Data Quality Explained
Summary
TLDRThis video script discusses the pivotal role of data quality in business outcomes, using the analogy of a chef with poor ingredients. It emphasizes four key data qualities: accuracy, completeness, consistency, and uniqueness, explaining each with examples from a lead generation company. The script concludes by suggesting the use of machine learning and AI to automate the detection of these qualities, thereby saving time and reducing manual data inspection.
Takeaways
- đœïž Data quality is crucial for business outcomes, similar to how quality ingredients are essential for a chef's dishes.
- đ Poor data quality can negatively impact a company's reputation, just as bad ingredients can ruin a restaurant's reputation.
- đ Four main qualities of data include accuracy, completeness, consistency, and uniqueness.
- đŻ Accuracy refers to how well the data reflects the true state of reality, unaffected by anomalies like bot traffic.
- đ Completeness is about ensuring all required fields in a dataset are filled out, providing a full picture of the data.
- đ Consistency is about the uniformity of data across different sources to avoid mismatches that can lead to incomplete customer profiles.
- đ Uniqueness is tied to the absence of duplicates in a dataset, which can inflate the perceived volume of data.
- đ€ Machine learning and AI can be used to automatically detect these data qualities as they enter the system, reducing manual effort.
- đ The script suggests leveraging technology to automate the inspection of data quality, which can save time and improve efficiency.
- đšâđ» The speaker invites viewers to explore more about these features and subscribe to the channel for more insights on technology.
Q & A
What is the main impact of poor data quality on a business?
-Poor data quality can significantly affect business outcomes, causing a company's reputation to suffer, similar to how poor quality ingredients can ruin a chef's dishes and harm a restaurant's reputation.
What are the four main qualities of data that affect its quality?
-The four main qualities of data that affect its quality are accuracy, completeness, consistency, and uniqueness.
How is accuracy in data defined in the context of the script?
-Accuracy in data refers to how well the current state of the data matches reality. For example, if a lead generation company does not account for a spike in bot-generated traffic, the data will not accurately reflect reality.
What does completeness in data mean and why is it important?
-Completeness in data means that all required fields in a dataset are filled out. It is important because incomplete data can lead to an incomplete picture of customers or clients, which can affect business decisions.
Can you explain the concept of consistency in data as mentioned in the script?
-Consistency in data refers to the uniformity of the data set across different sources. If different teams within a company collect the same data in different formats, it can lead to mismatches and an incomplete customer profile when the data is pulled from various systems.
What is uniqueness in data, and how can it affect a lead generation company?
-Uniqueness in data pertains to the absence of duplicate entries within a dataset. For a lead generation company, having a high percentage of duplicate leads can result in an inflated lead count, which can misrepresent the actual number of unique prospects and potentially skew business performance metrics.
How can machine learning and AI help in managing data quality?
-Machine learning and AI can be leveraged to automatically detect and manage key data quality features such as accuracy, completeness, consistency, and uniqueness as data enters the system, which saves time and reduces the need for manual inspection.
What is the analogy used in the script to explain the importance of data quality?
-The analogy used in the script compares a chef with poor quality ingredients to a business with poor data quality, emphasizing that both can lead to a poor end product and damage to reputation.
Why is it crucial for a lead generation company to account for bot-generated traffic?
-It is crucial for a lead generation company to account for bot-generated traffic to ensure that the data reflects actual human users and not automated bots, as this can lead to inaccurate data and misinformed business decisions.
How can the lack of required fields in a survey campaign affect the data collected?
-If a survey campaign does not require certain fields to be filled out, it can result in a dataset with missing information, leading to an incomplete understanding of the respondents and potentially biased or skewed results.
What challenges does a company face when different teams collect the same data in different formats?
-When different teams collect the same data in different formats, it can lead to inconsistencies and difficulties in integrating the data. This can result in an incomplete or inaccurate customer profile and hinder the effectiveness of data-driven decision-making.
Outlines
đ The Impact of Data Quality on Business Outcomes
This paragraph emphasizes the critical role of data quality in determining business outcomes. It uses the analogy of a chef with poor-quality ingredients to illustrate how even the most skilled teams can produce subpar results if the data they rely on is inaccurate or incomplete. The paragraph introduces four key qualities of data: accuracy, completeness, consistency, and uniqueness. It then transitions into a discussion of these qualities through the lens of a lead generation company, highlighting the importance of each aspect for maintaining a positive business reputation and achieving desired outcomes.
Mindmap
Keywords
đĄData Quality
đĄAccuracy
đĄCompleteness
đĄConsistency
đĄUniqueness
đĄLead Generation
đĄMachine Learning
đĄAI (Artificial Intelligence)
đĄData Sources
đĄDuplicate Data
đĄManual Inspection
Highlights
Data quality significantly impacts business outcomes, analogous to a chef's ingredients affecting the final dish.
Poor data quality can tarnish a company's reputation, similar to a restaurant serving low-quality meals.
Data quality is influenced by factors such as source number and company size.
Four main qualities of data are accuracy, completeness, consistency, and uniqueness.
Accuracy refers to how well data reflects the real-world state, using bot traffic as an example.
Completeness is about filling out all required fields in a dataset, illustrated by a survey campaign.
Consistency ensures uniformity across different data sources, highlighted by zip code formatting discrepancies.
Uniqueness is tied to the absence of duplicate data, exemplified by duplicate leads in a lead generation context.
Manual inspection of data for quality is time-consuming and prone to errors.
Machine learning and AI can automatically detect key data quality features as data enters the system.
Leveraging technology saves time and reduces the need for manual data inspection.
The video provides insights into how to maintain and improve data quality within a lead generation company.
The importance of a holistic approach to data quality is emphasized, covering all four main qualities.
The analogy of a chef and ingredients is used to explain the impact of data quality on business outcomes.
The video suggests that neglecting data quality can lead to an incomplete picture of customer profiles.
The necessity of addressing data quality issues to maintain a positive company image is discussed.
The video concludes by encouraging viewers to explore the provided links for more information on data quality features.
Transcripts
Your company generates lots of data, but the business outcomes you gain from that data can Â
be largely affected by data quality. To use an analogy, imagine you're a chef and you have Â
the highest accolades in the industry, a highly experienced team, but when the ingredients come Â
in, those are poor quality ingredients. Picture rotten tomatoes, rotten onions. So when you go Â
and make those entrees, the end result is poor quality and your restaurant reputation suffers. Â
This is the same impact that poor data quality can have on your business, causing your company's Â
reputation to suffer as a result. There are a lot of different factors that can impact data quality, Â
such as the number of sources or the size of your company. But today, I want to talk about Â
four main qualities within data itself. Accuracy, completeness, consistency and uniqueness. And I'm Â
going to talk about them through the lens of a lead generation company. Starting with accuracy. Â
Accuracy is about the current state of your data versus reality. So for my lead generation company, Â
imagine I'm driving traffic to a website and all of a sudden I get a sudden spike in usage from Â
bots that had the click generation. If I don't account for this spike when I go and pull that Â
data, at the end of the day, it's not going to reflect reality, so it's not going to be accurate. Â
Next, I want to talk about completeness, which is about how you have filled out all the Â
required fields in your dataset. So let's say I'm launching a survey campaign. And I'm collecting Â
names and email addresses, but I don't require this field. So when I go and pull that data, Â
I notice that some of my participants didn't put their name, Some of my participants didn't put Â
their email. So when I go and pull that picture of the client of the customer, I have an incomplete Â
dataset and incomplete picture. Next we talk about consistency, which is about how uniform your data Â
set is throughout different data sources. So back to my lead generation example. Let's say Â
I'm driving traffic for a Dropshipping campaign and I have my procurement team collecting zip Â
codes and my marketing team collecting zip codes, but my procurement team is looking at them in Â
a five digit format while my marketing team is collecting them in a nine digit format. Â
When I go tap into both of these databases and pull the customer profile, Â
it might be incomplete because those zip codes don't match up throughout my systems. And lastly, Â
there's uniqueness, which is largely tied to the number of duplicates I have in a dataset. Â
So in my lead generation context, you can imagine having 50,000 leads at the end of the year. Â
But when I actually go into those leads, I realize that 20% our duplicates from customers who filled Â
out the information previously. So now when I go and pull that report, I actually have 20% less Â
data and a lot less positive looking picture for my company. So looking at these aspects, Â
it's easy to think, Wow, there's a lot of manual inspection here. How can I go through all of Â
my data and understand these resources, these qualities? Right. Well, you can actually leverage Â
machine learning and AI to automatically sense these key features as data enters your system, Â
saving you time and manual inspection. If you're curious about these features, check out the links Â
below. And if you're curious about technology, subscribe to the channel. Thank you.
Voir Plus de Vidéos Connexes
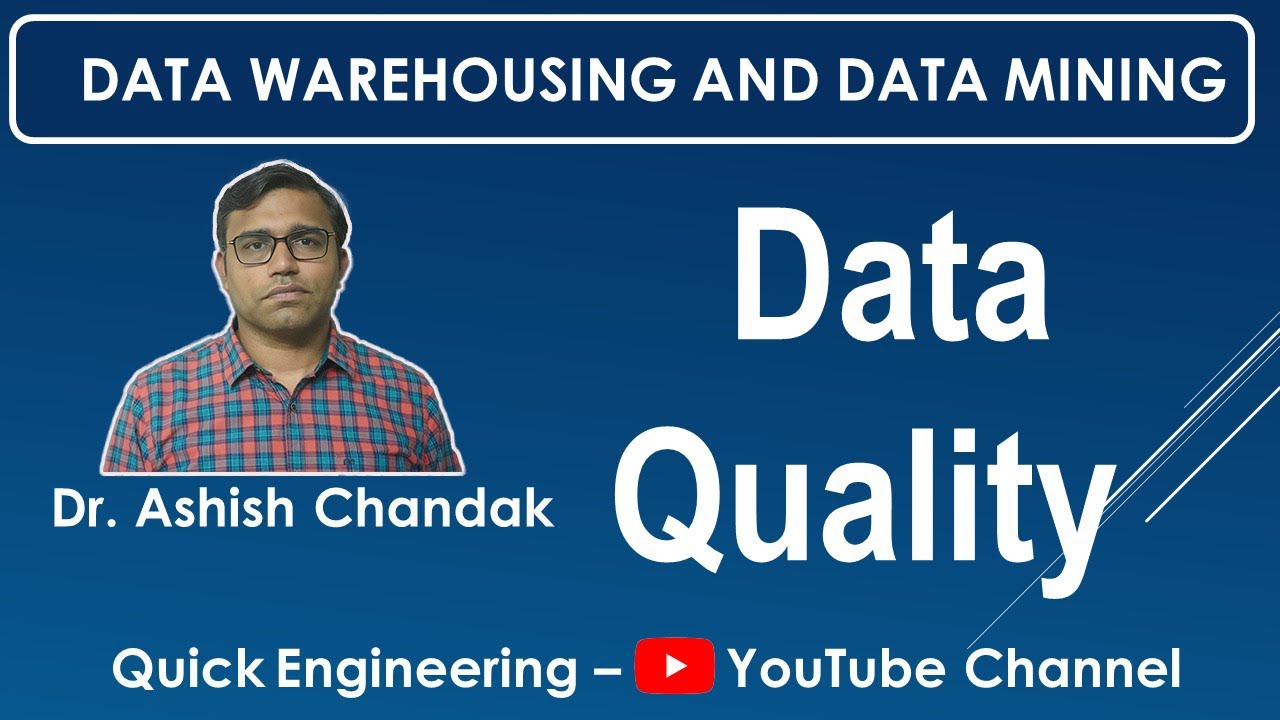
Data Quality | Data Warehousing and Data Mining | Quick Engineering | Ashish Chandak
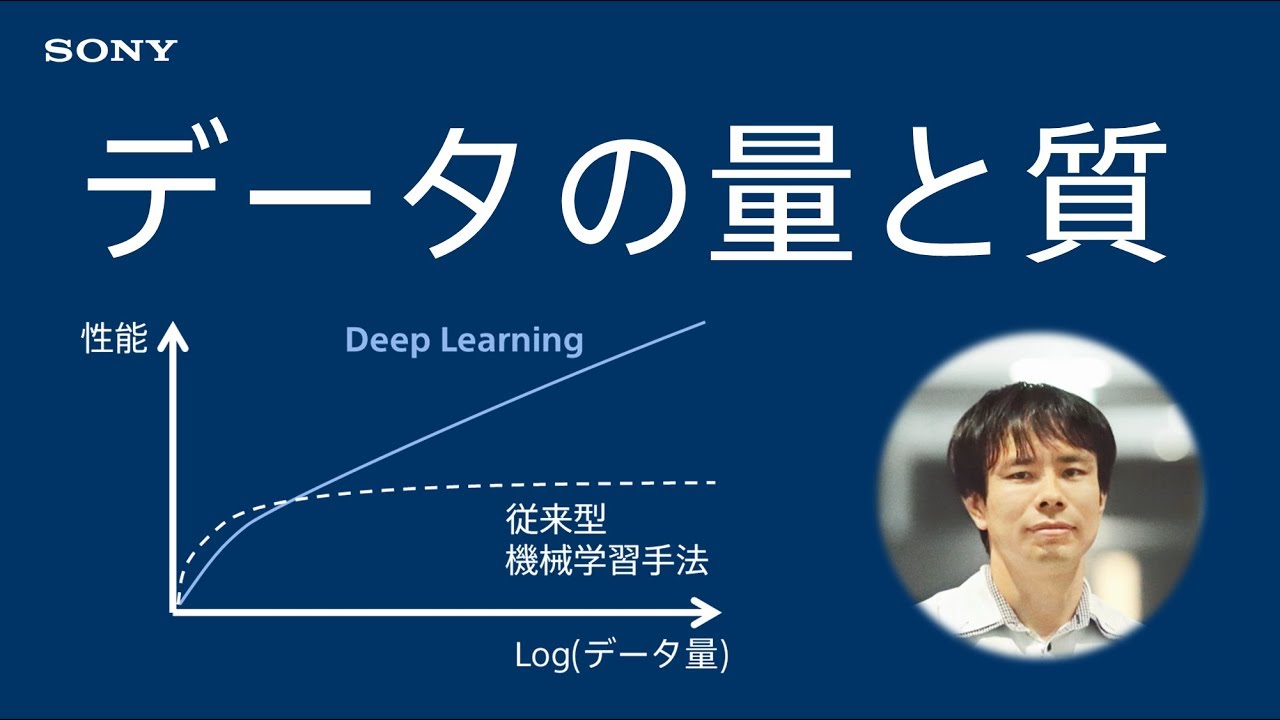
ăă„ăŒă©ă«ăăăăŻăŒăŻăźæ§èœăæ±șćźă„ăăăăŒăżăźéăšèłȘ
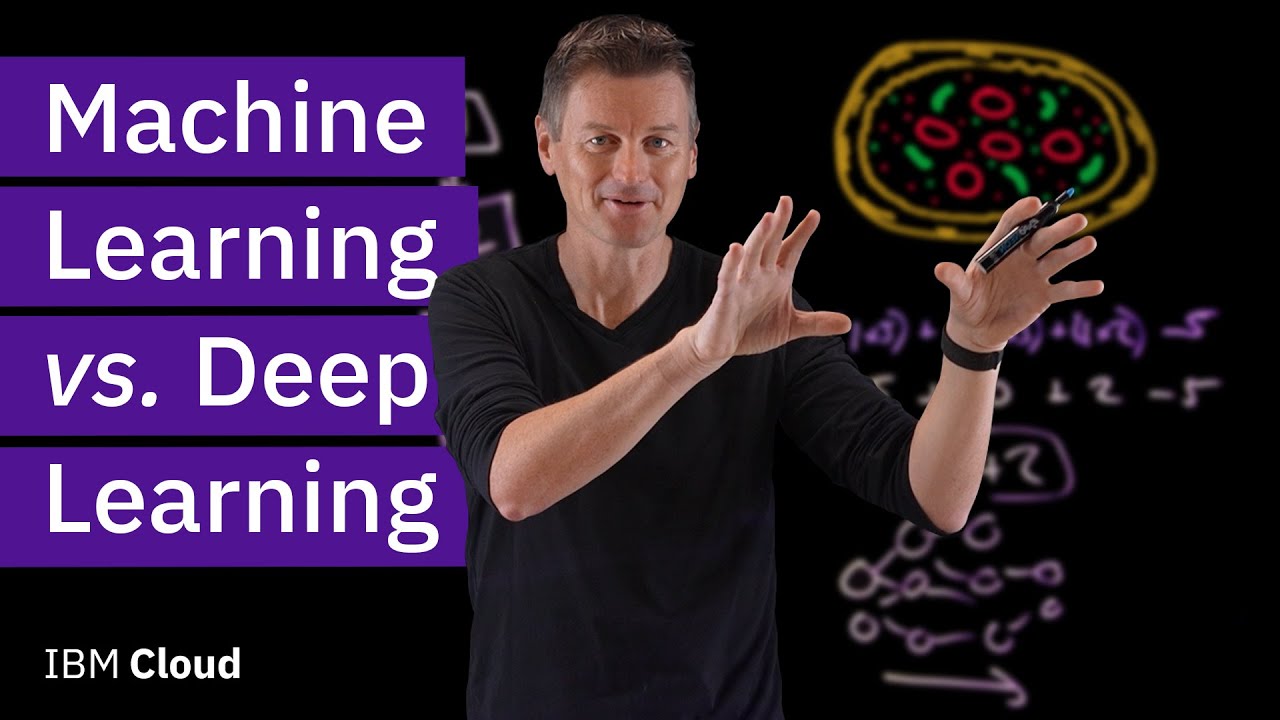
Machine Learning vs Deep Learning
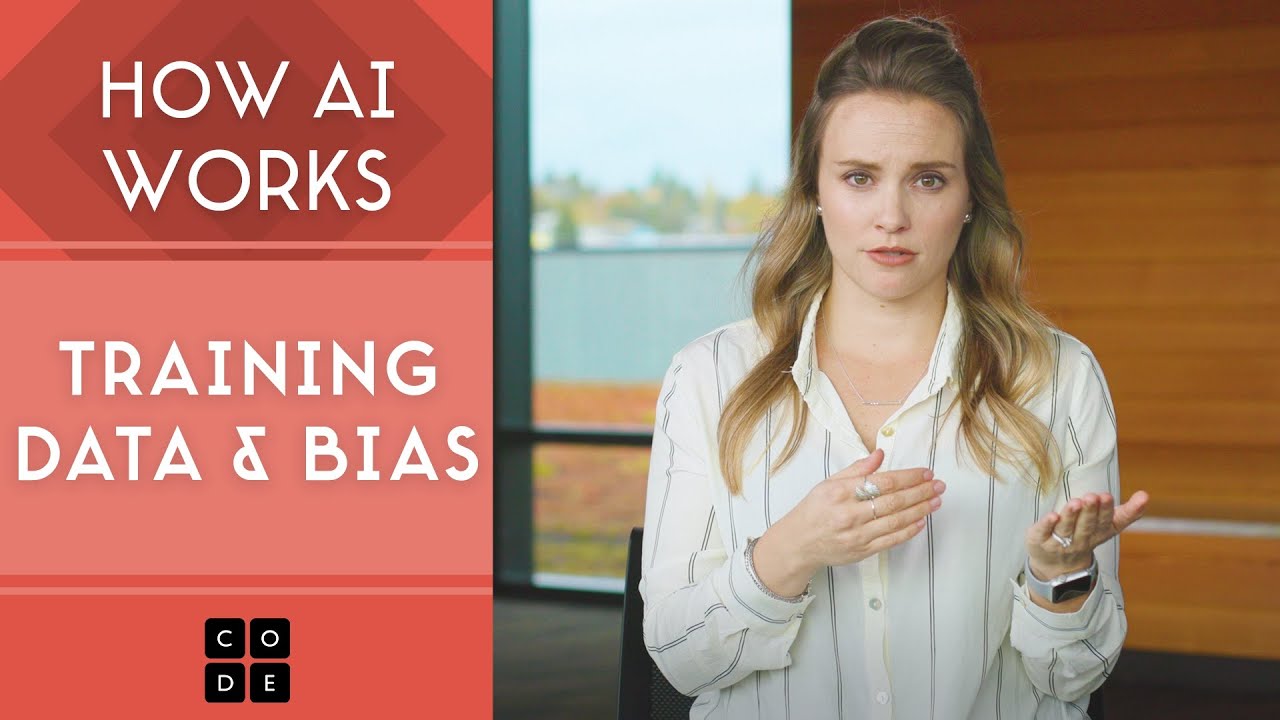
AI: Training Data & Bias
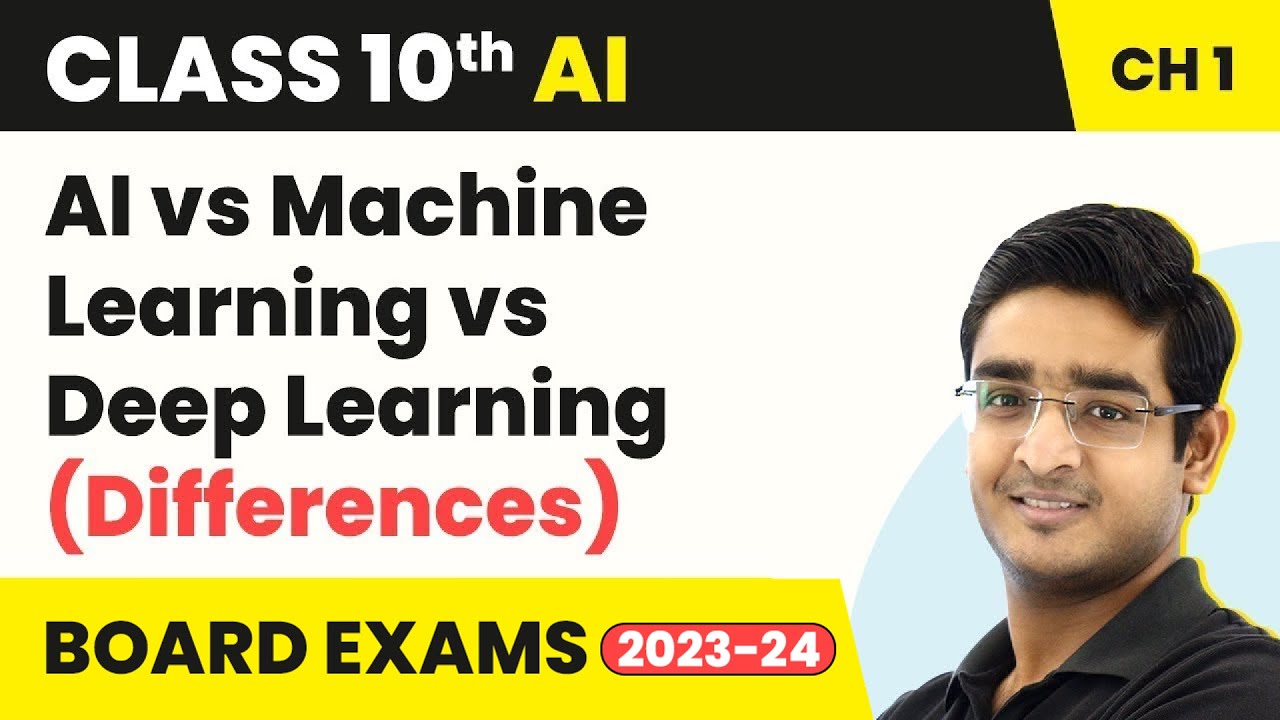
Artificial Intelligence Class 10 Ch 1 |AI vs Machine Learning vs Deep Learning (Differences) 2022-23
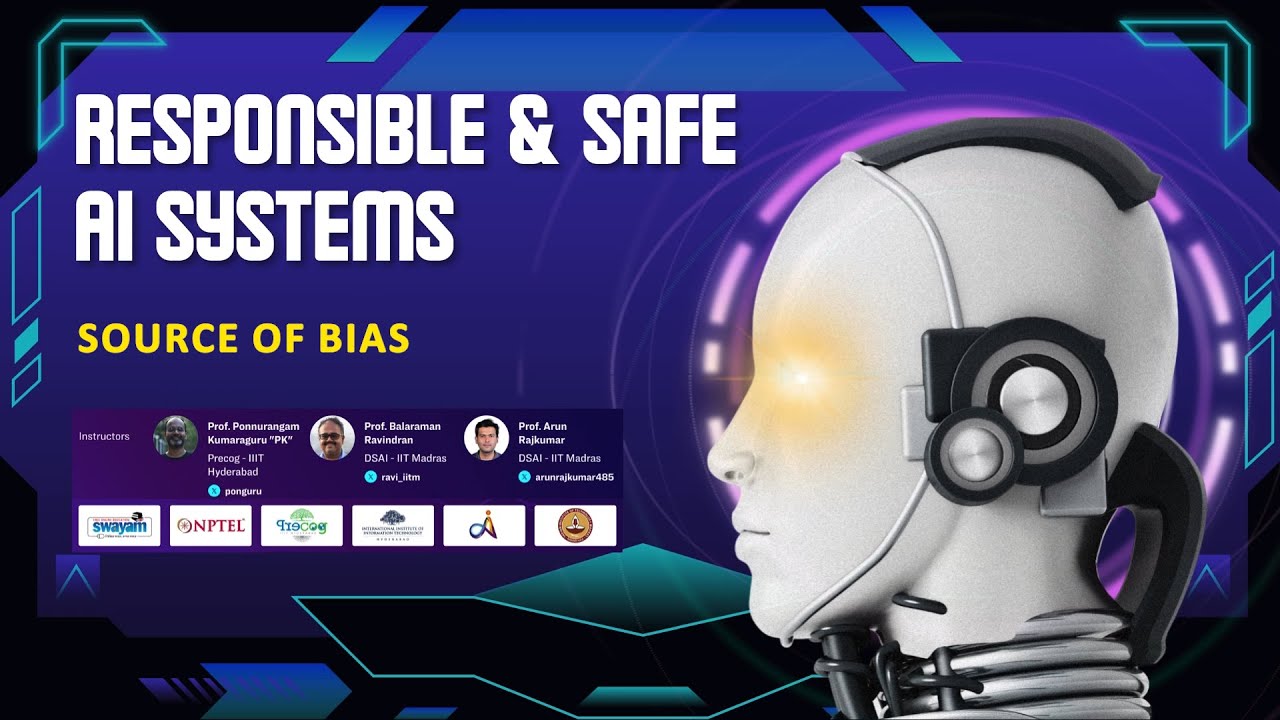
Source of Bias
5.0 / 5 (0 votes)