The Singularity Is Nearer featuring Ray Kurzweil | SXSW 2024
Summary
TLDR在这段对话中,Ray Kurzweil,一位在人工智能领域工作超过六十一年的科学家,分享了他对人工智能未来发展的深刻见解。Kurzweil预测,到2029年,计算机将通过图灵测试,而到了2045年,我们将达到技术奇点,届时人类将能够备份大脑,实现思维的数字化。他讨论了大型语言模型的快速发展,以及它们在创造新知识和解决问题方面的潜力。此外,Kurzweil还探讨了纳米机器人和脑机接口技术的重要性,以及这些技术可能带来的伦理挑战。他认为,尽管存在风险,但技术的进步将继续是人类发展的积极力量,并鼓励年轻人追随自己的激情,为创造一个更加智能和平等的未来做出贡献。
Takeaways
- 🧠 我们正在经历AI领域的快速发展,特别是在大型语言模型方面,这些模型的发展速度甚至超出了一些专家的预期。
- ⚙️ 大型语言模型和AI系统的发展并不完全依赖于对人类大脑工作原理的理解,而是更多地依赖于计算能力和连接的数量。
- 📈 库兹韦尔曲线(Kurzweil Curve)展示了技术进步的指数增长,这解释了为何大型语言模型在近期变得可行。
- 🧪 通过模拟生物过程,AI已经能够创造出新的医疗解决方案,如Moderna疫苗,这展示了AI在创造性任务中的潜力。
- 🤖 纳米机器人和脑机接口是实现技术奇点(singularity)的另外两个关键技术,尽管它们的发展速度慢于AI。
- 🧵 脑机接口和纳米机器人的发展速度受到人体试验伦理和安全性考量的限制。
- 💡 技术进步,包括AI和纳米技术,将继续推动经济增长和社会平等。
- 🔮 技术奇点预计将在2045年实现,届时人类将能够备份和恢复大脑的完整内容。
- 🧐 尽管我们对未来的预测存在不确定性,但历史表明,技术进步带来的利益通常超过风险。
- 👴 随着寿命的延长,人们对于生活规划和职业发展的观念将发生根本性变化,退休年龄可能会变得不那么重要。
- 🌐 随着技术的发展,人们对于工作的看法将转变,创造性和个人兴趣将成为选择职业的重要考量因素。
Q & A
Ray Kurzweil 在人工智能领域工作了多久?
-Ray Kurzweil 在人工智能领域工作了61年。
Kurzweil 预测的图灵测试将会在何时实现?
-Kurzweil 在1999年预测,计算机将在2029年通过图灵测试。
Kurzweil 对于意识的看法是什么?
-Kurzweil 认为意识虽然非常重要,但它不是一个科学概念,因为我们无法证明一个实体是否具有意识。
Kurzweil 认为人类的情感和感觉能否被计算和数学所捕捉?
-Kurzweil 认为人类的情感和感觉,特别是与人相爱时的行为和感觉,可以依赖于连接模式,因此可以被计算和数学所捕捉。
Kurzweil 提到的长寿逃逸速度是什么意思?
-长寿逃逸速度是指通过科学进步,人们每年因年龄增长而损失的健康时间将被科学进步所补偿,甚至超过,从而实现寿命的延长。
Kurzweil 预测的奇点(singularity)将在何时到来?
-Kurzweil 预测奇点将在2045年到来,届时技术将达到一个临界点,人类的生活将会发生翻天覆地的变化。
Kurzweil 如何看待人类与机器的结合对伦理的挑战?
-Kurzweil 认为人类与机器的结合将带来伦理挑战,例如权力分配、才能的定义以及个体的公平性等问题,但他相信这些问题可以通过人类的创造力和合作来解决。
Kurzweil 对于人类未来是否应该专注于他们的热情而非仅仅是收入的看法是什么?
-Kurzweil 建议年轻人应该追求他们的热情所在,因为未来将有更多的机会和可能性,而不仅仅是追求经济收入。
Kurzweil 认为大型语言模型的快速发展是否令人惊讶?
-Kurzweil 表示他对于大型语言模型的快速发展感到有些惊讶,因为他预计这些进展会发生在几年后,而不是当前。
Kurzweil 如何看待人类与机器的融合对个体独特性的影响?
-Kurzweil 认为即使人类与机器融合,分享记忆和信息,个体仍然是独特的,因为每个人都有不同的兴趣和选择。
Kurzweil 对于人工智能的未来发展有哪些担忧?
-Kurzweil 担忧人工智能的快速发展可能带来的风险,例如纳米技术可能被滥用造成灾难,但他也相信我们将开发出能够预防这些风险的技术。
Outlines
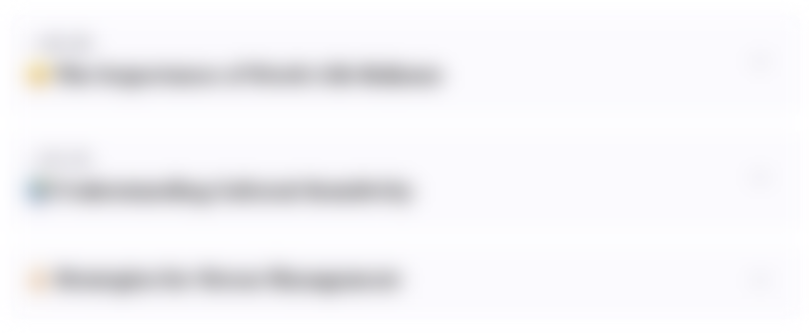
Cette section est réservée aux utilisateurs payants. Améliorez votre compte pour accéder à cette section.
Améliorer maintenantMindmap
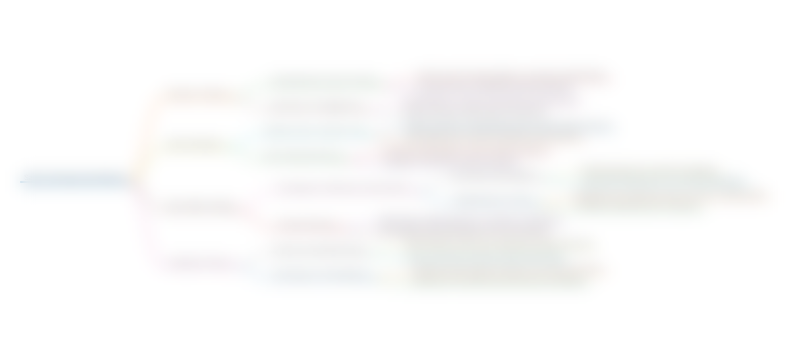
Cette section est réservée aux utilisateurs payants. Améliorez votre compte pour accéder à cette section.
Améliorer maintenantKeywords
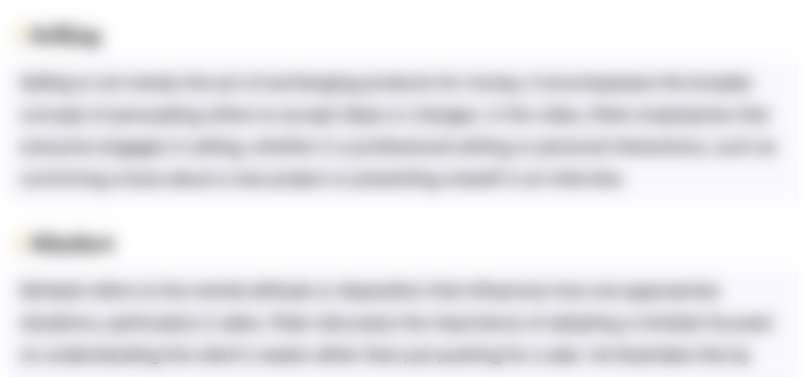
Cette section est réservée aux utilisateurs payants. Améliorez votre compte pour accéder à cette section.
Améliorer maintenantHighlights
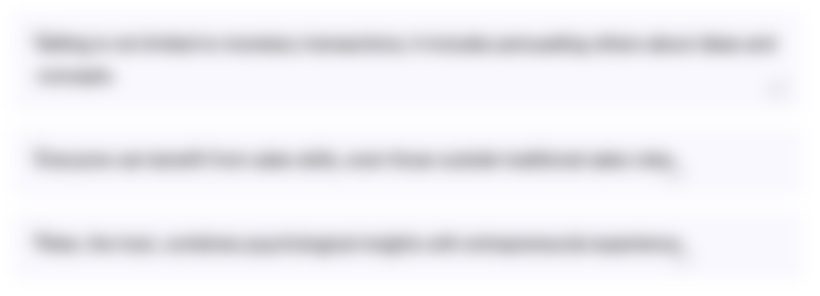
Cette section est réservée aux utilisateurs payants. Améliorez votre compte pour accéder à cette section.
Améliorer maintenantTranscripts
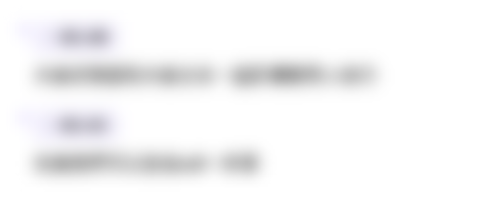
Cette section est réservée aux utilisateurs payants. Améliorez votre compte pour accéder à cette section.
Améliorer maintenantVoir Plus de Vidéos Connexes
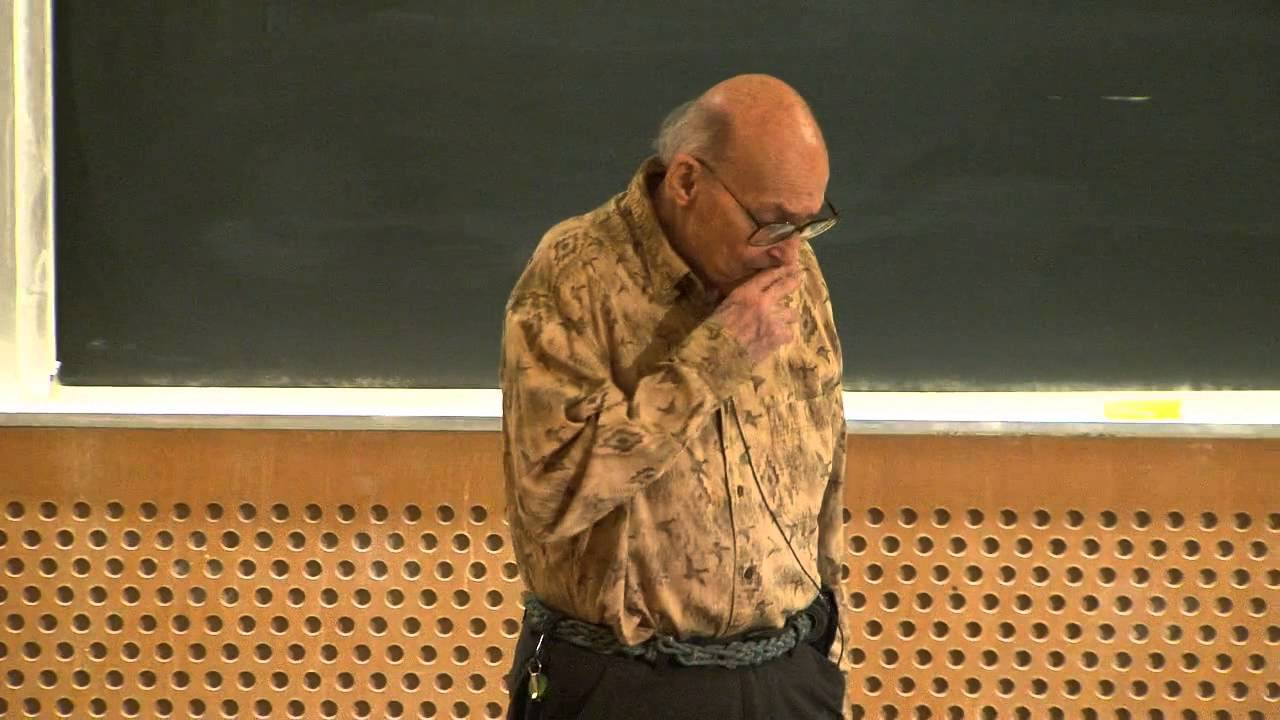
6. Layers of Mental Activities
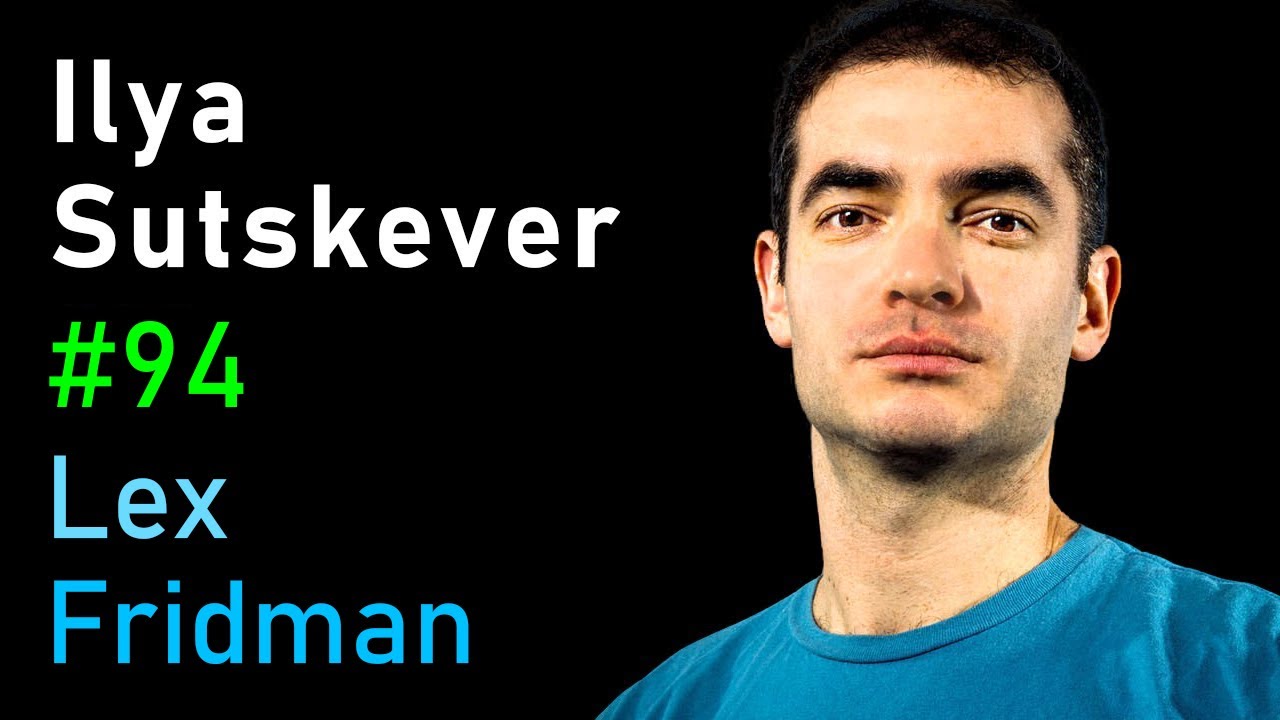
Ilya Sutskever: Deep Learning | Lex Fridman Podcast #94
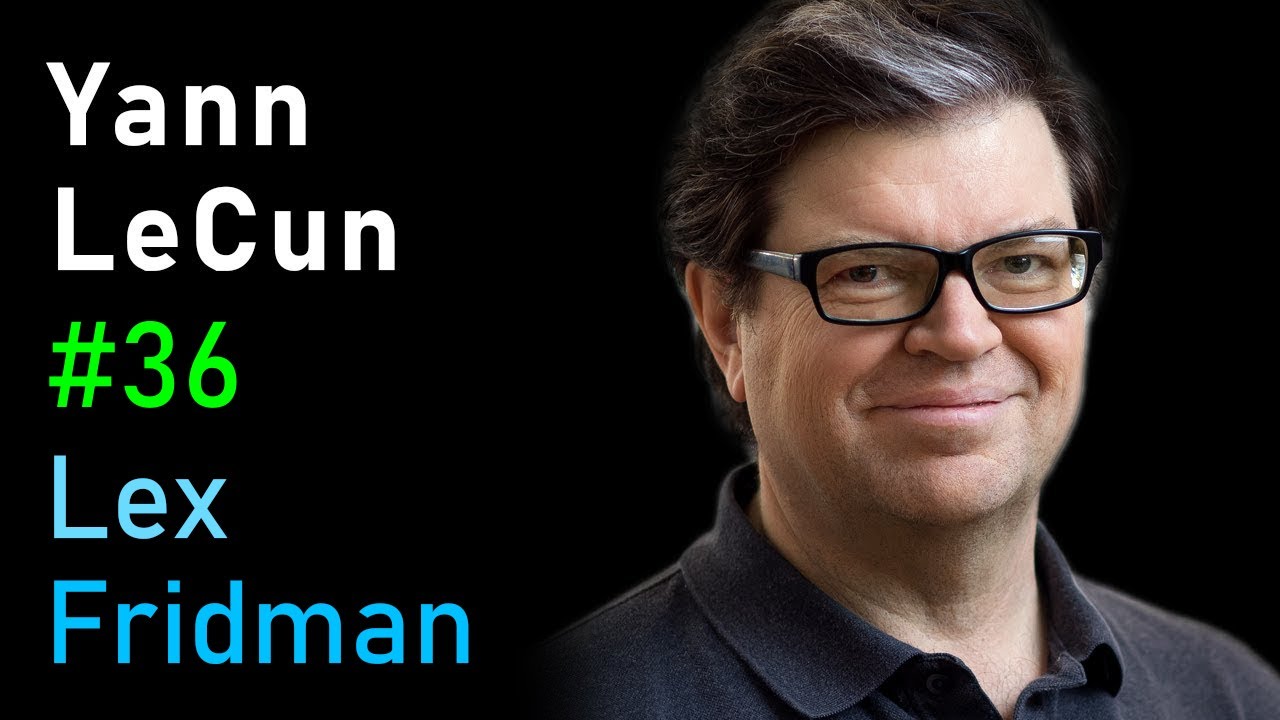
Yann LeCun: Deep Learning, ConvNets, and Self-Supervised Learning | Lex Fridman Podcast #36

8. Question and Answer Session 2
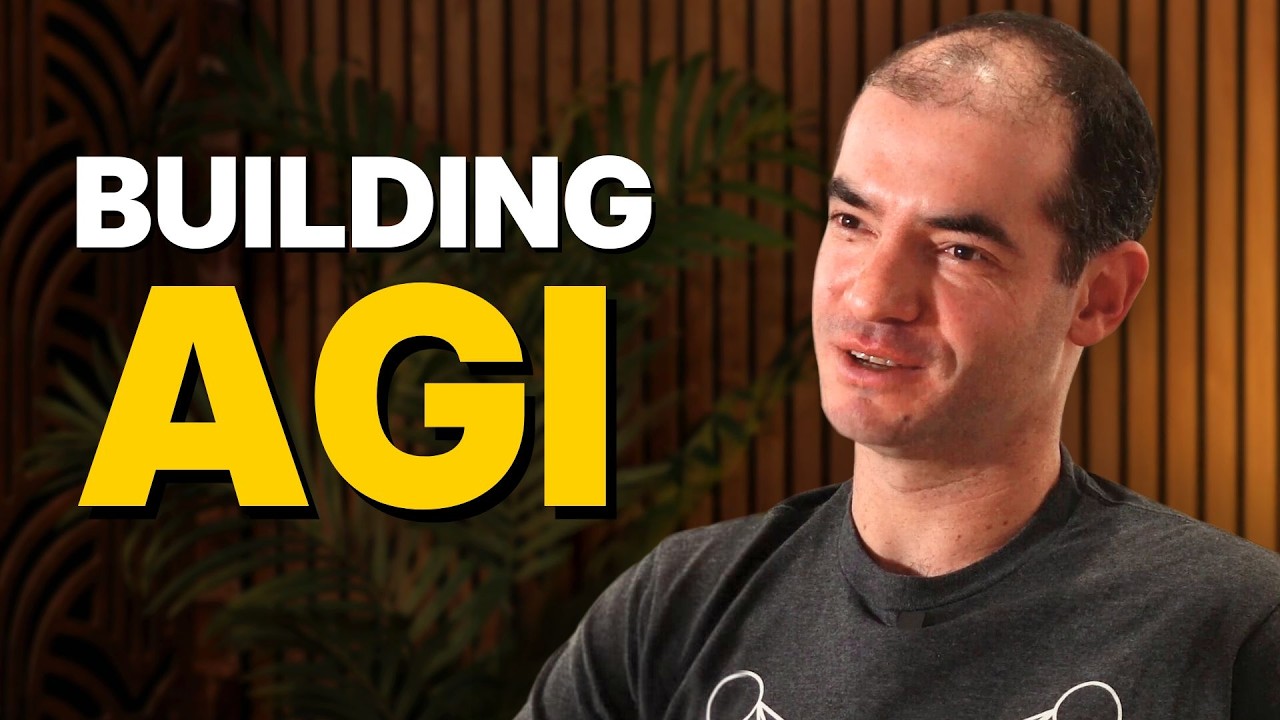
Ilya Sutskever (OpenAI Chief Scientist) - Building AGI, Alignment, Spies, Microsoft, & Enlightenment
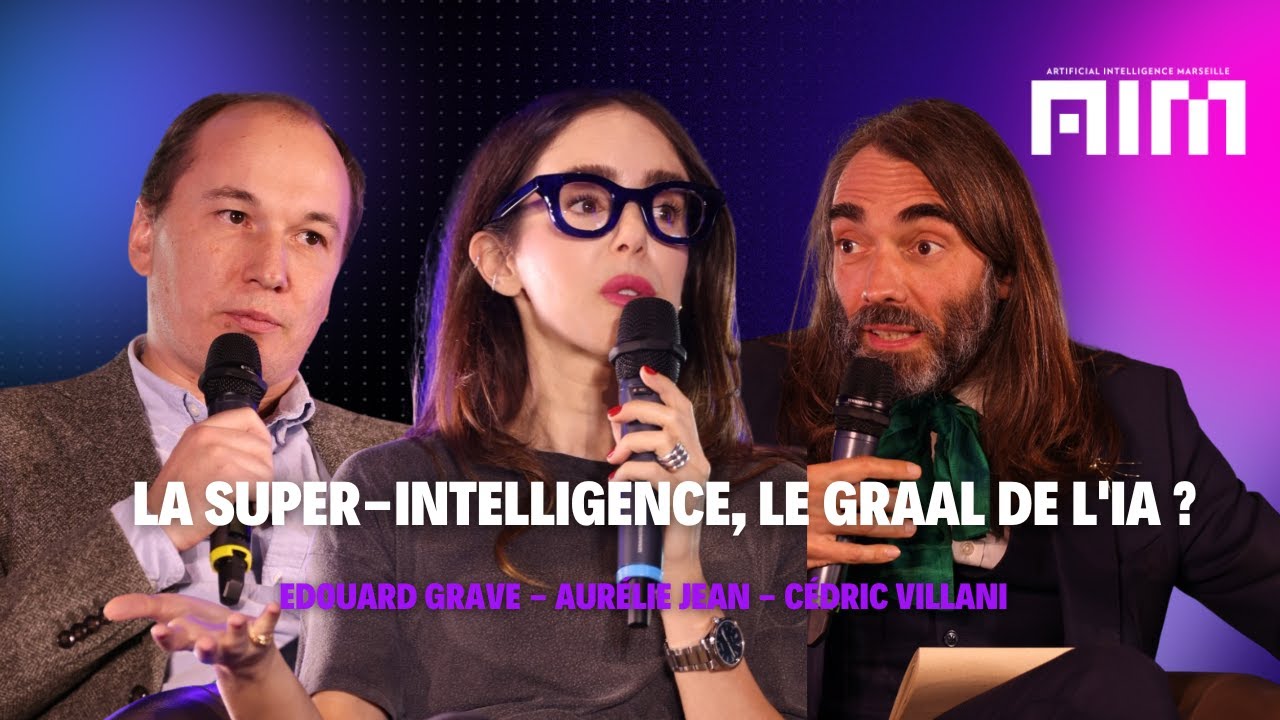
La super-intelligence, le Graal de l'IA ? | Artificial Intelligence Marseille
5.0 / 5 (0 votes)