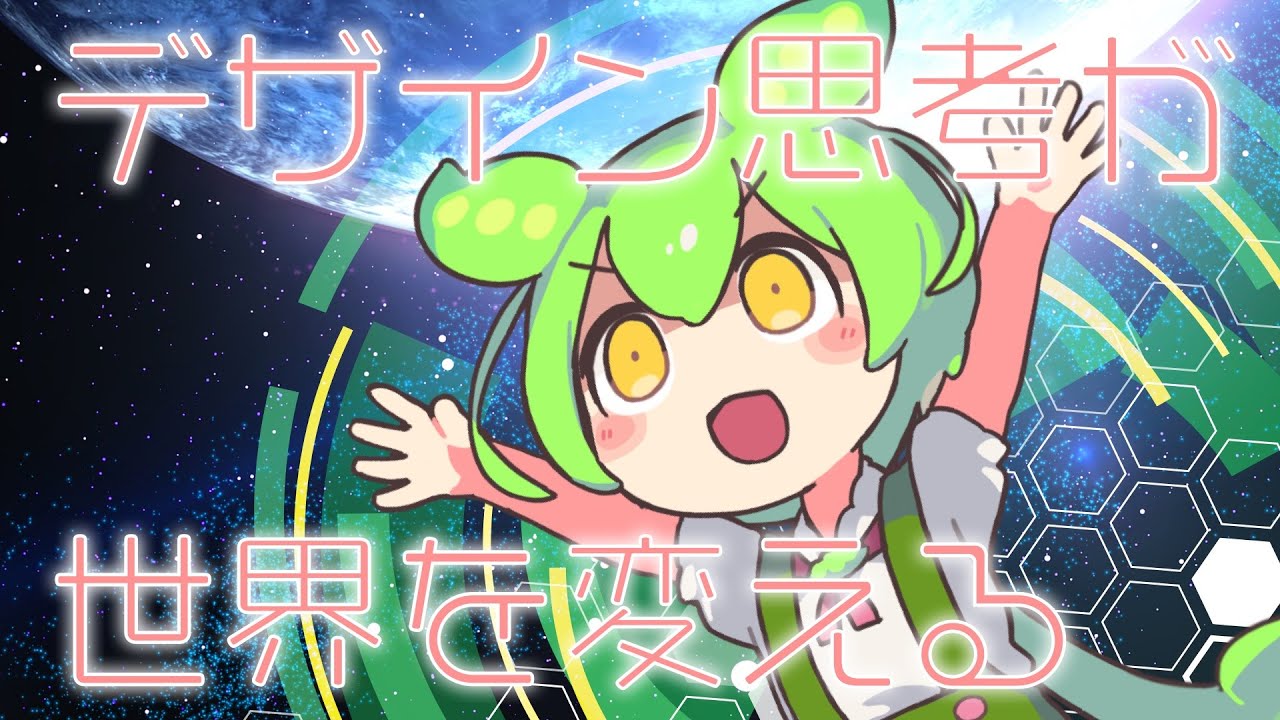
ずんだもんと学ぶデザイン思考【VOICEROID解説】
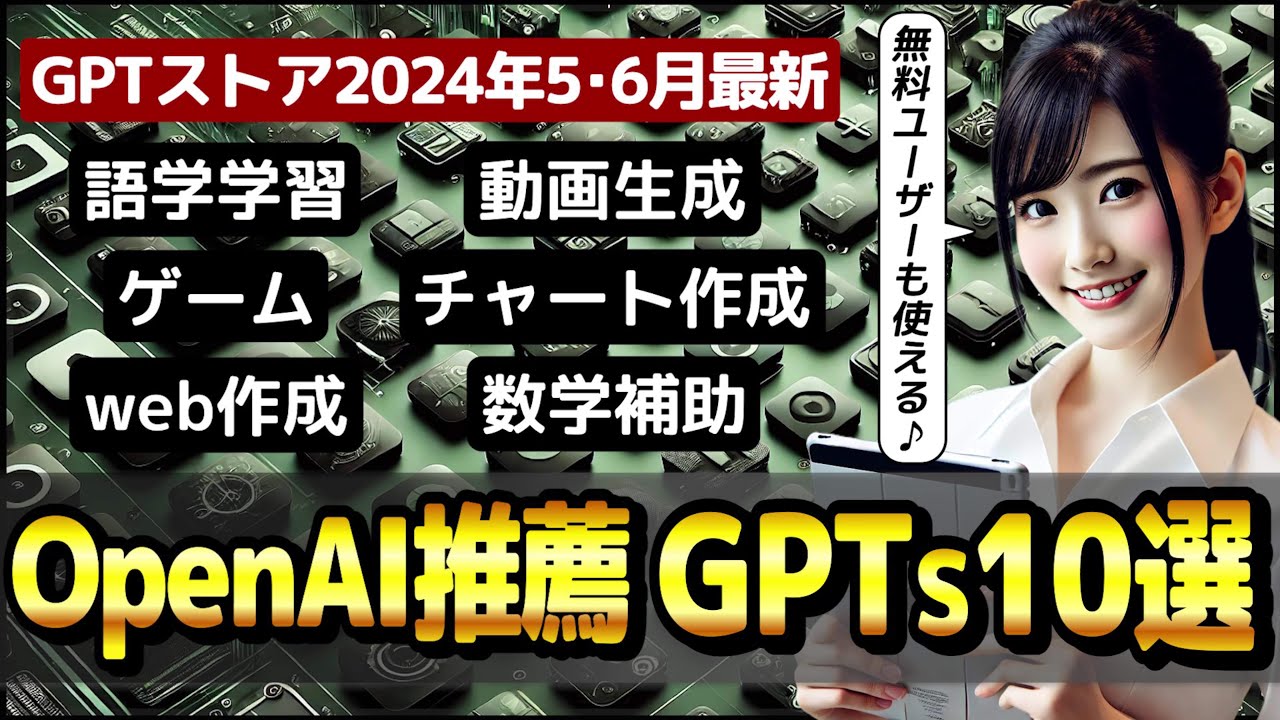
ChatGPT無料ユーザーも使えるのGPTストアのOpenAI公式オススメGPTs10選! ~2024年5月&6月最新版~
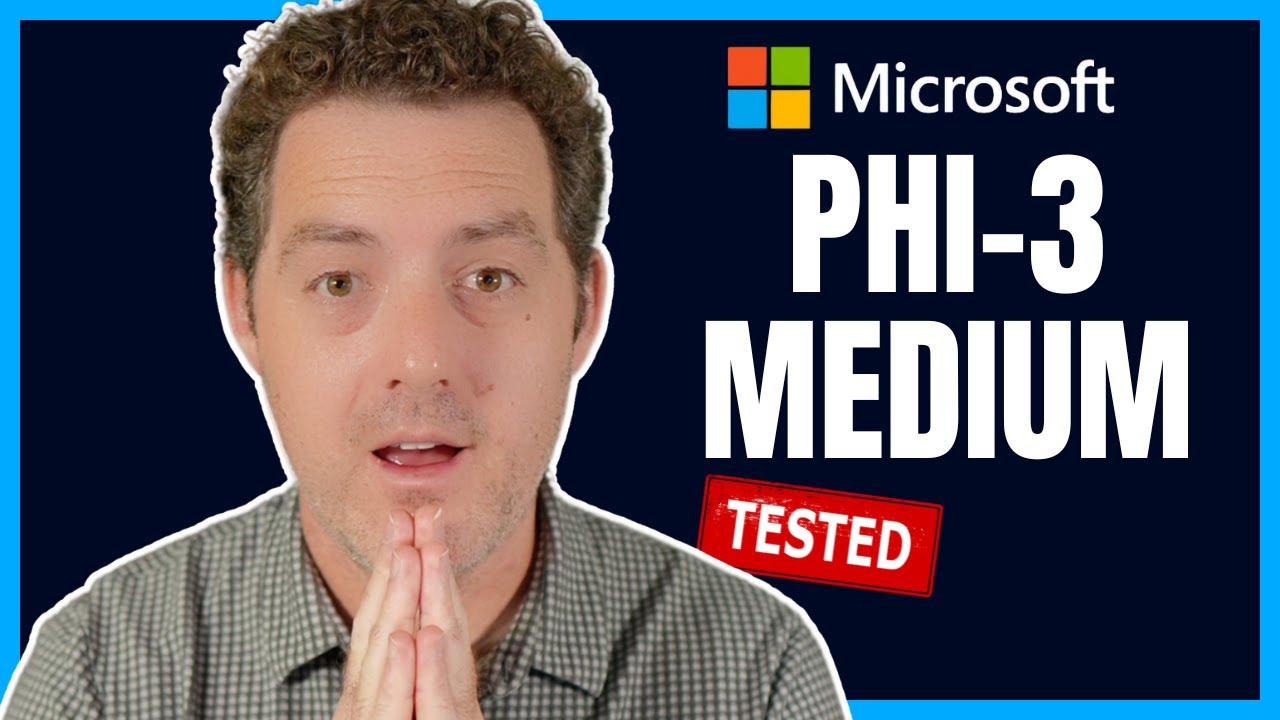
Phi-3 Medium - Microsoft's Open-Source Model is Ready For Action!
5.0 / 5 (0 votes)