Building a Plagiarism Detector Using Machine Learning | Plagiarism Detection with Python
Summary
TLDRThis video script outlines the development of a plagiarism detector using natural language processing. It covers understanding plagiarism, showcasing a user interface, and detailing the project's setup from scratch. Key aspects include utilizing libraries like NLTK and scikit-learn, data preprocessing, model training with classifiers, and evaluation metrics. The script also guides through deploying the model with Flask, creating a user interface, and ensuring the model's accuracy with tests, concluding with suggestions for further dataset expansion and development.
Takeaways
- đ The script outlines a project for creating a plagiarism detector using natural language processing (NLP) techniques.
- đ It defines plagiarism as the unauthorized use of someone else's work, ideas, or intellectual property without proper attribution or permission.
- đ ïž The project utilizes the NLTK library for NLP tasks and includes algorithms like Logistic Regression, Random Forest, and Naive Bayes for classification.
- đ The script demonstrates the use of TF-IDF vectorizer for feature extraction from textual data, which is crucial for any NLP project.
- đ It explains the preprocessing steps for text data, including the removal of punctuation, lowercasing, and elimination of stop words.
- đ The project involves training a model and evaluating it using metrics like accuracy score, precision, recall, F1 score, and confusion matrix.
- đ The script provides insights into handling data distribution and the importance of having a balanced dataset for training the model.
- đ» The tutorial covers deploying the model using a Flask web framework, creating a user interface for input, and displaying the detection results.
- đ§ The importance of matching the scikit-learn version used in training with the one in the deployment environment to avoid mismatch errors is highlighted.
- đ The script describes creating an HTML form for user input and using CSS for styling the web interface to make it more attractive.
- đ The final takeaway emphasizes the need for user support through likes, comments, and subscriptions for the channel, indicating the educational nature of the content.
Q & A
What is the main objective of the project described in the script?
-The main objective of the project is to create a plagiarism detector using natural language processing techniques.
What is plagiarism according to the script?
-Plagiarism is the act of using someone else's work, ideas, or intellectual property without proper attribution or permission.
What are the steps involved in creating the plagiarism detection model?
-The steps include understanding plagiarism, creating a user interface, importing necessary libraries, loading and preprocessing the dataset, feature extraction, model training, evaluation, and deployment.
Which libraries and tools are mentioned for natural language processing tasks?
-The libraries and tools mentioned include NLTK for natural language processing tasks, pandas for loading the dataset, and scikit-learn for machine learning classifiers and metrics.
What is the importance of removing stop words in an NLP project?
-Removing stop words is important because they are typically irrelevant to the meaning of the text and can reduce the effectiveness of text analysis or processing.
What is the role of TF-IDF vectorizer in the plagiarism detection project?
-The TF-IDF vectorizer is used for feature extraction, converting textual data into a numerical format that can be understood by machine learning models.
How is the model evaluated in the script?
-The model is evaluated using accuracy score, classification report for precision, recall, and F1 score, and confusion matrix to check for misclassifications.
What are the different machine learning classifiers used in the project?
-The classifiers used include Logistic Regression, Random Forest Classifier, Multinomial Naive Bayes, and Support Vector Classifier.
Why is it necessary to save the trained model and vectorizer?
-It is necessary to save the trained model and vectorizer to avoid retraining for new inputs and to facilitate easy deployment and integration into production systems.
What is the purpose of creating a user interface for the plagiarism detector?
-The purpose of creating a user interface is to allow users to input text and receive feedback on whether the text is plagiarized, making the model accessible and user-friendly.
How is the Flask framework used in the deployment of the plagiarism detector?
-The Flask framework is used to create a web application that receives user input, processes it through the plagiarism detection model, and displays the results on a webpage.
Outlines
đ Introduction to Plagiarism Detection Project
The speaker introduces a plagiarism detection project using natural language processing (NLP). They explain the concept of plagiarism and demonstrate the project's user interface, showing how it detects copied text. The speaker also outlines the project's technical requirements, including the NLTK library, machine learning classifiers, and evaluation metrics. They mention the need for feature extraction using TF-IDF vectorizer and the importance of cleaning text data.
đ Understanding the Dataset and Preprocessing
The speaker discusses the structure of the plagiarism dataset, which includes source text, plagiarized text, and labels indicating plagiarism status. They emphasize the need for data preprocessing, such as removing punctuation, converting text to lowercase, and eliminating stopwords, to prepare the data for the NLP model. The dataset is acknowledged to be small and potentially imperfect, and the speaker offers to share it for further enhancement.
đ€ Feature Extraction and Model Training
The speaker explains the process of converting text data into a numerical format using TF-IDF vectorization, which is essential for machine learning models. They describe the model training process, starting with logistic regression, and then moving on to random forest and other classifiers. The importance of model evaluation using accuracy scores, classification reports, and confusion matrices is highlighted.
đïž Model Selection and Deployment
The speaker compares the performance of different machine learning models, such as logistic regression, random forest, naive Bayes, and support vector machines, to select the best model for plagiarism detection. They discuss the process of saving the trained model and vectorizer using the pickle library for deployment, ensuring that the model can be reused without retraining.
đ ïž Setting Up the Flask Application
The speaker provides a step-by-step guide to setting up a Flask application for the plagiarism detection system. They explain the need for creating a virtual environment, installing Flask and the correct version of scikit-learn, and structuring the project files. The speaker also details the creation of HTML templates for the user interface and the initial setup of the Flask app.
đš Designing the User Interface
The speaker focuses on designing an attractive user interface for the plagiarism detector using HTML and CSS. They describe adding a title, form elements for text input, and a submit button. The speaker also discusses the importance of styling the page using CSS to make it visually appealing and user-friendly.
đ Integrating the Backend with the Frontend
The speaker explains how to integrate the backend logic with the frontend interface using Flask routes and templates. They demonstrate how to handle form submissions, process user input, perform predictions using the loaded model, and display the results on the webpage. The speaker also emphasizes the importance of matching the scikit-learn version between the development and production environments to avoid errors.
đ Finalizing the Plagiarism Detection System
The speaker wraps up the project by discussing the final steps, including creating a function to handle user input, vectorize the text, and return the plagiarism detection result. They also mention the need to test the system with various texts to ensure its accuracy and reliability. The speaker encourages viewers to support the channel through likes, comments, and subscriptions.
Mindmap
Keywords
đĄPlagiarism
đĄNatural Language Processing (NLP)
đĄUser Interface
đĄMachine Learning Classifiers
đĄTF-IDF Vectorizer
đĄFeature Extraction
đĄBinarized Classification
đĄConfusion Matrix
đĄAccuracy Score
đĄFlask Framework
đĄPickle Library
Highlights
Introduction to a plagiarism detector project using natural language processing.
Explanation of what constitutes plagiarism in the context of using someone else's work without proper attribution.
Demonstration of a user interface for inputting text to be checked for plagiarism.
Showcasing the output of the plagiarism detector model indicating 'plagiarism detected'.
Use of the TF-ID vectorizer for feature extraction in NLP tasks.
Importance of cleaning textual data by removing punctuation, converting to lowercase, and eliminating stop words.
Utilization of machine learning classifiers such as Logistic Regression, Random Forest, and Naive Bayes for the plagiarism detection model.
Discussion on the importance of model evaluation using accuracy score, classification report, and confusion matrix.
The process of training the plagiarism detection model using a dataset with labeled examples.
Addressing the need for a larger dataset for a more detailed plagiarism system.
Instructions on how to preprocess text data for NLP projects, including custom Python functions.
Conversion of textual data into numerical format for machine learning models using TF-IDF vectorization.
Splitting the dataset into training and testing sets for model training and evaluation.
Comparison of different machine learning models' performance in detecting plagiarism.
Final selection of the best-performing model for deployment in a plagiarism detection system.
Explanation of how to save and load a trained model and vectorizer using the pickle library.
Development of a function to detect plagiarism in user-provided text for deployment.
Instructions for setting up a Flask application for the web interface of the plagiarism detector.
Importance of matching the scikit-learn version between the training environment and the production environment.
Creation of a simple user interface using HTML and CSS for the plagiarism detector application.
Integration of the backend logic with the frontend interface to create a complete web application.
Final demonstration of the plagiarism detector web application in action.
Call to action for viewers to like, comment, and subscribe for support of the channel.
Transcripts
so we have another natural language
processing project plagerism uh detector
so first we need to understand what is
plagarism then I'll show couple of
outputs because we have also created
this user
interface plagarism is some kind of
actor uh in which you use someone else
work or someone else ideas or
intellectual property without proper
attribution or
permission so we have this user input
okay researchers have discovered a new
species of butterfly in the
rainforest now definitely I have copied
this from someone else article so yes
this could be a plagarism now let's see
what the model say so the moment I click
on this button it will give me the
output plagarism
detected uh let me try one uh more input
I have another input uh like practicing
yoga enhances physical
flexibility so clearly you can see that
that it could not be a plagarized text
because it could be someone else uh IDE
creativity so let's see uh no plagarism
detected so yeah this is the model now
let's start the project uh from scratch
soly so let's do that quickly first we
need ntk Library which is used for uh
natural language processing task and
within nltk we have couple of algorithms
pre-trend uh nltk do download you need
to download it directly in your jet
notebook Which is
popular and after that you need to
import uh pandas for loading the data
set
SPD uh you also need to import string uh
module in Python it will help us in
cleaning the text because we are dealing
with textual data and it's a NLP Pro
reor uh and from nltk carpus we need to
import Stop wordss uh because we have to
remove stop wordss irrelevant words so
from nltk do
carpus it should suggest me but it's not
suggesting me uh you need to
import toop
words again it's not suggesting me let
me type it
manually uh once you do do that you also
need to load the first basically for
this project we will use five machine
learning
classifier listic regression random
Forest
classifier uh let me quickly first
import from sk. linear
model logistic
[Music]
regation so we need to import
from scale learn do model selection you
have to import RPL to class it will help
us in splitting the data set into
different sets like testing and
training now once you train the model
you need to evaluate that model by using
couple of uh matrices so you need to
import from SK L do matrices you need to
import first we need to import accuracy
score it will help us calculate the
accuracy and then we need a
classification report it will help
us uh calculating uh Precision recall F1
score for each class so we have two
class plagarized and not plagarized so
basically it's a biner classification
problem and we also need confusion MX so
that we check how many mistakes our our
model is doing for each class or how
many correctly classify the
classes and the fin final thing which is
very important for any NLP project that
is feature extraction so for feature
extraction we use different tools but we
will use TF vectorizer is I mostly
recommend you for any NLP project so
from
skan do feature extraction clause. text
you need to import TFI
vectorizer okay I'll explain all the
stuffs a bit later but yes this these
are the Imports okay so let's run it uh
there's in some talkx issue
uh from nltk do Corpus inut
Stoppers just uh basically there should
be from okay so let me uh
rerun so yeah now we need to import the
data set so I'll give it a name data
then I'll use pendas Library read CSV
function and I'll pass my data and let
me show you the first few
cells so this is the data set now you
need to understand the structure and
format of this data set so you can
clearly see in this data set we have
three columns Source text plagerized
text and we have label so Source text
and plagerized text contains the
document uh of textual data so these are
our input features and label contain two
classes 0 and one here one
represent plagarized text and zero
represent not plagarized
text so let me quickly show you the
distribution of label
feature distribution check you need to
use this
function counts value counts so you can
clearly see we have equal distribution
so we don't need to balance this data
set one thing more which is important
for you and for everyone to use this
project this data set is created by me
so there might be mistakes and this is a
very uh lower data set because let me
show you the shap it's not a big data uh
it's I
think 370 records only so if you want to
use a very detailed plagerism system you
need to increase this data set you can
further work on this data set I'll share
with you the data and the entire
notebook code and the
development so the next thing that is
very important in any any NLP project
natural language
processing now in this textu data you
need to remove couple of things so let's
do that one by one for that you can use
uh different uh techniques our tools but
let's do it with our custom python
function so let me give it a name by the
name of pre
preprocessor
pre-process text this function will text
will take a text so here let me pass a
parameter by the name of text now first
we will
remove uh punctuation because
punctuation are the characters or terms
that we don't need for any model so we
will remove from each text all the
punctuation so you have string
Library so on the text but first let me
call this uh function pre process text
and let me pass my text this is my let
me add some punctuation or special
character something like
this uh like this
my text to use for dummy
test now the first thing I would like to
do is to
remove uh the punctuation so text will
be equal to text. translate function
here you can use translet
function and first we have to call St
strr parameter in this in this translate
function and we have to make translation
so here you have to call on this St Str
another function now you don't need to
worry about all the stuffs you don't
need to remember all this stuff but yeah
this is the
flow and now see first what we have done
on the text that this function is
getting function
okay you see this so for now just let me
return this text and let's see you can
clearly see we remove this punctuation
marks from the
text Second Step let's lower the text as
you can see here in this text we have uh
capital capital so the next
step uh we have
to
convert to lower case so lower casing is
very simple you can just call lower
function on text now you can see here t
is
smaller and the third and final step
which is again important removing stop
words you can skip this part you can
also skip this part but you are not able
to skip this third step for any NLP
project because in English language we
have a lot of stop words in a sentence
stop words like is from my this like
noun sorry pronouns and uh uh
preposition in English grammar so
preposition uh pronouns all these words
are stop words you need to remove them
so remove stop words now for stop words
removing we already imported from nltk
carpus stop wordss
class so you can call this stop wordss
and there are you can call words and
there you can specify the language
because internally it's used I think
five or six language like German French
language uh English but I'm I'm dealing
with English language so I'll pass here
English and I need a unique stop words
so you can call on this set function in
Python it will get all the unique stop
words like E
from and I'll store that in a variable
by the name of Stoppers because I'll use
this variable on my data to remove all
the Stu from each text so it's very
clear now what you can do you you can
use a list
parameter uh or something like you can
use Loops but I mostly use
Loop in a single line so first I'll
extract
word uh from this text but we need to
toonize this text
so for that you can use split function
okay now I will check that if the
word is not
located in the starware that I have
defined so basically when this text is
passed here it will remove all the
punctuation then lower casing here I'm
defining my stop words and here what I'm
doing I'm running a loop on this text
basically on this first I'm splitting it
uh like this on comma reconizing it
okay now it will take one by one first
it will take this and here I am checking
that if this word I mean this is located
in Stoppers and this is a stopper so it
is located and it will skip it it will
not pass here then it will take this
this is stopper then this then this text
now text is not a stopper so it will
check that if this text is not in stop
it will pass to this word and I'll store
it uh again in a text but it will return
a list so I don't want list I want a
string so I'll join it this entire by a
string something like this so let me run
it now you can see we have only
important words like text use dummy text
okay all the stop words has been removed
okay now let's apply this function for
our data set so let me add here a cell I
have two input columns Source text and
plagerized text so
data source text and I'll call here a
apply function and I'll simply pass my
custom python
preprocess
preprocess why it's not
working okay it should be
pre-processed let me run it
again and yeah pre-process
text so I will store the clean text
again in the same column our input
feature so let me copy paste the
same for plagerized
text and let me run it now if I show the
data
set this is the data set now here you
can clearly see uh this is the first
record on zero index researchers
discover new
species and plagarized text scientists
found previously unknown but if I check
the original
text researchers have disc discover word
now here you can see we have a stop word
a a stop word right but after cleaning
that data and removing all the stoppers
you can clearly see we don't see that
stops like the
stoppers researchers previously have but
here you can see we don't have have
researchers discovered new so now we
have important words now once now we
know that these two features are input
feature so we have to can can add both
these two features and label is our
Target
column we have to convert this textual
data into numerical format because
machine Any machine learning model can
only understand numerical data not texal
data so here we have to extract the
features and also we have to convert the
textual data into numerical format for
that as I already mentioned we'll
use TF ID vectorizer so I'll create an
object of this TF F
IDF vectorizer something like that then
I'll call tfid vectorizer I I already
important now what I can do I can simply
convert all my text to
numerical uh format by calling this
function fit
transform now here I have to pass both
features so first I can do data source
text
and here I will
concatenate by space with data my
plagerized
text okay
and yeah this is the code in single line
we have converted and I'll store the
result in
X so basically now we have converted all
the textual data into numerical format
by using tfid vectorizer now let's
create y our Target and we know that
it's a
label now we have input feature and we
have Target feature we only need to do
Trent
split so for Trent split we already have
a function by the name of but first let
me create four variable
extr X test y TR
y test and I'll use here Trend test BL
function and I'll pass my input feature
and Target feature now here we have to
specify the test size I want uh to store
only 20% data for test and remaining 80%
data for training
sets and I want shuffling so here you
can pause random stat mostly I use 42
you can use any number it doesn't matter
now you have also TR test plate now
let's train our first model logistic
regression so logistic regession we
already uh imported I think so we need
to do just create an object for logistic
regation like this now we have to turn
logistic regation by using fit method
or TR set and test
set sorry trans set from input feature
as well as corresponding Target
feature now let's test the model but for
that we need to do some prediction for
test data these things are very basic
Basics you have learned a lot in my
projects uh because I've done a lot of
projects in this channel so I'm not
going to repeat all the stuff again
again but yes here we are turning the
model and here we are doing some
prediction or
testing so you can call predict method
here and you can pause your input
feature test
data uh now let's calculate accuracy so
accuracy of the model will
be we we already important accuracy
method here you can pass two
things your y
test and so here basically we are going
comparon
confusion
classification
information I have a very beautiful
playlist and very knowledgeable playlist
Cy learn tutorial you can watch that you
will understand a lot about all the
stuffs that we use in any machine
learning project using Psy lar and
finally I'll calculate confusion Matrix
so this is confusion Matrix and let me
run
now the model is giving attitude
accuracy which is good honestly which is
good class this is the classification
report now let's let me give you a quick
overview otherwise I have explained a
lot of detail about this stuff the
mathematics behind each method like
Precision recall F1 score in my Cy L
playlist you can view it in my this
channel artificial intelligence so we
have two claes zero not plagarized one
plagarized for zero the Precision is 79
which is good 86 is the recall uh 87 is
the F score so for now just the the
moment you get higher values higher
Precision recall and F1 score it's mean
for this class your model is doing quite
amazing and the same thing goes with
another class if you have higher values
like 90 or like this one 86 it mean your
model is doing great job this is the
average accuracy and you can also
calculate average
Precision add3 for both
classes and the same thing goes for
recall F1 score
now uh confusion Matrix is very
important let me zoom out a bit so you
can clearly
see the
output uh let me
rerun I think I have to zoom out
again now let me rerun again again it's
again giving but yeah it's okay let me
zoom in I just wanted to show you a
proper output so that you can understand
confusion Matrix now in confusion Matrix
we have 2X two D array because we have
two classes so this is uh actual this is
the correct class uh correct prediction
for one class let's say here we have a
zero and this is the correct classes for
another class let's say we have a one
but diagonally up and diagonally down
like five is the mistakes of our model
and at here is the mistakes for another
class for for our model I can't explain
clearly here if I plot it then you will
better understand but I'm not going to
do that because I've already explained
the mathematics behind this the
theoretical part but yeah this is the
general overview if you want to just
understand you can uh view the diagonal
part if the diagonal part has larger
value it's mean your model is doing job
but diagonal up and diagonal down if you
see some zeros again if let's say here
is a zero and here is a zero it mean
that your model didn't misclassified any
class so it mean that your model didn't
perform any mistakes but here you can
see we have at so here model done some
kind of mistakes and here again five
okay now let's let me copy paste this
code because now we are applying the
same method for random Forest
so let's apply random forest for random
Forest I'll use from scal
learn uh you can uh emble method esal
Lan
do
emble you can
import random forest
classifier and now let me past the same
code here I'll simply
repeat modify random Forest
classifier now one thing
random for take couple of parameter like
an estimator so you have to pause that
estimator I want
100 and it's also take random State
again I'm going to give it 42 now other
method is same as it is can just run the
sell So Random forest model accuracy
is uh
79 uh yeah it's good I think again this
model is performing a little bit better
but logistic was better than this model
let's try Nave
Bas because whenever you deal uh in
classification problem and you are using
machine learning model then there you
have two models support Vector machine
and Nave base for textual data so they
will perform better in comparison to the
other model because they espcially met
for textual data okay so let let me
paste the same code and we need to
import NV Bas so importing NV
Bas you you need again Cy L and you have
to import it from
Nev Base
Class
multinomial
andb okay multinomial Nave
base you might be thinking why I'm not
going to explain this model so I already
explained you can explore in this
channel but this model is performing
better than the previous two
model uh where is you can see the
accuracy is 86 and precision recall F1
score for both classes are amazing so
this model is good but let's try support
Vector machine so you have to import it
from
sk.
svm you have to
import
SVC meaning support vector
classifier and
then oops let
me copy the previous
code this part
only contrl
C
and let me pass to
here this should be SVC now SVC has two
types linear and nonlinear and we are
dealing with uh linearity because we are
drawing a linear line between two
classes zero and one PL eyes not
plagerized so here you have to pause uh
that
parameter uh because it's used
internally two parameters and sorry two
types of uh linear support Vector
classifiers so here you have to specify
kernel and since we are dealing with
linearity so I'll use linear and again
you have to pause random stat 42 let me
run it so this model is performing
better than all the previous model
because we are achieving
at7 so finally we have to select this
model for deployment for production so
let's save this model saving
model is very important because I'm not
going to uh train again and again all
the previous code we have to save this
model and the vectorizer
uh for production for new input so for
that you use pickle library and in
pickle you use dump method and here you
have to pass two two things first the
model you are going to save second open
parameter where you have to give again
two things name it the model we have
model and here you have to give the
extension PK and binary mode so we are
going to save you here you have to use
WB W WB means right binary mode and you
have to do the same thing for vectorizer
you might be thinking why we need
vectorizer because user will not put
numerical data user will put as I
already shown you in the introductory
part of this video in website in the
user interface where user was putting
some kind of textual data so there we
also need the train vectorizer I mean
the tfid
vectorizer and you also need to save
this one by this name now I'm not going
to run this because previously I already
save this too here you can see model. PK
and tfid Dev vectorizer do pkl now we
need to load this two why we need to
load these two things I mean the model
and TF vectorizer that we have
saved we need to use them so for saving
you can use this code again pickle but
this time instead of dump you have to
use load method and here you have to
pass open specify the name of the model
and this time you have to give RB read
binary mode because this time we are
reading not saving and the same thing
goes for T So finally I have a model and
tfid vectorizer which are
loaded uh I think I didn't run the
previous cell so let me copy this pickle
and let me past it here so now we have
loaded model now the final thing in this
jupter notebook is to create a
function or detection system then we
will work on the deployment part so I
will create here a function uh that will
Tech user input user input
text in this function I'll do two things
first I'll
vectorizer the
text
then will we'll do
prediction by model and then a return
final
output so this is the user input
researchers have discovered a new
species of butterfly in Amazon ran
forest and I have called the function
detect and I have passed input text so
this function will get this input text
here so let's vectorize it vectorize it
so we already loaded Trend TFI
vectorizer and here this time we don't
need to call F transform we are not
going to tr we are just doing some
transformation so here you have to use
transform method and you have to pass in
list keep in mind here you have to pass
in
list user input text right and finally
we will have
this
vectorized text now let's do
prediction and and store the result in
result variable so model. predict method
and here just pass the vectorized text I
hope you get the flow and logic of this
function and finally return result but
it will be it will return either zero or
one as per the prediction but I will use
here a logic the logic would be again in
single line so I will write here
plure plagerism
[Music]
detected when if the
result is equal equal to
one when the model predict for this text
this vectorized text it will give you
either zero or one at a time so here if
the result you might you don't confuse
with this zero this zero is just for
removing the list because the zero or
one that's uh that's return returned by
this model will will be in a list form
so I'm removing them from list so if it
is equal to one then the model will
return that plagerism
detected
else so if it is not one so it mean that
it is zero and for zero we have
no plure Rison I hope you get the idea
let me run
this uh inv valid syntox
[Music]
okay we don't need to here colum and now
let me run this so you can see plagarism
detected because it was a plagarized
text I have another text as I already
shown I think I didn't shown you it has
no plagiarism playing musical
instruments enhances creativity you can
clearly see any anyone can write this
thing can use uh his or her own idea a
text so let's see again I have passed
this input text to the function no pler
rism this is practicing yoga I have
already shown and again here we we do
not have any
plagerism so that was the Jitter
notebook code I hope you get everything
from scratch now we need to work on the
simple user interface using floss
framework in Python and the background
code
but always for any project some students
say that uh we are facing some
unmatching issues in py charm in the
deployment
part
model so you need to install the same
version of cyut learn in p environment
where you use in the jet notebook
because you train the model and TFI
vectorizer with this version so you have
to exactly install that version here now
so we have open pyam now here are few
things uh important for all the student
that mostly get errors related to
installation or something first you need
to create virtual environment and I hope
you have learned how to create virtu
environment while open pyam or any ID
like vs code for python now in
this in this folder I have this folder
in this folder you must have model. pkl
file that we already save there in
jupyter Notebook and tfid vectorizer PK
you must have these two files you don't
need to this Jupiter notebook or data
set you can keep it no worries but you
can remove it but these two files are
must then you have to create app.py file
which is empty you can see it is empty
in this we will create the flask
framework code backend code now flask or
Jango need templates for user interface
for HTML files so here you have to
create templates directory or folder
with the same spelling without any
mistake
templates some student just create
template but you have to add plural as
well ES
and within this template you need to
create index.html currently it's empty
so first we will create the user
interface now this is the basic andn
talkx of any HTML file this is the ti
title plagerism detector now in body for
now I'll just use H1 tag and here I will
say I think you are just watching this
video and not liking and
subscribing this
channel
yet we will print this thing on our
website so let's do that from back end
using flask
framework now again you need to go to
your terminal okay because you need to
install couple of things now here you
can see I'm here and my environment is
already created here
and here you have to PP install flask
you need to install flask I already
installed so I'm not going to install
just enter and it will be installed
after that you need to install cyut
learn equal equal to the version you
have used in jupter Notebook while
training the model so I have used
1.3.2 version so you you use the same
version here as well and once you do
that you will not get any mismatch error
now first we need to import couple of
things from flask uh
framework first you need to import
capital F whilea flask which will help
us to create an object then you need to
import render template which will help
us to communicate with user interface
HTML files or redirect it will work
something like communication okay I'll
show a bit
later and also request because we we
have to request to the form for for
taking the user
input now here you have to
create uh app object now this is
built-in syntax you you will not be able
to change it now you have to call this
app in Python main function and if you
are a python programmer you might have
seen if name equal equal to under uncore
man this is the python man and here you
have to run the app by specifying
debugger will be so this is the basics
and tax you will never be able to change
it now between app and between Python
man you have to implement all the logic
The
Roots getting data from user interface
and passing data to the user interface
to be displayed so between this part we
will do the logic first we need to load
the two
things uh I have to import
uh pickle Library as well now first I
will load with the help of pickle load
function I'll pass open and here the
model with read binary mode okay let me
check the recording
quickly and I will do the same for
vectorizer so let me replicate this line
with the by pressing control and
d and here vectorizer
PK let me copy this and give me a proper
name so I have successfully imported
sorry loaded model and tfid
vectorizer and
viewers English speakers that's why I'm
use combination both okay so we have
load loaded this to uh model and tfid
vectorizer now let's communicate with
the index.html file I mean the user
interface so for that you use
root okay and in root the first root
I'll just pass empty because by default
I want to open directly the index.html
file when I run this app so here you
have to give a function by any name and
with the help of
return and render template you can go to
that file HTML file as I already
mentioned that render template is used
for communication between back end and
front
end so let me right click and run app
now in console you will get a
URL just click on this URL and your app
will be pop up in your Chrome browser
you can clearly see it's I zoomed it a
lot so let me zoom out I I think you are
just watching this video and liking and
subscribing this channel yet I should
write here let me change it basically
this message for all the user who are
watching this video I think you are just
watching this video and not liking and
subscribing this channel so yeah in this
way uh you create user interface and
communication but we will not displaying
this thing
what we can do I will here use container
so D I will give a class container cuz I
will also apply my CSS for making it
attractive and appealing and within this
container I will use H1 tag by title
pledge
rism detector so if I go to my page and
reload
it now we have
plagerism
detector after
that I will use a
form uh action will be detect this part
again important for web development I'll
explain a bit later and Method will be
post and within the
form I will pass text area where user
will
input text but this text area TT couple
of things things
like
name name will be text again this part
important but I will explain a bit later
plus holder plus holder when you want to
show something before so that user can
understand it enter text
here dot dot
dot
and one last attribute that is
required required mean
important
so in form we have passed two things
action and method in action we have
passed URL or path detect because with
the help of this action in back end when
we will implement the code for back end
you will get and understand this thing
so here
specifying action just for getting the
form there in backround and we have two
methods get and post post for sending
and receiving data between back end and
front end so here we are passing post
and this is the just getting input for
user so let me refresh now this is
the uh our text
area here we pass name text because when
user input here text I will get the text
in back end I mean in app.py with the
help of this
name now within the same form you need
to pass a
button so button I would like to pass
the type will be
submit
and title will be check
for Ledger rism let me quickly show
you so we have this button but this page
is not designed this page is not
appealing this page is not attractive we
have to make it attractive and appealing
now for that either you can you can use
bootstrap and bootstrap classes or you
can use your you your own
CSS like inline CSS you can call it now
on this container and this entire body
I'll do couple of
styling so here in
style you can do that by just calling
the classes so I have done this CSS all
ready let me quickly explain it within
the style on on the entire body of my
page I have uh kept this properties and
attributes okay
now like background color set display
will be Flex a lot of properties and
their corresponding values and we have
done some kind of CSS for container I
want the WID width 50% background will
be this padding so these are some
attributes and also added box Shadow now
uh just let me quickly
reload now we have something like
attractive
okay so you can clearly see these are
the Box Shad now we have to work on this
text area and this button
okay so I have
already uh done the CSS just let me past
it here so this is the CSS for the
button and text area and
result this is the button and this is
the text area you can clearly see uh I
didn't implemented result yet but we
will see it a bit later so just let me
refresh so yeah this is the form now
when we click on this check for
plagerism there we will display the
result so but for now we need to get
this
text uh in f.p so in f.p I will create
another
root
so f.
root and here we have detect in form and
here you have to give methods will be
equal to either get or just post let's
say and here you have to define a
function let's say
predict and within this prediction
function we will do
something or we can give it a proper
name to this function like
uh
detect
uh
plagerism
PLM
okay now the first thing
uh we have to
do uh what should I first do first we
have to get the data from the form so
it's very simple we have a request
method from floss and here you can call
form and here you can pause the name of
that input field so that was text so it
will get the text and we will we will
store it input
text got it
and
secondly now we have to do we have to
apply the two
steps
like uh vectorized tfid vectorized
dot
transform this
input
text and I will give it a name
vectorized
text and finally let's do
prediction so we have a model.
predict this
veiz
text and
finally
uh we we will update this result with
this
message uh like
like
plagerism
detected
if
result equal equal to one else no
[Music]
plagerism right and finally we will send
this
result to our index.html to be displayed
there so you can use render
template and go to
index.html and there result will be
equal to result now let me explain the
flow so in this function I mean in this
root first we are getting the root the
form then we are creating this function
and within this function first we are
getting uh from this
text where user input the text we are
getting that text and storing in input
text then we are doing vectorization
ition prediction creating the message as
per the result either zero or one and
then we are passing that method so
either we will this or we will this in
this result finally so finally we have
to print this result on
index.html so outside the form outside
the form uh I will use this template
when you implement python code in HTML
then you can use this template so if
result first I'll check
many get that we have geted we have get
the result or not then for now I'll just
print with this syntax result again the
syntax is built in you cannot
change and finally I will end the if so
this is the syntax sometime we call it
Ginger template and this is the printing
like in Python we do print but if you
print variable in index using python or
flos or Jango sorry not Jango Jango
there is a difference between Jango and
ginger template so yeah this is the
template uh now I have to run this again
because I have made a lot of
changes so just click on the
URL
and hello friend for now this is
something no please lism so it's
working
and uh let's make this output a little
bit attractive so I can go to
index.html and I'll use this
code because I've already result class
where I've done some CSS you can see it
like padding margin top now let's
[Applause]
refresh
here and let me use this text I mean
this
one so the model is working uh quite
amazingly because it has great result so
no plagarism
uh let
me let me try from my data uh what was
the name this was the data let me just
use Source
text uh let's go to Source text and
let's
use
uh this one on this index I first I'll
get this
text now
volunteering Fosters Community spirit I
don't know what it
saying let's see but what the model
say uh no
plagerism let's try one more let me take
a random text on 50 index I don't know
what honey bees communicate series Don M
I think this could be or not but let's
see what the models
in uh this is plagarized
uh let me try one
more on N
index Sahara Desert largest H desert
world now let's see what the model say
plagerism detected because this could be
someone else idea someone else hardw
working someone else article text so
yeah this is the project and
finally one thing uh your support in the
form of likes comments and subscription
to this channel would be really
appreciated so so thank you so much for
watching this video I have shared this
entire code with you this is the Jupiter
notebook code f.p code data set
index.html model. p and tfid vectorizer
PK if you want to use model. pkl tfid
vectorizer I mean my one then in Pam you
have to create virtual environment and
there you have to install the same
version of Cy L like
1.3.2 so thank you so much for watching
this video and we will see uh in
upcoming another amazing NLP project
thank you and finally again subscribe to
this Channel and like this video
Voir Plus de Vidéos Connexes
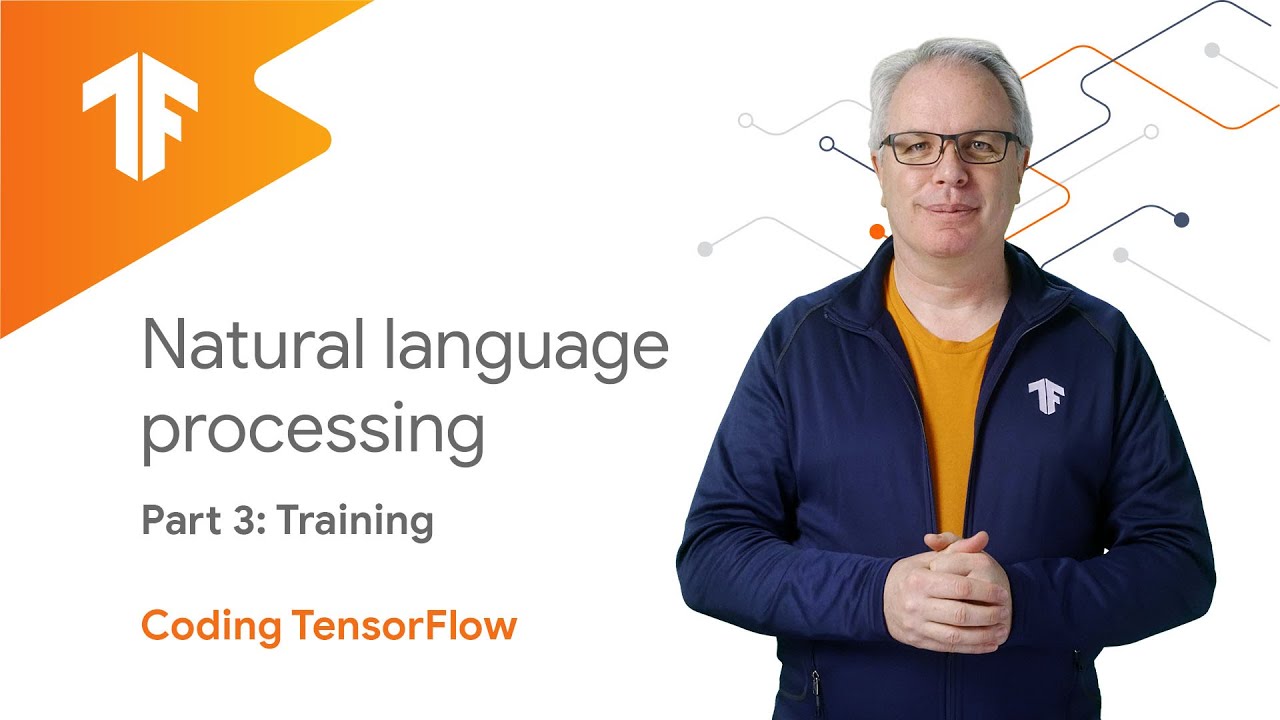
Training a model to recognize sentiment in text (NLP Zero to Hero - Part 3)
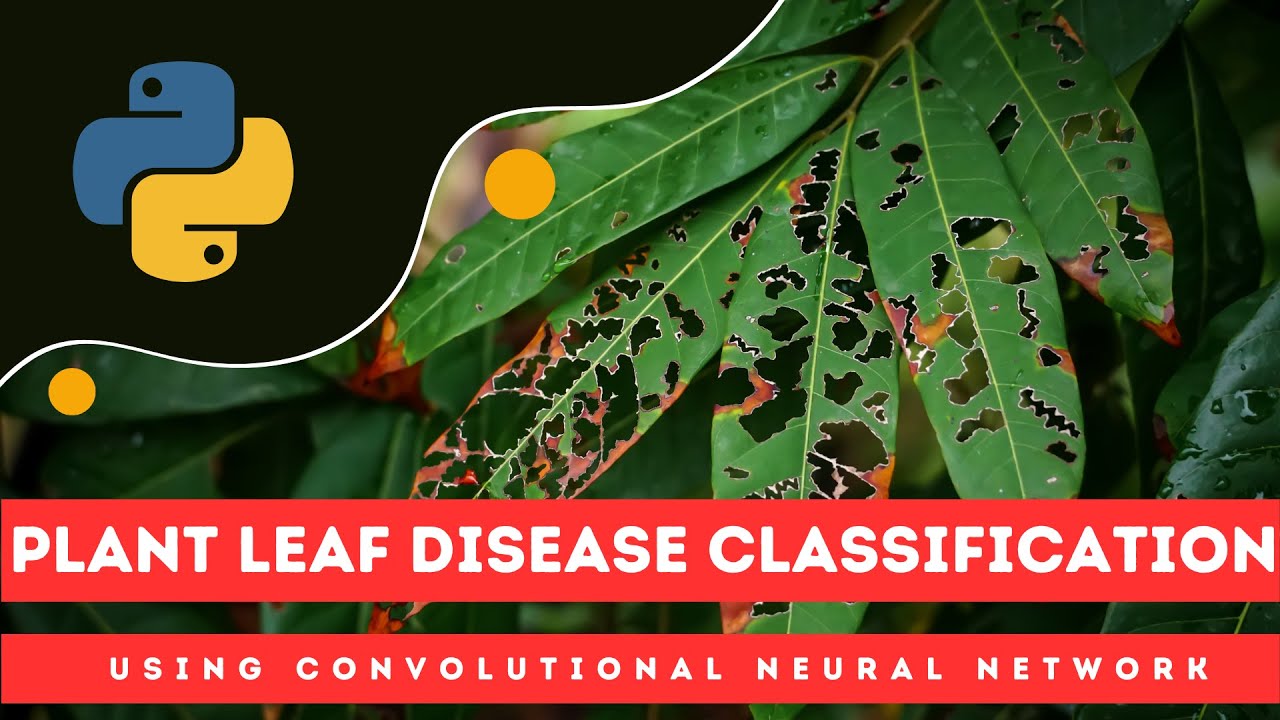
Plant Leaf Disease Detection Using CNN | Python
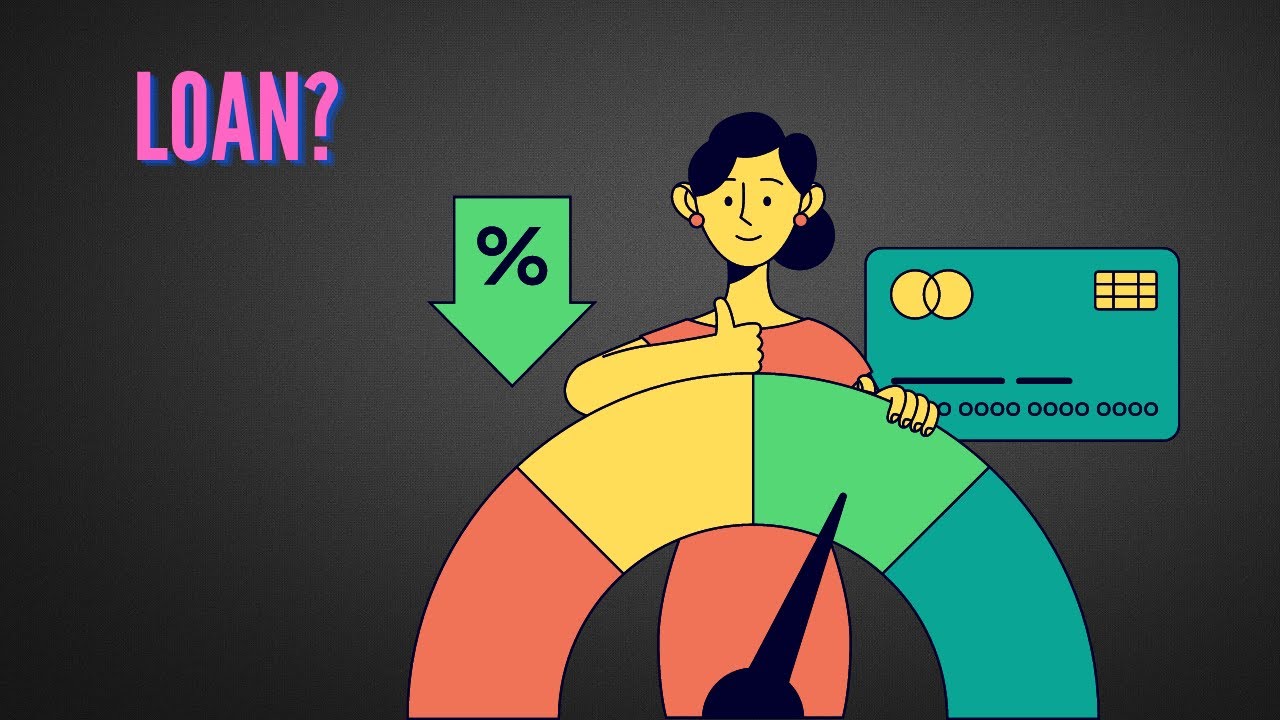
3.1. Credit Scoring | DATA SCIENCE PROJECT
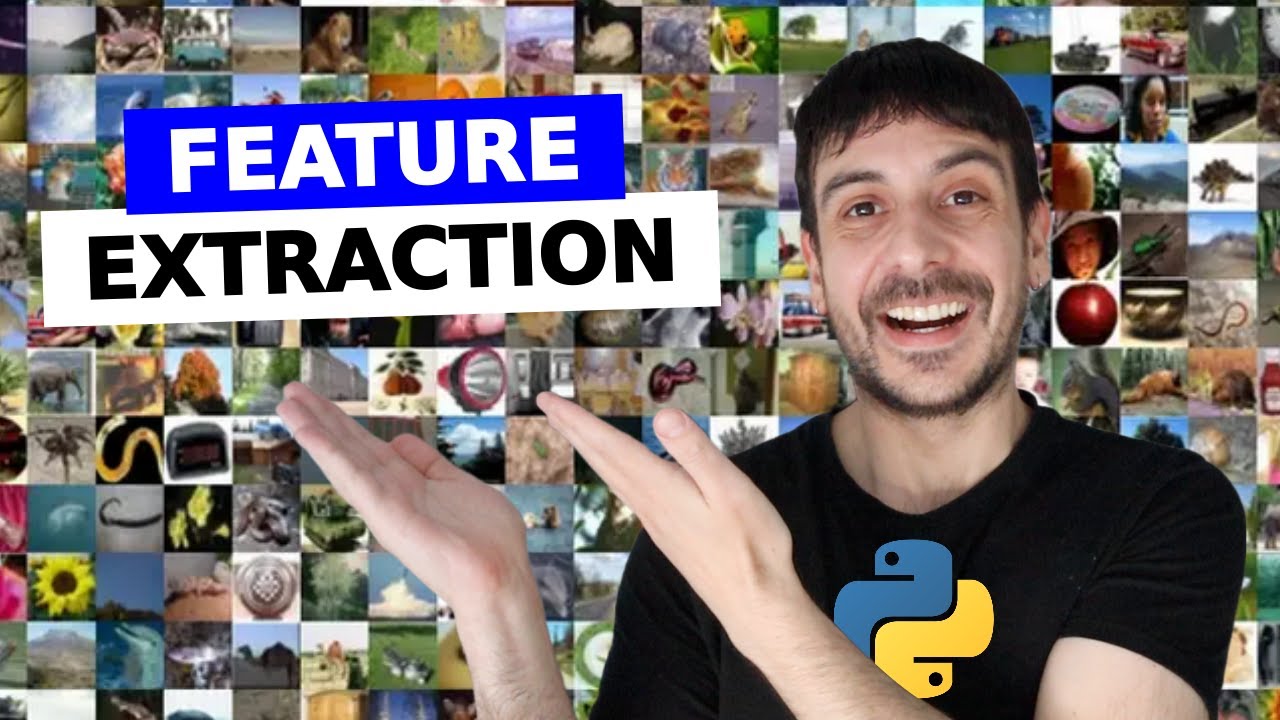
Image classification + feature extraction with Python and Scikit learn | Computer vision tutorial
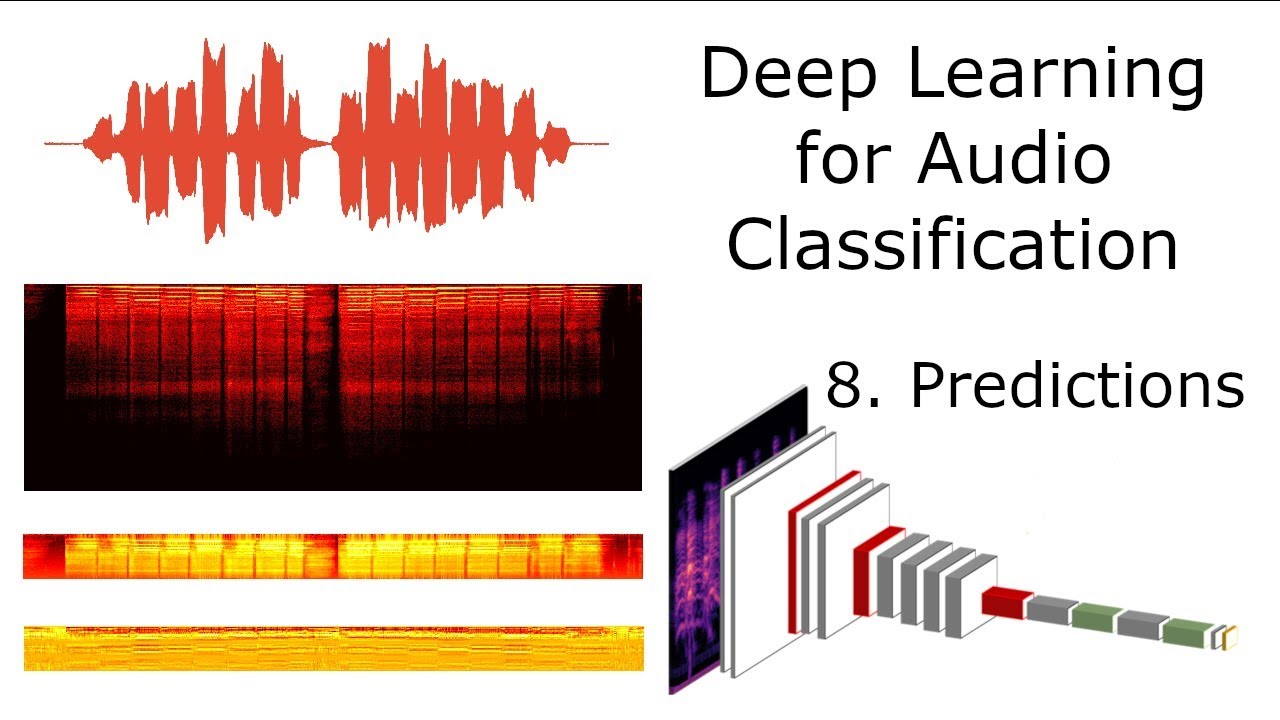
Predictions - Deep Learning for Audio Classification p.8
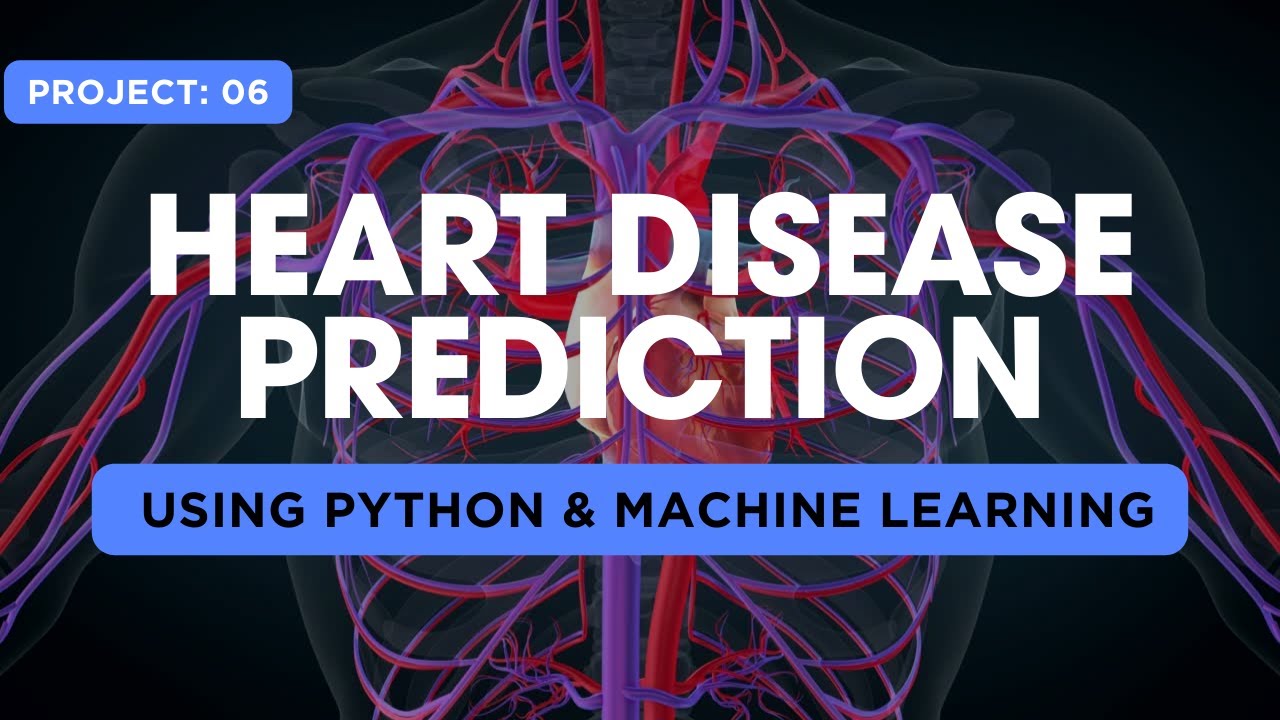
Project 06: Heart Disease Prediction Using Python & Machine Learning
5.0 / 5 (0 votes)