The Central Limit Theorem, Clearly Explained!!!
Summary
TLDRIn this StatQuest episode, Josh Starmer explains the Central Limit Theorem (CLT), a fundamental concept in statistics. The CLT states that the distribution of sample means will be normal, regardless of the underlying distribution of the data, as long as the sample size is large enough. The video provides clear examples using uniform and exponential distributions, demonstrating how the means of samples from these distributions converge to a normal distribution. This theorem is crucial for statistical inference, allowing for the use of means in confidence intervals, t-tests, and ANOVA, even when the original data distribution is unknown. The video also addresses the common misconception that a sample size of at least 30 is required for the CLT to apply, showing that a smaller sample size can suffice.
Takeaways
- 📚 The Central Limit Theorem (CLT) is fundamental in statistics and is relatively straightforward to understand.
- 📊 To comprehend the CLT, familiarity with the normal distribution and the concept of sampling is beneficial.
- 🔢 The CLT demonstrates that the distribution of sample means tends to be normal, regardless of the underlying distribution of the population.
- 📊 An example using a uniform distribution shows that as more samples are taken, the distribution of their means approaches a normal distribution.
- 📈 Similarly, an exponential distribution, when sampled, results in means that form a normal distribution, highlighting the CLT's broad applicability.
- 🧐 The practical implication of the CLT is that it allows for the use of normal distribution-based statistical tests even when the population distribution is unknown.
- 🔄 The theorem is particularly useful for creating confidence intervals, conducting t-tests, and performing ANOVA, which are all based on the sample mean.
- 🗣️ A common misconception is that the CLT requires a sample size of at least 30; however, this is a rule of thumb and not a strict requirement, as demonstrated with a sample size of 20.
- 🤔 The CLT has 'fine print' caveats, primarily that the samples must be able to produce a mean, which is true for most distributions except for a few exotic ones like the Cauchy distribution.
- 🎓 The video concludes by encouraging viewers to subscribe for more educational content and to support the channel through purchases of original songs.
Q & A
What is the central limit theorem?
-The central limit theorem is a fundamental concept in statistics that states that the distribution of sample means will be approximately normally distributed, regardless of the underlying distribution of the population, provided the sample size is large enough.
Why is the central limit theorem important?
-The central limit theorem is important because it allows statisticians to make inferences about populations based on sample data. It underpins many statistical tests and confidence intervals, simplifying the analysis of data from various distributions.
What is the minimum sample size required for the central limit theorem to hold?
-There is no strict minimum sample size for the central limit theorem to apply, but a common rule of thumb is 30. However, the video demonstrates that the theorem can be observed with sample sizes as small as 20.
What does the video demonstrate about the distribution of sample means from a uniform distribution?
-The video shows that even though the data is sampled from a uniform distribution, the distribution of the sample means becomes normally distributed as more samples are taken.
How does the central limit theorem apply to an exponential distribution?
-Similar to the uniform distribution, the video demonstrates that the sample means from an exponential distribution also become normally distributed as the number of samples increases.
What practical implications does the central limit theorem have for conducting experiments?
-The central limit theorem allows researchers to assume that sample means are normally distributed, which simplifies the process of creating confidence intervals, performing t-tests, and conducting ANOVA, even when the population distribution is unknown.
What does the video suggest about the necessity of knowing the population distribution?
-The video suggests that knowing the population distribution is not crucial because the central limit theorem allows us to treat the sample means as normally distributed for statistical analysis.
What is the significance of overlaying a normal distribution on the histogram of sample means?
-Overlaying a normal distribution on the histogram of sample means visually demonstrates that the distribution of the means approximates a normal distribution, which is a key aspect of the central limit theorem.
Can you provide an example of a distribution that does not have a mean?
-The video mentions the Cauchy distribution as an example of a distribution that does not have a mean, which is an exception to the central limit theorem.
What does the video suggest about the universality of the central limit theorem?
-The video suggests that the central limit theorem is universal in the sense that it applies to any distribution from which samples can be taken and means can be calculated.
Outlines
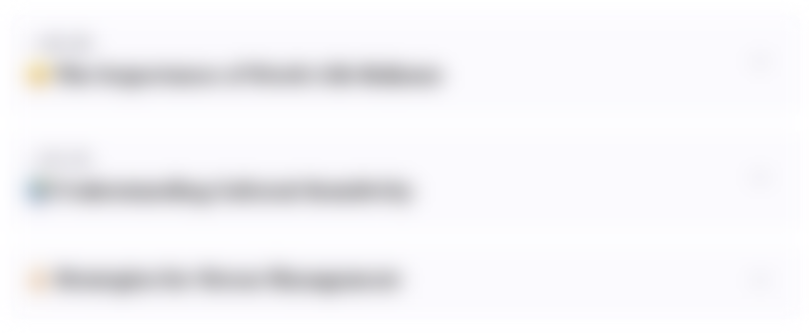
このセクションは有料ユーザー限定です。 アクセスするには、アップグレードをお願いします。
今すぐアップグレードMindmap
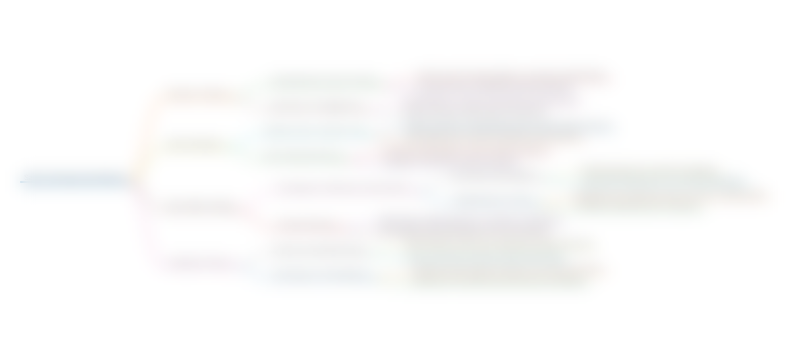
このセクションは有料ユーザー限定です。 アクセスするには、アップグレードをお願いします。
今すぐアップグレードKeywords
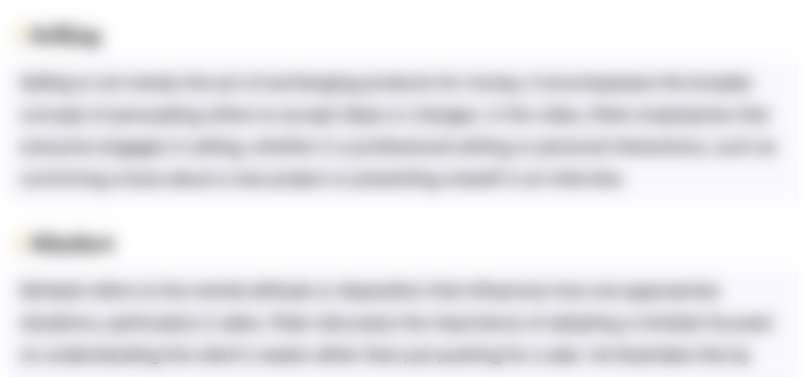
このセクションは有料ユーザー限定です。 アクセスするには、アップグレードをお願いします。
今すぐアップグレードHighlights
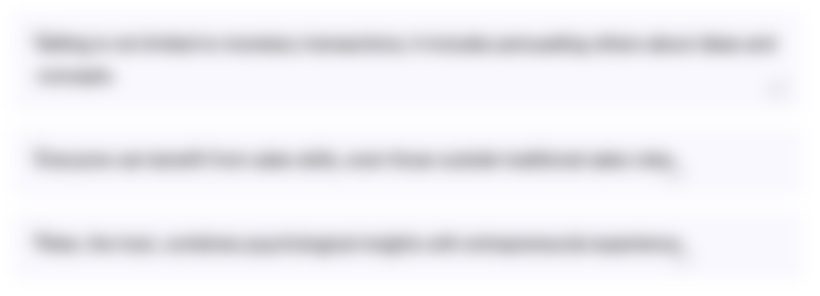
このセクションは有料ユーザー限定です。 アクセスするには、アップグレードをお願いします。
今すぐアップグレードTranscripts
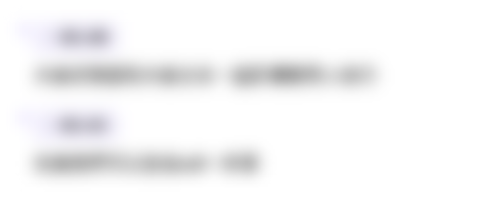
このセクションは有料ユーザー限定です。 アクセスするには、アップグレードをお願いします。
今すぐアップグレード関連動画をさらに表示

MINI-LESSON 4: CLT, The Central Limit Theorem, a nontechnical presentation.

Sampling distributions

Normal Approximation to Sample Mean
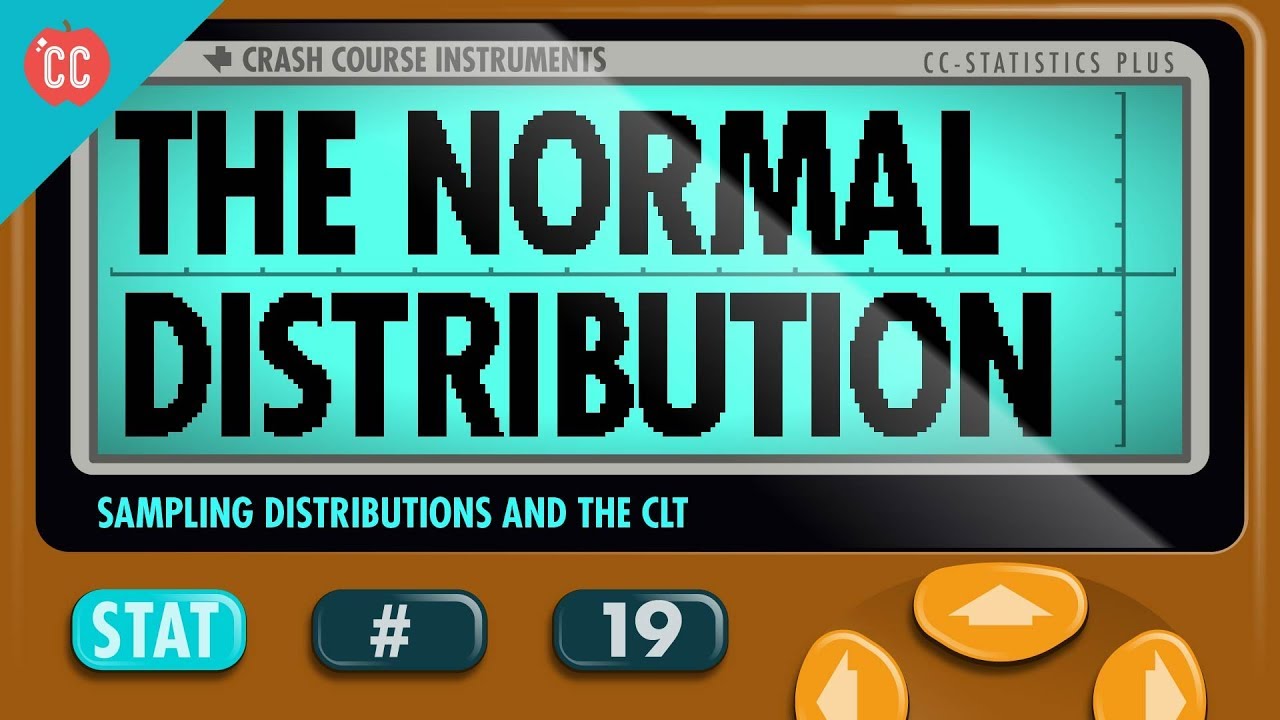
The Normal Distribution: Crash Course Statistics #19
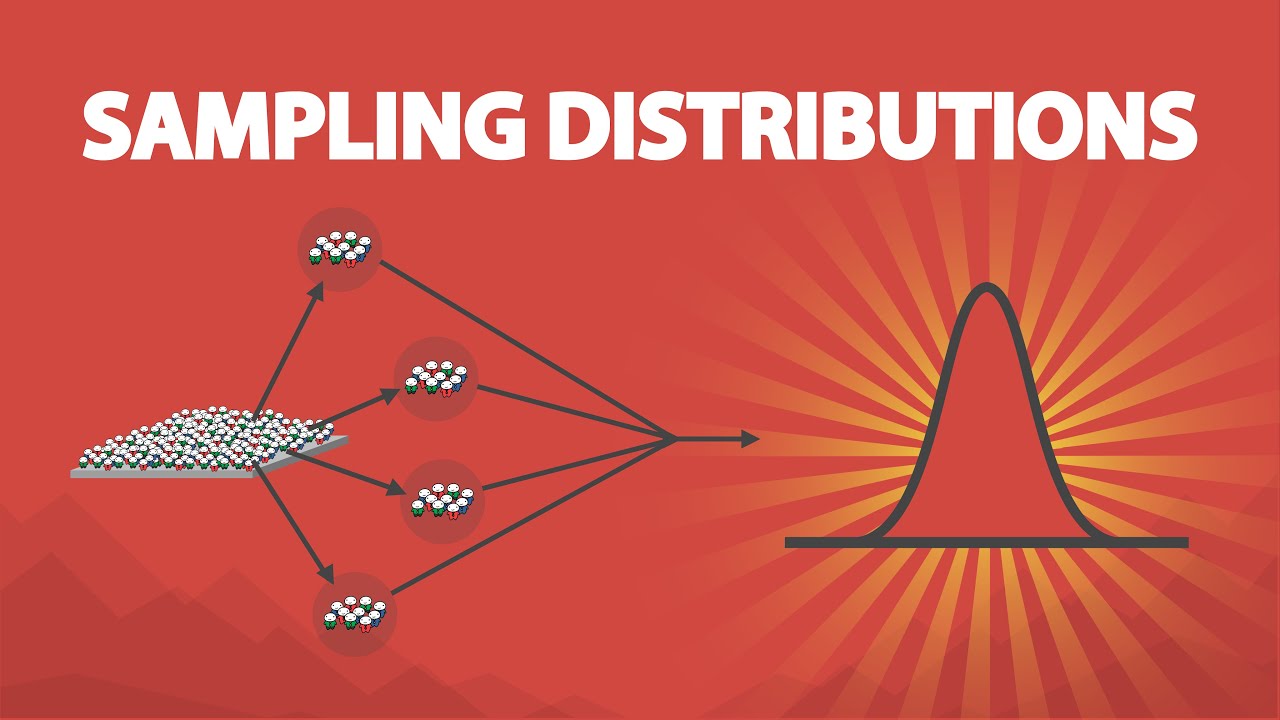
Sampling Distributions (7.2)
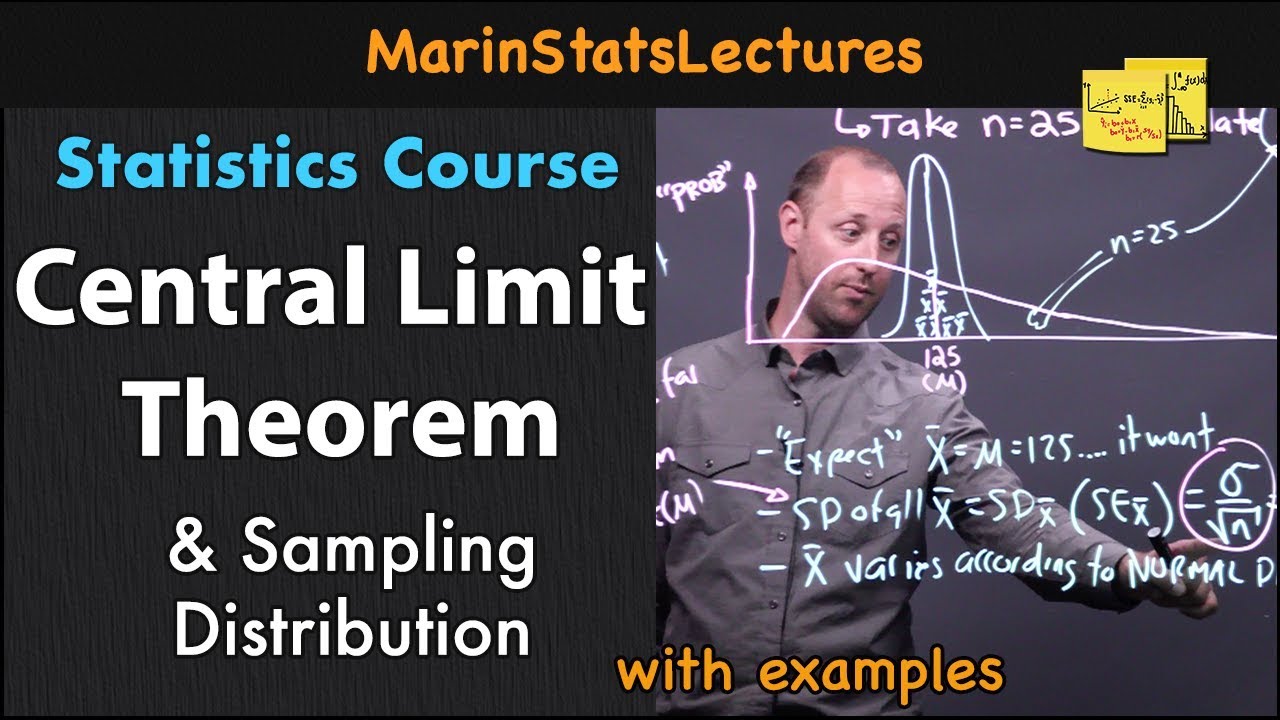
Central Limit Theorem & Sampling Distribution Concepts | Statistics Tutorial | MarinStatsLectures
5.0 / 5 (0 votes)