Data Observability: MultiCloud, GenAI Make Challenges Harder
Summary
TLDRThe video explores the critical role of data observability in maintaining the integrity and trustworthiness of AI models, especially in sectors like finance and healthcare. It emphasizes the need for high-quality data input and ongoing monitoring to prevent discrepancies between model outputs and real-world conditions. The featured company highlights its comprehensive platform designed to help enterprises manage data pipelines, ensuring data quality and infrastructure reliability. As businesses increasingly rely on cloud technologies, the discussion outlines various use cases showcasing the platform's capabilities and its future growth potential as a leading data management solution.
Takeaways
- 😀 High-quality data input is essential for training AI models effectively.
- 🔍 Continuous monitoring of AI model inferences is crucial to ensure they reflect real-world changes.
- 📊 Data observability plays a critical role in maintaining trust in AI outputs, especially in finance and healthcare.
- ⚠️ The erosion of trust can occur quickly if AI models produce inaccurate or misleading information.
- 🏗️ A robust data observability platform helps enterprises monitor data quality, infrastructure, and spending.
- 📈 Large enterprises are increasingly adopting data observability tools to enhance customer data quality and operational reliability.
- 🔄 Companies must adapt to changing data landscapes to prevent discrepancies between model inferences and actual business conditions.
- 🌐 Trust is paramount in sectors like finance and healthcare, where data accuracy impacts decision-making.
- 💡 Enterprises are leveraging data observability to manage cloud costs and ensure efficient resource usage as AI deployment grows.
- 🚀 The ongoing development of data observability capabilities is crucial for organizations to remain competitive and responsive.
Q & A
What is the significance of data quality in AI model training?
-Data quality is crucial for AI model training because high-quality data ensures that the models can make accurate predictions. Poor data input can lead to erroneous outputs, often summarized by the phrase 'garbage in, garbage out.'
What ongoing challenges do businesses face after training AI models?
-Businesses need to continuously monitor the inferences made by AI models, as these can diverge from the ground truth over time due to changes in business conditions. This divergence can lead to significant issues if not managed properly.
How does data observability contribute to AI model reliability?
-Data observability plays a critical role in ensuring AI model reliability by allowing organizations to monitor data quality, infrastructure, and model performance continuously, thus maintaining trust in the system.
What are some key sectors mentioned that rely heavily on data observability?
-The sectors mentioned include finance and healthcare, where accurate data handling is essential for maintaining customer trust and ensuring compliance with regulations.
What has the company discussed in the video achieved in the last six years?
-The company has developed a comprehensive data observability platform and established itself as a leader in enterprise data observability, helping businesses monitor their data pipelines effectively.
What functionalities does the company's platform offer to enterprises?
-The platform offers capabilities to monitor data quality, track schema changes, manage data drift, and oversee the overall health of data infrastructure, which is vital for modern organizations.
How are large banks utilizing the discussed platform?
-Large banks are using the platform to improve customer data quality, which is essential for making informed credit decisions and enhancing customer service.
Why is real-time monitoring of transactions important?
-Real-time monitoring of transactions helps prevent outages and ensures that services remain operational, which is critical for maintaining trust and efficiency in financial operations.
What impact do rising cloud costs have on businesses?
-Rising cloud costs prompt businesses to be more vigilant about their data management strategies, ensuring that they optimize their resources while still leveraging cloud technologies effectively.
What was the key takeaway from the conversation about data management?
-The key takeaway is the importance of establishing a robust data management system that incorporates observability to enhance trust, reliability, and efficiency in business operations, particularly in sensitive sectors.
Outlines
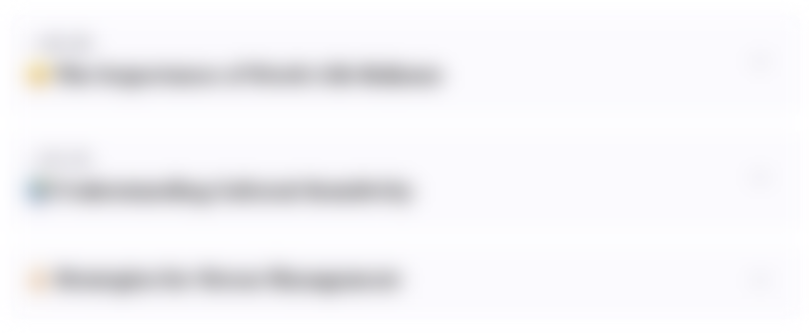
Cette section est réservée aux utilisateurs payants. Améliorez votre compte pour accéder à cette section.
Améliorer maintenantMindmap
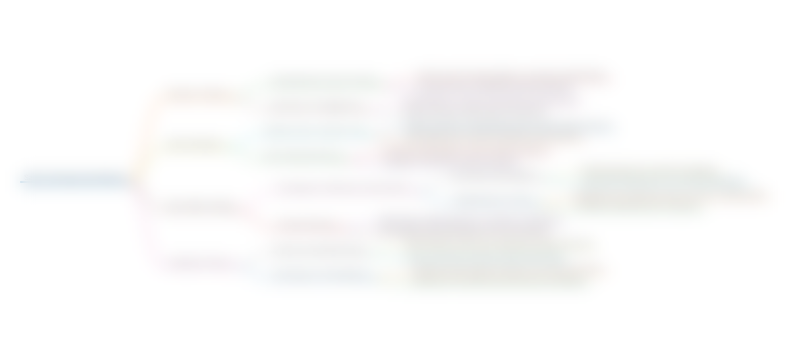
Cette section est réservée aux utilisateurs payants. Améliorez votre compte pour accéder à cette section.
Améliorer maintenantKeywords
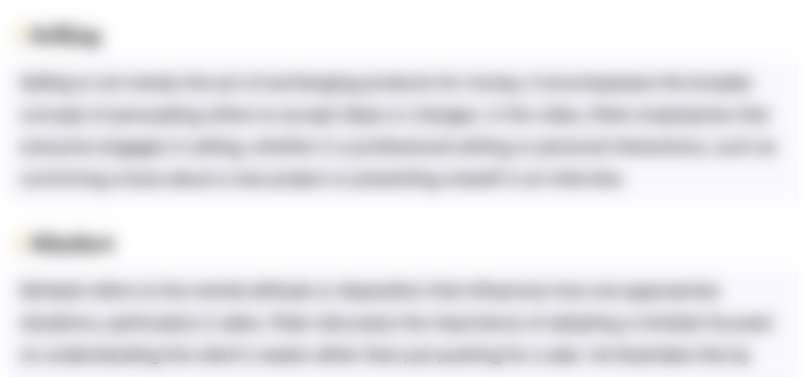
Cette section est réservée aux utilisateurs payants. Améliorez votre compte pour accéder à cette section.
Améliorer maintenantHighlights
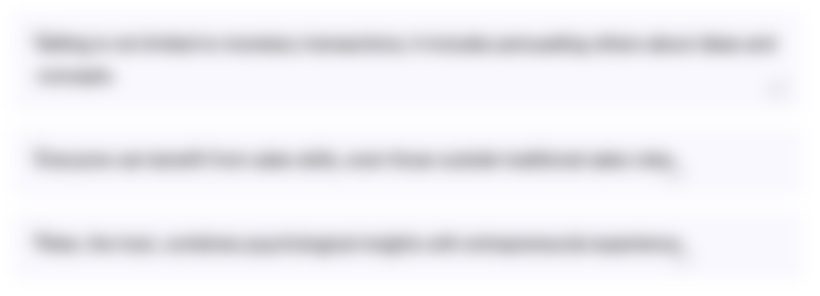
Cette section est réservée aux utilisateurs payants. Améliorez votre compte pour accéder à cette section.
Améliorer maintenantTranscripts
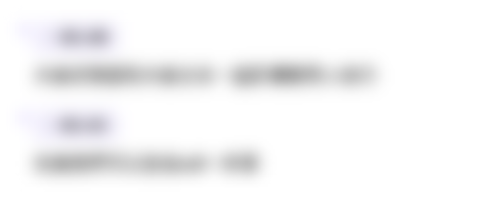
Cette section est réservée aux utilisateurs payants. Améliorez votre compte pour accéder à cette section.
Améliorer maintenantVoir Plus de Vidéos Connexes
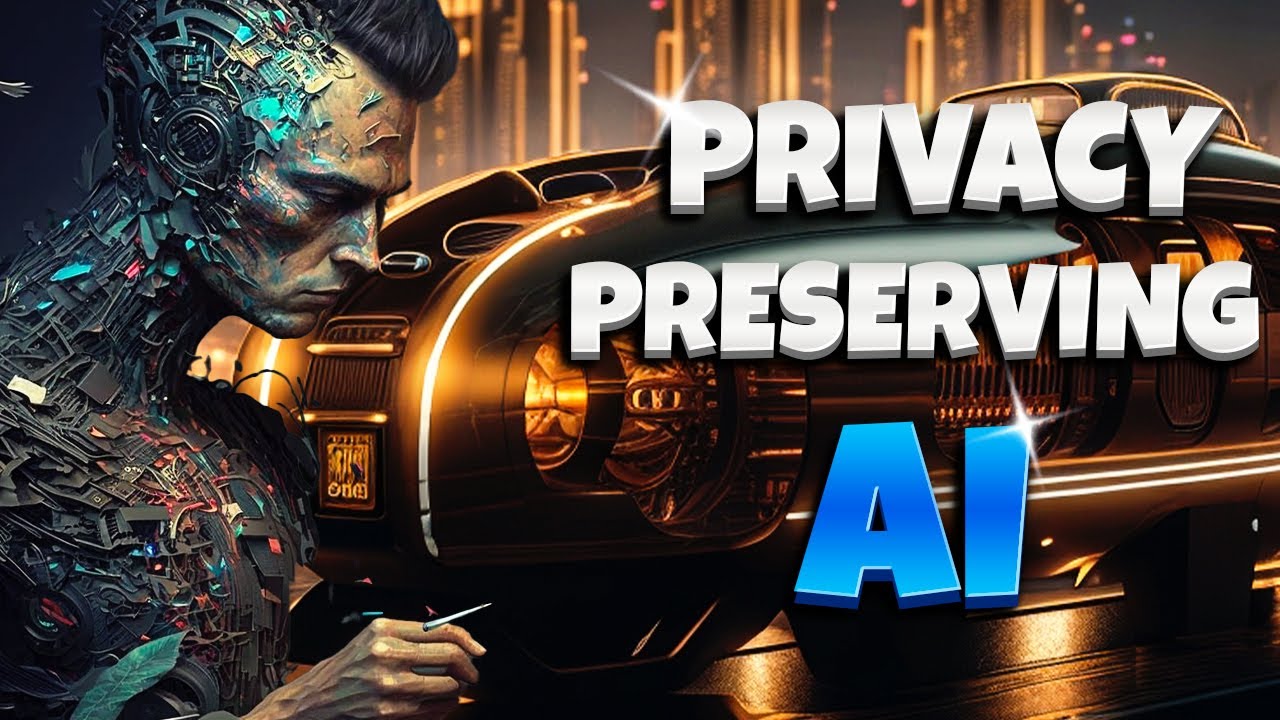
AI and Data Privacy: Balancing Innovation and Personal Security
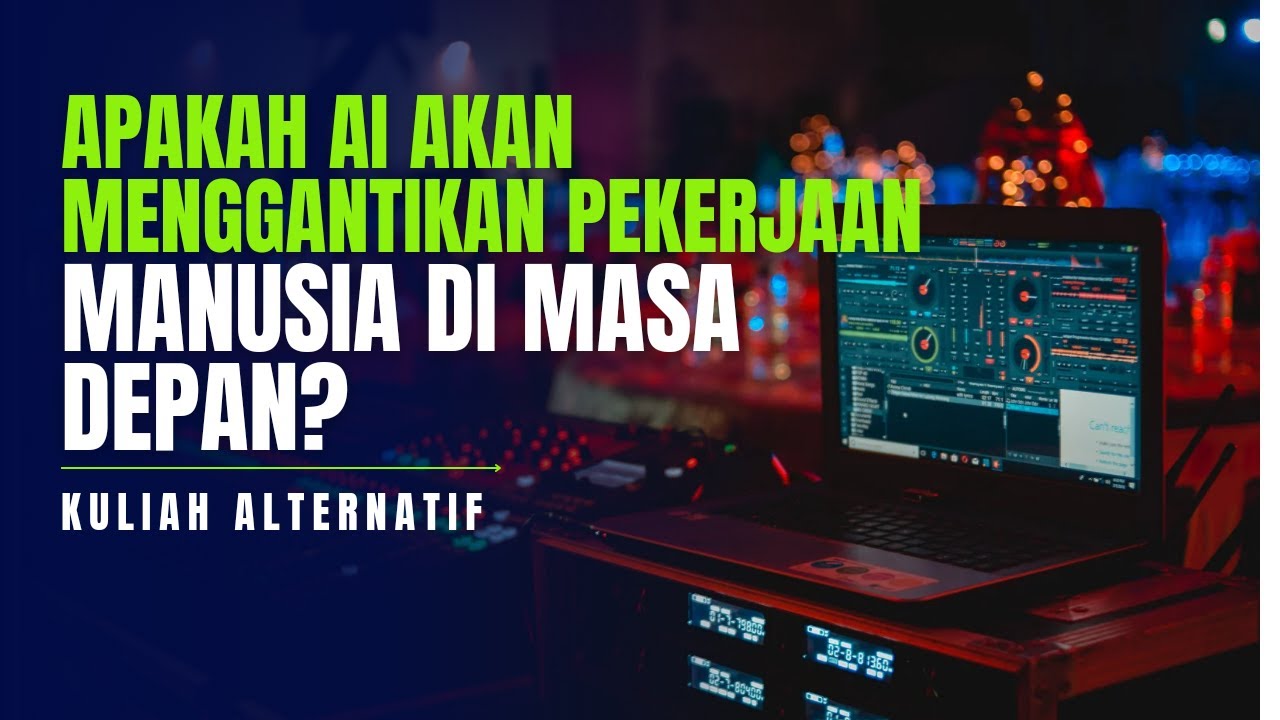
Apakah AI akan menggantikan Pekerjaan Manusia di Masa Depan..?
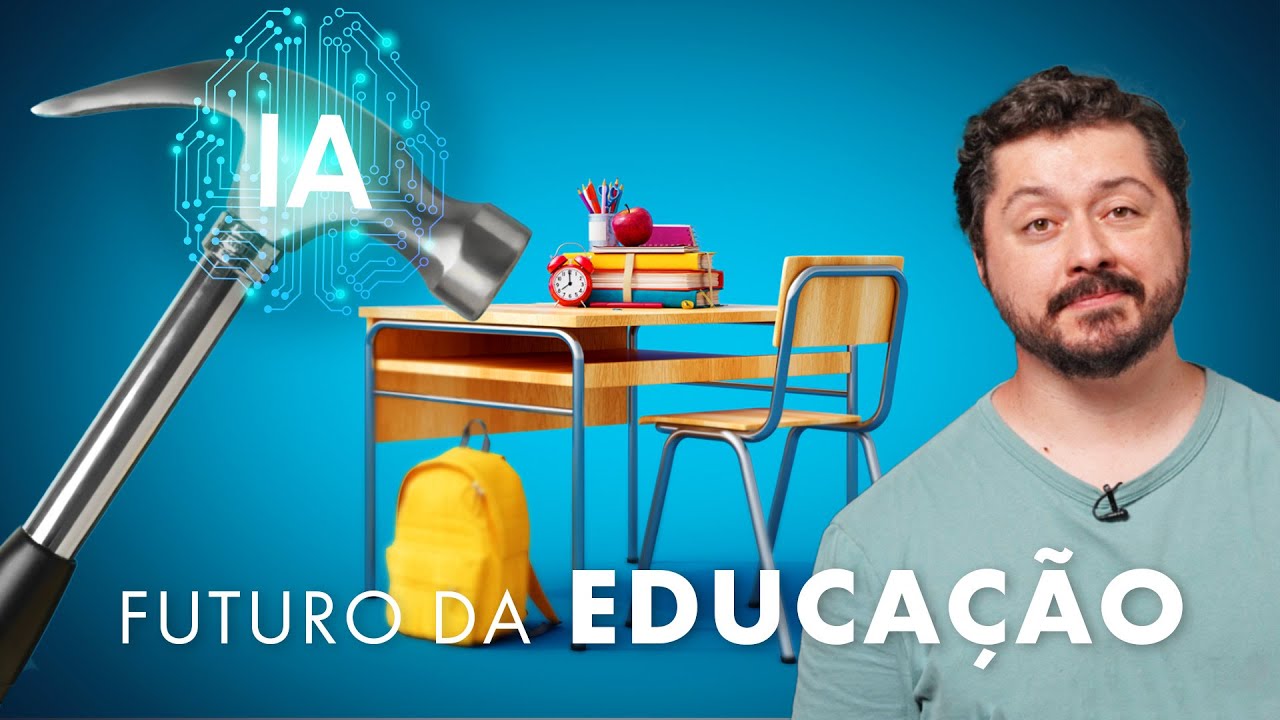
Inteligência Artificial vai destruir o futuro da educação
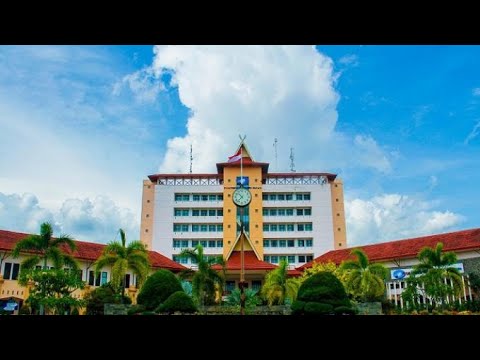
Perkuliahan Robotik dan Kecerdasan Buatan : Pengenalan AI

UKURAN PENYEBARAN DATA - Matematika Wajib Kelas 12
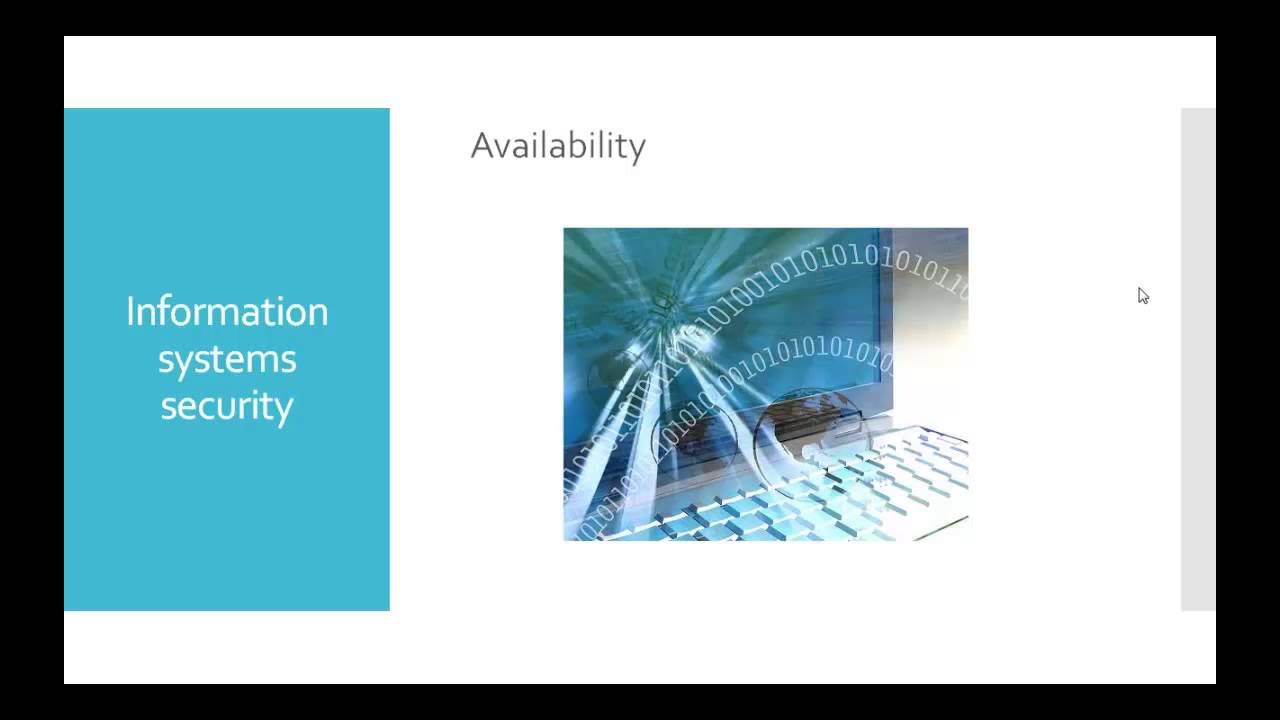
Information systems security
5.0 / 5 (0 votes)