AI art, explained
Summary
TLDRThe video script explores the evolution of AI-generated images, from the early experiments in 2015 to the present capabilities of models like DALL-E. It discusses the concept of 'prompt engineering' and the creative potential unlocked by these technologies, while also highlighting the ethical and societal implications, including biases in training data and the impact on professional artists.
Takeaways
- 🧠 The major development in AI research in 2015 was automated image captioning, where machine learning algorithms could label objects and describe them in natural language.
- 🔄 Researchers became curious about the reverse process, text-to-image generation, aiming to create novel scenes that didn't exist in reality.
- 🚀 Early attempts resulted in rudimentary images, but the 2016 paper showcased the potential for future advancements in this field.
- 🌐 The technology has advanced dramatically in a short time, with AI now capable of generating images from text prompts that were previously unimaginable.
- 🎨 AI-generated art, like the portrait sold for over $400,000 in 2018, required specific datasets and models to mimic styles, unlike the newer, more versatile models.
- 📈 The newer models are so large that they can't be trained on individual computers, but once trained, they can generate a wide range of images from a simple text prompt.
- 🤖 The process of communicating with deep learning models to generate images is known as 'prompt engineering', which involves refining the text prompts to get desired results.
- 🖼️ The models use a 'latent space' to generate images, a mathematical space with meaningful clusters representing different concepts, rather than copying from the training data.
- 🔮 The generative process involves starting with noise and arranging pixels into a coherent image through a process called diffusion, which adds an element of randomness.
- 🌐 The technology raises copyright and ethical questions, as it can replicate styles and generate images from biased datasets found on the internet.
- 🔑 This technology has the potential to change the way humans imagine, communicate, and work within their culture, with both positive and negative long-term consequences.
Q & A
What was the major development in AI research seven years ago that led to the concept of text-to-image generation?
-The major development was automated image captioning, where machine learning algorithms could label objects in images and put those labels into natural language descriptions.
What was the initial challenge faced by researchers when they attempted to create images from text descriptions?
-The initial challenge was to generate entirely novel scenes that didn't exist in the real world, rather than retrieving existing images like a search engine does.
Can you describe the first attempt to generate an image from the text prompt 'the red or green school bus'?
-The first attempt resulted in a 32 by 32 tiny image that was barely recognizable, appearing as a blob of something on top of something else.
What is 'prompt engineering' and why is it significant in the context of text-to-image AI?
-Prompt engineering is the craft of communicating with deep learning models by providing the right text prompts to generate desired images. It's significant because it allows users to refine their interaction with the machine, creating a dialog that guides the AI to produce specific outputs.
What is the significance of the 'latent space' in the context of deep learning models used for image generation?
-The latent space is a multidimensional mathematical space where the deep learning model organizes variables that represent different aspects of images. It allows the model to generate new images that have not been seen before, based on the navigation within this space using text prompts.
How does the generative process called 'diffusion' work in creating an image from a point in the latent space?
-Diffusion starts with noise and, over a series of iterations, arranges pixels into a composition that makes sense to humans. Due to randomness in the process, the same prompt will not always result in the exact same image.
What is the role of a large, diverse training dataset in training image-generating AI models?
-A large, diverse training dataset provides the AI model with a wide range of images and their text descriptions, which helps the model learn to associate concepts with visual patterns and generate new images from text prompts.
What ethical and copyright concerns arise with the use of AI-generated images, especially when mimicking the style of known artists?
-Ethical and copyright concerns include the use of artists' work in datasets without their consent and the potential for AI to mimic their style, which may infringe on their intellectual property rights and raise questions about originality and attribution.
How does the AI's latent space reflect societal biases and cultural representation?
-The latent space of AI models contains biases and cultural representations based on the data they were trained on, often reflecting stereotypes and underrepresentation of certain groups or cultures, as it mirrors the content and biases found on the internet.
What potential long-term impacts does the advancement in text-to-image AI have on creators and the way humans imagine and communicate?
-The advancement in text-to-image AI has the potential to revolutionize the way humans create, communicate, and interact with visual content. It may lead to new forms of artistic expression, challenges in copyright and originality, and changes in the job market for creators.
What is the significance of the name 'DALL-E' given to the AI model announced by OpenAI, and what does DALLE-2 promise?
-DALL-E is named after the famous artist Salvador Dalí, reflecting the model's ability to create images from text prompts. DALLE-2, its successor, promises more realistic results and seamless editing capabilities, though it has not been released to the public yet.
Outlines
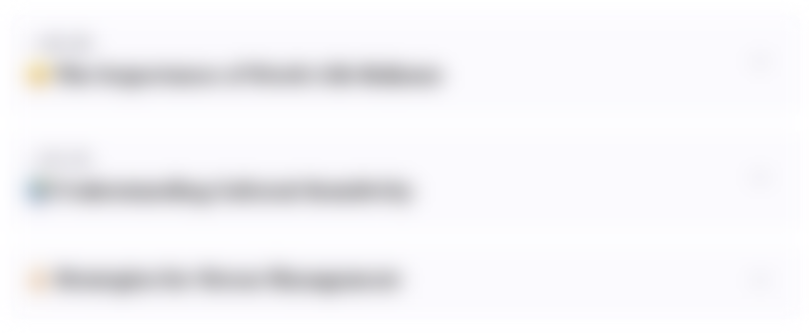
Cette section est réservée aux utilisateurs payants. Améliorez votre compte pour accéder à cette section.
Améliorer maintenantMindmap
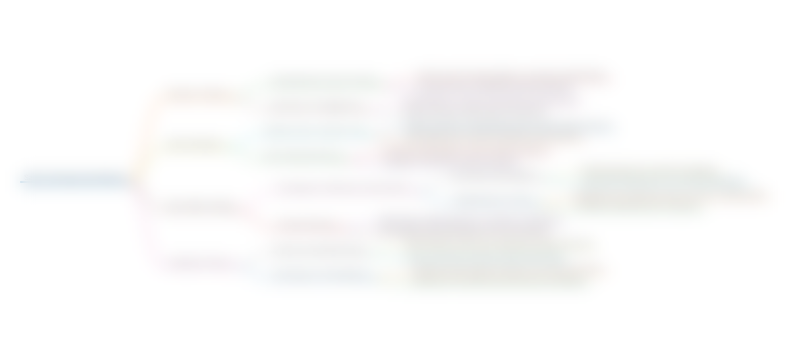
Cette section est réservée aux utilisateurs payants. Améliorez votre compte pour accéder à cette section.
Améliorer maintenantKeywords
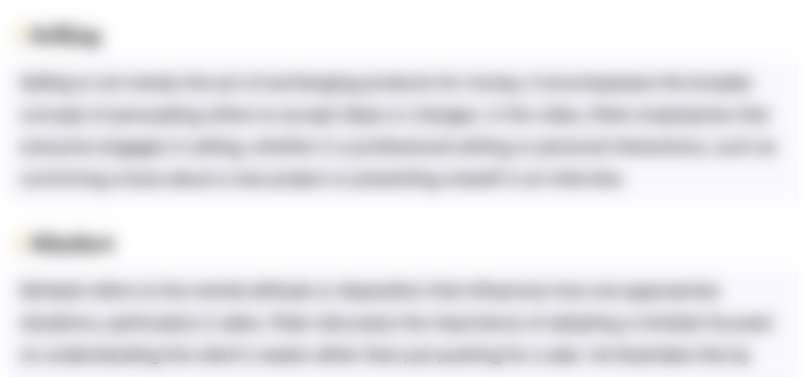
Cette section est réservée aux utilisateurs payants. Améliorez votre compte pour accéder à cette section.
Améliorer maintenantHighlights
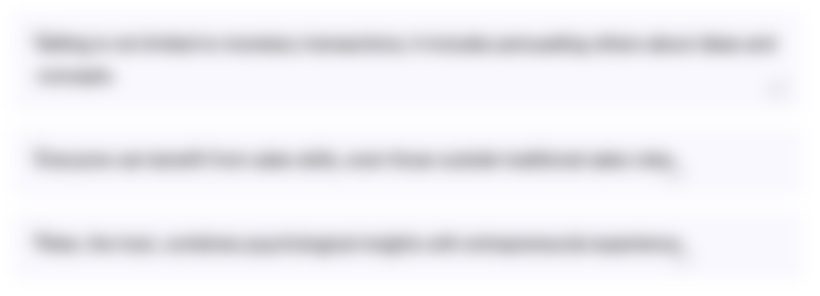
Cette section est réservée aux utilisateurs payants. Améliorez votre compte pour accéder à cette section.
Améliorer maintenantTranscripts
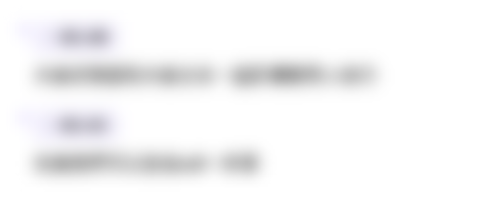
Cette section est réservée aux utilisateurs payants. Améliorez votre compte pour accéder à cette section.
Améliorer maintenantVoir Plus de Vidéos Connexes
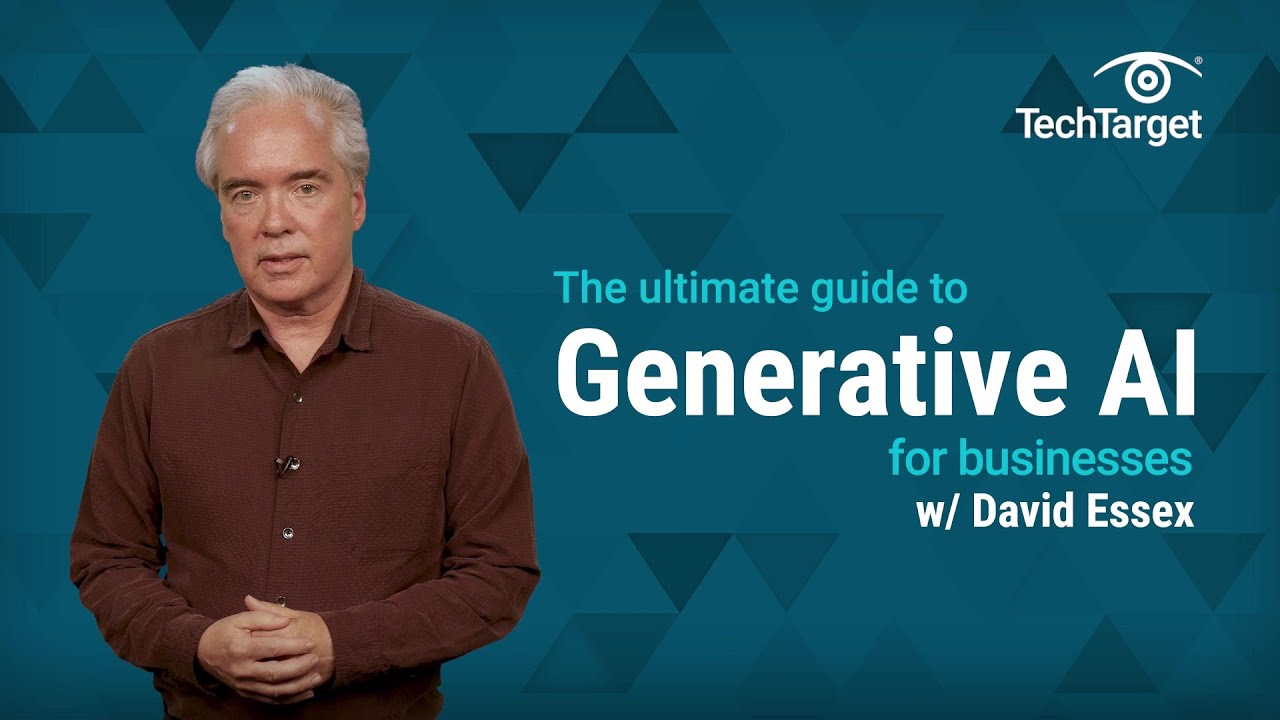
Ultimate Guide to Generative AI for Businesses
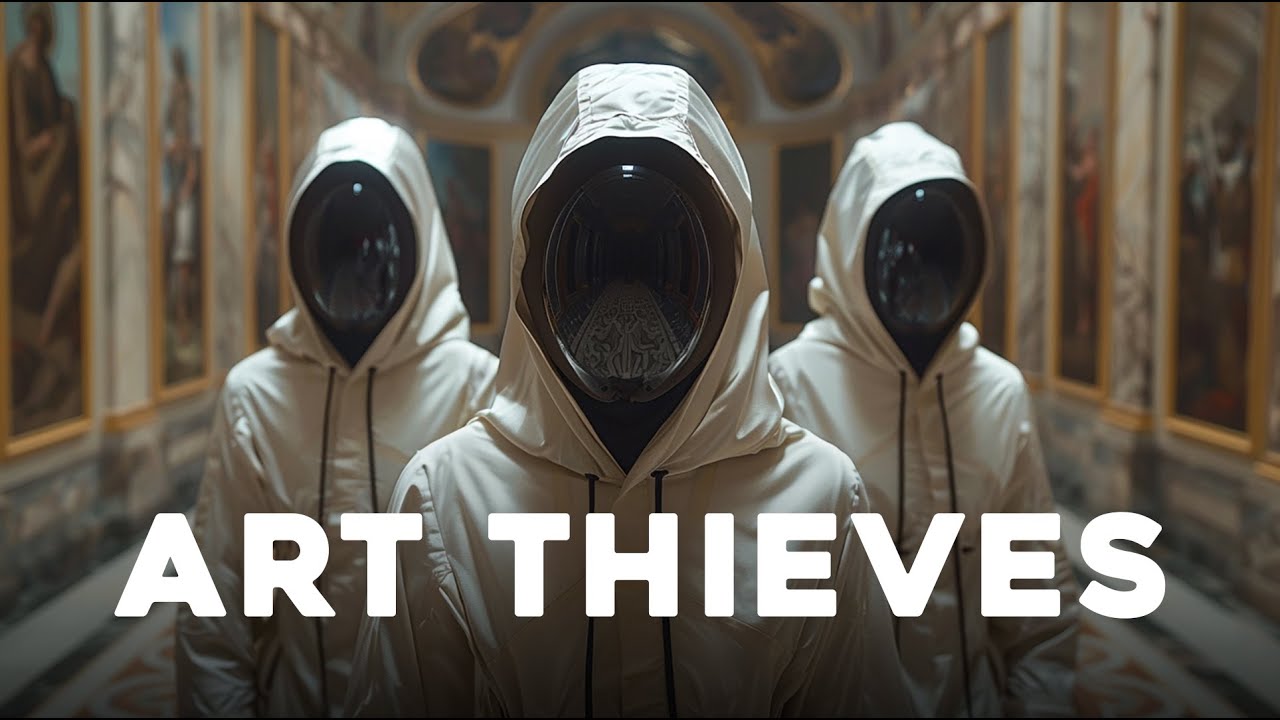
AI vs Artists - The Biggest Art Heist in History

DALL·E 2, Stable Diffusion, Midjourney: How do AI art generators work, and should artists fear …
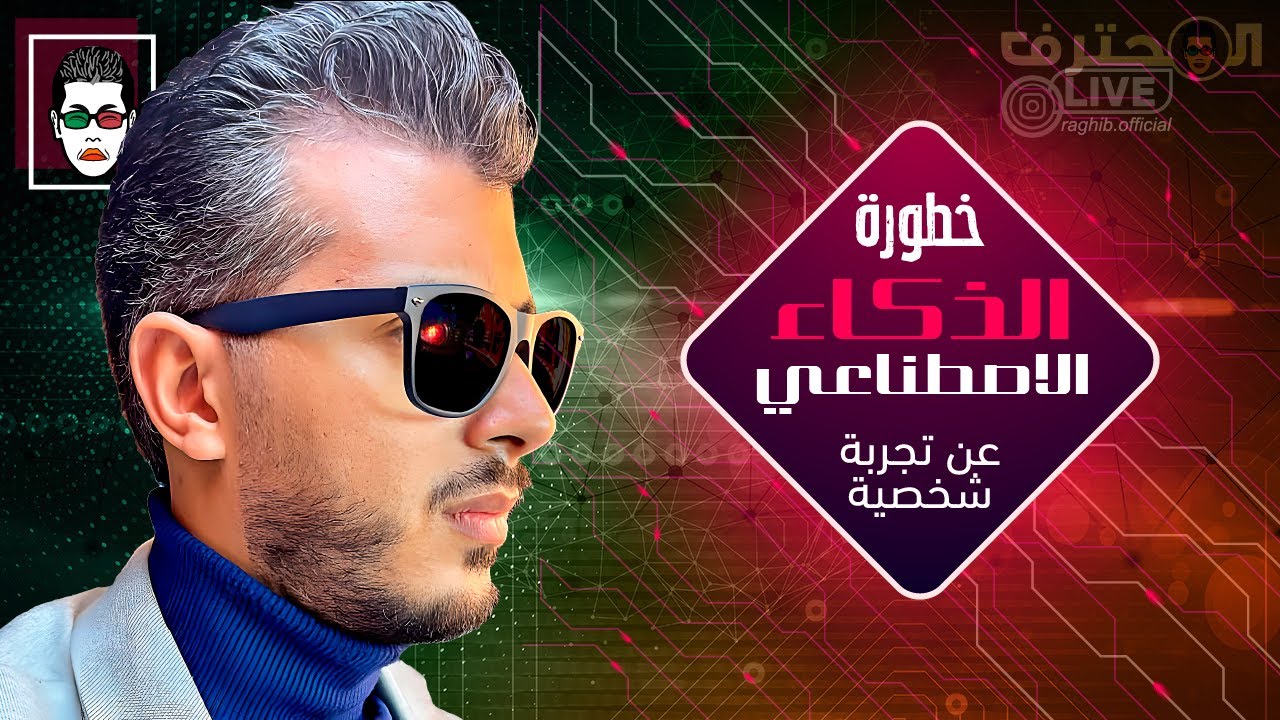
Amine Raghib | عن تجربة شخصية !! 😳 أمين رغيب : Artificial Intelligence 🤖 خطورة الذكاء الصناعي
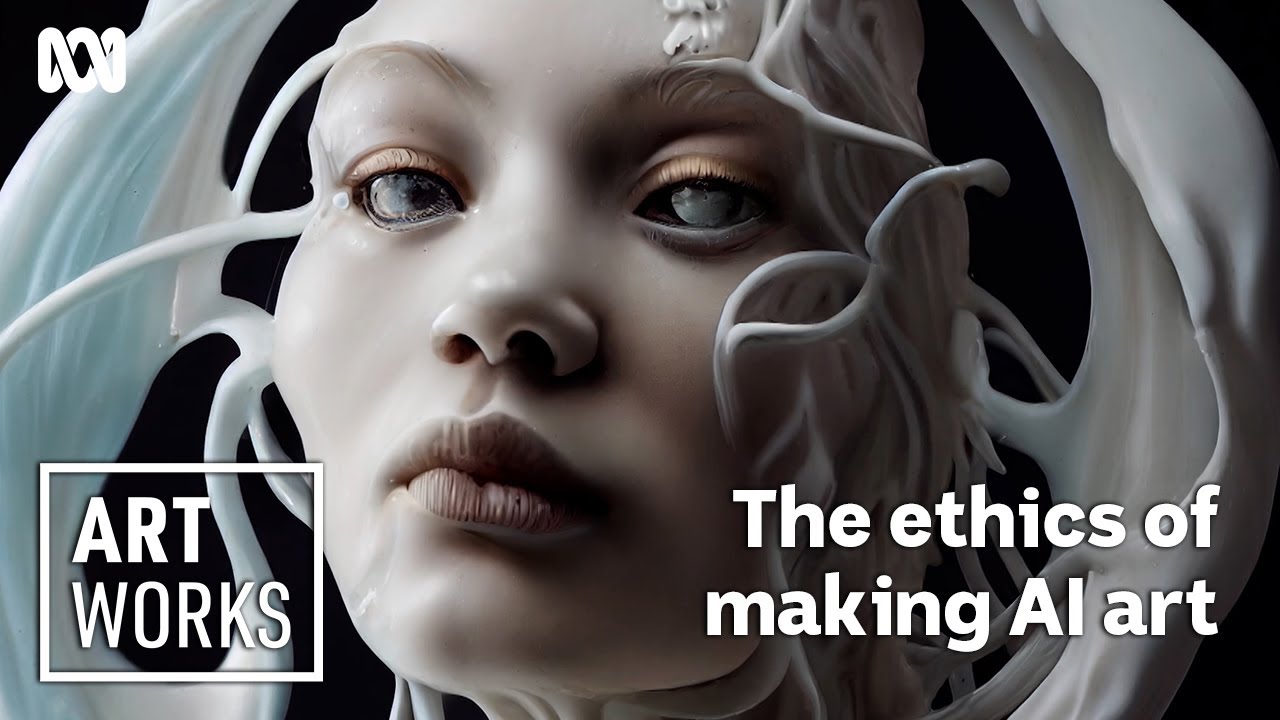
How artists and critics think AI art will transform the industry | Art Works

Why Artists are Fed Up with AI Art.
5.0 / 5 (0 votes)