W2 5 Retrieval Augmented Generation RAG
Summary
TLDRThis video explains the technique of Retrieval Augmented Generation (RAG), which enhances large language models (LMs) by providing them with additional relevant information from external documents. By retrieving specific context from a collection of resources, RAG enables LMs to generate more accurate answers, such as company-specific policies, beyond their pre-existing knowledge. The video highlights RAG applications in chatbots, PDF interactions, web search, and software development. It encourages thinking of LMs not as static knowledge databases but as dynamic reasoning engines that process and generate responses from real-time information.
Takeaways
- 😀 RAG (Retrieval Augmented Generation) enhances a language model's ability to provide more accurate answers by retrieving relevant external documents.
- 😀 The process of RAG involves three main steps: retrieving documents, augmenting the prompt with the retrieved information, and generating an informed answer.
- 😀 RAG allows language models to answer questions based on specific external content, such as company policies or PDFs, rather than relying solely on their internal training data.
- 😀 In RAG, a question like 'Is there parking for employees?' will lead the system to search relevant documents (like parking policies) before generating an answer.
- 😀 When constructing a RAG prompt, only the most relevant portion of a document is used to avoid overwhelming the model with excessive information.
- 😀 The final answer in RAG-based applications is typically accompanied by a link to the original source document, giving users the option to verify the information.
- 😀 Real-world applications of RAG include tools for chatting with PDF files, such as Panda Chat and AIO PDF, that allow users to ask specific questions about documents.
- 😀 RAG is also used by websites like Coursera and HubSpot, where chatbots answer questions based on content from the site, enhancing user interactions.
- 😀 Major search engines like Microsoft Bing, Google, and startups like You.com are utilizing RAG to transform web search, providing chat-like interfaces that generate responses instead of just showing links.
- 😀 The key takeaway from RAG is that it emphasizes using LMs as reasoning engines, not just as repositories of stored knowledge, expanding their range of applications.
- 😀 RAG can also be useful for personal users, allowing them to copy relevant text into a prompt and generate answers based on that information, expanding its use beyond just software development.
Q & A
What is Retrieval Augmented Generation (RAG)?
-RAG is a technique that enhances the capabilities of language models (LMs) by allowing them to access external, up-to-date, or specialized information. It improves the accuracy of responses by augmenting the model’s input with relevant information from external sources.
How does RAG differ from traditional language models?
-Unlike traditional LMs, which rely solely on their internal knowledge base, RAG allows the LM to retrieve and incorporate external, relevant information to generate more accurate answers to specific questions.
What are the three steps involved in RAG?
-The three steps in RAG are: 1) Retrieving relevant documents or information, 2) Augmenting the prompt with the retrieved data, and 3) Generating a response using the augmented prompt.
Why is it important to retrieve specific documents in RAG?
-Retrieving specific documents ensures that the LM has the most relevant and up-to-date information to answer a question accurately, instead of relying on general knowledge learned from training data.
What type of documents can be used in the RAG process?
-Documents such as company manuals, policies, white papers, or any other relevant data repositories can be used in the RAG process, depending on the type of question being asked.
Can RAG be applied to real-world applications? If so, how?
-Yes, RAG is widely used in real-world applications like chatting with PDFs, answering questions based on website content, and powering business chatbots. It improves the ability to answer specific questions by using relevant data from external sources.
What are some examples of companies using RAG in their products?
-Companies like Panda Chat, AIO PDF, Coursera, Snapchat, and HubSpot use RAG to provide more accurate answers in their chatbots and applications by retrieving and incorporating relevant information from documents or websites.
How does RAG improve web search functionalities?
-RAG enhances web search by using external data sources to generate more detailed, contextually relevant answers to search queries, as seen in platforms like Microsoft Bing, Google, and startups like You.com.
What is the significance of thinking about language models as reasoning engines rather than knowledge stores?
-Thinking of language models as reasoning engines, rather than just data stores, allows for more sophisticated applications. It emphasizes processing and reasoning through retrieved information, rather than relying solely on stored facts.
How can users apply RAG in day-to-day tasks without complex software?
-Users can apply RAG by copying relevant text into the prompt of an online language model interface, asking the model to process the information and generate answers. This simple use of RAG can be effective for obtaining information based on specific context.
Outlines
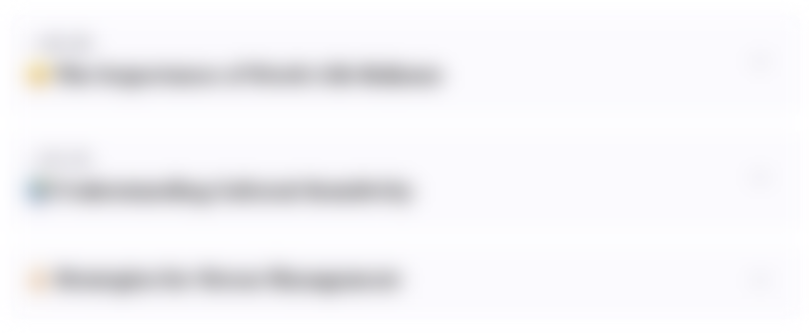
Cette section est réservée aux utilisateurs payants. Améliorez votre compte pour accéder à cette section.
Améliorer maintenantMindmap
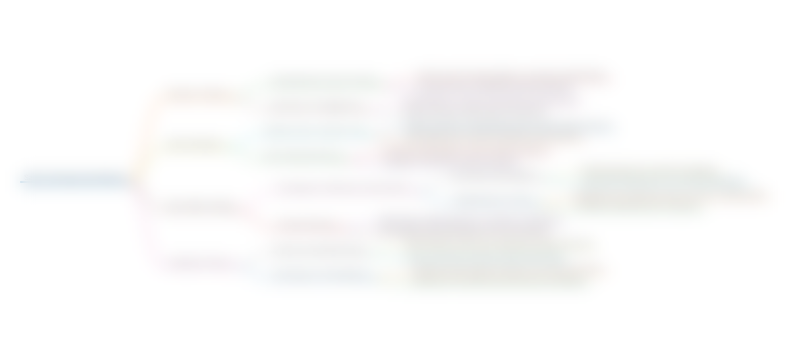
Cette section est réservée aux utilisateurs payants. Améliorez votre compte pour accéder à cette section.
Améliorer maintenantKeywords
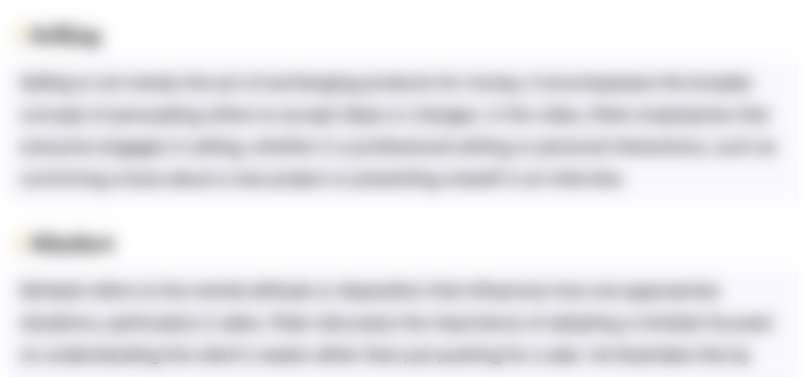
Cette section est réservée aux utilisateurs payants. Améliorez votre compte pour accéder à cette section.
Améliorer maintenantHighlights
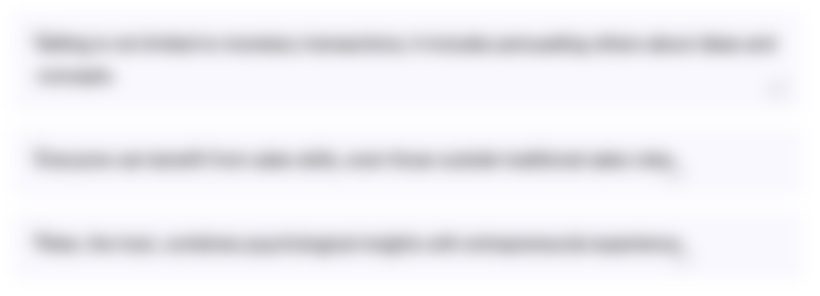
Cette section est réservée aux utilisateurs payants. Améliorez votre compte pour accéder à cette section.
Améliorer maintenantTranscripts
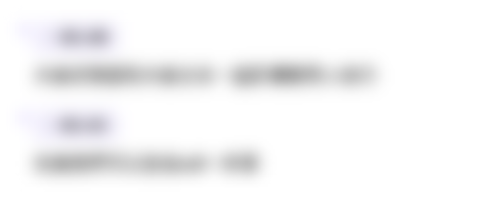
Cette section est réservée aux utilisateurs payants. Améliorez votre compte pour accéder à cette section.
Améliorer maintenantVoir Plus de Vidéos Connexes

Build your own RAG (retrieval augmented generation) AI Chatbot using Python | Simple walkthrough
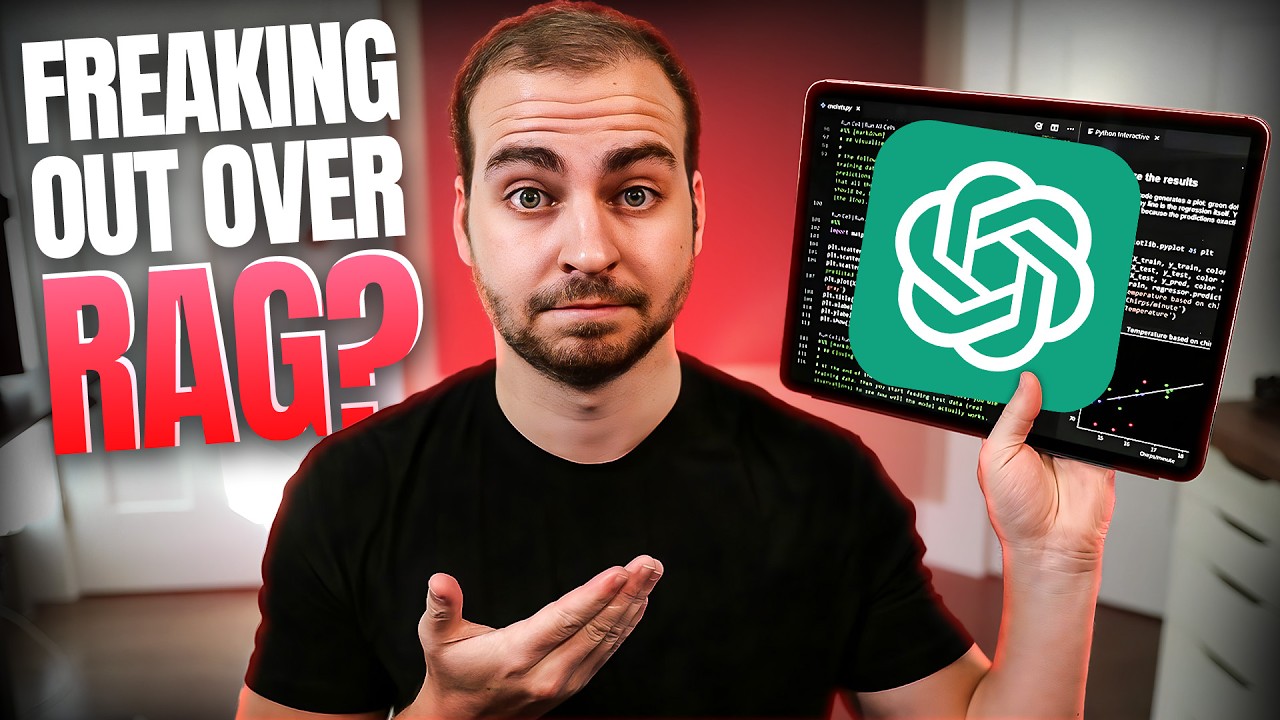
Why Everyone is Freaking Out About RAG
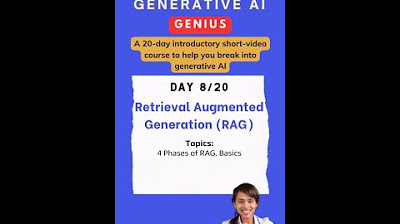
Introduction to Generative AI (Day 7/20) #largelanguagemodels #genai
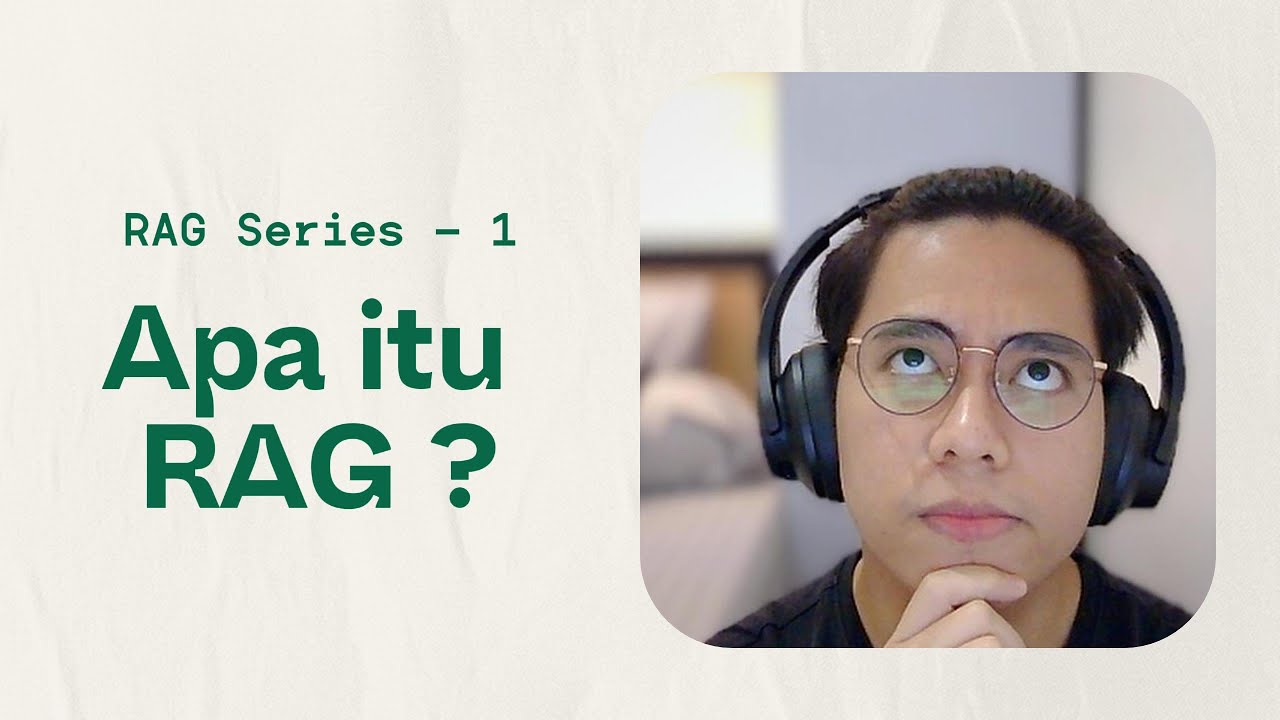
[RAG Series #1] Hanya 10 menit paham bagaimana konsep dibalik Retrieval Augmented Generation (RAG)
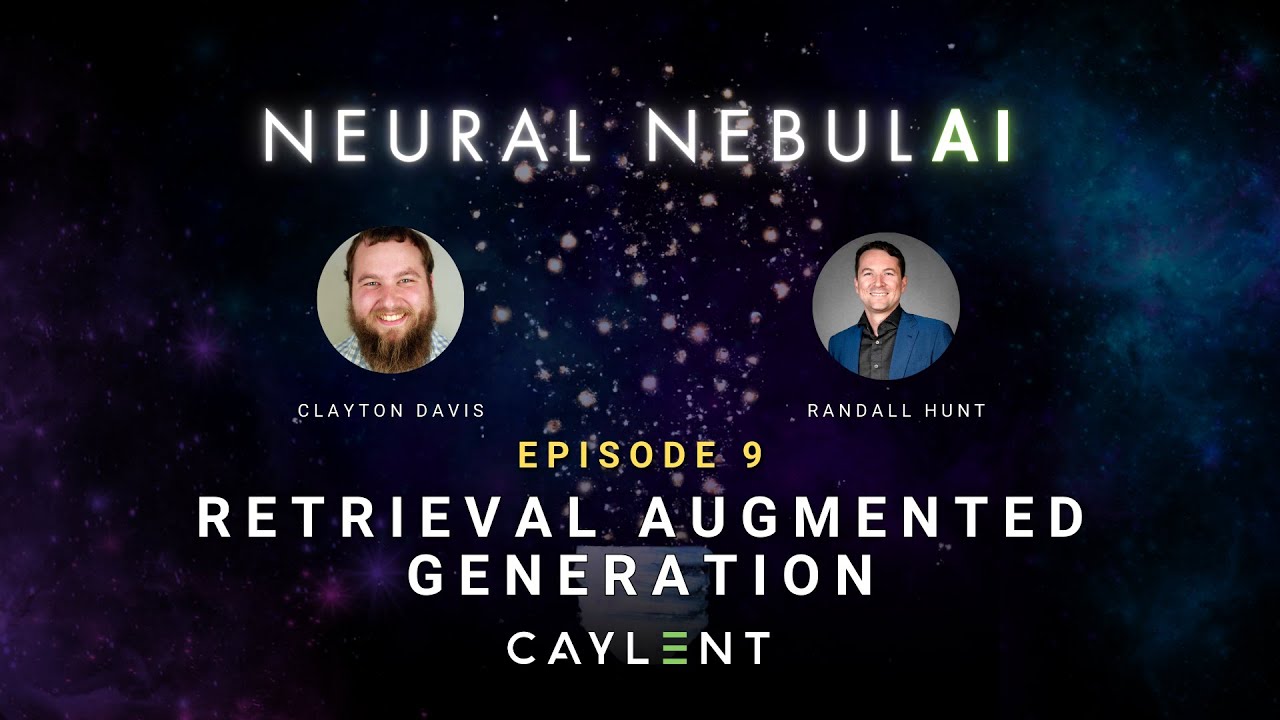
Retrieval Augmented Generation - Neural NebulAI Episode 9
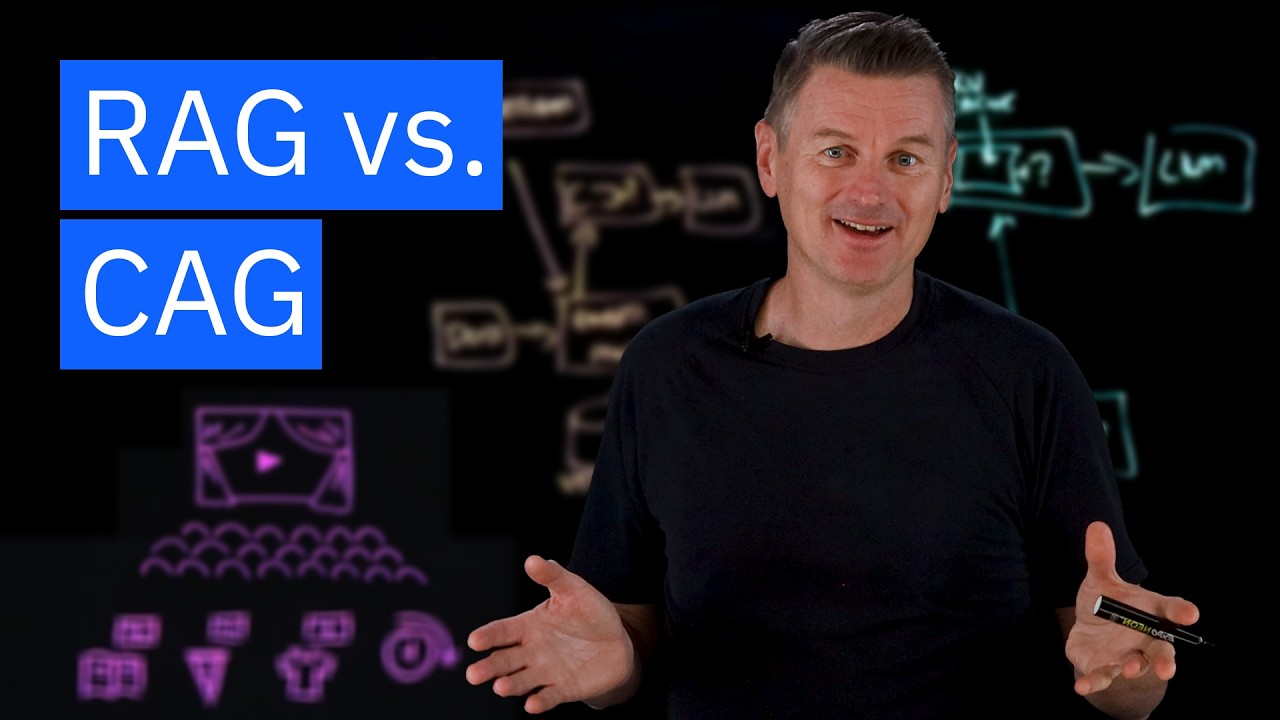
RAG vs. CAG: Solving Knowledge Gaps in AI Models
5.0 / 5 (0 votes)