You NEED to AVOID these Mistakes as a Data Analyst | raw truth
Summary
TLDRIn this video, Rohan discusses three common mistakes made by beginner data analysts and offers advice on how to avoid them. He emphasizes the importance of avoiding sampling bias by ensuring representative data sets, maintaining high data quality to prevent errors in analysis, and cautions against confusing correlation with causation. Rohan also stresses the need for critical thinking and proper data validation to become a high-quality data analyst.
Takeaways
- 😀 Most data analysts struggle not due to coding skills but because they neglect the analysis itself.
- 📊 Statistics is crucial and forms the backbone of data analysis and data science.
- 🚫 Avoiding sampling bias is essential; ensure the sample size is representative of the entire population.
- 🏥 Examples of sampling bias include political polling in urban areas or medical studies focusing only on hospital visitors.
- 💡 Proper sampling methods are critical for accuracy, reliability, and integrity in studies, and are more cost-effective.
- 📈 Poor data quality can severely impact analysis and subsequent business decisions.
- 🔍 Data analysts should validate data from multiple sources to ensure accuracy before conducting analysis.
- 🧼 Data cleaning is vital, but be cautious not to introduce bias through incorrect handling of missing values.
- 🔗 Understanding the difference between correlation and causation is fundamental; correlation does not imply causation.
- 🌡 Common examples used to illustrate this include ice cream sales and shark attacks, or chocolate consumption and Nobel Prizes.
- 🔍 As a data analyst, it's important to critically think and investigate potential confounding variables that may affect data interpretation.
Q & A
What is the main reason most data analysts are not great at their job according to the speaker?
-The speaker suggests that most data analysts are not great at their job not because of their coding skills with SQL, Python, R, or BI tools, but because they neglect the actual analysis, particularly the importance of statistics in data analysis.
What is sampling bias and how does it affect data analysis?
-Sampling bias occurs when a small sample size taken for analysis is not representative of the entire population. This can lead to inaccurate conclusions because the data collected may not reflect the broader population's characteristics, thus affecting the reliability of the analysis.
Why is it important to use proper sampling methods in data analysis?
-Proper sampling methods ensure accuracy, reliability, and integrity in a study. They are also more cost-effective than analyzing an entire population, making it feasible to conduct large-scale analyses without incurring excessive costs.
What is the second biggest mistake data analysts make according to the video?
-The second biggest mistake data analysts make is using poor data quality. This can be due to issues like data pipeline errors, inaccurate data entry, or unreliable data sources, which can significantly impact the accuracy of analysis and subsequent business decisions.
How can data analysts validate the data they are using for analysis?
-Data analysts can validate the data by comparing it with a second or third source, or even third-party data, to ensure consistency and accuracy. This due diligence helps in identifying discrepancies and ensuring the data used is reliable before conducting analysis.
Why is data cleaning important in the data analysis process?
-Data cleaning is crucial to ensure data quality. It involves handling missing values, correcting errors, and removing inconsistencies. However, improper data cleaning can introduce bias or incorrect values, so it should be done carefully with proper automation and testing.
What is the difference between correlation and causation as explained in the video?
-Correlation refers to the relationship between two or more variables, while causation implies that one variable causes the other to change. The video emphasizes that just because two variables are correlated, it does not mean one causes the other, which is a common misconception that data analysts must avoid.
What is a confounding variable and how does it relate to correlation?
-A confounding variable is an external factor that influences two different variables, making them appear correlated when they are not. The video uses the example of hot weather leading to both increased ice cream sales and shark attacks, where the weather is the confounding variable.
Why is it important for data analysts to consider sample size when analyzing correlations?
-Smaller sample sizes can lead to higher correlations that may not accurately represent the true population. Data analysts should ensure their sample size is large enough to provide meaningful and representative correlations in their analysis.
What is the speaker's stance on the future demand for data analysts and data scientists?
-The speaker believes that data analysts and data scientists will continue to be in high demand, as data collection and analysis are still in their early stages and critical for businesses to make informed decisions, especially with advancements in AI.
Outlines
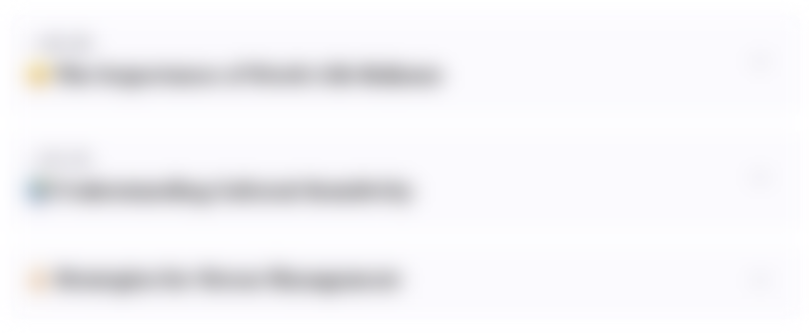
Cette section est réservée aux utilisateurs payants. Améliorez votre compte pour accéder à cette section.
Améliorer maintenantMindmap
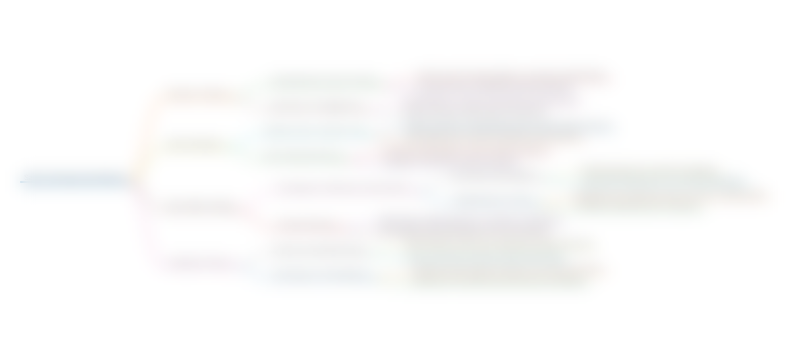
Cette section est réservée aux utilisateurs payants. Améliorez votre compte pour accéder à cette section.
Améliorer maintenantKeywords
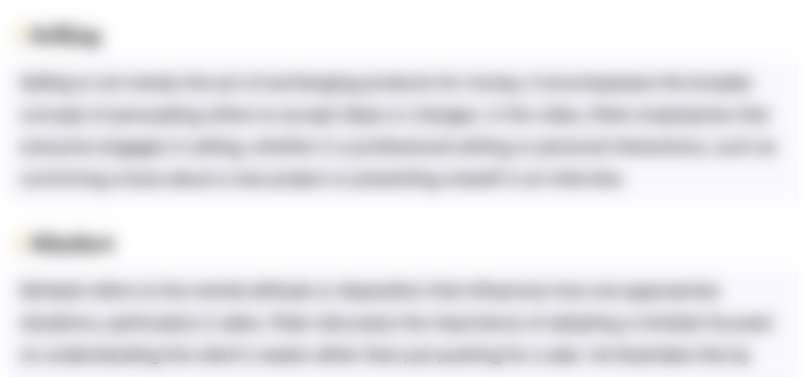
Cette section est réservée aux utilisateurs payants. Améliorez votre compte pour accéder à cette section.
Améliorer maintenantHighlights
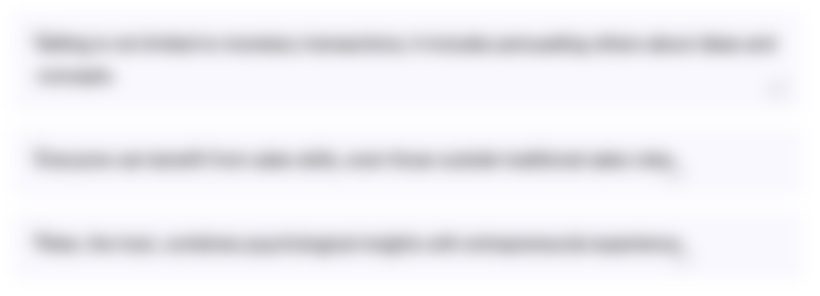
Cette section est réservée aux utilisateurs payants. Améliorez votre compte pour accéder à cette section.
Améliorer maintenantTranscripts
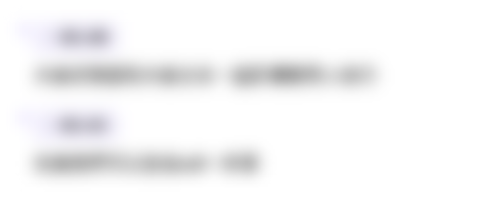
Cette section est réservée aux utilisateurs payants. Améliorez votre compte pour accéder à cette section.
Améliorer maintenantVoir Plus de Vidéos Connexes

Statistical and Critical Thinking
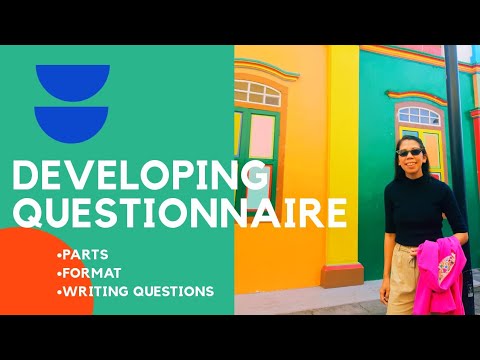
DEVELOPING A QUESTIONNAIRE
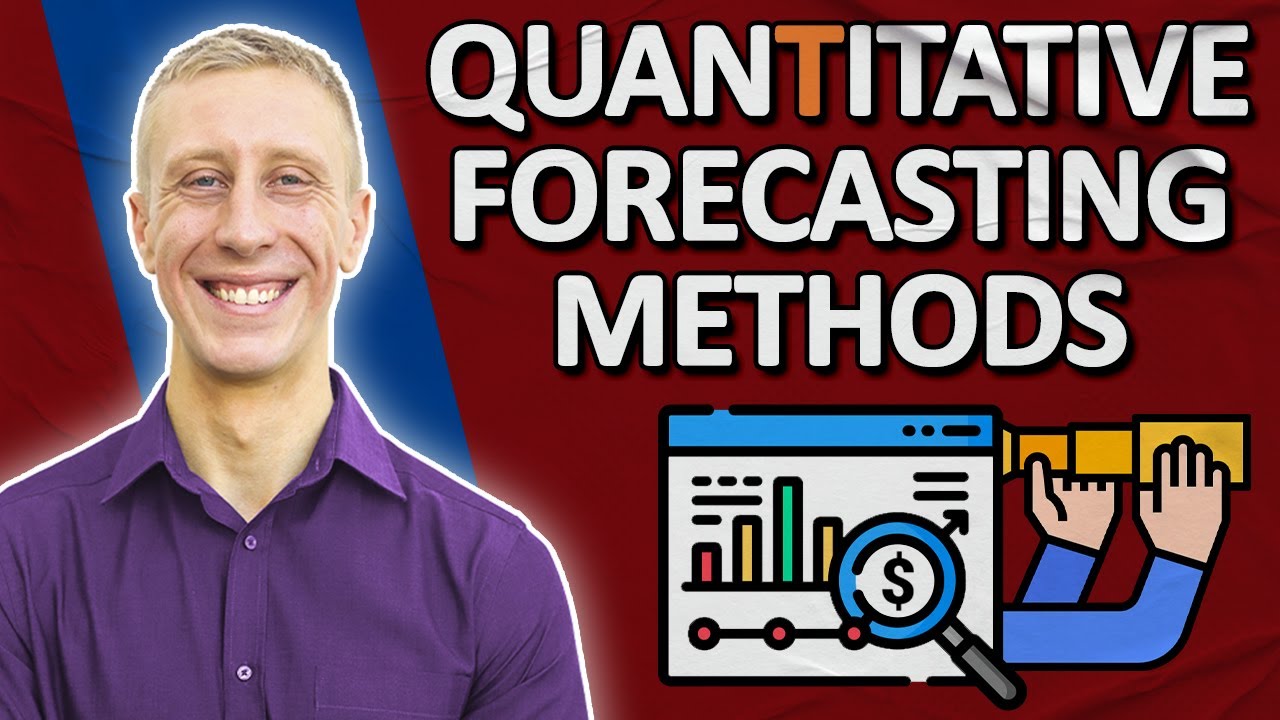
Quantitative Forecasting Methods in Business Operations

You need data literacy now more than ever – here’s how to master it | Talithia Williams
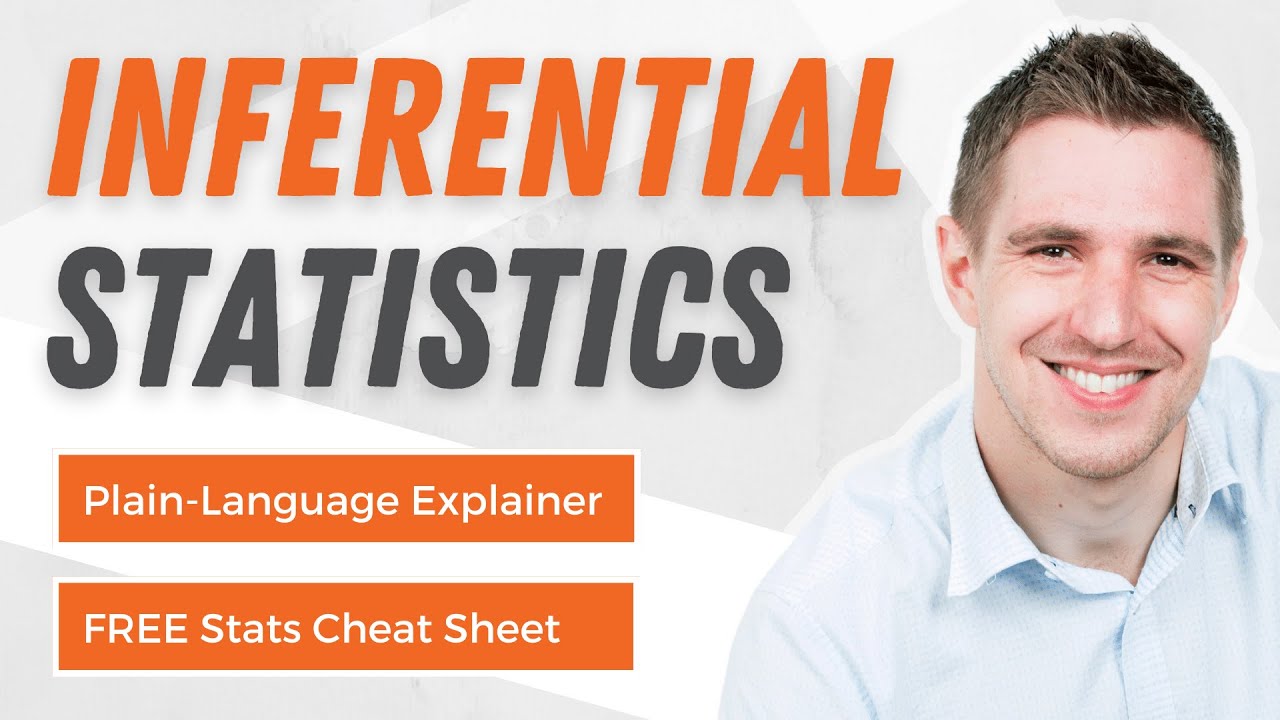
Inferential Statistics FULL Tutorial: T-Test, ANOVA, Chi-Square, Correlation & Regression Analysis

Data Observability: MultiCloud, GenAI Make Challenges Harder
5.0 / 5 (0 votes)