What Makes Large Language Models Expensive?
Summary
TLDRThis video discusses the true cost of generative AI for enterprises, focusing on large language models (LLMs) and highlighting the factors that influence cost beyond simply subscribing to a chatbot like ChatGPT. Key cost drivers covered include use case, model size, pre-training, inferencing, tuning, hosting, and deployment. The video emphasizes the importance of choosing the right platform and vendor to meet specific enterprise needs, as well as the trade-offs between cloud and on-premise deployment options. It also touches on the complexities of adapting models and the associated costs, providing a comprehensive overview of AI implementation in businesses.
Takeaways
- 😀 Generative AI costs for enterprises go beyond simple subscriptions; various factors need to be considered.
- 😀 Use cases for generative AI vary, and it's important to tailor the solution to your specific needs, much like selecting a car at a dealership.
- 😀 Model size plays a key role in cost; larger models with more parameters will drive up computational and pricing needs.
- 😀 Pre-training an LLM from scratch is costly and requires vast computational resources, making it prohibitive for many enterprises.
- 😀 Inferencing costs depend on the number of tokens processed, both in the prompt and the completion of the model’s response.
- 😀 Prompt engineering is a cost-effective method to improve results without needing extensive model adjustments or increased compute.
- 😀 Tuning adjusts the model’s internal settings to improve performance, but it can be expensive depending on the method used.
- 😀 Fine-tuning is ideal for specialized tasks but comes with high costs, whereas parameter-efficient fine-tuning offers a cheaper alternative.
- 😀 Hosting costs are incurred when a model needs to be deployed for continuous interaction, which can vary depending on your usage needs.
- 😀 Deployment choices (SaaS vs. on-premise) greatly influence costs, with SaaS being more cost-effective for most enterprises, while on-premise is necessary for regulatory requirements.
Q & A
What is the main focus of the video?
-The video focuses on the true costs of generative AI for enterprises, specifically large language models (LLMs), and highlights various cost factors beyond simply subscribing to a consumer-level AI service.
How does the cost of generative AI for enterprises differ from consumer use?
-For enterprises, the cost of generative AI involves additional factors such as model size, pre-training, inferencing, and deployment, unlike consumer-level costs that are more affordable for casual or small-scale use cases.
What are the seven key cost factors enterprises need to consider when scaling generative AI?
-The seven key cost factors are: 1) Use case, 2) Model size, 3) Pre-training costs, 4) Inferencing, 5) Tuning, 6) Hosting, and 7) Deployment.
What is the importance of understanding the use case when implementing generative AI in an enterprise?
-Understanding the use case is crucial as it determines the methods and computational resources needed for the AI solution. Enterprises should pilot different models to evaluate effectiveness, cost, and speed based on specific needs.
Why does model size significantly impact the cost of generative AI?
-Larger models with more parameters require more computational resources to operate, which drives up costs. Enterprises need to assess whether the benefits of a larger model justify the increased expenses.
What are the challenges and costs associated with pre-training an LLM from scratch?
-Pre-training an LLM from scratch requires substantial compute time, resources, and data, often costing millions of dollars. For example, GPT-3's pre-training cost over $4.6 million. This is why many enterprises prefer to use pre-trained models.
What is inferencing, and how does it influence costs?
-Inferencing is the process by which a model generates responses based on input prompts. The cost is based on the number of tokens processed during inferencing, with each token representing roughly ¾ of a word.
What role does prompt engineering play in reducing costs for generative AI?
-Prompt engineering is a cost-effective method of tailoring the AI's responses by carefully crafting the prompts. This method does not require altering the model itself and helps achieve desired results without extensive compute resources.
What is the difference between fine-tuning and parameter-efficient fine-tuning (PFT)?
-Fine-tuning involves extensive modification of a model's parameters, requiring large amounts of labeled data, and is costly. Parameter-efficient fine-tuning (PFT) aims to achieve specific task performance without altering the core model, using fewer data and resources.
How does hosting a model impact the overall cost for an enterprise?
-Hosting a model involves maintaining the necessary infrastructure, including GPUs and computational resources, which can lead to significant costs. Enterprises must decide whether to host a model themselves or use an API for inferencing based on their needs.
What are the differences in cost between SaaS deployment and on-premise deployment?
-SaaS deployment offers predictable subscription-based pricing, avoiding the need for hardware and GPU procurement, while on-premise deployment requires purchasing and maintaining hardware, offering more control but with higher upfront and maintenance costs.
Why should enterprises partner with a vendor that offers flexibility in generative AI deployment?
-A flexible vendor allows enterprises to experiment with various models and deployment methods, helping them choose the most cost-effective solution. It is particularly beneficial to work with a vendor that provides access to both API-based inferencing and hosting options.
Outlines
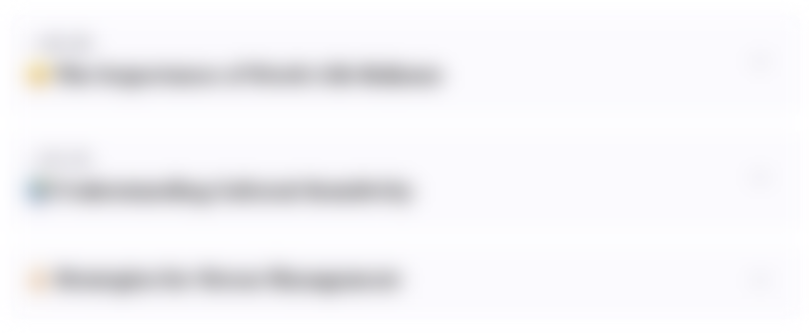
Cette section est réservée aux utilisateurs payants. Améliorez votre compte pour accéder à cette section.
Améliorer maintenantMindmap
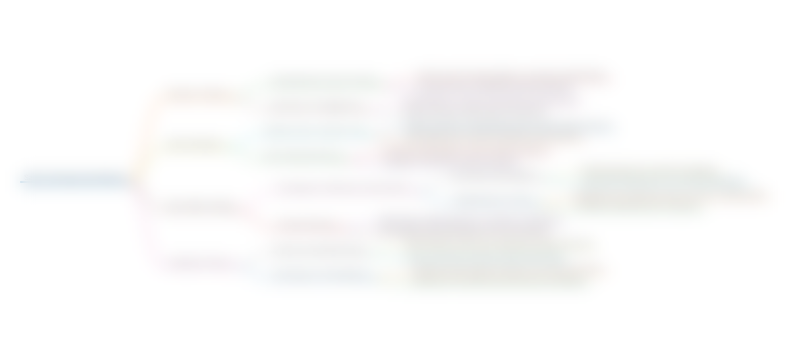
Cette section est réservée aux utilisateurs payants. Améliorez votre compte pour accéder à cette section.
Améliorer maintenantKeywords
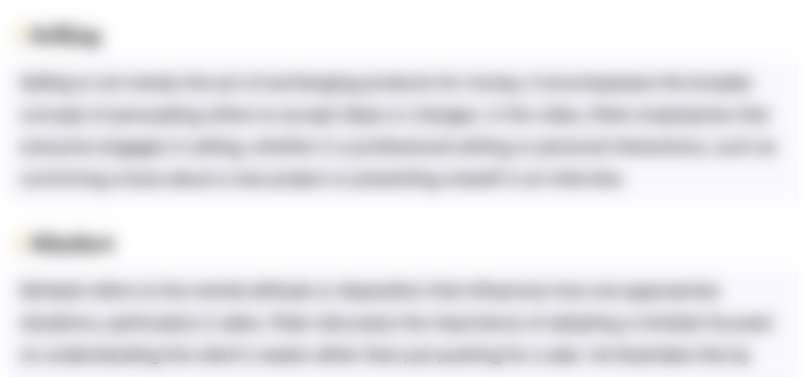
Cette section est réservée aux utilisateurs payants. Améliorez votre compte pour accéder à cette section.
Améliorer maintenantHighlights
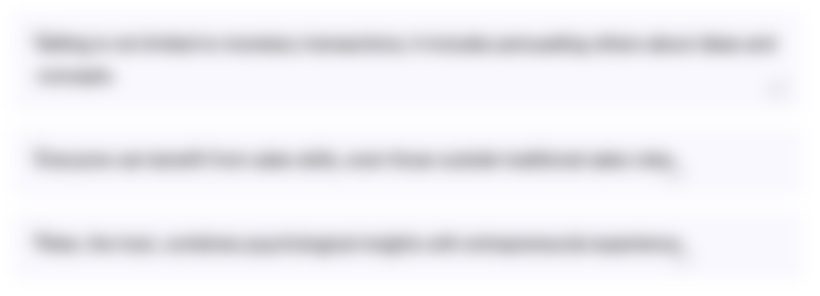
Cette section est réservée aux utilisateurs payants. Améliorez votre compte pour accéder à cette section.
Améliorer maintenantTranscripts
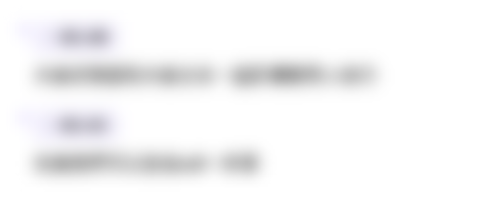
Cette section est réservée aux utilisateurs payants. Améliorez votre compte pour accéder à cette section.
Améliorer maintenantVoir Plus de Vidéos Connexes
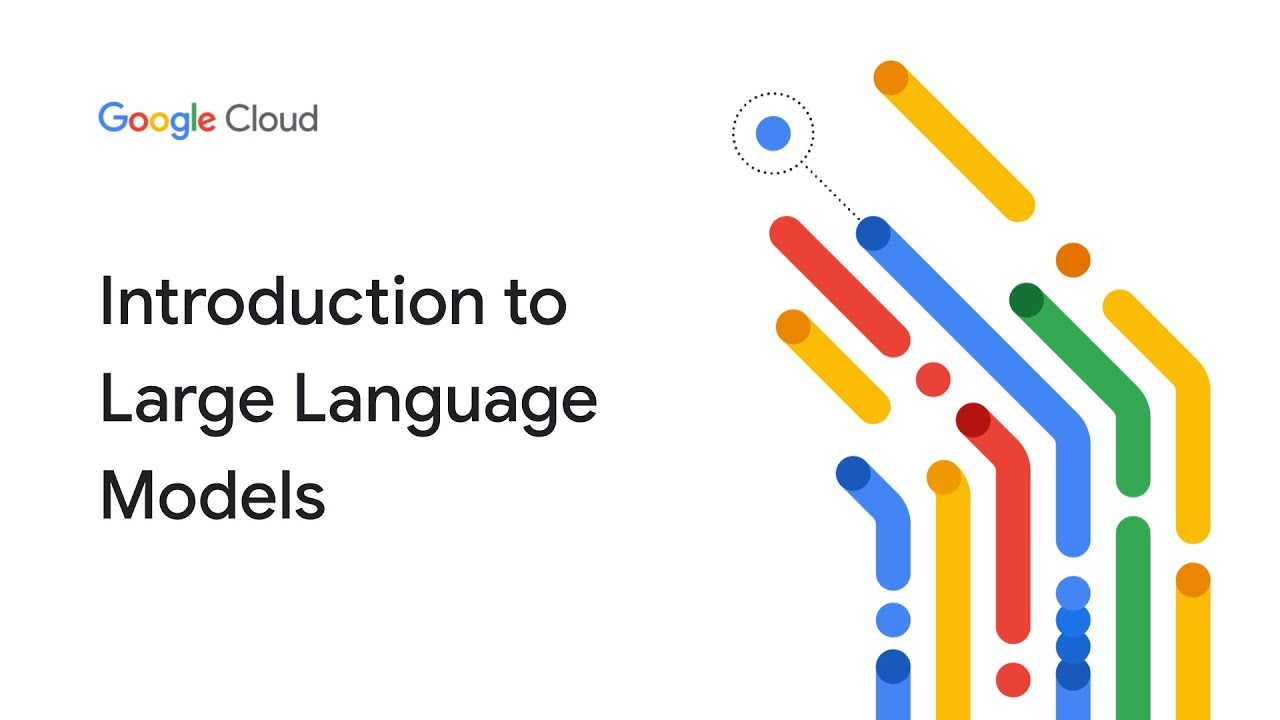
Introduction to large language models
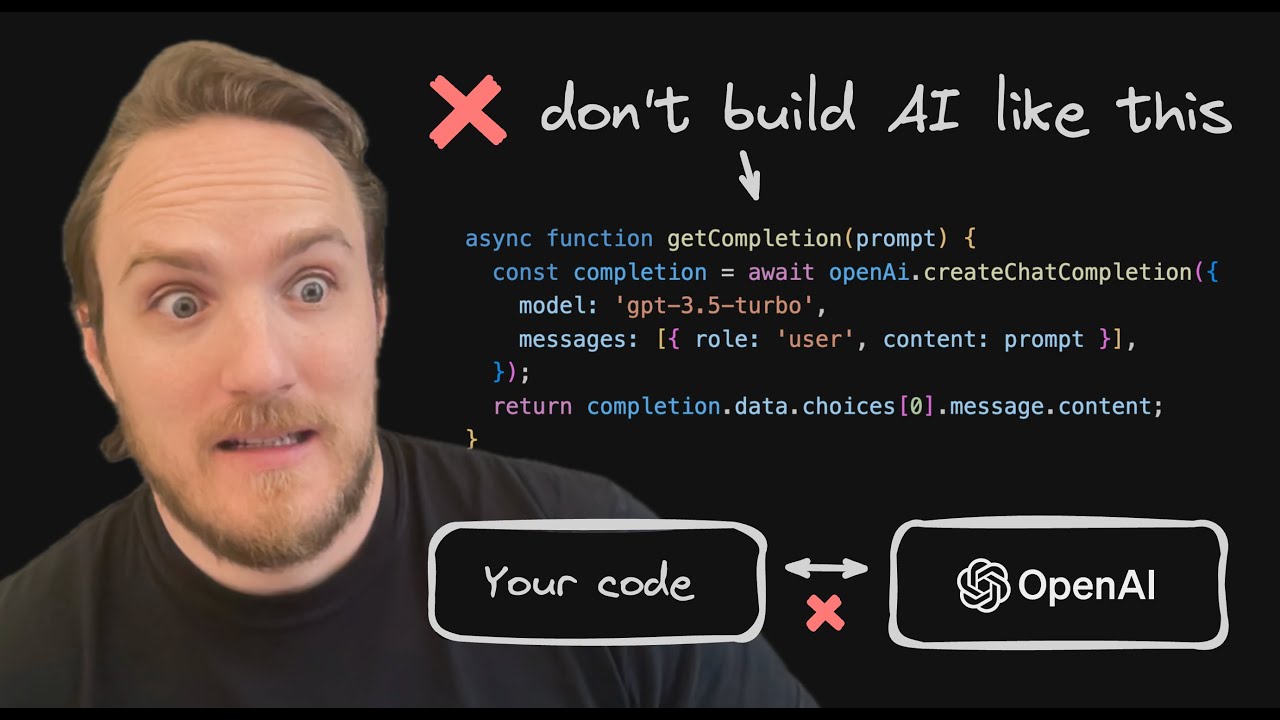
Don’t Build AI Products The Way Everyone Else Is Doing It
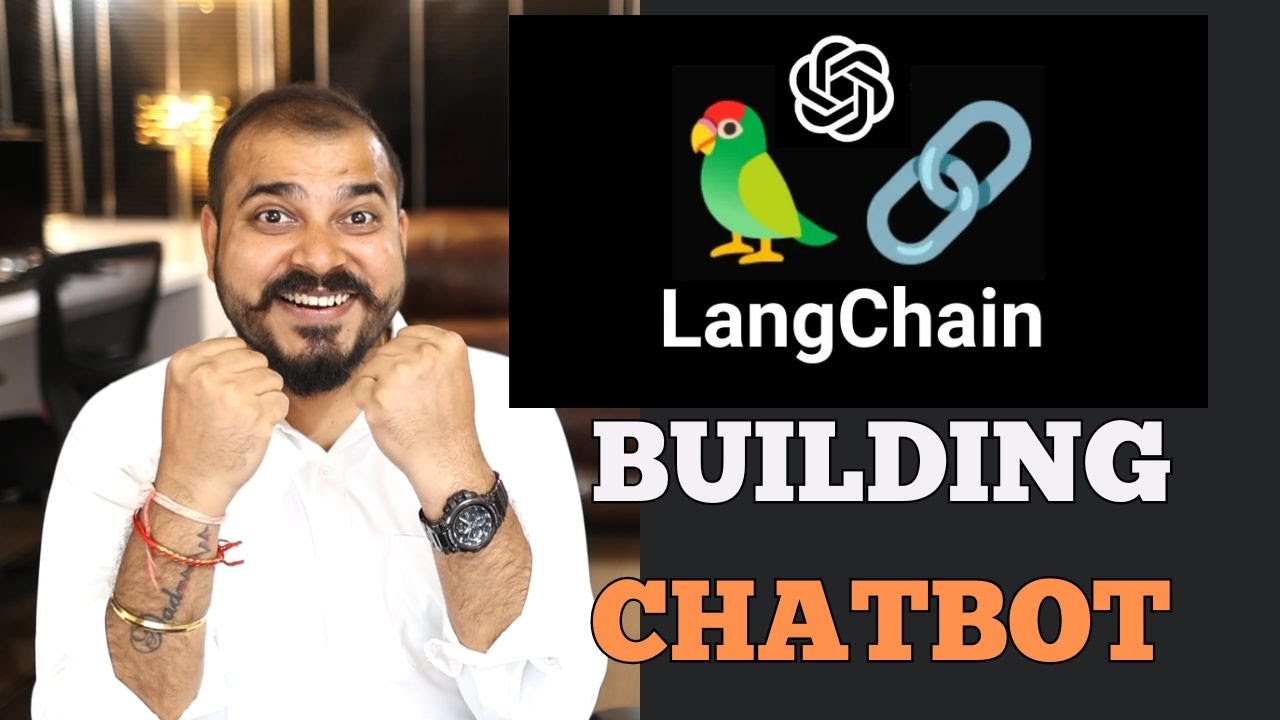
2-Langchain Series-Building Chatbot Using Paid And Open Source LLM's using Langchain And Ollama
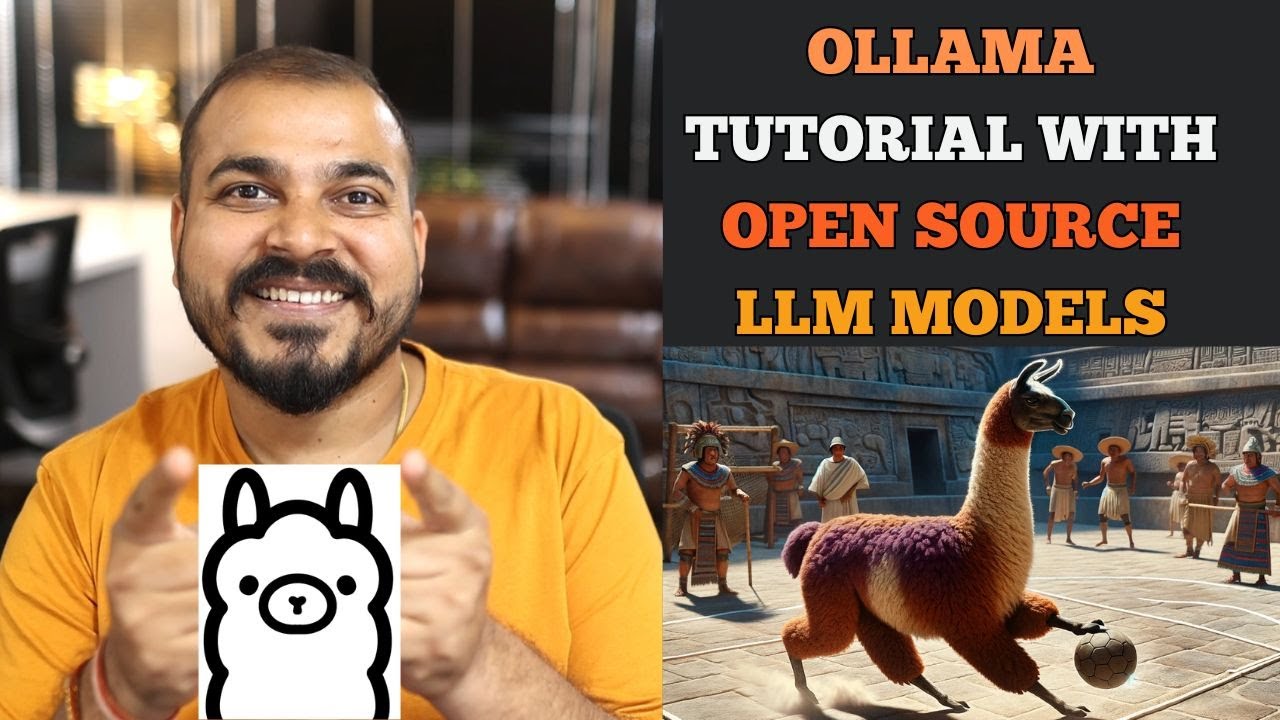
Ollama-Run large language models Locally-Run Llama 2, Code Llama, and other models
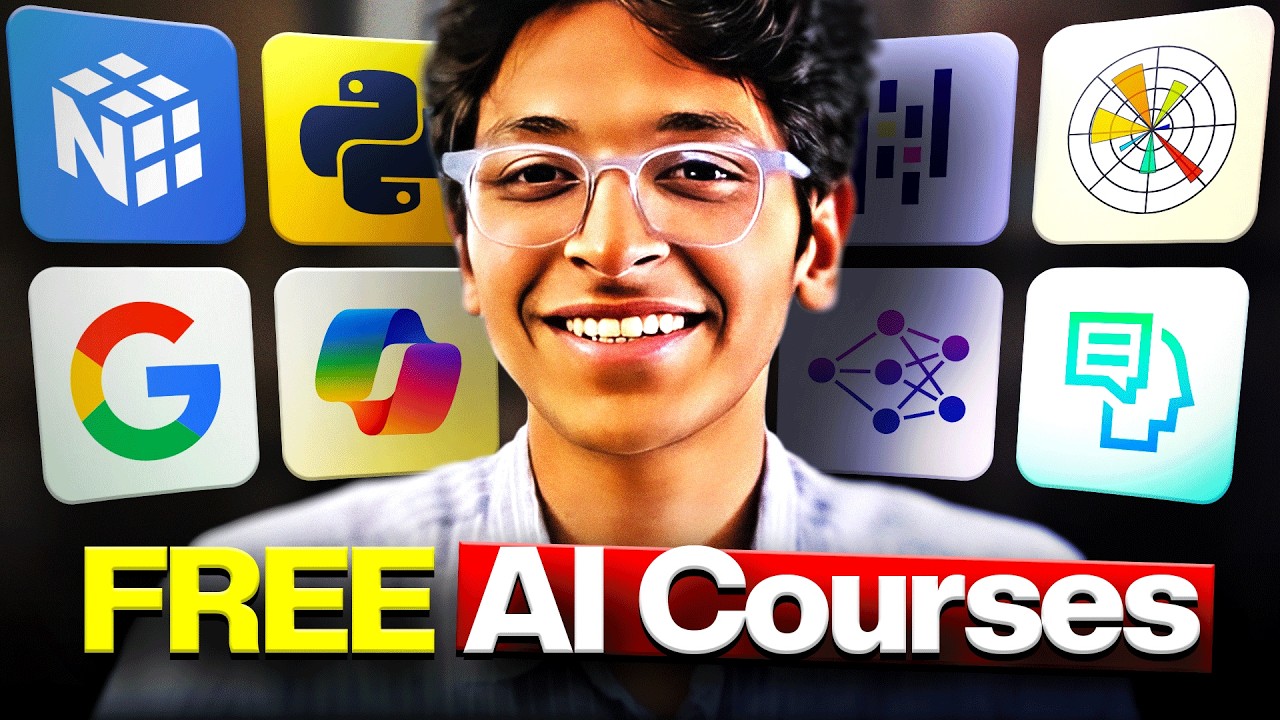
Best FREE AI Courses for Beginners in 13 Minutes 🔥| Become an AI Engineer in 2024
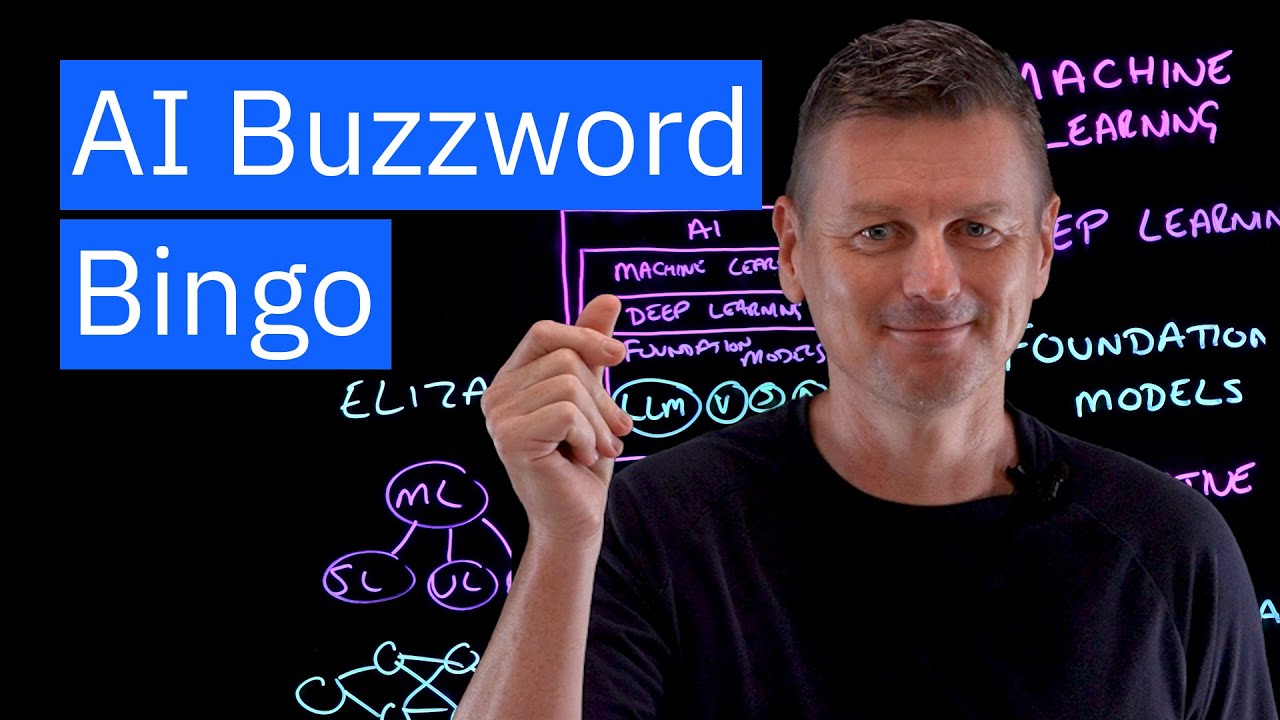
Machine Learning vs. Deep Learning vs. Foundation Models
5.0 / 5 (0 votes)