Bias - II
Summary
TLDRThe video script explores the bias inherent in AI models, highlighting how demographic factors like geography and race influence model outputs. It discusses examples of biased AI behavior in legal systems, media, and hiring practices, showing how these models often reflect and reinforce existing societal prejudices. The video also emphasizes the need for awareness and regulation in AI development, referencing studies and real-world incidents to illustrate the ethical implications of biased AI. The script concludes with a recommendation to watch the documentary 'Coded Bias,' which delves deeper into these issues.
Takeaways
- 🔍 The perception of bias varies significantly depending on geographic and demographic contexts.
- 🌍 Models and datasets often exhibit biases that align more with Western societies, potentially leading to different outcomes for similar inputs in different regions.
- 🧠 The use of terms like 'black' can have different connotations and impacts depending on the cultural context, such as in India versus the U.S.
- 📊 The Perspective API and other similar tools may perform differently across different populations due to the inherent biases in the training data.
- 👥 Gender bias in models is highlighted through the different responses generated when prompts are phrased differently but convey the same meaning (e.g., 'men are stronger than women' vs. 'women are weaker than men').
- ⚖️ Legal document analysis in India reveals biases where the outcomes of cases may change based solely on the community identity of the involved individuals.
- 📉 Biases in AI models are evident in various applications, such as bail prediction and name-based discrimination, which can lead to different legal outcomes.
- 🤖 Historical examples, like Microsoft's Tay chatbot, demonstrate how AI can quickly adopt and amplify biases from user interactions, leading to problematic behaviors.
- 👨⚖️ Biases in AI-generated content can manifest in subtle ways, such as racial and gender biases in job-related images, where certain roles are depicted with specific demographics.
- 📚 The importance of addressing bias in AI is emphasized through case studies and ongoing research, with legal frameworks being developed to ensure responsible AI usage in areas like hiring and law.
Q & A
What is the main focus of the video transcript?
-The main focus of the transcript is on bias and perception in AI models, particularly how demographic and geographic differences affect the interpretation of terms and how AI models trained on biased data sets can produce biased outputs.
How do geographic differences impact the perception of certain phrases?
-Geographic differences can significantly impact how certain phrases are perceived. For example, the phrase 'black man got into a fight' may have different connotations and elicit stronger reactions in the US compared to India, due to different historical and cultural contexts.
What was the conclusion of the paper discussed in the transcript regarding the bias in AI models?
-The paper concluded that many existing data sets and AI models are aligned towards Western societies, which introduces bias. This is because the data and design choices are often influenced by the researchers' positionalities, leading to performance mismatches when the models are used in non-Western contexts.
What is Perspective API, and how does it relate to the discussion on bias?
-Perspective API is a tool used to detect and filter toxic content on platforms, such as news websites. The discussion highlights how Perspective API's performance varies depending on the user's geographic location, which suggests that the API might be biased towards Western standards of toxicity.
What example was given to illustrate gender bias in AI model responses?
-An example was provided where two prompts—'Men are stronger than women' and 'Women are weaker than men'—elicited very different responses from the AI. The first prompt generated a more neutral response, while the second prompt was flagged as potentially violating usage policies, illustrating bias in how the AI handles gender-related statements.
How does name switching in legal documents reveal bias in AI models?
-The transcript discusses a study where names in legal documents were switched (e.g., from 'Keralite' to 'Punjabi'), and the AI model produced different legal predictions, such as whether a certain law applied or whether bail should be granted. This demonstrated that the AI model's predictions were influenced by the perceived identity of the individuals involved, revealing inherent bias.
What was the purpose of the 'Insa' project mentioned in the transcript?
-The 'Insa' project aimed to fine-tune large language models (LLMs) with legal prompts, both with and without identity information, to evaluate the bias in these models. The project developed a Legal Safety Score (LSS) to measure how biased the models were after fine-tuning.
What real-world examples of biased AI systems were mentioned?
-Real-world examples included Microsoft's 'Tay' chatbot, which was quickly shut down after it started generating racist and offensive responses, and an AI tool from Allen Institute of AI, which produced biased outputs when asked ethical questions. These examples illustrate how AI systems can reinforce harmful stereotypes if not properly managed.
What is 'Coded Bias,' and why is it recommended in the transcript?
-'Coded Bias' is a documentary that explores the biases embedded in AI systems and how these biases can have real-world consequences. It is recommended as a resource for understanding the impact of AI bias and the importance of addressing it in research and development.
How does the transcript address the issue of AI in hiring practices?
-The transcript discusses emerging regulations, such as New York City's AI hiring law, which aim to prevent AI systems from entrenching racial and gender biases in hiring processes. The MIT Tech Review article referenced in the transcript highlights the controversy and importance of such regulations in ensuring fair hiring practices.
Outlines
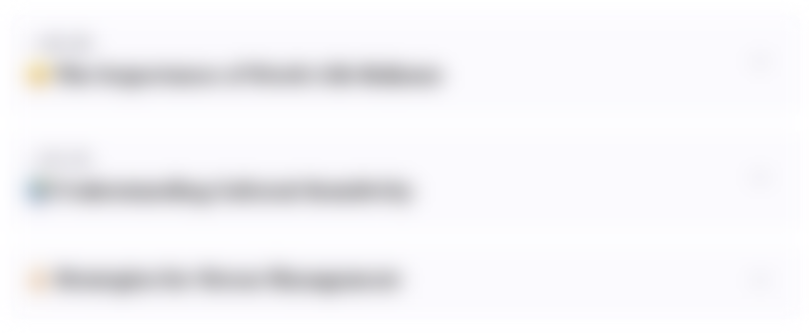
Esta sección está disponible solo para usuarios con suscripción. Por favor, mejora tu plan para acceder a esta parte.
Mejorar ahoraMindmap
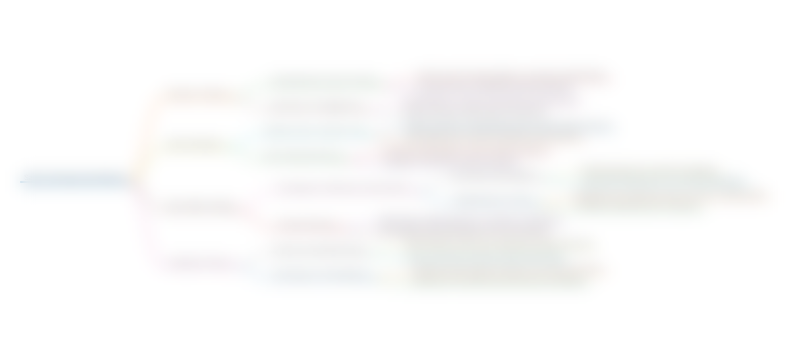
Esta sección está disponible solo para usuarios con suscripción. Por favor, mejora tu plan para acceder a esta parte.
Mejorar ahoraKeywords
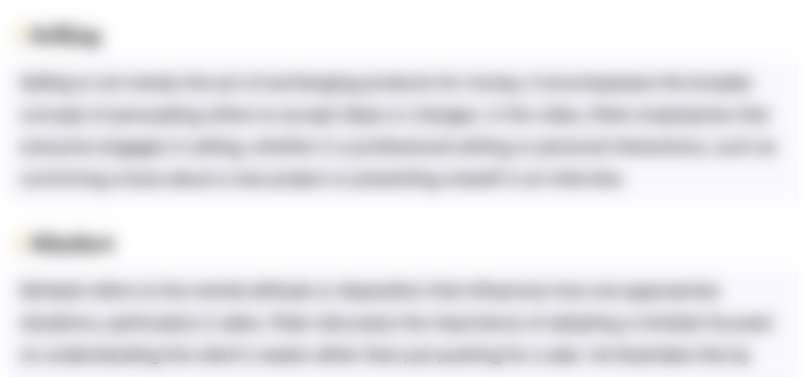
Esta sección está disponible solo para usuarios con suscripción. Por favor, mejora tu plan para acceder a esta parte.
Mejorar ahoraHighlights
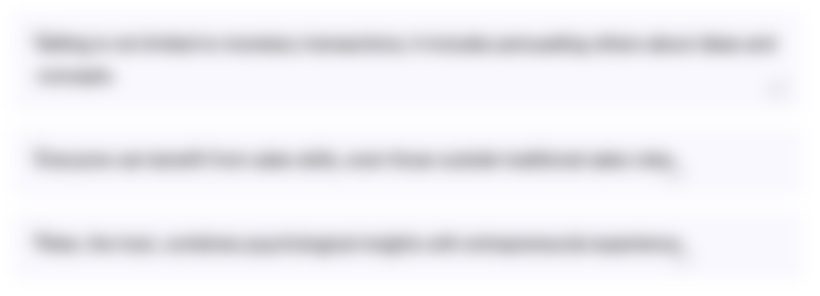
Esta sección está disponible solo para usuarios con suscripción. Por favor, mejora tu plan para acceder a esta parte.
Mejorar ahoraTranscripts
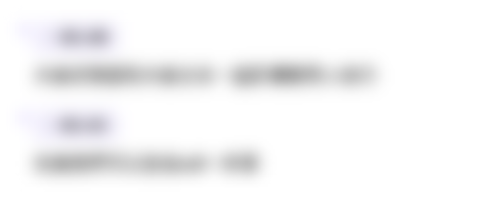
Esta sección está disponible solo para usuarios con suscripción. Por favor, mejora tu plan para acceder a esta parte.
Mejorar ahoraVer Más Videos Relacionados
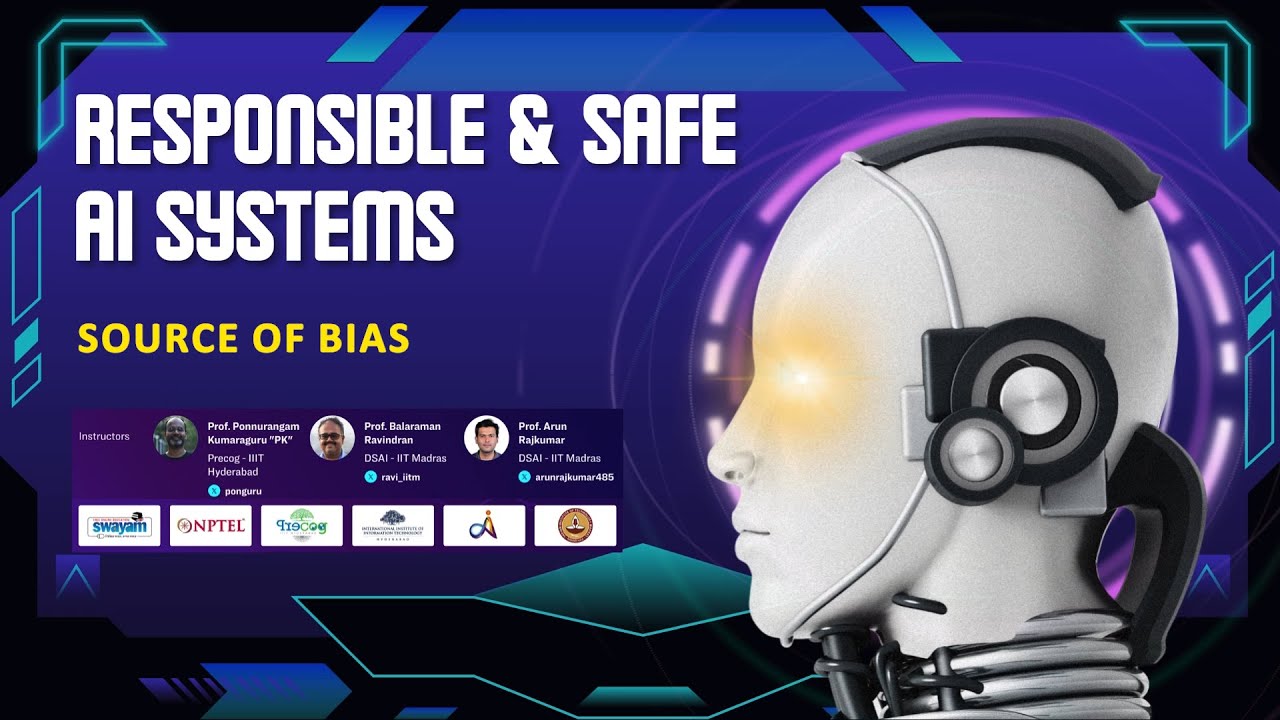
Source of Bias
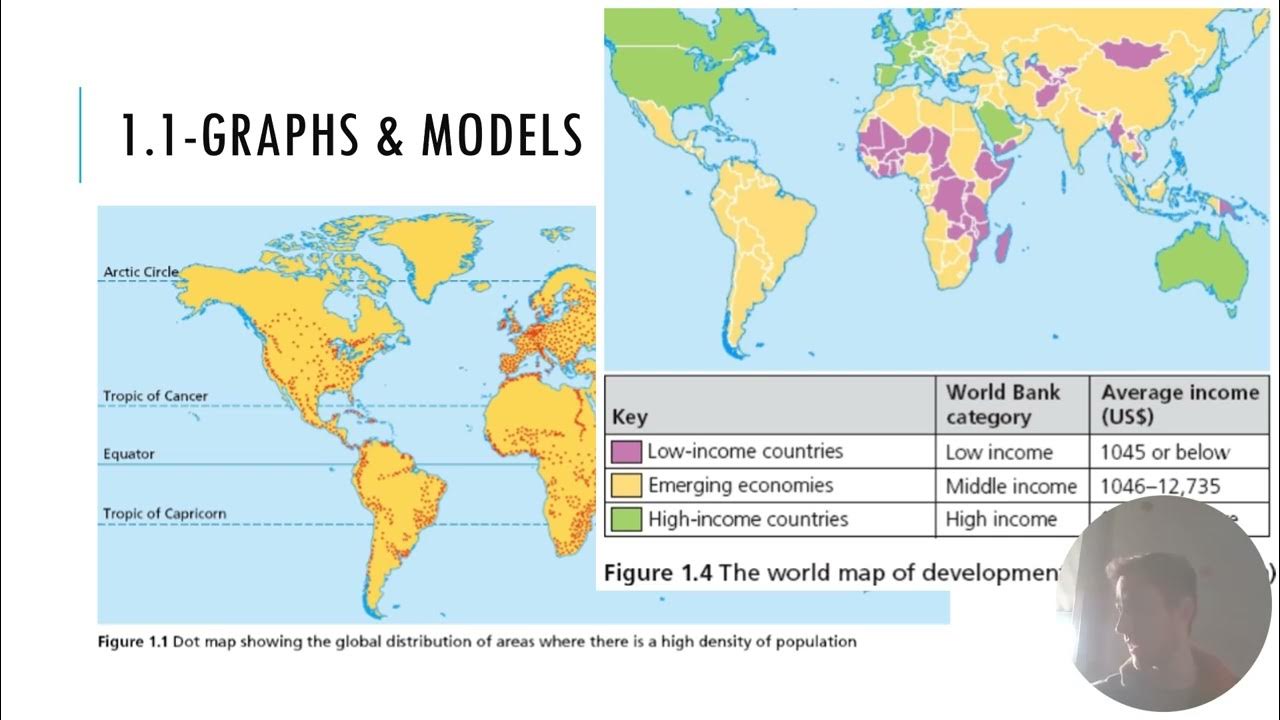
Finnian & Mr. Carley Review IB Geo Unit 1 (Part 1)
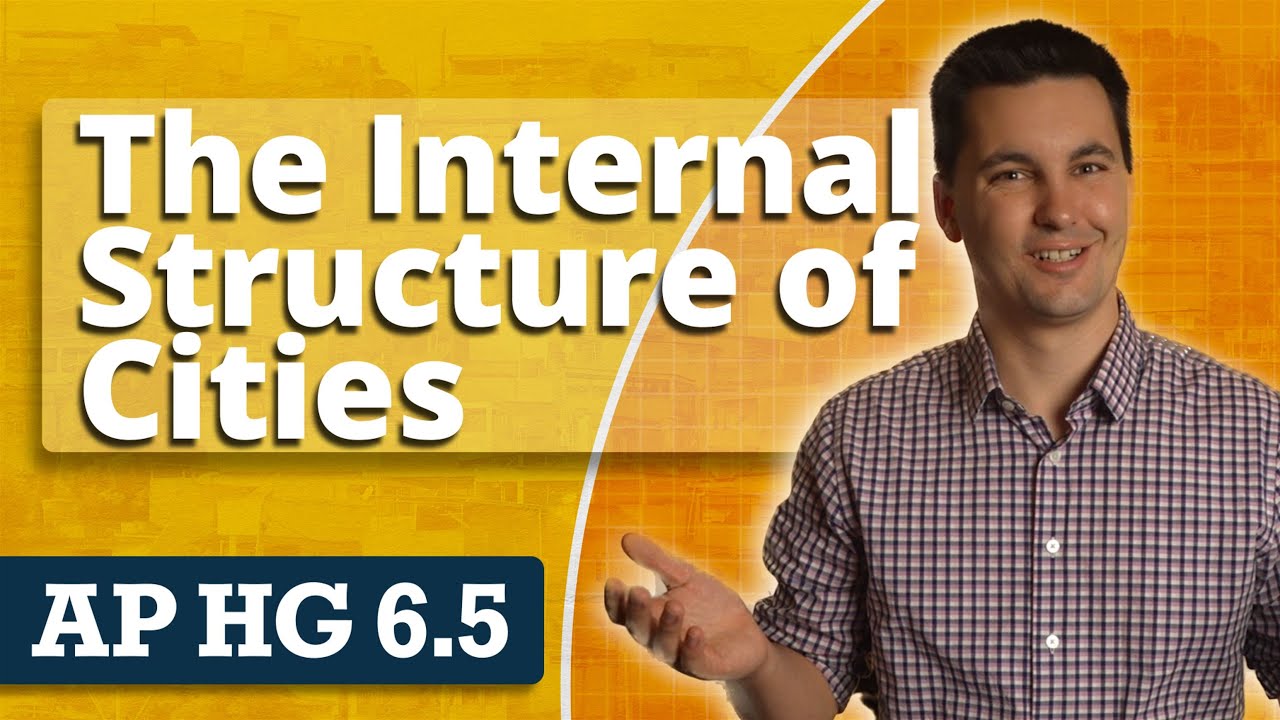
7 Urban Models Every APHG Student Must Know! [AP Human Geography Unit 6 Topic 5]
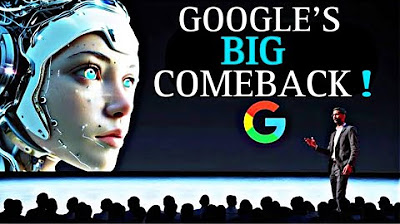
Google's Upgraded Gemini Pro 0801 Surpasses GPT-4 and Shakes Up the Industry!

Geostrategi_ TanNas [3]
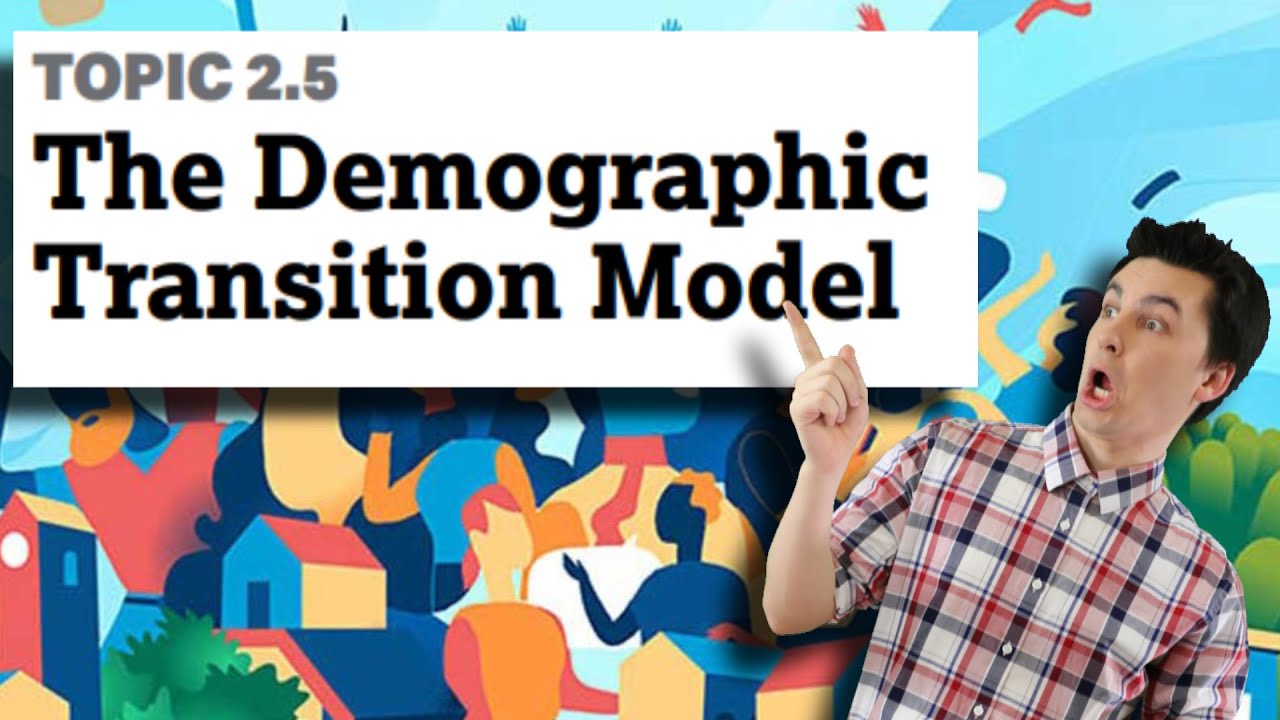
Demographic & Epidemiological Transition Model [AP Human Geography Unit 2 Topic 5] (2.5)
5.0 / 5 (0 votes)