Are Hallucinations Popping the AI Bubble?
Summary
TLDRThe video script discusses the recent dip in Nvidia stocks and attributes it to the bursting of a bubble surrounding Large Language Models (LLMs) in AI, rather than AI as a whole. It highlights the issue of 'hallucinations' in LLMs, where nonsensical outputs are confidently produced. The speaker argues for the integration of symbolic language and logic into AI to address these issues, citing Deepmind's progress in AI for mathematical proofs as an example of 'neurosymbolic' AI. The script concludes that companies focusing solely on LLMs may struggle, while those building AI with logical reasoning and real-world models, like Deepmind, are more likely to succeed.
Takeaways
- 📉 The speaker bought Nvidia stocks hoping to benefit from the AI boom but has seen the stocks drop, attributing the decline to a bubble bursting in the market, specifically around Large Language Models (LLMs).
- 🧐 The speaker believes that the current AI enthusiasm will resume once people realize there's more to AI than just LLMs, and that their stocks will recover as a result.
- 😵 The main issue with LLMs, as highlighted, is the phenomenon of 'hallucinations' where the models confidently produce nonsensical outputs.
- 📚 Improvements have been made in LLMs to avoid obvious errors, such as providing real book recommendations, but they still struggle with logical consistency, like referencing non-existent papers and reports.
- 🤔 The speaker uses the example of Kindergarten riddles to illustrate how LLMs can provide answers that are 'close' to correct but fundamentally flawed due to missing context.
- 🔍 The crux of the problem is identified as the difference in metrics for 'good' output between humans and models, suggesting that models lack an understanding of what makes their output valuable to humans.
- 💡 A proposed solution is the integration of logic and symbolic language into AI, termed 'neurosymbolic', which could address many of the issues with LLMs by providing a more human-like logical structure.
- 🏆 The speaker cites Deepmind's progress in AI for mathematical proofs as an example of the potential of neurosymbolic AI, emphasizing the importance of logical rigor in AI development.
- 🤖 The speaker suggests that building intelligent AI requires starting with mathematical and physical reality models, then layering language on top, rather than focusing solely on language models.
- 💸 There is a warning that companies heavily invested in LLMs may not recover their expenses, and that those building AI on logical reasoning and real-world models, like Deepmind, are likely to be the winners.
- 😸 The speaker ends with a humorous note about the potential for AI to manage stocks and the recommendation of Brilliant.org for those interested in learning more about AI and related fields, offering a discount for new users.
Q & A
Why did the speaker decide to buy Nvidia stocks?
-The speaker bought Nvidia stocks to benefit from the AI boom, as they wanted to get something out of the advancements in artificial intelligence.
What is the speaker's opinion on the current state of AI and its impact on the stock market?
-The speaker believes that the current drop in AI-related stocks is not due to a problem with AI itself, but rather with a specific type of AI called Large Language Models, and they are optimistic that AI enthusiasm will resume once people realize there's more to AI.
What is the main issue with Large Language Models according to the script?
-The main issue with Large Language Models is that they sometimes produce 'hallucinations,' which means they confidently generate incorrect or nonsensical information.
How have Large Language Models improved in providing book recommendations?
-Large Language Models have become better at avoiding obvious pitfalls by listing books that actually exist, tying their output more closely to the training set.
What is an example of a problem that Large Language Models still struggle with?
-An example is when solving riddles like the wolf, goat, and cabbage problem, where leaving out critical information leads the model to provide an answer that is logically incorrect, even though it uses similar words to the original problem.
What is the fundamental problem the speaker identifies with Large Language Models?
-The fundamental problem is that Large Language Models use a different metric for 'good' output than humans do, and this discrepancy cannot be fixed by simply training the models with more input.
What solution does the speaker propose to improve the output of AI models?
-The speaker suggests teaching AI logic and using symbolic language, similar to what math software uses, and combining this with neural networks in an approach called 'neurosymbolic' AI.
Why does the speaker believe that AI needs to be built on logical reasoning and models of the real world?
-The speaker believes that because the world at its deepest level is mathematical, building intelligent AI requires starting with math and models of physical reality, and then adding words on top.
What is the speaker's view on the future of companies that have invested heavily in Large Language Models?
-The speaker thinks that companies that have invested heavily in Large Language Models may never recover those expenses, and the winners will be those who build AI on logical reasoning and models of the real world.
What does the speaker suggest for AI researchers to focus on?
-The speaker suggests that AI researchers should think less about words and more about physics, implying that a deeper understanding of the physical world is crucial for developing truly intelligent AI.
What resource does the speaker recommend for learning more about neural networks and large language models?
-The speaker recommends Brilliant.org for its interactive visualizations and follow-up questions on a variety of topics, including neural networks and large language models.
Outlines
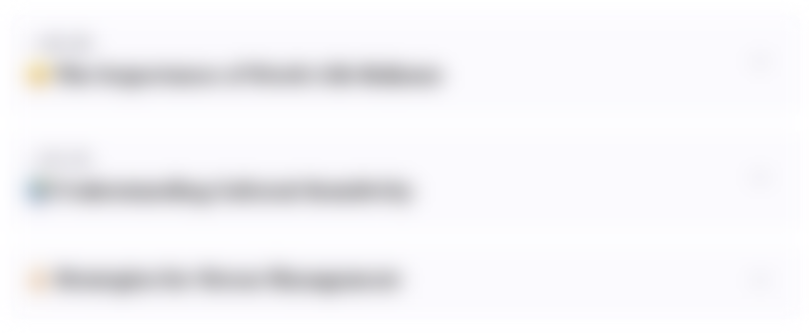
Esta sección está disponible solo para usuarios con suscripción. Por favor, mejora tu plan para acceder a esta parte.
Mejorar ahoraMindmap
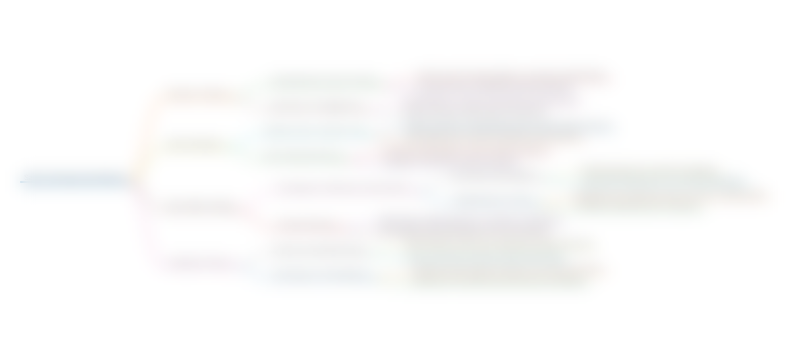
Esta sección está disponible solo para usuarios con suscripción. Por favor, mejora tu plan para acceder a esta parte.
Mejorar ahoraKeywords
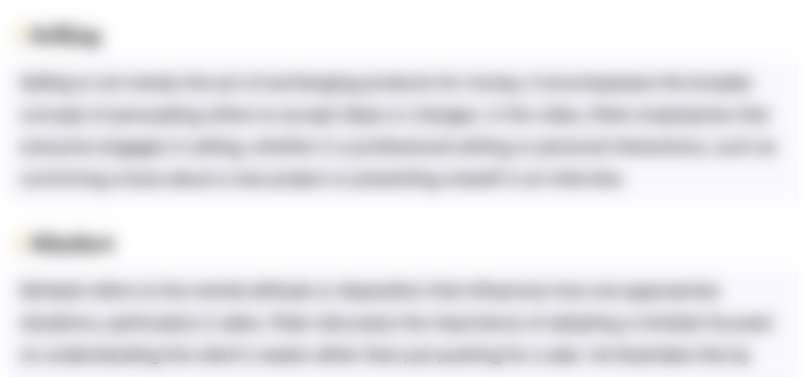
Esta sección está disponible solo para usuarios con suscripción. Por favor, mejora tu plan para acceder a esta parte.
Mejorar ahoraHighlights
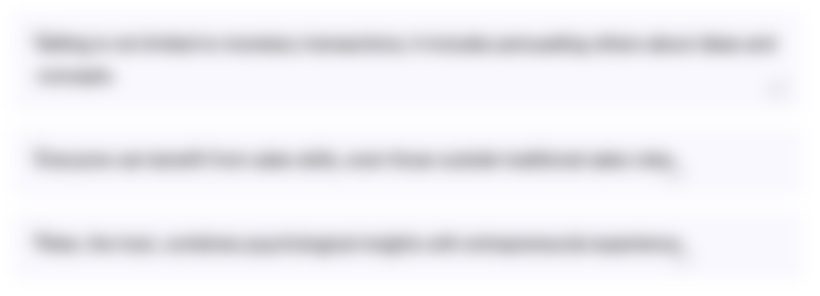
Esta sección está disponible solo para usuarios con suscripción. Por favor, mejora tu plan para acceder a esta parte.
Mejorar ahoraTranscripts
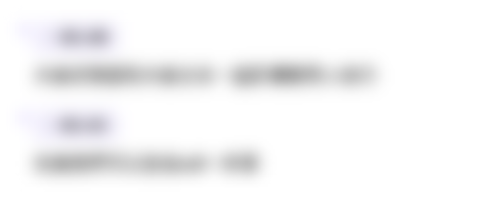
Esta sección está disponible solo para usuarios con suscripción. Por favor, mejora tu plan para acceder a esta parte.
Mejorar ahoraVer Más Videos Relacionados
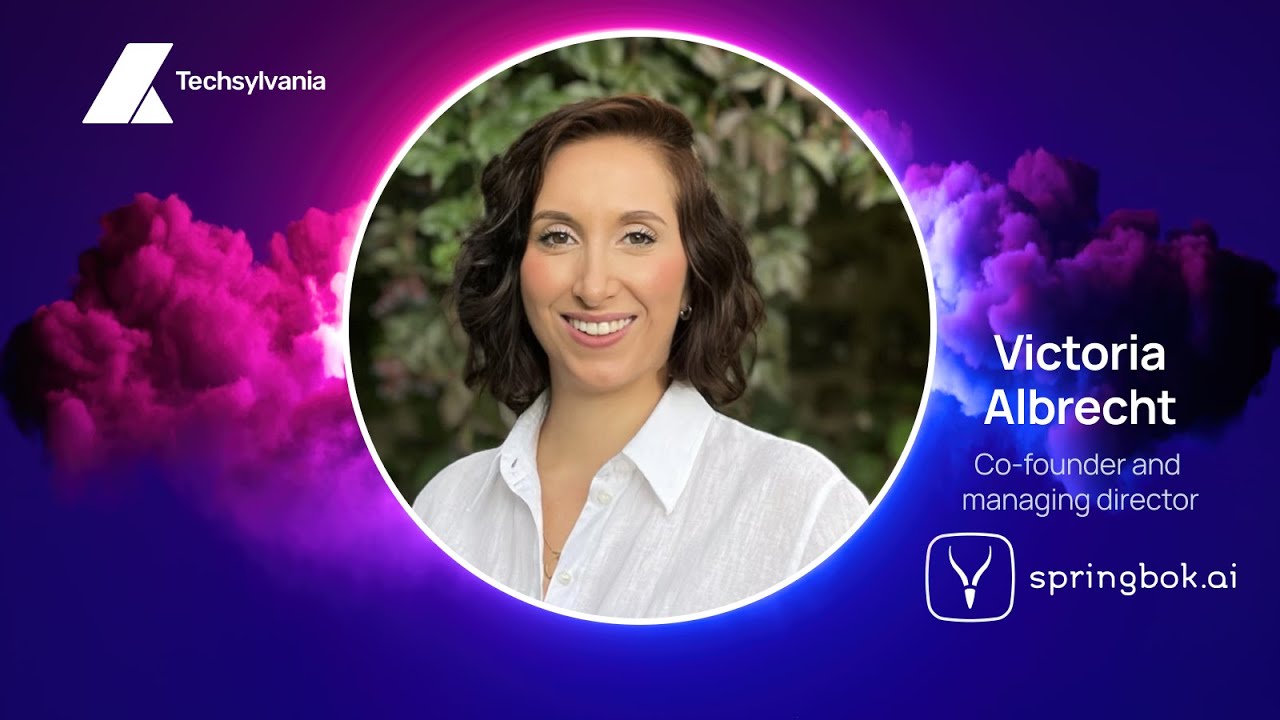
Conversation w/ Victoria Albrecht (Springbok.ai) - How To Build Your Own Internal ChatGPT
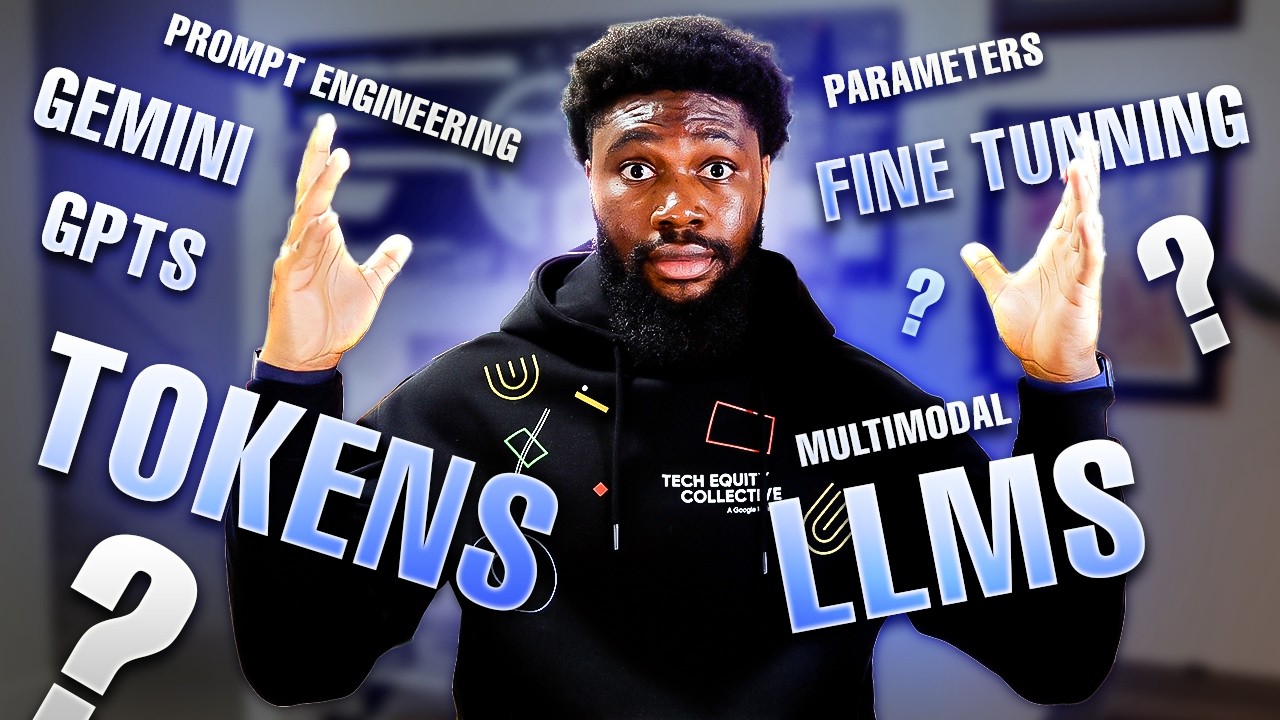
Simplifying Generative AI : Explaining Tokens, Parameters, Context Windows and more.
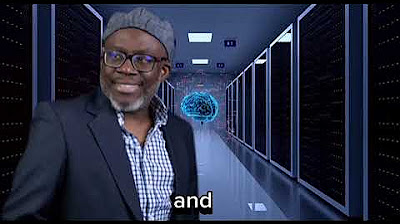
AI Unveiled beyond the buzz episode 4
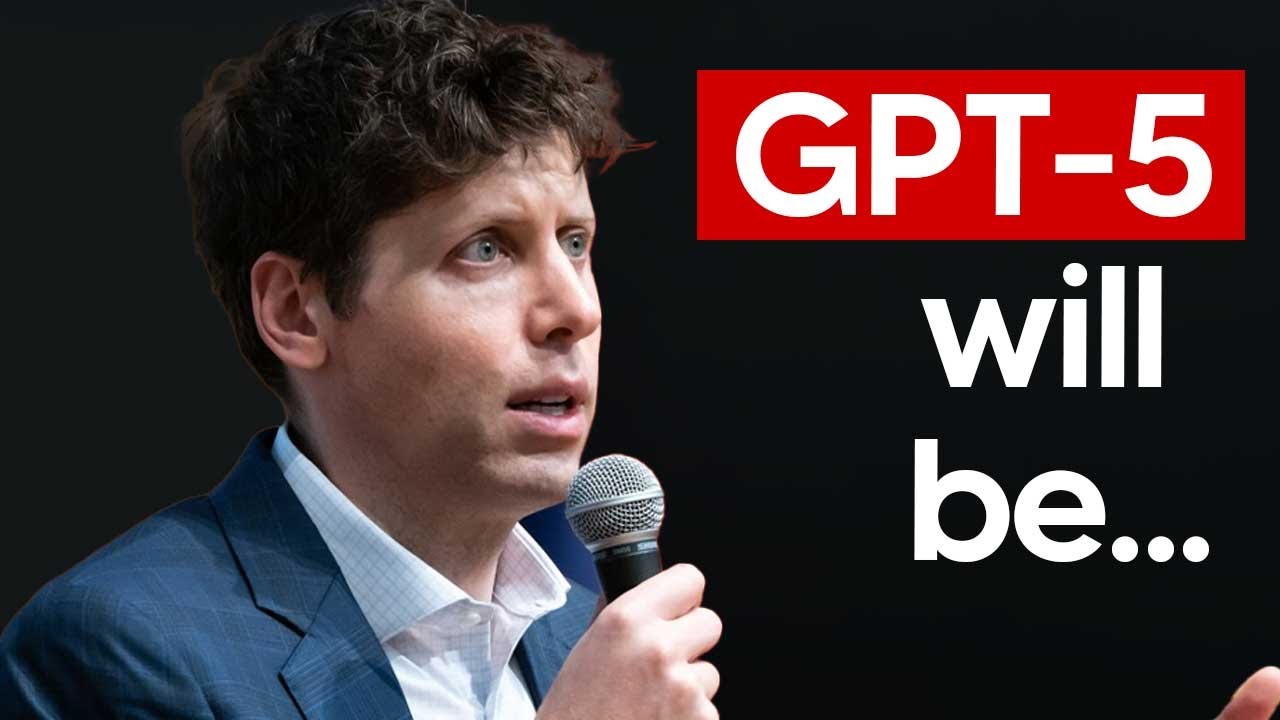
The AI Hype is OVER! Have LLMs Peaked?
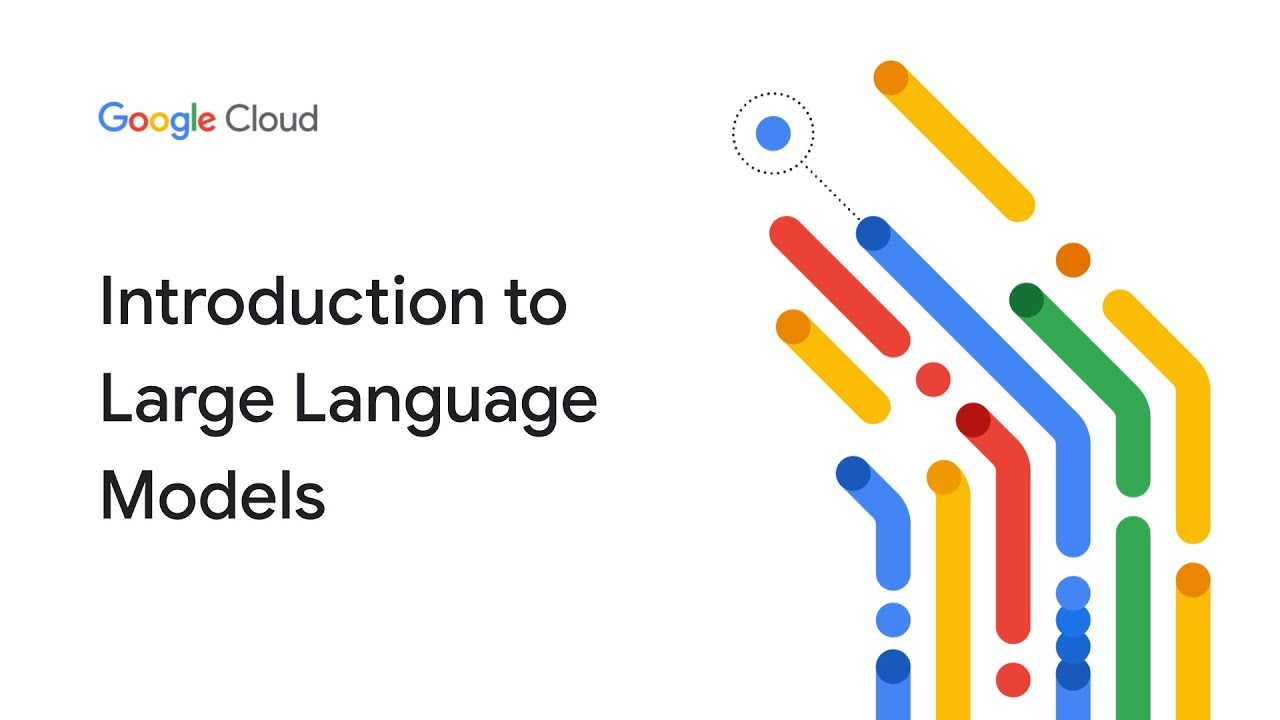
Introduction to large language models
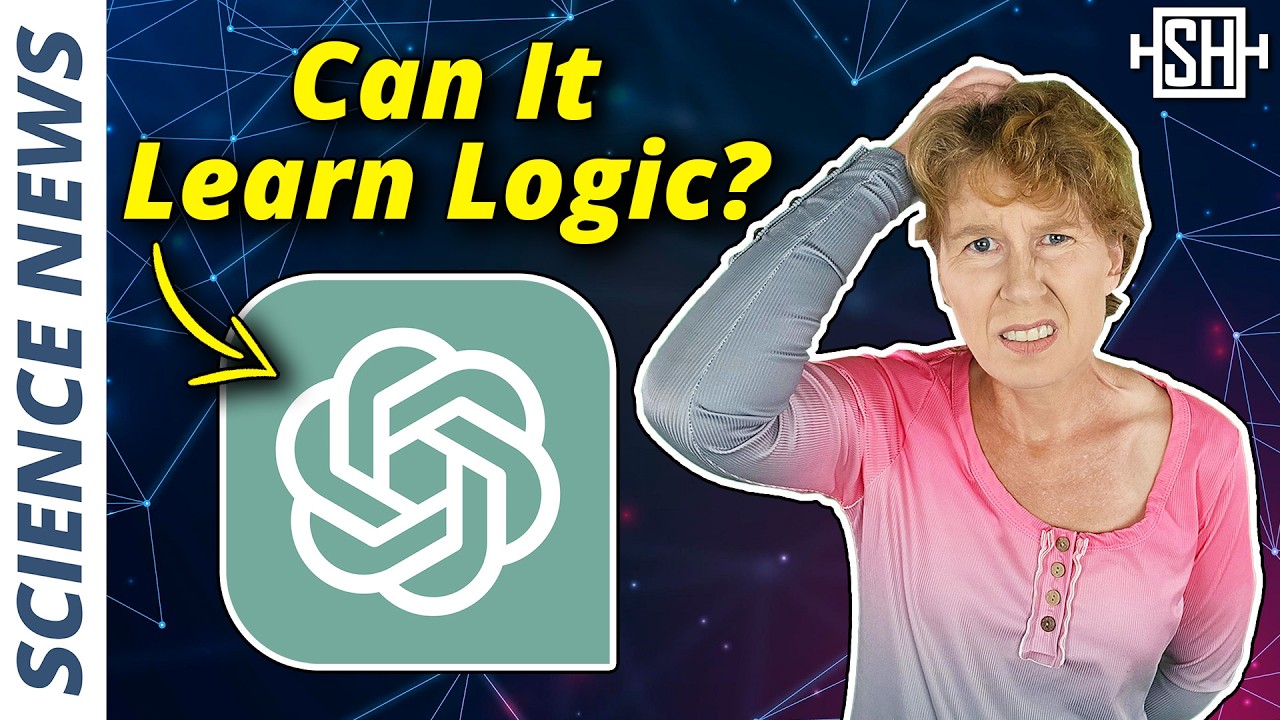
AI Can’t Reason. Should It Drive Cars?
5.0 / 5 (0 votes)