Source of Bias
Summary
TLDRThis video script discusses the various stages where bias can infiltrate the AI and machine learning pipeline, from data collection to model deployment. It emphasizes the importance of considering representativeness in data, annotator beliefs, and the potential for biased metrics like accuracy on unbalanced data. The script also touches on user perception of bias and the feedback loop from user behavior to further data collection. Interactive examples illustrate how prompts can lead to unexpected AI outputs, highlighting the need for critical thinking about bias in AI models. The module includes hands-on activities to explore bias datasets and metrics, encouraging practical understanding and engagement with the topic.
Takeaways
- π Bias can enter at various stages of the AI development pipeline, starting from data collection to model deployment.
- π The representativeness of collected data across different demographics is crucial to avoid bias.
- π·οΈ Data labeling involves annotators whose beliefs and geographical origins can influence the labeling process, potentially introducing bias.
- π Training models with biased data or using metrics like accuracy on unbalanced data can result in biased models.
- π Once a model is deployed, user interactions can affect its performance and may reveal biases in unexpected ways.
- π€ Users might perceive bias even when it's not present, which is an important consideration for model evaluation.
- π User behavior can inform further data collection, creating a feedback loop that can either mitigate or exacerbate bias.
- πΌοΈ The script discusses the potential for AI vision models to misinterpret prompts, leading to outputs that may not align with reality.
- π It highlights the importance of questioning the representation and accuracy of AI model outputs, using examples of house images from different countries.
- π οΈ The module includes hands-on activities to understand and study bias, encouraging learners to engage with datasets and metrics.
- π Supplemental video content and live sessions are provided for further exploration of bias topics, emphasizing the importance of practical understanding.
Q & A
What is the main focus of the video script?
-The main focus of the video script is to discuss the various sources of bias in the AI and machine learning pipeline, from data collection to model deployment and user interaction.
Why is data representativeness important during data collection?
-Data representativeness is important because it ensures that the model is trained on a diverse set of data that reflects all demographics, which can help to prevent biased outcomes.
What factors could influence the labeling of data for model training?
-Factors that could influence data labeling include the annotators' beliefs, their cultural background, and the part of the world they are from, which might introduce bias into the training data.
What is the potential issue with using accuracy as a metric on unbalanced data?
-Using accuracy as a metric on unbalanced data can lead to biased models because it may not accurately reflect the model's performance across different classes, especially the minority ones.
How can user behavior impact the AI model after deployment?
-User behavior can impact the AI model by providing feedback that may indicate perceived bias, even if the model is not actually biased, which can affect user trust and model usage.
What is the role of the feedback loop in the context of AI model deployment?
-The feedback loop allows for continuous monitoring and improvement of the AI model based on user interactions and perceptions, helping to identify and mitigate biases over time.
Why is it important to question the prompts used for AI model outputs?
-Questioning the prompts is important because it helps to understand the context and potential biases that might have influenced the AI's output, ensuring a more critical evaluation of the model's performance.
What does the script suggest about the image of an 'Indian person' produced by a vision model?
-The script suggests that the image produced by the vision model might not accurately represent all Indian people, as it may be based on a stereotype or limited data, highlighting the issue of representation in AI models.
How can the hands-on section of the module help participants understand AI bias?
-The hands-on section allows participants to actively engage with creating datasets and using metrics to study bias, providing practical experience and deeper insights into the mechanisms and impacts of bias in AI.
What is the purpose of the supplement video content mentioned in the script?
-The purpose of the supplement video content is to provide additional information and examples that can enhance understanding of AI bias, encouraging participants to explore the topic further.
What is the next step suggested for participants after watching the module?
-The next step suggested is to watch the supplement video content, engage with the hands-on activities, and participate in live sessions to continue exploring and understanding AI bias.
Outlines
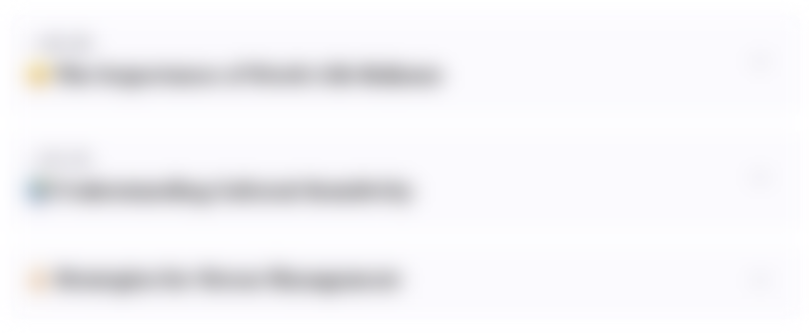
This section is available to paid users only. Please upgrade to access this part.
Upgrade NowMindmap
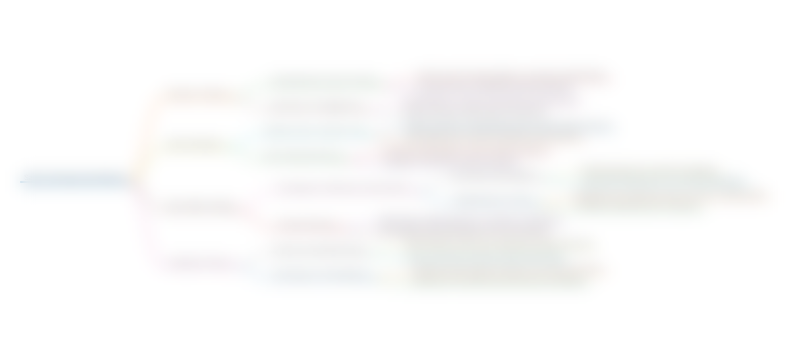
This section is available to paid users only. Please upgrade to access this part.
Upgrade NowKeywords
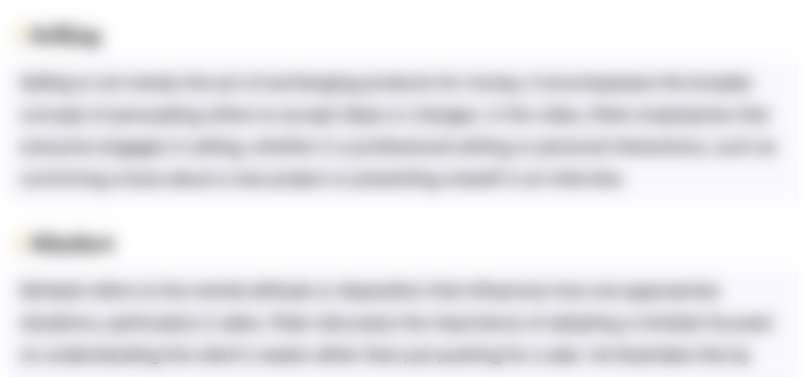
This section is available to paid users only. Please upgrade to access this part.
Upgrade NowHighlights
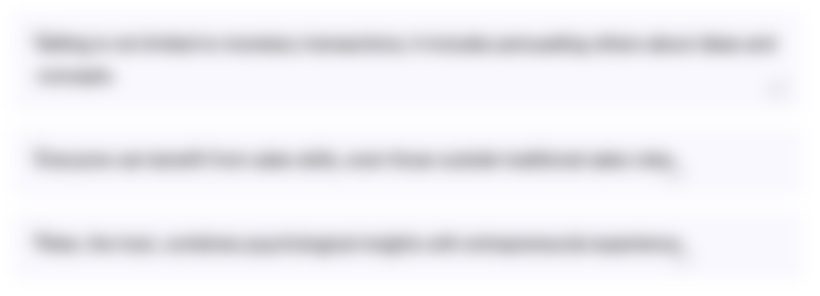
This section is available to paid users only. Please upgrade to access this part.
Upgrade NowTranscripts
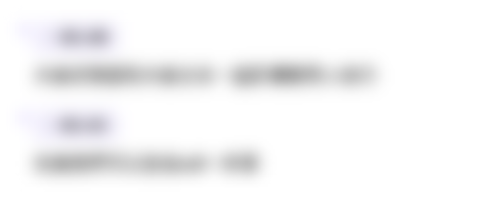
This section is available to paid users only. Please upgrade to access this part.
Upgrade Now5.0 / 5 (0 votes)