Confirmatori Factor Analysis dengan SEM
Summary
TLDRThis video tutorial explores Confirmatory Factor Analysis (CFA) and construct validity using AMOS software within Structural Equation Modeling (SEM). The presenter discusses key concepts such as convergent validity, average variance extracted (AVE), construct reliability, and discriminant validity, explaining their importance in research involving latent variables. Through a practical example involving departmental conflict, administrative innovation, and technical innovation's impact on organizational performance, the video demonstrates the process of setting up the SEM model, analyzing data, and evaluating construct validity and reliability. The content is ideal for researchers aiming to validate their models in social sciences or business studies.
Takeaways
- 😀 Convergent Validity ensures that the indicators of a construct are well correlated with each other, with a factor loading threshold of 0.5 or higher to confirm validity.
- 😀 Construct Reliability (CR) assesses the internal consistency of the indicators, and values of CR greater than or equal to 0.7 are considered acceptable.
- 😀 Discriminant Validity measures how distinct a construct is from others, with higher square root values of Average Variance Extracted (AVE) compared to inter-construct correlations being a sign of good discriminant validity.
- 😀 Extracted Variance (AVE) indicates how much variance is explained by a construct's indicators, with an AVE value of 0.5 or higher being ideal for good validity.
- 😀 Confirmatory Factor Analysis (CFA) is crucial for validating the measurement model in Structural Equation Modeling (SEM), particularly when working with latent variables.
- 😀 In the example discussed, the model includes exogenous variables such as 'conflict between departments' and 'administrative innovation,' with their corresponding indicators assessed for validity.
- 😀 The process in Amos software starts with defining exogenous variables, followed by data input and variable assignment, ensuring that the correct names match with SPSS.
- 😀 The model requires careful placement of arrows and labels in Amos, ensuring that the relationships between variables and indicators are correctly represented.
- 😀 SPSS data files need to be correctly imported into Amos for the analysis, where researchers can visualize and process the data more efficiently.
- 😀 The importance of using Excel for calculating factor loadings, CR, and AVE is emphasized, as it helps in simplifying the analysis and verifying the outcomes from Amos.
- 😀 After calculating factor loadings, researchers perform checks on discriminant validity by comparing the square root of AVE with the correlation matrix between constructs.
Q & A
What is Construct Validity in the context of Structural Equation Modeling (SEM)?
-Construct validity refers to the ability of a set of indicators to measure a latent variable or construct accurately. It ensures that the indicators truly represent the intended concept in a research model.
What are the four types of Construct Validity discussed in the script?
-The four types of construct validity discussed are: 1) Convergent Validity, 2) Extracted Variance, 3) Construct Reliability, and 4) Discriminant Validity.
How is Convergent Validity assessed in SEM?
-Convergent Validity is assessed by checking the factor loadings of the indicators. If the factor loadings are 0.5 or higher, it indicates good convergent validity. A factor loading of 0.7 or above is considered excellent.
What role does Extracted Variance (AVE) play in evaluating Construct Validity?
-Extracted Variance (AVE) shows the proportion of variance that a construct explains from its indicators. An AVE value greater than or equal to 0.5 indicates good convergent validity, as the construct explains more than 50% of the variance.
What is the significance of Construct Reliability (CR) in SEM?
-Construct Reliability (CR) indicates the internal consistency of the indicators used to measure a latent variable. A CR value greater than or equal to 0.7 is generally considered acceptable and shows that the construct is measured consistently.
What is Discriminant Validity and how is it measured in SEM?
-Discriminant Validity ensures that a construct is distinct from other constructs in the model. It is measured by comparing the square root of the AVE of a construct with its correlations with other constructs. If the square root of the AVE is higher than the correlation, the construct has good discriminant validity.
Why is it important to check for Convergent and Discriminant Validity in SEM?
-Checking for both Convergent and Discriminant Validity ensures that the constructs are measured correctly and that they are distinct from each other. This improves the overall reliability and validity of the model, making the results more trustworthy.
How are factor loadings used in calculating Construct Reliability?
-Factor loadings are squared and summed up to calculate the Construct Reliability (CR). This is then divided by the sum of the squared factor loadings and the error variance. A CR value above 0.7 indicates good reliability.
In the context of the provided script, what software was used for the Confirmatory Factor Analysis?
-The software used for Confirmatory Factor Analysis (CFA) in the script is Amos. It is employed to draw the model, input data, and calculate statistics for validity and reliability checks.
What is the significance of the example provided in the script involving organizational performance?
-The example demonstrates how SEM can be used to analyze the impact of variables such as inter-departmental conflict, administrative innovation, and technical innovation on organizational performance. It shows how CFA is applied to ensure the validity of constructs like inter-departmental conflict and innovation measures.
Outlines
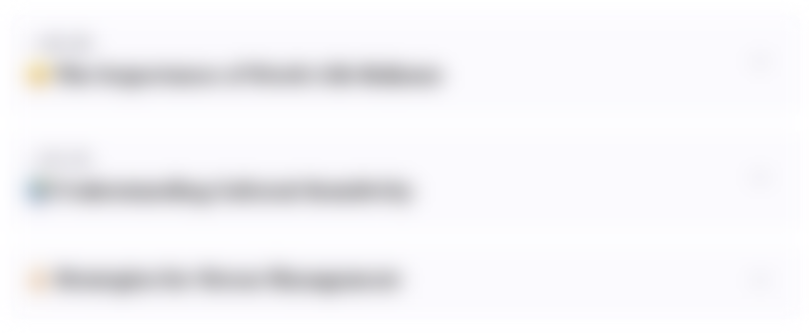
Cette section est réservée aux utilisateurs payants. Améliorez votre compte pour accéder à cette section.
Améliorer maintenantMindmap
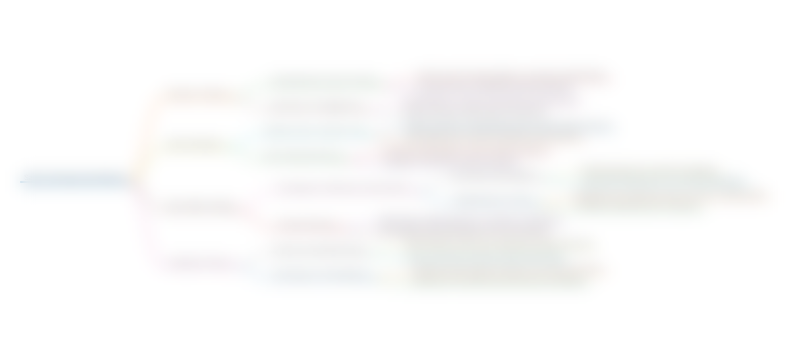
Cette section est réservée aux utilisateurs payants. Améliorez votre compte pour accéder à cette section.
Améliorer maintenantKeywords
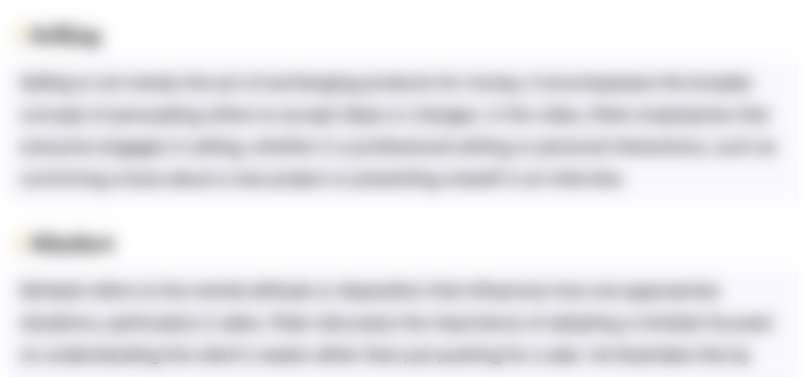
Cette section est réservée aux utilisateurs payants. Améliorez votre compte pour accéder à cette section.
Améliorer maintenantHighlights
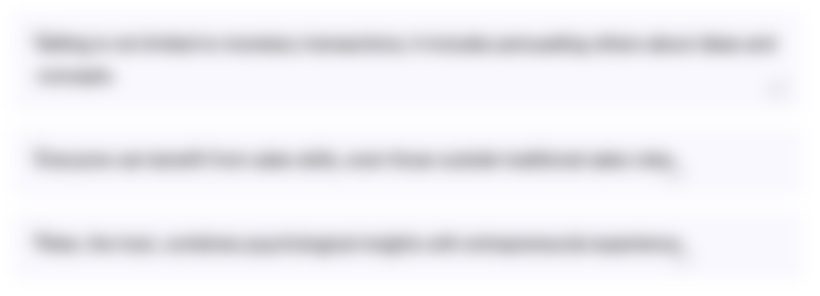
Cette section est réservée aux utilisateurs payants. Améliorez votre compte pour accéder à cette section.
Améliorer maintenantTranscripts
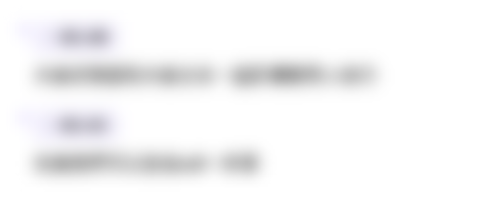
Cette section est réservée aux utilisateurs payants. Améliorez votre compte pour accéder à cette section.
Améliorer maintenantVoir Plus de Vidéos Connexes

HASIL ANALISIS SEM part 1
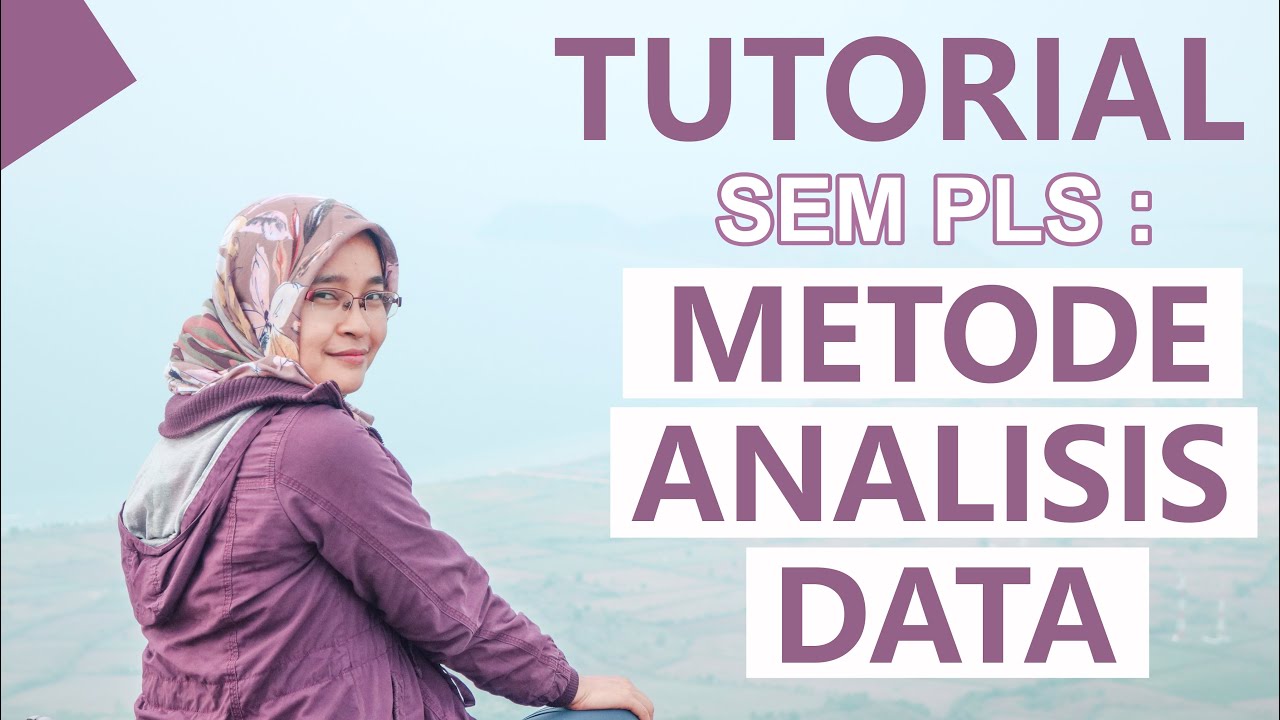
TUTORIAL SEM PLS: METODE ANALISIS DATA
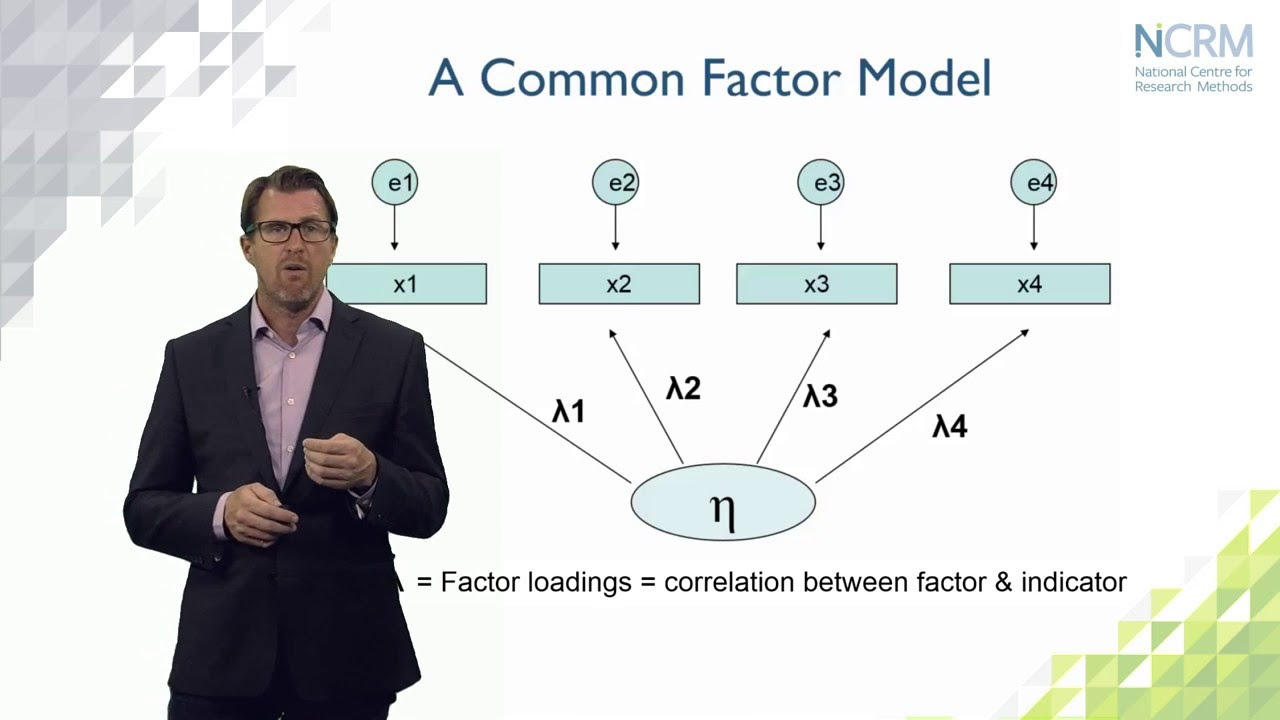
Structural Equation Modeling: what is it and what can we use it for? (part 1 of 6)

SEM Series (2016) 5. Confirmatory Factor Analysis Part 2
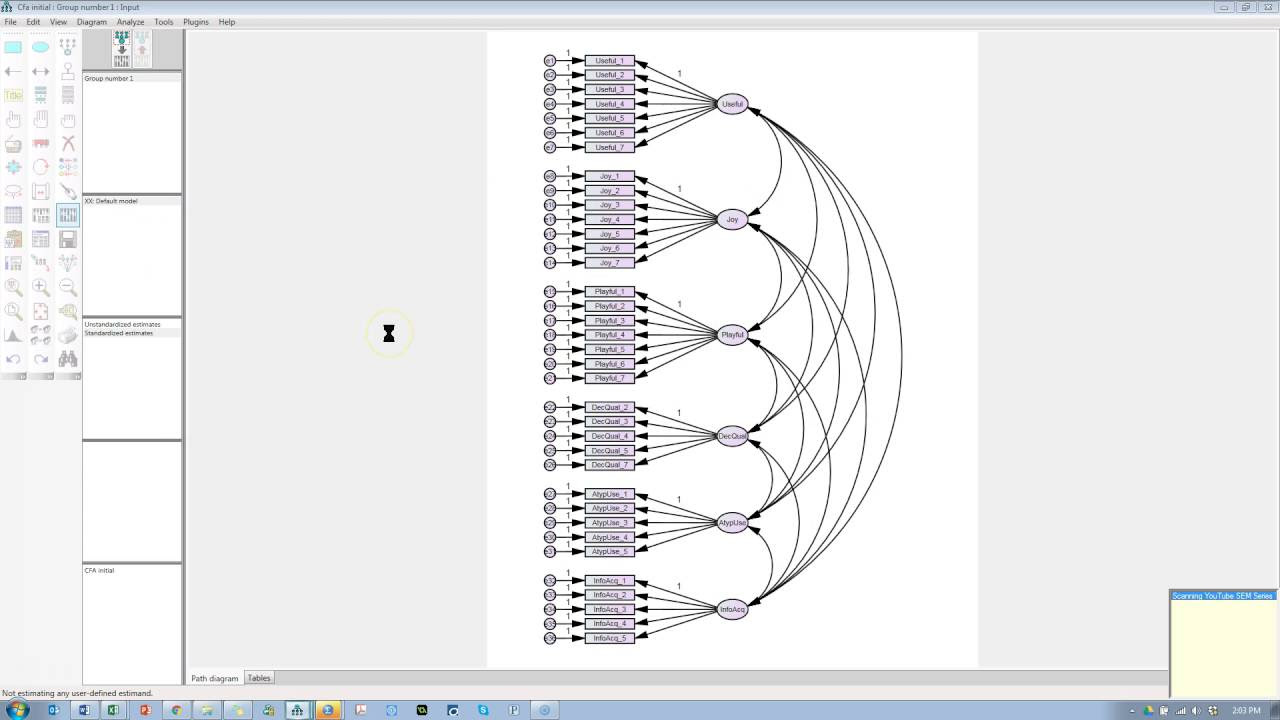
SEM Series (2016) 4. Confirmatory Factor Analysis Part 1
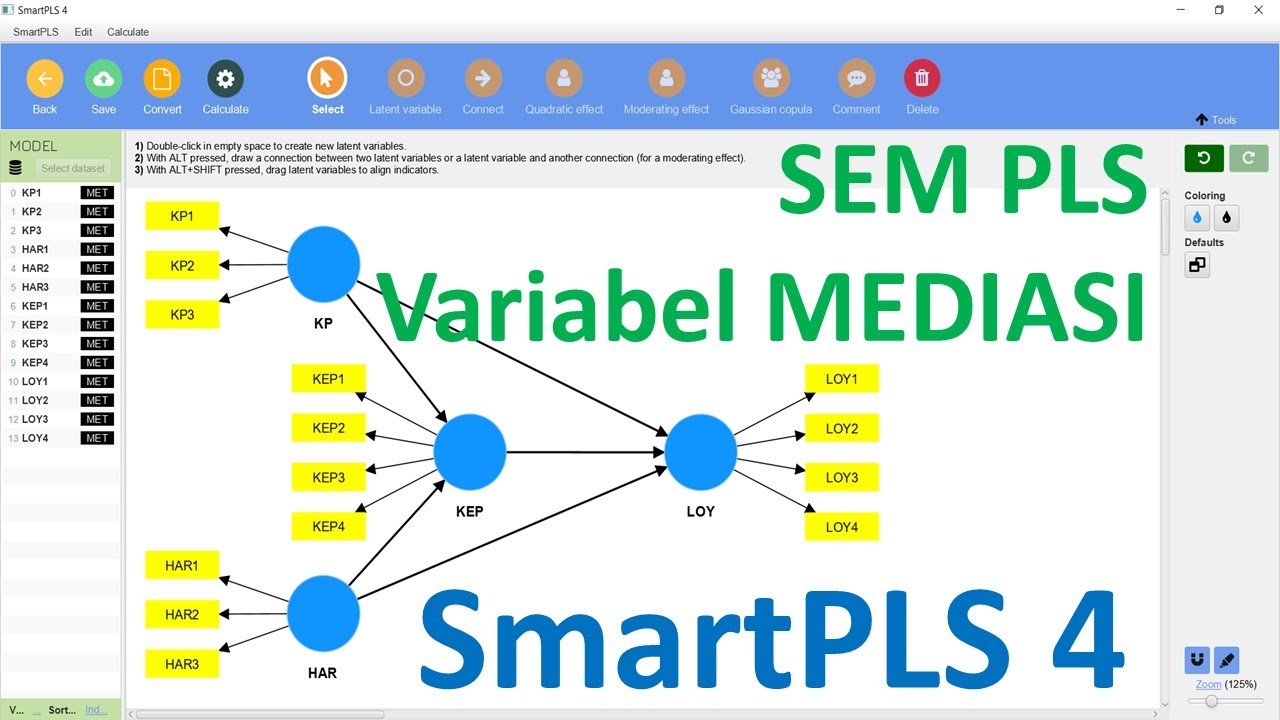
Tutorial SEM PLS dengan Variabel Mediasi Menggunakan SmartPLS 4 || Lengkap dengan referensi
5.0 / 5 (0 votes)