Conversation w/ Victoria Albrecht (Springbok.ai) - How To Build Your Own Internal ChatGPT
Summary
TLDRVictoria Albrecht, co-founder of Springbok AI, discusses the complexities and considerations of building and utilizing large language models (LLMs) in business. She emphasizes the high costs and expertise required for developing proprietary LLMs, suggesting that for most companies, fine-tuning existing models or using prompt architecture may be more practical. Albrecht outlines a framework for decision-making regarding LLM integration, highlighting the importance of aligning AI strategy with business goals rather than blindly following tech giants.
Takeaways
- 👋 Victoria Albrecht, co-founder and managing director of Springbok AI, leads a team of engineers and a commercial team on various projects focusing on AI development.
- 🌟 Victoria's previous experience includes scaling a food tech business and working with Rasa, a conversational AI framework, which aligns with her session's focus on building internal chat GPTs.
- 🤖 The session aims to provide insights on whether building your own large language model (LLM) is the right approach for a company or if there are alternative solutions.
- 🚀 Building an LLM is resource-intensive, with costs reaching into billions of dollars and requiring significant expertise and time investment, making it a strategy suitable primarily for tech giants aiming for global domination.
- 🔮 A common misconception is that building an LLM will automatically lead to a 'money printing machine,' but the reality is much more complex and costly.
- 🛠 For companies not seeking global dominance or lacking the resources to build an LLM, fine-tuning an existing LLM can be a viable option, provided they have a substantial dataset and the need for on-premise hosting.
- 🔒 Fine-tuning an LLM can offer domain-specific information, data security, and compliance, but it also comes with challenges such as the black-box nature of the model and the high costs associated with retraining.
- 💼 Many companies express a desire for a bespoke AI solution to automate and streamline processes, improve decision-making, and maintain data security, but often they do not require building or fine-tuning an LLM to achieve this.
- 🏢 Prompt architecture is introduced as a scalable and controllable method for leveraging LLMs, allowing for high data security and low risk without the need for developing or fine-tuning an LLM.
- 🔑 Prompt architecture involves context-based text enhancement and response accuracy checks, providing a software layer for control and steerability, which can be adapted based on company needs and data.
- 🌐 Springbok AI has developed an enterprise platform utilizing prompt architecture, enabling clients to upload documents and query them effectively, showcasing the practical application of LLMs in business processes.
Q & A
Who is Victoria Albrecht and what is her role at Springbok AI?
-Victoria Albrecht is the co-founder and managing director of Springbok AI, where she leads a team of 40 engineers and a commercial team on multiple projects, driving the further development of the business.
What was the topic of Victoria's session at the event?
-Victoria's session was about sharing insights on how to build your own internal chat GPT, which is a conversational AI framework.
What is the significance of the story about Andreas and Vlad in Victoria's talk?
-The story about Andreas and Vlad illustrates the early support and trust that helped Victoria and Springbok AI grow, highlighting the importance of networking and connections in the tech industry.
Why did Victoria share her experiences in Japan during her presentation?
-Victoria shared her experiences in Japan to emphasize the global reach and recognition of AI technologies, showing that the interest in AI extends beyond tech circles and has a widespread impact.
What is the main challenge companies face when considering building their own large language model (LLM)?
-The main challenge is the significant investment in resources, expertise, and time required to develop an LLM, which may not be justifiable for companies that do not aim for global domination in the tech industry.
Why did the executive of a major toy company consider developing their own LLM?
-The executive considered developing their own LLM as part of their AI strategy, possibly influenced by the actions of big tech companies, without necessarily considering whether it was the most suitable path for their business.
What is the cost implication of developing a large language model like Chat GPT?
-Developing a large language model like Chat GPT involves a substantial financial investment, with OpenAI alone having spent roughly two billion dollars on its development.
What is the alternative to building or fine-tuning an LLM for companies that do not have the resources or need for such extensive AI models?
-The alternative is prompt architecture, which allows companies to leverage existing LLMs like Chat GPT through a software layer that provides high control, data security, and low risk.
How does prompt architecture help companies utilize LLMs for their specific needs?
-Prompt architecture enables companies to input contextual information and instructions to tailor the LLM's responses to their specific requirements, enhancing control and ensuring data security.
What are some examples of use cases where companies might not need their own LLM but can benefit from prompt architecture?
-Examples include sales leaders wanting to automate the generation of sales contracts, HR departments providing 24/7 access to company policies, and investors querying internal databases for startup information.
Outlines
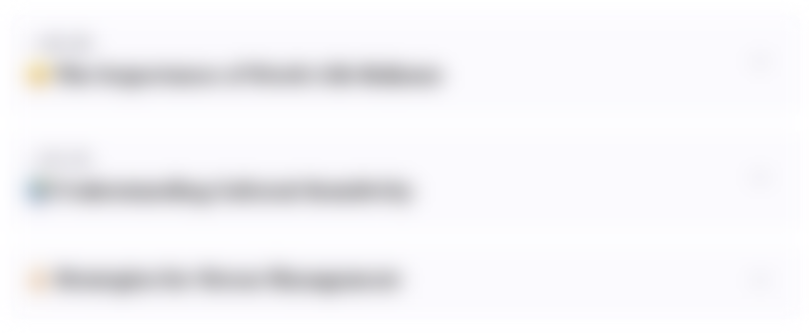
This section is available to paid users only. Please upgrade to access this part.
Upgrade NowMindmap
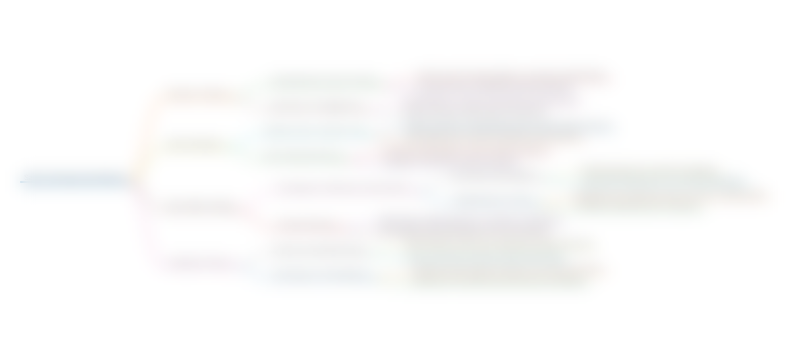
This section is available to paid users only. Please upgrade to access this part.
Upgrade NowKeywords
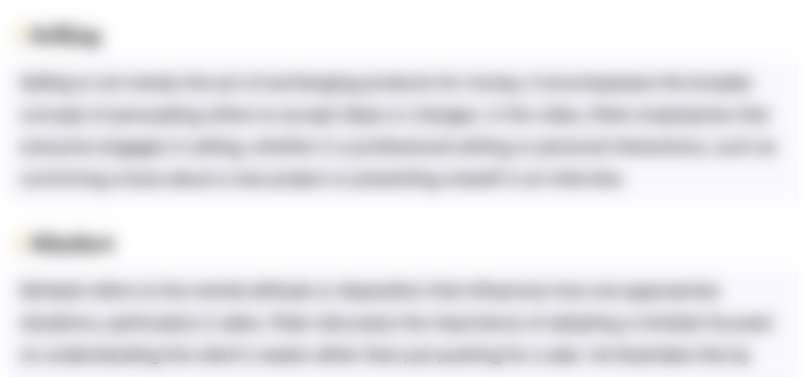
This section is available to paid users only. Please upgrade to access this part.
Upgrade NowHighlights
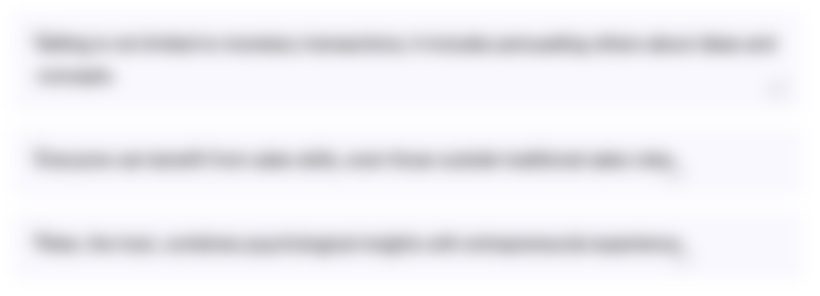
This section is available to paid users only. Please upgrade to access this part.
Upgrade NowTranscripts
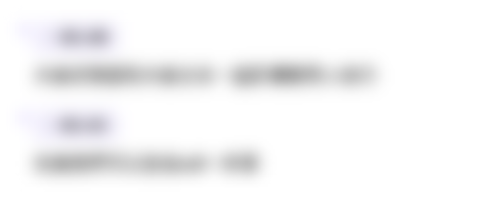
This section is available to paid users only. Please upgrade to access this part.
Upgrade Now5.0 / 5 (0 votes)