Week 5-Lecture 33 : Spearman’s Rank Correlation.
Summary
TLDRThe video script delves into Spearman's rank correlation, a statistical measure that complements Pearson's correlation by addressing non-linear relationships and outliers. It explains the computation of Spearman's rho, its scale from -1 to +1, and its robustness against non-linearity and outliers compared to Pearson's r. The script also emphasizes the importance of data visualization and choosing the right correlation coefficient based on data characteristics, reminding viewers that correlation does not imply causation.
Takeaways
- 🔍 Spearman's rank correlation is an alternative to Pearson's correlation coefficient, especially useful when dealing with non-linear relationships or outliers.
- 📊 Both Spearman's and Pearson's correlation coefficients measure the strength and direction of a relationship between two variables, but Spearman's is based on ranks rather than actual values.
- 📈 The value of Spearman's rank correlation coefficient (ρ) ranges from -1 to +1, similar to Pearson's, where a positive value indicates a direct relationship and a negative value indicates an inverse relationship.
- 📝 Spearman's rank correlation is calculated by first ranking the variables, then applying Pearson's correlation coefficient to these ranks.
- 🤔 The script emphasizes the importance of visualizing data to identify outliers and the nature of relationships before choosing the appropriate correlation coefficient.
- 📉 Spearman's rank correlation is less sensitive to outliers compared to Pearson's because it focuses on the rank of data rather than the actual values.
- 📚 The script provides an example of calculating Spearman's rank correlation using student attendance and exam scores, illustrating the process step by step.
- 🔢 The formula for Spearman's rank correlation is given as "1 - (6 * Σd_i^2) / (n * (n^2 - 1))", where "d_i" is the difference between the ranks and "n" is the number of samples.
- 📋 The script contrasts Spearman's and Pearson's coefficients by showing how they react differently to outliers and non-linear relationships in data.
- 📊 It's important to remember that correlation does not imply causation; correlation coefficients only indicate the presence of a relationship, not the cause of it.
- 📝 The video script concludes by advising viewers to choose the right correlation coefficient based on their data's characteristics and to always explain their choice when reporting it.
Q & A
What is Spearman's rank correlation?
-Spearman's rank correlation is a non-parametric measure of rank correlation that assesses how well the relationship between two variables can be described using a monotonic function. It is a measure that varies between -1 and 1, with -1 indicating a perfect inverse relationship, 1 indicating a perfect direct relationship, and 0 indicating no relationship.
Why might Spearman's rank correlation be preferred over Pearson's correlation coefficient?
-Spearman's rank correlation is preferred when the relationship between variables is not linear or when the data contains outliers, as it is less sensitive to outliers and non-linear relationships compared to Pearson's correlation coefficient.
What is the range of values for Spearman's rank correlation?
-The values of Spearman's rank correlation range from -1 to 1, where -1 indicates a perfect negative correlation, 1 indicates a perfect positive correlation, and values close to 0 suggest a weak or no correlation.
How is Spearman's rank correlation calculated?
-Spearman's rank correlation is calculated by first ranking the variables, then applying Pearson's correlation coefficient to these ranks. The formula used is a simplified version of Pearson's r, which is 1 minus the sum of the squared differences between the ranks, divided by the number of samples times the number of samples minus one.
What does a Spearman's rank correlation coefficient of 0.8 indicate?
-A Spearman's rank correlation coefficient of 0.8 indicates a strong positive correlation between the two variables, meaning that as one variable increases, the other variable also tends to increase.
How does Spearman's rank correlation handle outliers?
-Spearman's rank correlation is less sensitive to outliers because it uses the rank of the data points rather than the actual values. This means that extreme values do not disproportionately affect the correlation coefficient.
What is the difference between Spearman's rank correlation and Pearson's correlation coefficient?
-While both are measures of correlation, Pearson's correlation coefficient assumes a linear relationship between variables and is sensitive to outliers. Spearman's rank correlation, on the other hand, does not assume a linear relationship and is less sensitive to outliers, making it suitable for non-linear relationships and data with outliers.
Can Spearman's rank correlation be used when the variables are on different scales?
-Yes, Spearman's rank correlation can be used when variables are on different scales because it is based on the rank of the data rather than the actual values, making it scale-invariant.
What is the importance of visualizing data before choosing a correlation coefficient?
-Visualizing data helps to identify outliers, understand the nature of the relationship between variables, and determine if the variables are on the same scale. This information is crucial for selecting the appropriate correlation coefficient to accurately represent the relationship between the variables.
Why should correlation not be confused with causation?
-Correlation indicates a relationship between two variables but does not imply that one variable causes the other to change. Causation requires evidence of a direct effect, which cannot be determined solely by correlation coefficients.
How does the script demonstrate the sensitivity of Pearson's correlation coefficient to outliers?
-The script provides an example where two outlier values are introduced into a dataset. It then shows that the Pearson's correlation coefficient decreases significantly due to the presence of these outliers, while Spearman's rank correlation remains relatively stable, demonstrating its robustness to outliers.
Outlines
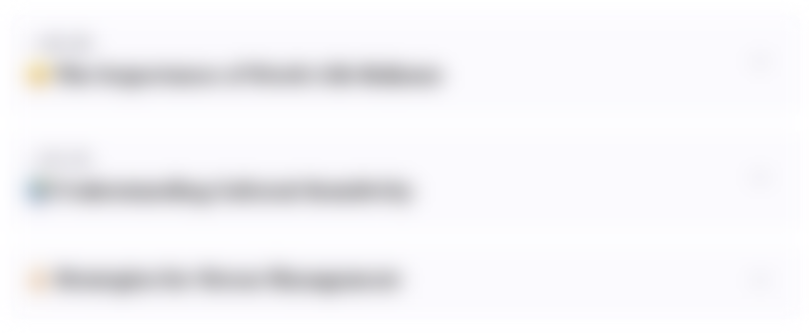
Cette section est réservée aux utilisateurs payants. Améliorez votre compte pour accéder à cette section.
Améliorer maintenantMindmap
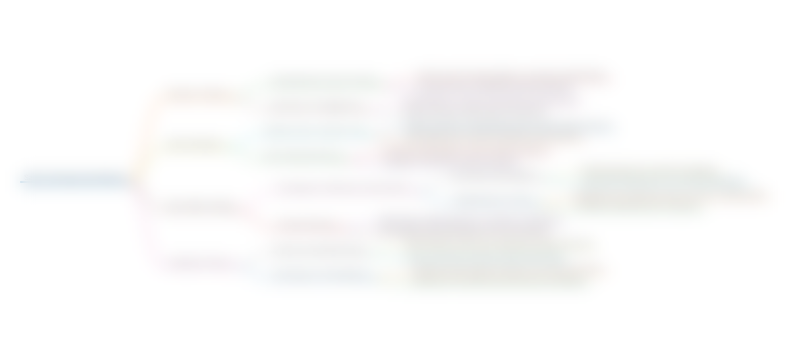
Cette section est réservée aux utilisateurs payants. Améliorez votre compte pour accéder à cette section.
Améliorer maintenantKeywords
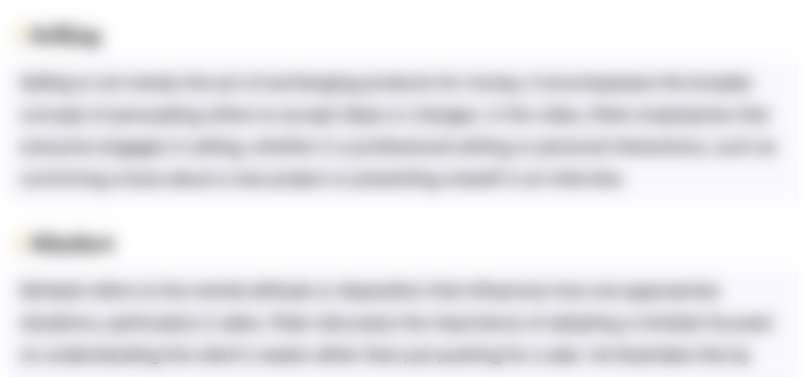
Cette section est réservée aux utilisateurs payants. Améliorez votre compte pour accéder à cette section.
Améliorer maintenantHighlights
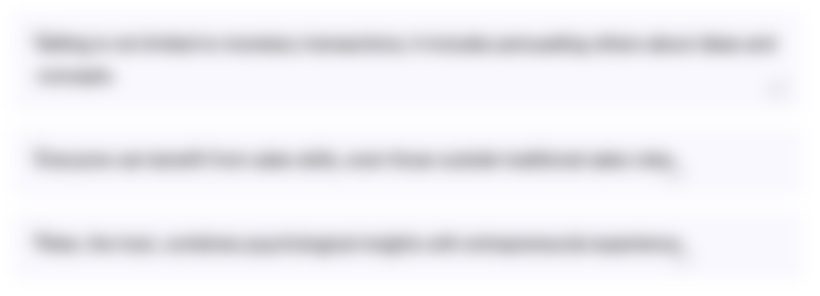
Cette section est réservée aux utilisateurs payants. Améliorez votre compte pour accéder à cette section.
Améliorer maintenantTranscripts
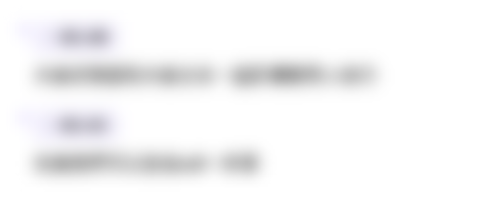
Cette section est réservée aux utilisateurs payants. Améliorez votre compte pour accéder à cette section.
Améliorer maintenantVoir Plus de Vidéos Connexes
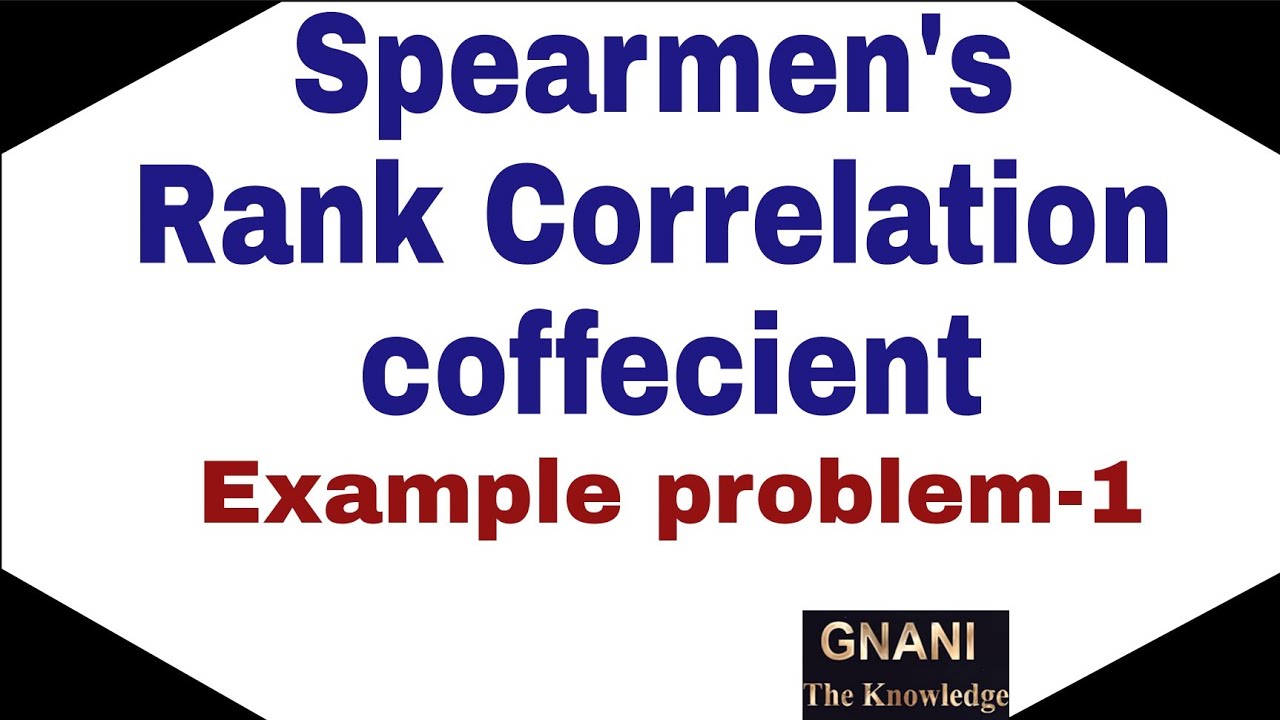
Spearmen's Rank Correlation || Gnani The Knowledge ||
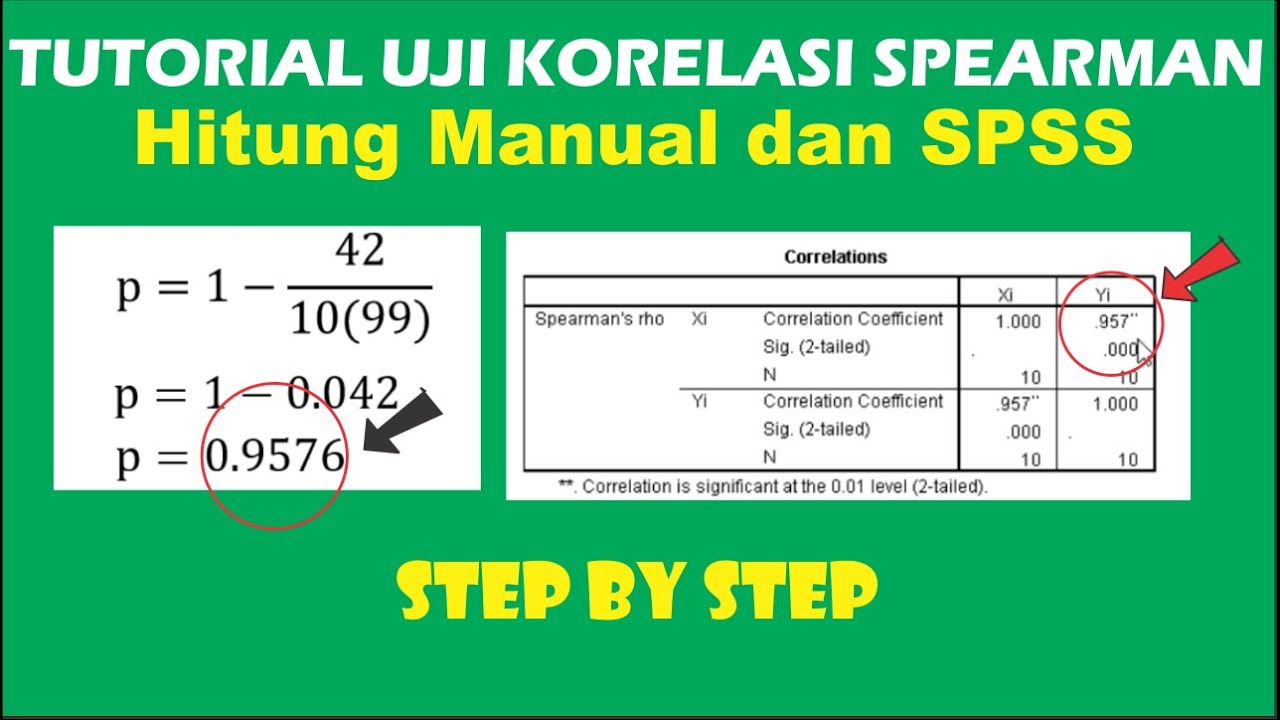
Tutorial atau Cara Uji Korelasi Spearman Hitung Manual dan SPSS
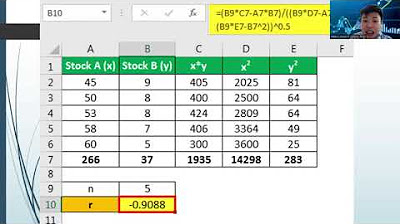
Statistics Lecture 5 Test of Relationship
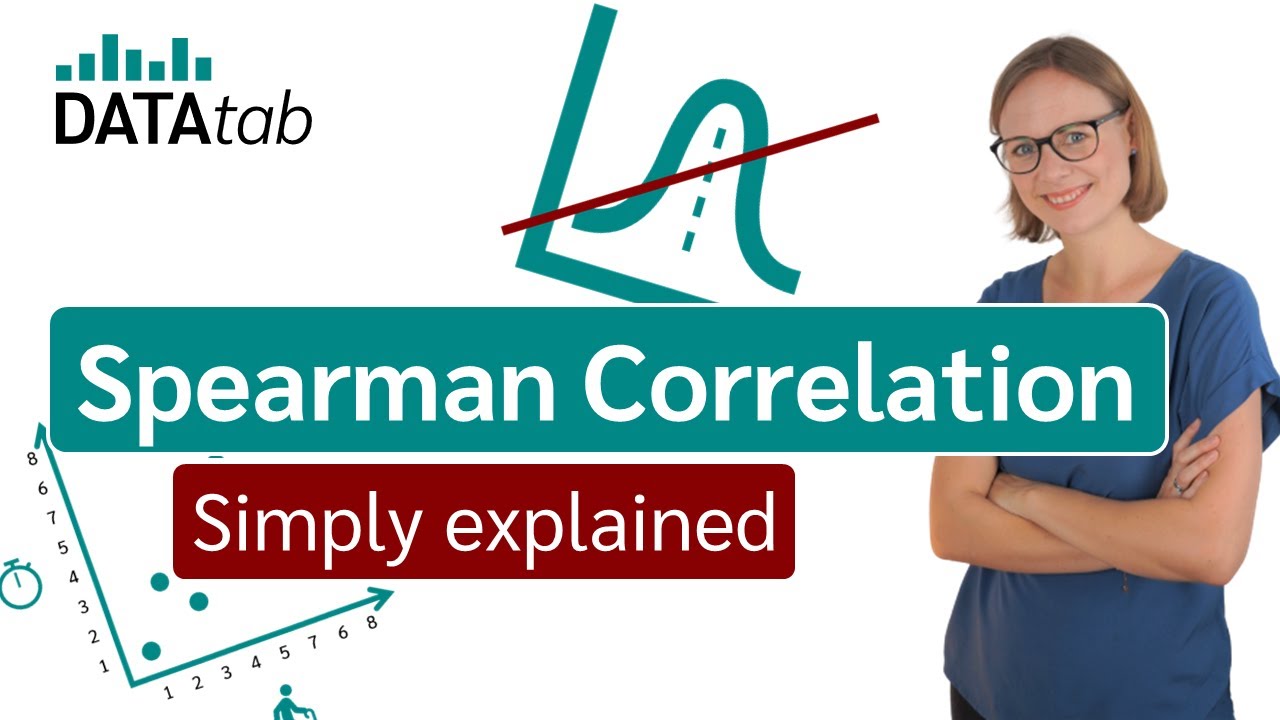
Spearman Rank Correlation [Simply explained]

A-Level Biology - Spearman's rank correlation coefficient
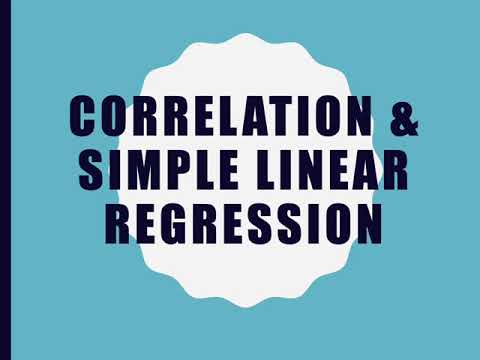
[Mathematics in the Modern World] Correlation & Simple Linear Regression
5.0 / 5 (0 votes)