Scientists warn of AI collapse
Summary
TLDRThe video discusses the potential collapse of AI creativity due to a feedback loop where AI systems are trained on content they generate themselves, leading to a decrease in output diversity. Studies on language and image generation models show a drop in diversity as AI consumes its own output. The implications are significant, as AI-generated content infiltrates our environment, potentially requiring labeling laws. The future may see either acceptance of AI-generated content or advancements in AI models that enforce variety to overcome this issue.
Takeaways
- 🤖 AI-generated content (text, images, audio, videos) is becoming increasingly common.
- 🚨 There are concerns that AI creativity might collapse due to self-feeding on its own output.
- 🧠 AIs are deep neural networks that learn from vast amounts of data to recognize and reproduce patterns.
- 🔄 The data AIs learn from is originally created by humans, raising the risk of a feedback loop.
- 📉 Research shows that AI-generated content tends to have less variety when trained on its own output.
- 📈 A study on language models found that language diversity decreases with higher levels of creativity tasks.
- 🖼️ AI-generated images also show a decrease in diversity, becoming more homogeneous over time.
- 🐘 Examples of AI-generated images of elephants demonstrate a loss of detail and an increase in errors.
- 🌐 AI-generated content is contaminating our environment, potentially affecting future training data.
- 🔄 Possible outcomes include marking AI-generated content or developing new AI models that enforce variety.
- 📚 The video script recommends a Neural Network course on Brilliant.org for a deeper understanding of AI.
Q & A
What is the main concern regarding AI-generated content?
-The main concern is that AI-generated content may lead to a decrease in creativity and diversity, as AI systems are fed data that they have produced themselves, resulting in a homogenization of outputs.
How do deep neural networks learn to create content?
-Deep neural networks learn by being fed large amounts of data, which allows them to recognize and reproduce patterns in language, images, and videos.
What was the outcome of the study conducted by French scientists on language diversity in AI-generated text?
-The study found that the diversity of language in AI-generated text decreases as the AI consumes more of its own output, with the drop being especially rapid for tasks requiring high creativity, such as storytelling.
What did the Japanese group's research on AI-generated images reveal?
-The research showed that AI-generated images become less diverse when trained on their own output, leading to a more uniform set of images with familiar problems and a lack of variety.
What is the potential consequence of AI-generated content contaminating our environment?
-The consequence is that it becomes increasingly difficult to distinguish between AI-generated and human-generated content, which could lead to a loss of originality and creativity in the long term.
How might the issue of AI-generated content diversity be addressed in the future?
-One possibility is that future AI models may be designed to enforce variety, for example, by incorporating more randomness or other mechanisms to prevent the repetition of patterns.
What is the alternative scenario if AI-generated content diversity cannot be improved?
-If the issue cannot be overcome, it might be necessary to mark AI-generated content as such, potentially leading to new laws and regulations to ensure the distinction between human and AI creations.
What is the significance of the term 'Midjourney-ish' in the context of AI-generated images?
-'Midjourney-ish' refers to a recognizable style of images generated by the AI platform Midjourney, which tend to look similar and often depict people as white, young, and attractive, even without specific instructions.
What is the potential impact of AI-generated content on human creativity?
-If AI-generated content continues to lack diversity, human creativity may become more valuable, as AI may not be able to replace the unique and varied outputs of human minds.
How can one deepen their understanding of neural networks and AI?
-One can deepen their understanding by taking courses on platforms like Brilliant.org, which offer a variety of courses on neural networks, quantum computing, linear algebra, and other scientific topics.
What is the offer for new users on Brilliant.org mentioned in the script?
-New users can try Brilliant.org for free for 30 days, and the first 200 users to use the provided link will receive a 20% discount on the annual premium subscription.
Outlines
🤖 AI Creativity and Its Potential Collapse
The video discusses the increasing use of AI in generating text, images, audio, and videos, and the concerns about the potential collapse of AI creativity. It explains that AIs, particularly deep neural networks, learn from vast amounts of data to recognize and reproduce patterns. The problem arises when AIs are fed data created by previous AI outputs, leading to a decrease in the diversity of their outputs. This issue is illustrated with examples from language models and AI-generated images, showing a reduction in variety and an increase in homogeneity. The consequences of this trend are uncertain, but it could lead to a need for marking AI-generated content or the development of new AI models that enforce variety.
📚 Learning Resources on Neural Networks and Quantum Mechanics
The video concludes with a recommendation for viewers interested in understanding the science behind AI and quantum computing. It suggests taking courses on Brilliant.org, which offers a Neural Network course and a Quantum Mechanics course, among others. The video creator's own course on quantum mechanics is also mentioned. A special offer is provided for the first 200 viewers who use the link brilliant.org/sabine, offering a 20% discount on the annual premium subscription. The video ends with an invitation to try Brilliant for free and a reminder to return for more content the next day.
Mindmap
Keywords
💡AI generated content
💡Deep neural networks
💡Language diversity
💡Image diversity
💡Midjourney
💡Content contamination
💡Creativity
💡Randomness
💡Neural Networks course
💡Brilliant.org
Highlights
AI generated content is becoming increasingly common in text, images, audio, and videos.
There are concerns about the impact of AI on creative professions like writing and art.
AI creativity might collapse due to a feedback loop of self-generated data.
AIs learn from data created by humans, but there's a risk of them being trained on their own output.
The more AI consumes its own output, the less diverse the output becomes.
A study showed that language diversity decreases with higher levels of AI creativity tasks.
AI-generated images based on stable diffusion also show a decrease in diversity.
AI-generated images tend to have familiar issues like incorrect body parts and a lack of variety.
AI-generated content may contaminate our environment and training data, making it hard to distinguish from human content.
There's a possibility that AI-generated content will need to be marked to differentiate it from human creations.
The next generation of AIs might solve the diversity issue by enforcing variety and randomness.
The consequences of AI-generated content saturation are still unknown.
The video discusses the potential need for laws to address AI-generated content.
The speaker suggests that AI-generated content might not replace human creativity after all.
The video is sponsored by Brilliant.org, offering courses on neural networks and other scientific topics.
The speaker recommends Brilliant.org for building a background knowledge on various scientific subjects.
A special link is provided for a 20% discount on the annual premium subscription to Brilliant.org.
Transcripts
In the past year or so, we’ve all become used to AI generated text and images and
audio and increasingly also videos. There’s been a lot of talk about how
terrible this is for writers and artists and so on, but some computer scientists
are warning that this AI creativity may soon collapse. Let’s have a look.
The problem is fairly easy to understand but difficult to quantify. The AIs that we
currently use are deep neural networks that are fed huge amounts of data and basically learn to
recognize and reproduce patterns. Large language models recognize grammatic rules and words that
belong to each other, image creation software recognizes shapes and shadows and gradients,
video software recognizes moving shapes and their context and so on.
But where does that data come from that they need to learn? Well that
was created by the original neural networks, humans. The issue is now
that the more people use AIs to create new content, the higher the risk that
future AIs will be fed data that they have produced themselves. And what will this do?
It’s not a priority all that obvious, you might think that with AI having a
random element and sometimes being prone to generate nonsense, the result might be
that it just produces increasingly weird stuff. But actually the opposite seems to be the case,
both for language and images. The more AI eats its own output the less variety the output has.
For example in a paper from November, a group of scientists from France tested this for a
large language model. They used an open source model called OPT from Meta and developed several
measures for diversity of language. Then they test what happens for the diversity of language for
tasks requiring different levels of creativity. For example, summarizing a news article requires
low creativity, writing a story from a prompt requires high creativity. In this table they
summarize the language diversity score for the levels of training iteration. As you can see,
they pretty much all drop. The language diversity drop is especially rapidly for story telling.
A similar finding was made earlier by a group from Japan for AI generated images
based on stable diffusion. The AIs decrease the diversity of the image
set and if you train them on their own output, diversity continues to decrease.
You can see this rather clearly in the image sets that they use as examples.
These are some examples of real elephant images from the original data set that they
used. These are some examples of the images that the AI generated after training. As you can see
they have some of the familiar problems, some legs too many or two few two heads,
some conflation of body parts. But the most striking thing is if you look at
a collage. On the left is a sample of the original images, on the right the
AI generated ones. You see immediately that the AI generated ones are much more alike.
I think that many of us have by now noticed that. If you’ve been using Midjourney for some
while you’ll have learned to recognize Midjourney-ish images. Even leaving
aside the obvious problems that these images continue to have, they tend to output similar
looking images. For example unless otherwise instructed, people tend to be white, young,
and good looking. These are four images that Midjourney created when promoted with “human face,
photorealistic” without further instructions. As you can see, they all look more or less the same.
What are the consequences? Well, no one really knows. The issue is that
our entire environment is basically being contaminated by AI generated content and
since there’s no way to identify its origin, it will inevitably leak into training data.
It's like plastic pollution, won’t be long until we all eat and breathe the stuff.
There are two ways things can go from here. One is that it turns out that this is a
general problem which can’t be overcome with these types of models, in which case, well,
good news for humans, our creativity will still be needed. It also seems likely to me that AI
generated content will have to be marked as such, I suspect that this is where laws will take us.
The other way it could go is that the next generation of AIs will remedy this problem
by deliberately enforcing variety for example by making more use of randomness, and that
we’ll simply give up trying to distinguish AI generated content from human generated content.
What do you think? Let me know in the comments.
If you want to learn more about how Neural Networks work, I recommend you check out the
Neural Network course on Brilliant.org who've been sponsoring this video. The Neural Network
course will give you deeper understanding of how intelligent artificial intelligence really
is with some hands on examples. And Brilliant has courses on many other topics in science and
mathematics too. Whether you're interested neural nets or quantum computing or linear algebra,
they have you covered. I even have my own course there that's an introduction
to quantum mechanics. It'll bring you up to speed on all the basics - interference,
super positions, entanglement, and up to the uncertainty principle and Bell's theorem.
Brilliant is really the best place to build up your background knowledge on all those science
videos which you've been watching. You can try it out for free for 30 days but if you go there,
use our link brilliant.org/sabine because the first 200 to use our link will get 20%
off the annual premium subscription. So go and give it a try, Brilliant is time well spent.
Thanks for watching, see you tomorrow.
Voir Plus de Vidéos Connexes
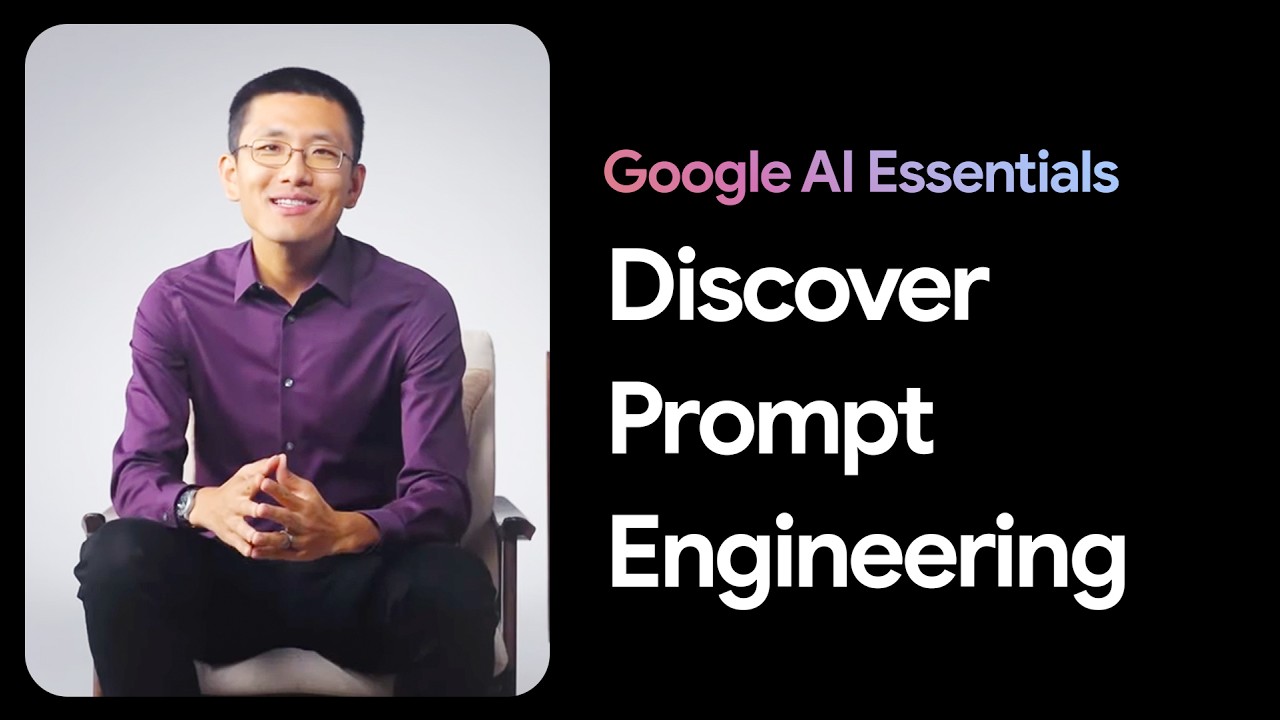
Discover Prompt Engineering | Google AI Essentials
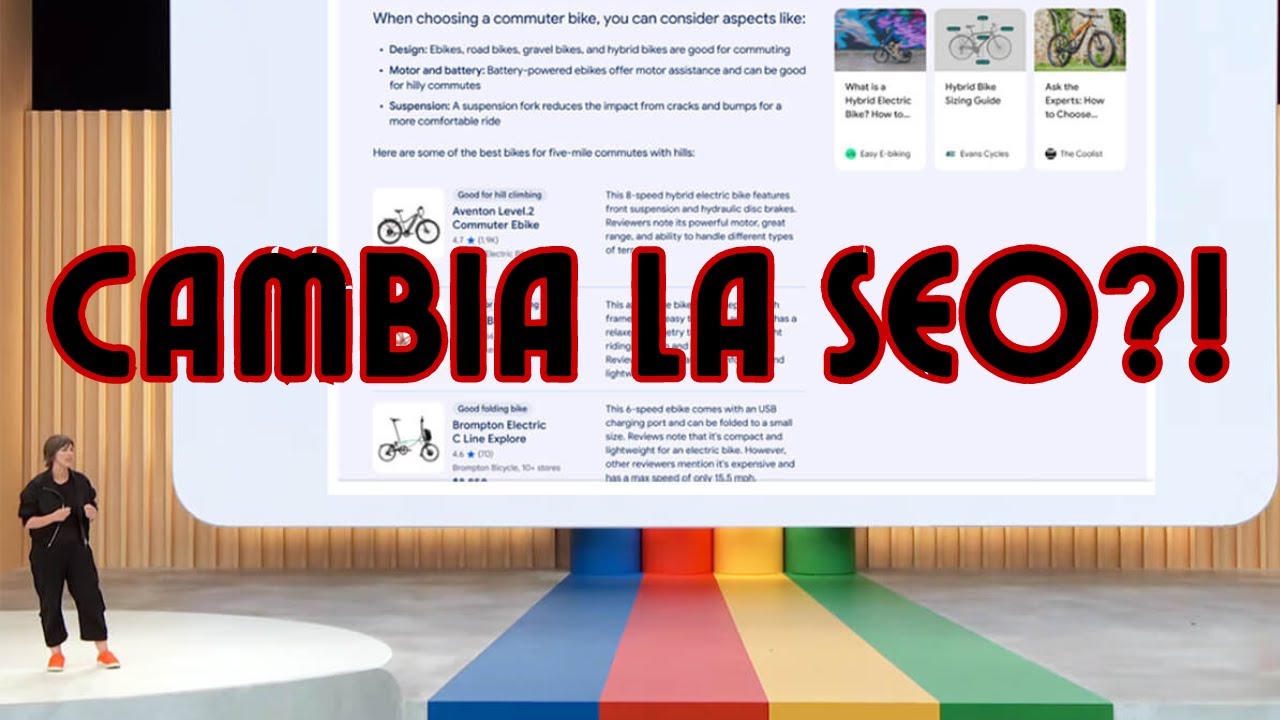
Google SGE: l'IA di Google che cambia tutto [Tutorial]
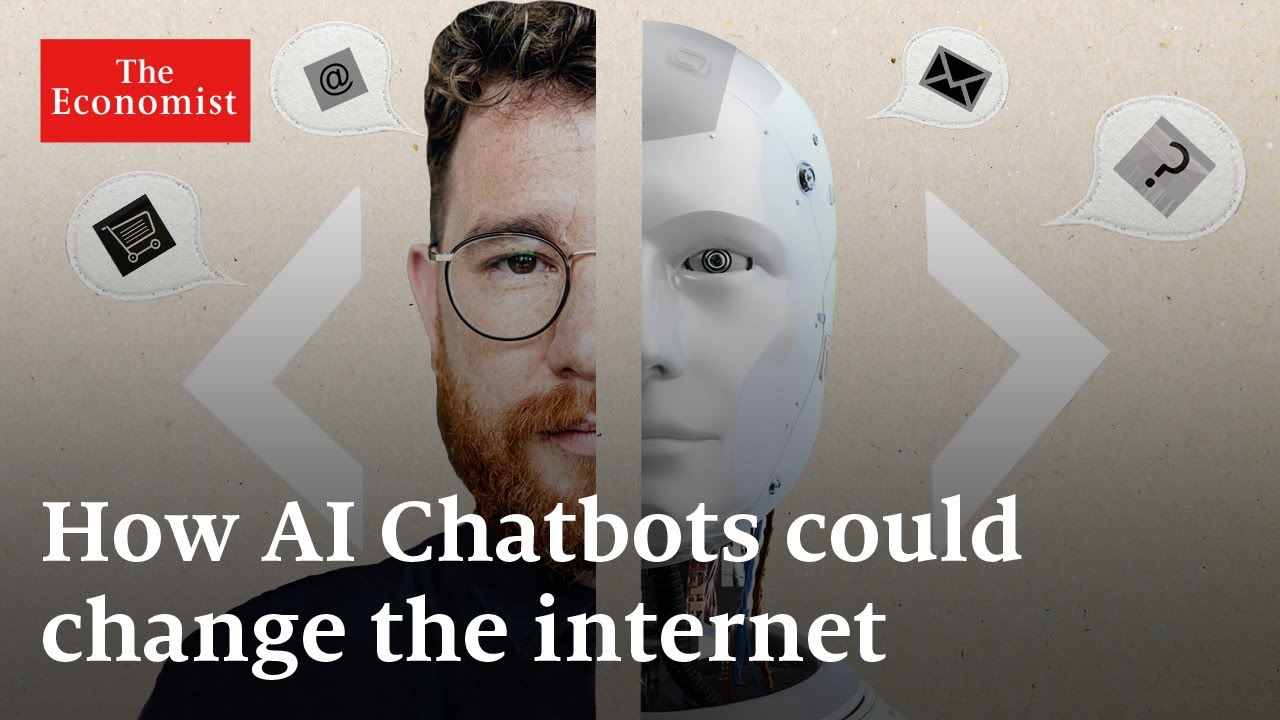
Beyond ChatGPT: what chatbots mean for the future
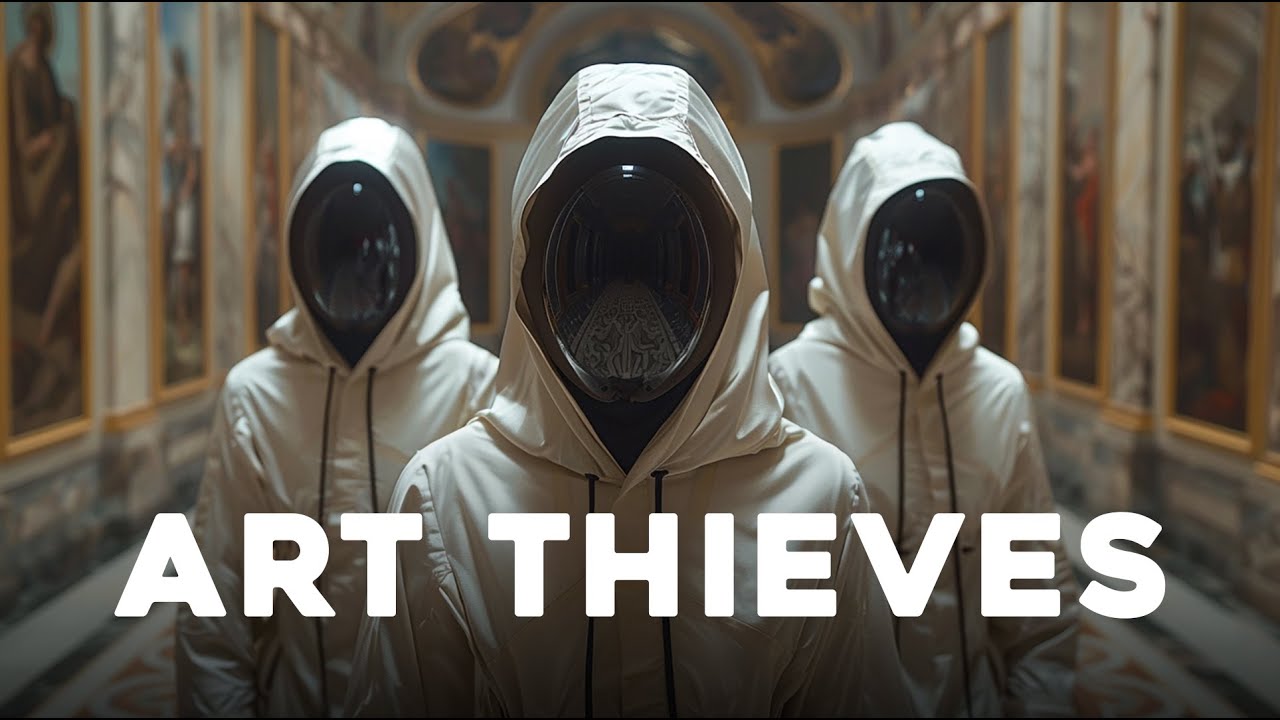
AI vs Artists - The Biggest Art Heist in History

AI NEWS Google's AI Leveling Up | Mystic v2, Pliny and AI Movies...

الذكاء الاصطناعي في أسبوع 🔥 | الحلقة 12 | نهاية سيطرة OpenAI أخبار مثيرة ونماذج وأدوات قوية ومجانية
5.0 / 5 (0 votes)