Text to Video: The Next Leap in AI Generation
Summary
TLDRThe podcast features a discussion on the development of Stable Video Diffusion, an open-source generative video model that transforms images into short video clips. Researchers Andreas Blatman and Robin Rombach, from Stability AI, delve into the challenges of video generation, such as computational demands and data set selection, and the potential for future innovation with the model. They highlight the importance of diffusion models in visual media and the impact of open-sourcing on the AI research community, emphasizing the collaborative nature of the field.
Takeaways
- 🌟 Introduction to Stable Video Diffusion: A state-of-the-art, open-source generative video model that generates short video clips from images.
- 🤖 Background on Stable Diffusion: A text-to-image generative model that has gained significant popularity and success since its release.
- 🧠 The Challenge of Text-to-Video AI: Video generation is more complex due to the need for dynamic representation, including physics of movement and 3D objects.
- 🚀 Prioritizing Innovation: The decision to focus on stable video was driven by the challenge it presents and the potential for computational constraints to drive innovation.
- 🧬 The Evolution of Generative Models: Diffusion models have become the go-to for visual media, favoring perceptually important details and offering iterative refinement.
- 🔍 The Role of Open Sourcing: The open-source release of stable diffusion has led to a thriving ecosystem and extensive research building upon the model.
- 🎥 The Future of Video Editing: Insights into how the video editor of the future might look, incorporating AI and new technologies for content creation.
- 🌐 The Impact of AI on Creativity: The potential for AI to enable personalized content creation and enhance user experiences in video generation.
- 🚧 Infrastructure Challenges: The need for improved infrastructure to handle the computational demands of training and processing large video models.
- 🔗 Collaboration and Competition: The importance of maintaining a balance between competitive research and collaborative contributions to the AI community.
Q & A
What is stable diffusion?
-Stable diffusion is a text-to-image generative model that takes a text prompt and generates an image based on that input. It is particularly known for its efficiency and the quality of the images it produces.
Why was stable video a priority for the research team?
-The research team prioritized stable video because it presents a significant challenge due to the additional temporal dimension. Solving video generation requires the model to understand physical properties of the world, making it a next-level computational demand and an exciting problem to tackle.
What are the main differences between diffusion models and autoregressive models?
-Diffusion models differ from autoregressive models in that they don't represent data as a sequence of tokens. They are based on a pixel grid, which is beneficial for visual media like images and videos. They also focus on perceptually important details and use an iterative refinement process, gradually transforming noise to data rather than generating data token by token.
How does one-step sampling in diffusion models benefit creators and users?
-One-step sampling allows creators and users to see the image generation process in real-time as they type in their prompts. This immediate feedback enhances the user experience and can lead to faster and higher quality image production.
What were some of the surprising developments in image models that the researchers did not expect?
-The researchers were surprised by the significant improvements in performance, text understanding, and spatial compositionality of the models. They did not expect the level of detail and quality that could be achieved by simply typing in a single prompt.
How did the open-sourcing of stable diffusion impact the research ecosystem?
-Open-sourcing stable diffusion led to an explosion of research and development around the model. It provided a set of building blocks for developers and creators to experiment with, leading to innovative applications and improvements that might not have been possible with a closed model.
What are some of the infrastructure challenges in training video models?
-Training video models presents challenges such as scaling the dataset and data loading, dealing with higher GPU memory consumption, and building efficient data pipelines for video. These challenges are compounded by the additional computational work required to transform video data into a suitable input for the generative model.
How did the researchers approach selecting the data set for their video model?
-The researchers divided the training process into three stages. They first trained an image model, then trained on a large dataset to incorporate temporal dimensionality and motion, and finally refined the model on a high-quality, smaller dataset. This approach allowed them to filter out unwanted elements like text in the videos and focus on object and camera motion.
What is the role of luras in the architecture of generative models?
-Luras, or lightweight adapters, are fine-tuned onto an existing base model to adapt the attention layers. They allow the model to incorporate different properties, such as understanding various camera motions, in a lightweight and efficient manner.
What are the future directions the researchers see for generative video models?
-The researchers see future directions in generating longer videos, improving processing for longer videos, and enhancing coherence in generated content. They also envision integrating multimodality, such as adding audio tracks to videos, and increasing the speed of video generation to unlock more exploration and creativity.
What are the biggest open challenges in video generation that the researchers want to prioritize next?
-The biggest open challenges include generating longer, more coherent video content, improving the speed of video generation and processing, and potentially adding multimodal elements like audio to enhance the generated videos.
Outlines
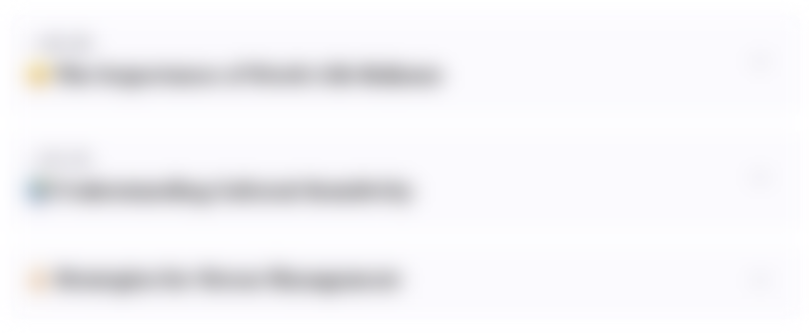
Cette section est réservée aux utilisateurs payants. Améliorez votre compte pour accéder à cette section.
Améliorer maintenantMindmap
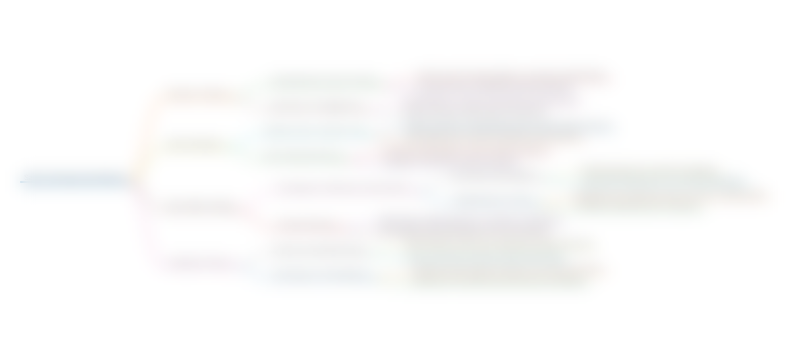
Cette section est réservée aux utilisateurs payants. Améliorez votre compte pour accéder à cette section.
Améliorer maintenantKeywords
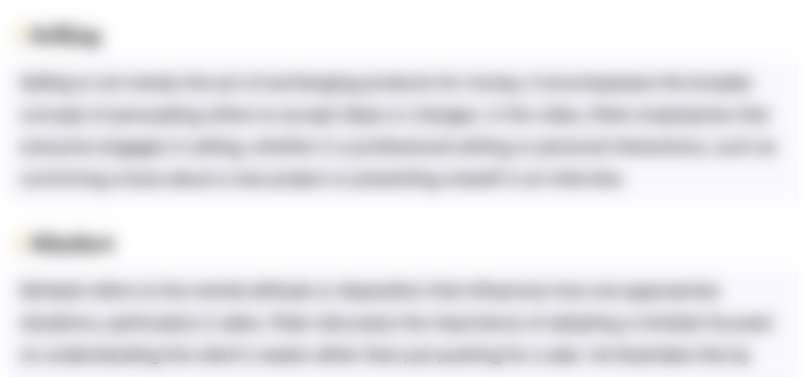
Cette section est réservée aux utilisateurs payants. Améliorez votre compte pour accéder à cette section.
Améliorer maintenantHighlights
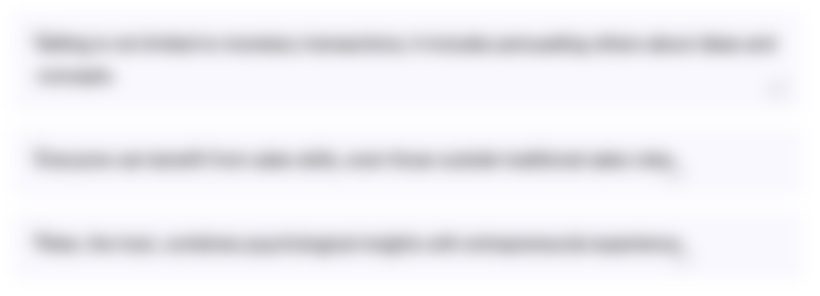
Cette section est réservée aux utilisateurs payants. Améliorez votre compte pour accéder à cette section.
Améliorer maintenantTranscripts
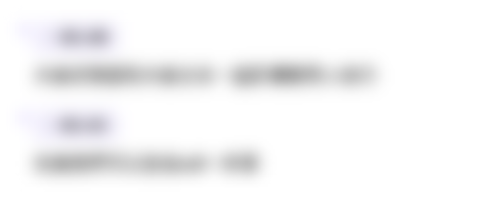
Cette section est réservée aux utilisateurs payants. Améliorez votre compte pour accéder à cette section.
Améliorer maintenantVoir Plus de Vidéos Connexes
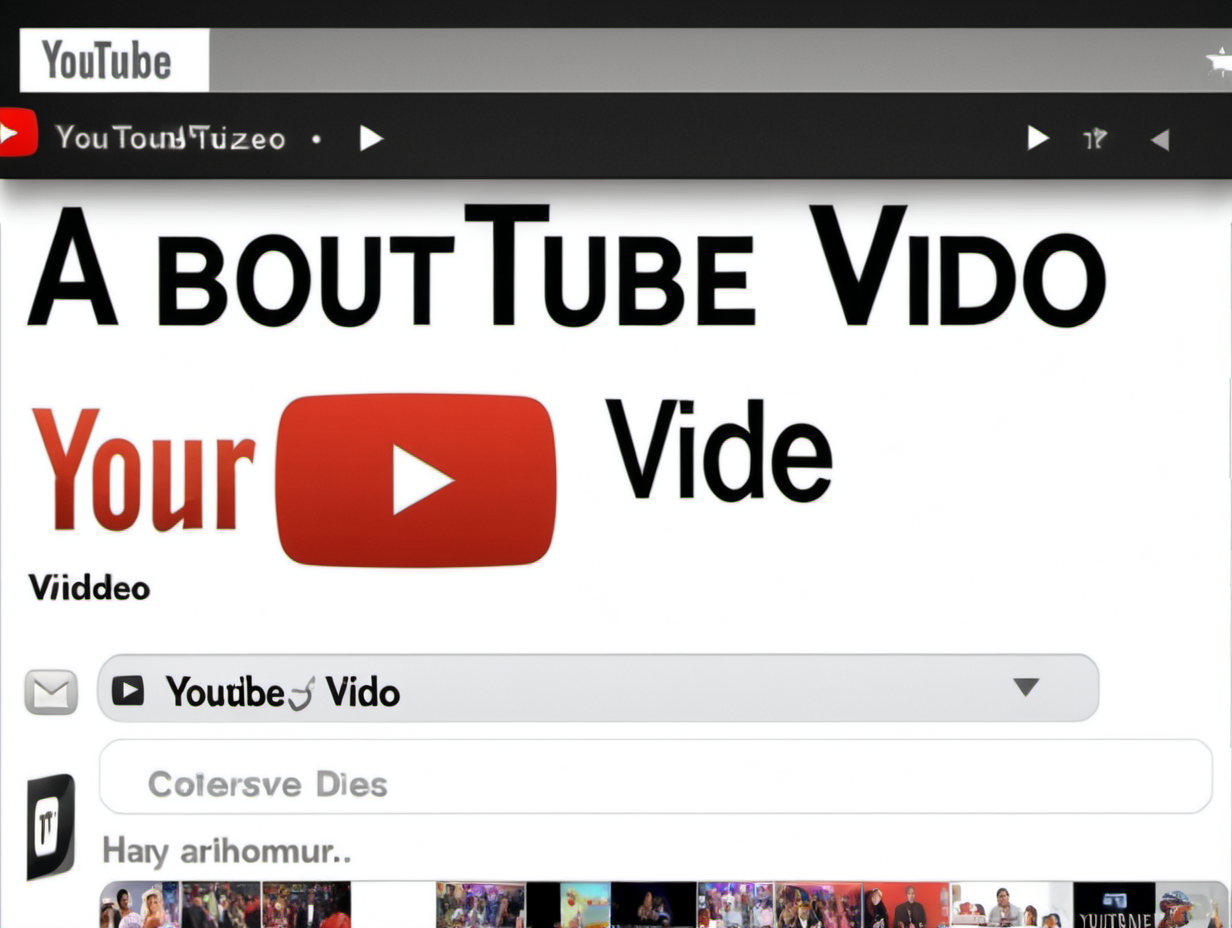
HOW much 💵💰💵 did Stable Diffusion COST to Train?
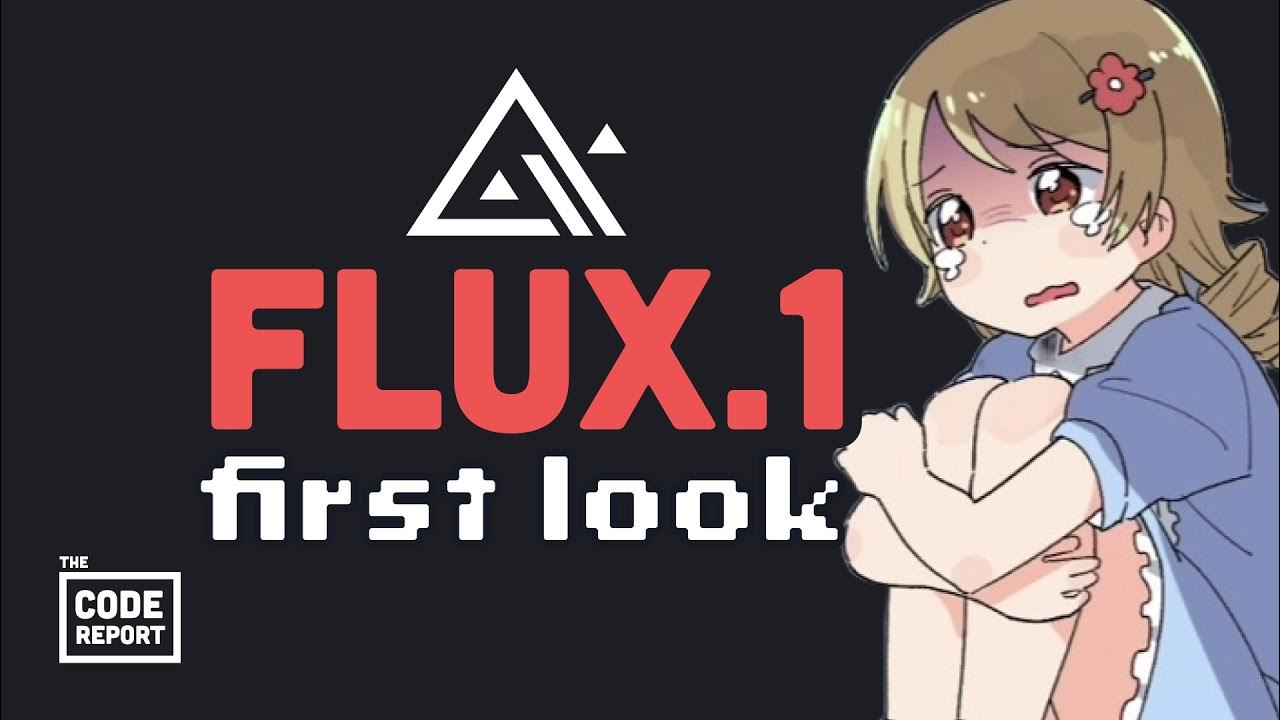
Wake up babe, a dangerous new open-source AI model is here
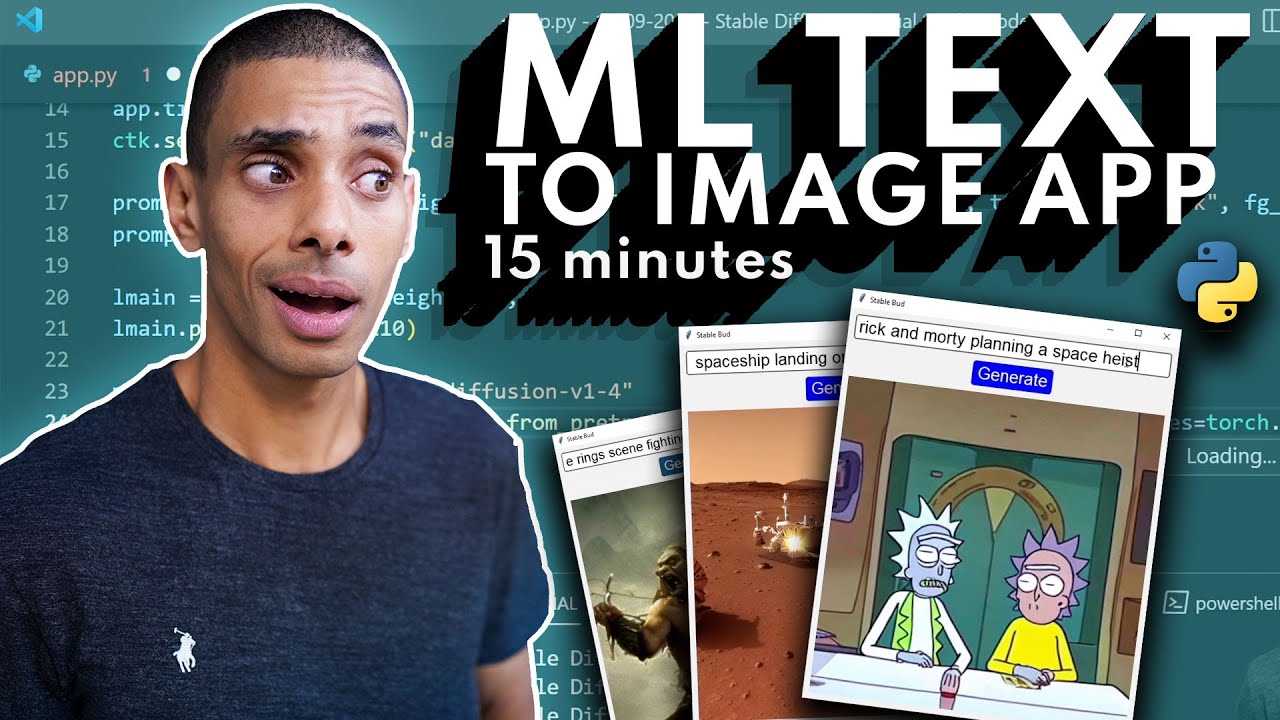
I tried to build a ML Text to Image App with Stable Diffusion in 15 Minutes

Create Unlimited Logos with AI (For FREE)

Generative AI Engineer Roadmap 2025 | Step-by-Step Complete Guidance..

How to Install & Use Stable Diffusion on Windows in 2024 (Easy Way)
5.0 / 5 (0 votes)