Le paradoxe de Simpson
Summary
TLDRIn this video, the concept of Simpson's Paradox is explored through an intriguing example involving medical treatments for tumors. The paradox demonstrates how aggregate data can mislead, revealing different outcomes when data is broken down by confounding factors like tumor size. Through relatable examples, such as the effects of redoubling students and beer consumption on libido, the video highlights the importance of understanding data analysis methods. It emphasizes the need for careful consideration of confounding factors and recommends using prospective studies to avoid misleading conclusions and better establish cause-effect relationships.
Takeaways
- 😀 Simpson's Paradox reveals how trends in statistics can appear to be true in individual groups but reversed when the groups are combined.
- 😀 When presented with statistics, it's crucial to analyze data by different factors (e.g., tumor size, sex) to avoid misleading conclusions.
- 😀 The paradox is illustrated through the comparison of chemotherapy and surgery for tumor treatment, showing that overall statistics can mislead if confounding variables are ignored.
- 😀 In medical treatments, although chemotherapy seems better in the global statistics, surgery is actually more effective for both small and large tumors when analyzed separately.
- 😀 The paradox occurs because some factors, like tumor size, affect both the choice of treatment and the outcome, leading to misleading conclusions if not carefully considered.
- 😀 A confounding factor can skew data by influencing both the cause (treatment) and the effect (healing rates), leading to incorrect interpretations of the data.
- 😀 An example of Simpson's Paradox is shown with the supposed relationship between redoubling students and their final exam scores, where prior academic difficulties (confounding factor) affect the results.
- 😀 Statistical findings need to be approached critically, especially when factors like age, sex, or previous conditions could influence the outcomes.
- 😀 Prospective studies (experiments designed to gather data from scratch) are more reliable for determining cause-and-effect relationships, as they can control for confounding variables.
- 😀 In retrospective studies (analyzing existing data), there is a higher risk of falling into the Simpson's Paradox, making it essential to identify and account for confounders to draw accurate conclusions.
Q & A
What is the Simpson's Paradox?
-The Simpson's Paradox is a statistical phenomenon where trends appear in different groups of data but disappear or reverse when the groups are combined. It highlights how the way data is grouped or analyzed can lead to misleading conclusions.
How does the Simpson's Paradox apply to the medical treatment example in the transcript?
-In the medical treatment example, the paradox occurs when a study shows that medication has a higher cure rate overall compared to surgery. However, when the data is split by tumor size, surgery proves more effective for both large and small tumors. The paradox occurs due to the different distributions of tumor sizes between the treatment groups.
Why do medications appear to have a higher success rate initially in the study?
-Medications seem to have a higher cure rate because, overall, the patients receiving medication had smaller tumors, which are easier to treat. This results in a misleading conclusion when the data is combined without considering tumor size.
What role does tumor size play in the statistical outcome?
-Tumor size is a confounding factor. Larger tumors are harder to treat and have lower cure rates. The paradox arises because, historically, surgery was more often used for large tumors, which skewed the overall comparison between treatments.
What is a confounding factor in statistics?
-A confounding factor is an external variable that affects both the cause and the effect being studied, potentially leading to misleading results. In this case, tumor size is the confounding factor influencing both the choice of treatment and the treatment outcome.
How can we avoid falling into the trap of Simpson's Paradox?
-To avoid Simpson's Paradox, it's important to carefully analyze data, considering potential confounding factors. This can be done by disaggregating data into subgroups based on relevant characteristics (e.g., tumor size, gender, etc.) to ensure the analysis accounts for all influencing variables.
What would a prospective study involve in the context of the medical treatment example?
-A prospective study would involve randomly assigning patients to treatment groups (medication or surgery) and tracking their outcomes over time. This approach would reduce the influence of confounding factors like tumor size, providing more reliable results.
Why is it difficult to draw conclusions from retrospective studies in the examples given?
-Retrospective studies rely on existing data, which may not control for confounding factors. In the examples, historical treatment choices (like using surgery for larger tumors) make it challenging to determine the true effect of a treatment without further analysis or prospective studies.
What does the example of redoubling in education demonstrate about statistical analysis?
-The redoubling example shows how confounding factors (such as the student's academic level) can skew statistical conclusions. Simply comparing the performance of students who repeated a year without controlling for other factors might lead to false conclusions about the effects of redoubling.
How does the beer and libido example illustrate Simpson's Paradox?
-In the beer and libido example, the data initially shows a correlation between beer consumption and libido. However, when separated by gender, no correlation is found in either group. This happens because the average beer consumption and libido levels differ significantly between men and women, illustrating how aggregation can obscure real trends.
Outlines
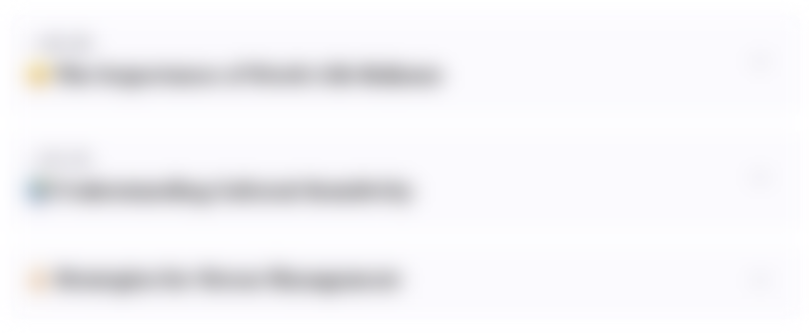
Cette section est réservée aux utilisateurs payants. Améliorez votre compte pour accéder à cette section.
Améliorer maintenantMindmap
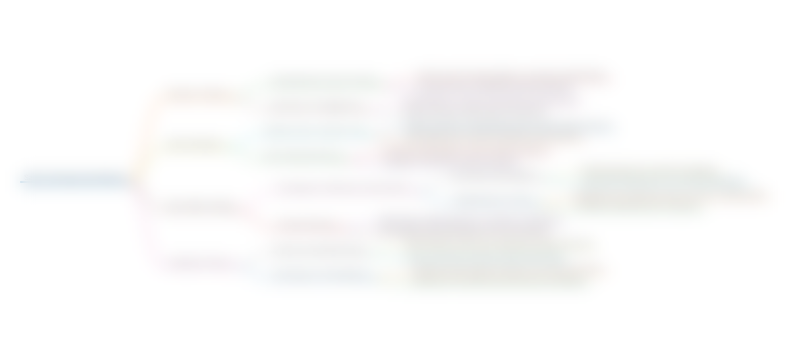
Cette section est réservée aux utilisateurs payants. Améliorez votre compte pour accéder à cette section.
Améliorer maintenantKeywords
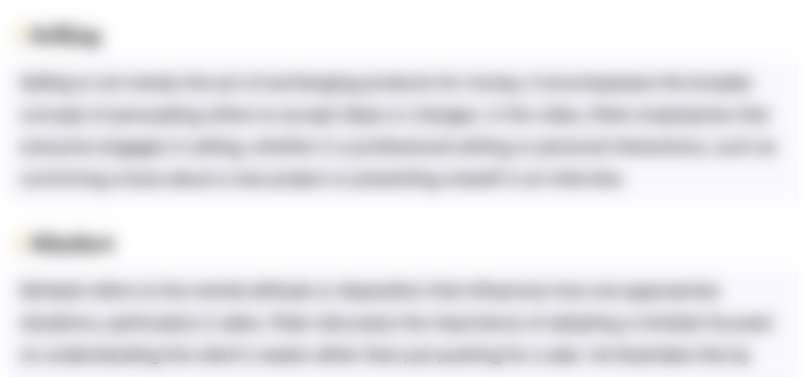
Cette section est réservée aux utilisateurs payants. Améliorez votre compte pour accéder à cette section.
Améliorer maintenantHighlights
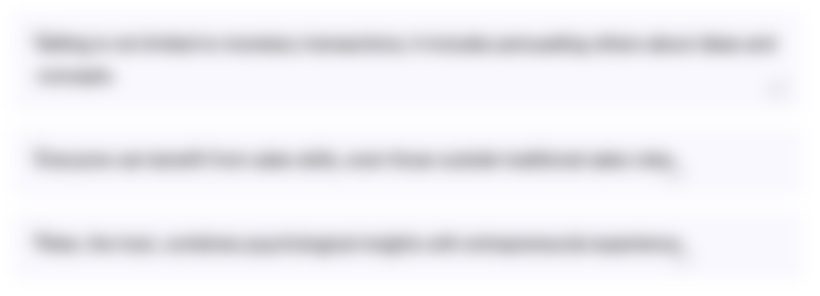
Cette section est réservée aux utilisateurs payants. Améliorez votre compte pour accéder à cette section.
Améliorer maintenantTranscripts
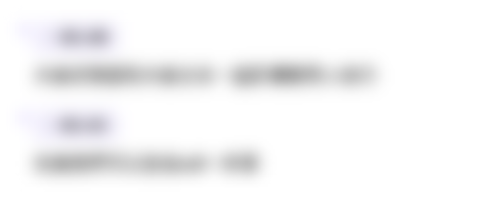
Cette section est réservée aux utilisateurs payants. Améliorez votre compte pour accéder à cette section.
Améliorer maintenantVoir Plus de Vidéos Connexes
5.0 / 5 (0 votes)