Introduction
Summary
TLDRThis transcript presents an introduction to machine learning, covering its history, key developments, and applications. The speaker discusses early advancements, like Perceptrons in the 1950s, and highlights the resurgence of neural networks. The importance of data, learning models, and algorithm design in machine learning is emphasized, with real-world applications in healthcare, computer vision, natural language processing, finance, and fraud detection. The lecture sets the stage for future discussions on various learning approaches and the nuances of representing and solving complex problems.
Takeaways
- 🤖 Introduction to Machine Learning: The script discusses an overview of the course, beginning with a brief introduction to machine learning and its evolution.
- 🧠 Historical Background: Machine learning systems were first developed in the 1950s, with significant milestones like the perceptron in 1957 and the advancement of artificial intelligence in the 1980s.
- 📈 Evolution of Machine Learning: There has been a resurgence of interest in neural networks, especially with the combination of deep learning and other innovations.
- 💡 Learning Systems: Machine learning involves using input and output data to create models that can solve functions, thereby aiding in learning.
- 🧩 Components of Learning Systems: The script explains that a learning system comprises two main units: learners and logical units, where learners create models from experience and knowledge.
- 🔍 Applications of Machine Learning: Various domains such as medical diagnostics, computer vision, natural language processing, finance, and fraud detection benefit from machine learning.
- ⚙️ Training Data: The process of learning begins with using training data or experiences to optimize functions that help in solving tasks.
- 🧠 Function Representation: Proper representation of the target function and training experience is crucial for the success of machine learning algorithms.
- 📊 Complex Representation: While complex representations can solve more sophisticated problems, they are also more challenging to learn.
- 🎓 Future Learning Topics: The course will delve deeper into different learning algorithms, and students should keep these foundational concepts in mind throughout.
Q & A
What is the main topic discussed in the script?
-The main topic discussed in the script is machine learning, its history, development, and various applications.
What are the four parts mentioned in the first module of the course?
-The script does not specify the exact four parts in the first module, but it mentions that a brief introduction is provided, followed by discussions on various learning methods in subsequent lectures.
How did the concept of machine learning evolve from the initial idea of computer scientists?
-The concept of machine learning evolved from the initial idea of computer scientists to the first machine learning system developed in the 1950s, with significant advancements made in the 1952 with the introduction of the programming ability of machines.
Who is Arthur Samuel and what is his contribution to machine learning?
-Arthur Samuel is known for defining the concept of machine learning in 1952, emphasizing the ability of machines to learn from experience.
What was the role of Rosenblatt in the development of machine learning?
-Rosenblatt introduced the perceptron in 1957, which was designed to simulate basic learning mechanisms in the brain and marked a fundamental step in the development of machine learning.
What happened to the research in machine learning in the 1980s?
-The research in machine learning faced a lull in the 1980s, with advancements in the field being halted until the research was revitalized with the advent of deep learning and neural networks.
What is the significance of the 1990s in the context of machine learning?
-The 1990s saw the introduction of a new powerful machine learning paradigm by Freund, which created strong classifiers from weak ones, marking a significant advancement in the field.
What is the importance of deep learning in recent years?
-Deep learning has become very popular in recent years due to its ability to make significant contributions to various fields by combining with other discoveries, thus enhancing the capabilities of neural networks.
How are machine translation systems mentioned in the script?
-Machine translation systems are mentioned as being very effective, performing better than other numerical machine translation systems, indicating advancements in language processing.
What is the role of the model in machine learning as discussed in the script?
-The model in machine learning is used to generalize from the training data, making predictions or decisions for new, unseen data, thus playing a crucial role in the learning process.
How does the script describe the relationship between a machine's performance and its learning ability?
-The script describes the relationship between a machine's performance and its learning ability through the use of functions and the model's capacity to learn from experience, which can then be applied to improve performance on new tasks.
Outlines
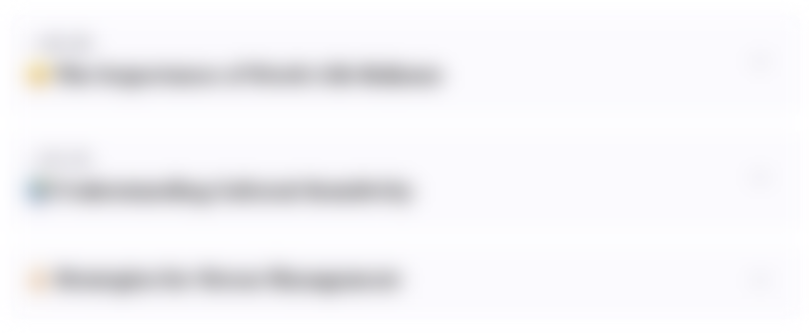
Esta sección está disponible solo para usuarios con suscripción. Por favor, mejora tu plan para acceder a esta parte.
Mejorar ahoraMindmap
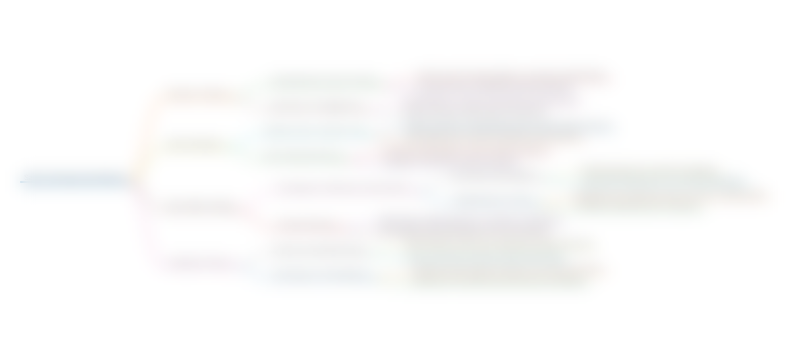
Esta sección está disponible solo para usuarios con suscripción. Por favor, mejora tu plan para acceder a esta parte.
Mejorar ahoraKeywords
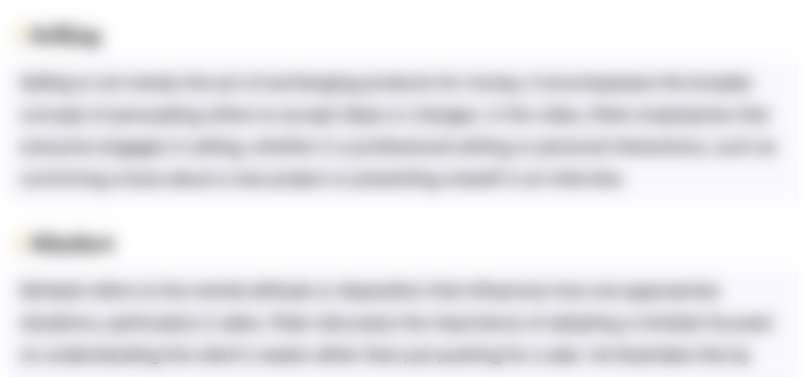
Esta sección está disponible solo para usuarios con suscripción. Por favor, mejora tu plan para acceder a esta parte.
Mejorar ahoraHighlights
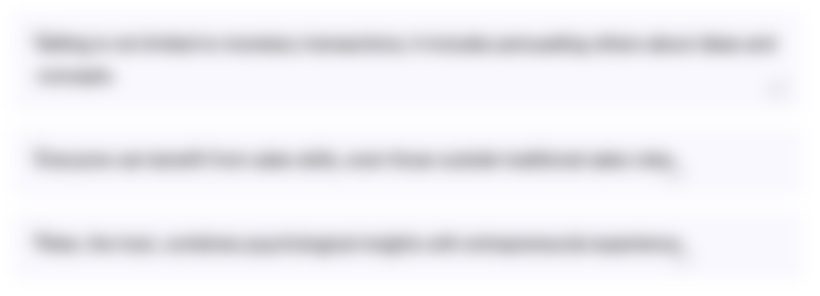
Esta sección está disponible solo para usuarios con suscripción. Por favor, mejora tu plan para acceder a esta parte.
Mejorar ahoraTranscripts
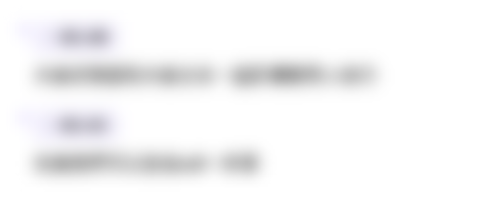
Esta sección está disponible solo para usuarios con suscripción. Por favor, mejora tu plan para acceder a esta parte.
Mejorar ahoraVer Más Videos Relacionados
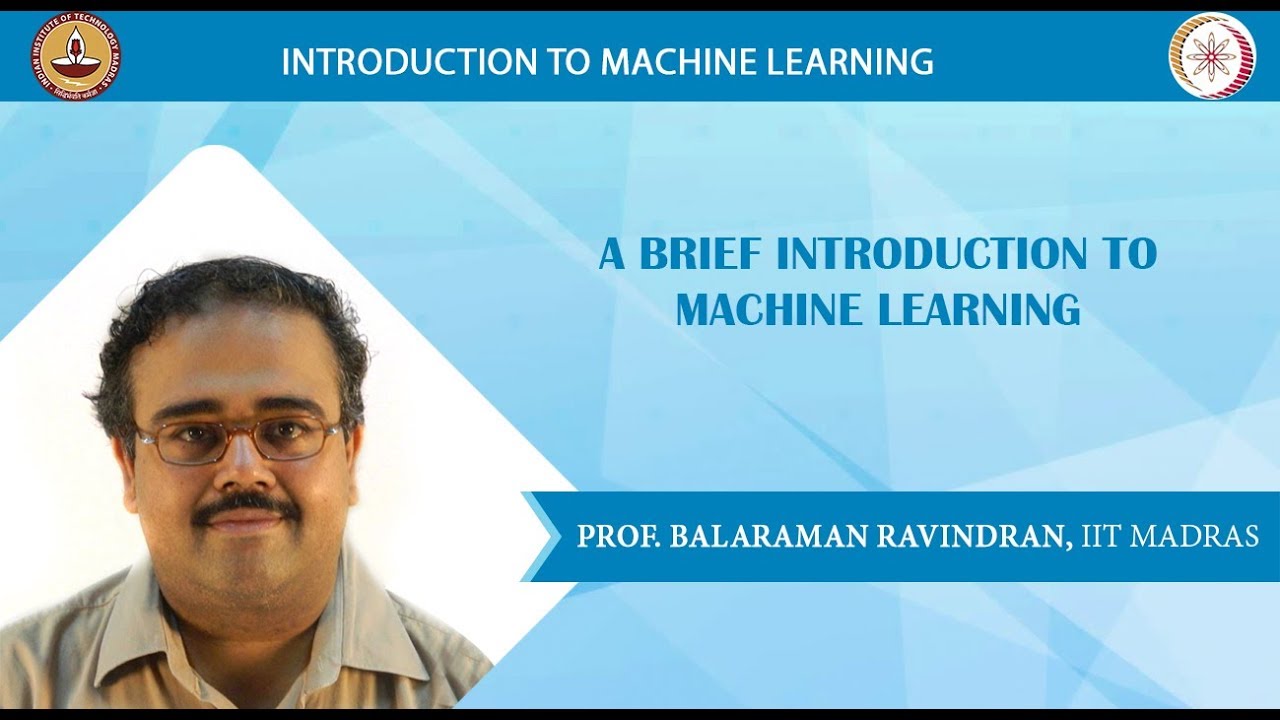
Week 1 - Lecture 1 - Introduction to Machine Learning
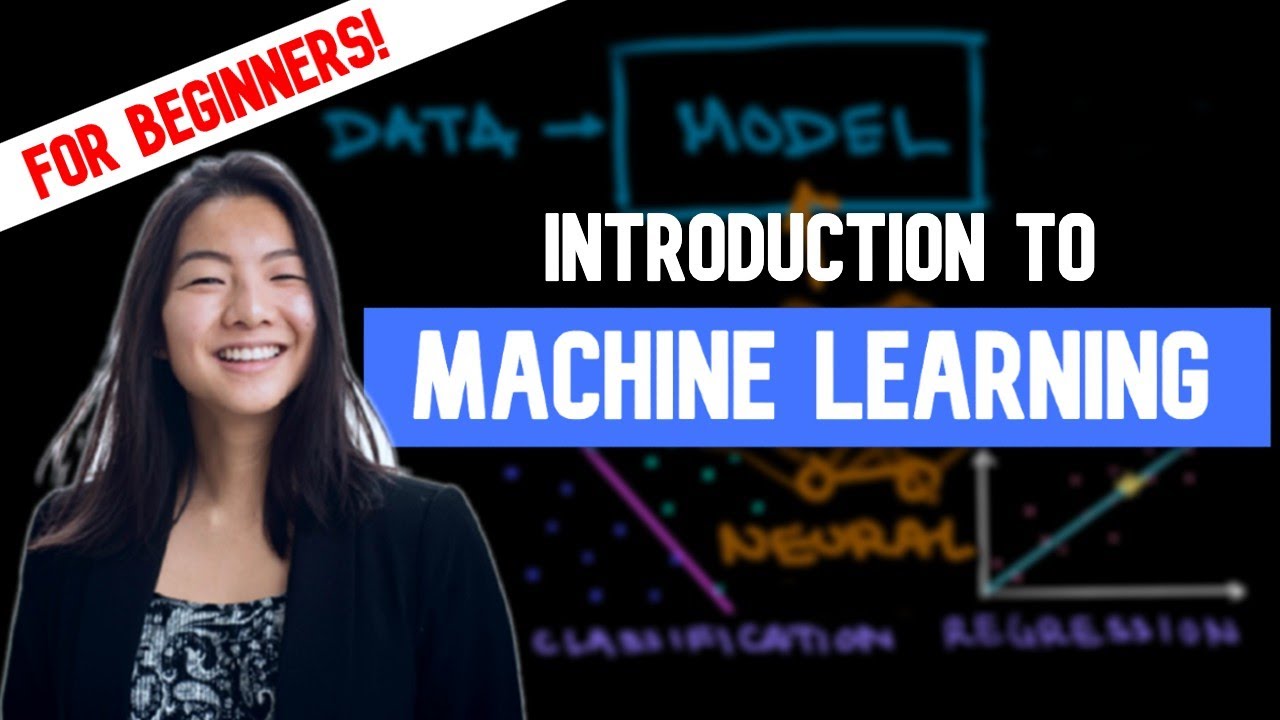
Introduction to ML: What is Machine Learning? | ML for Beginners
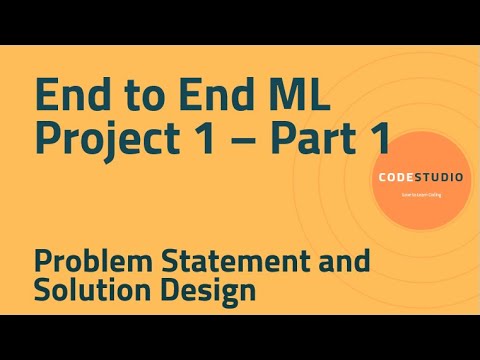
End to End ML Project 1 - P1 - Problem Statement and Solution Design
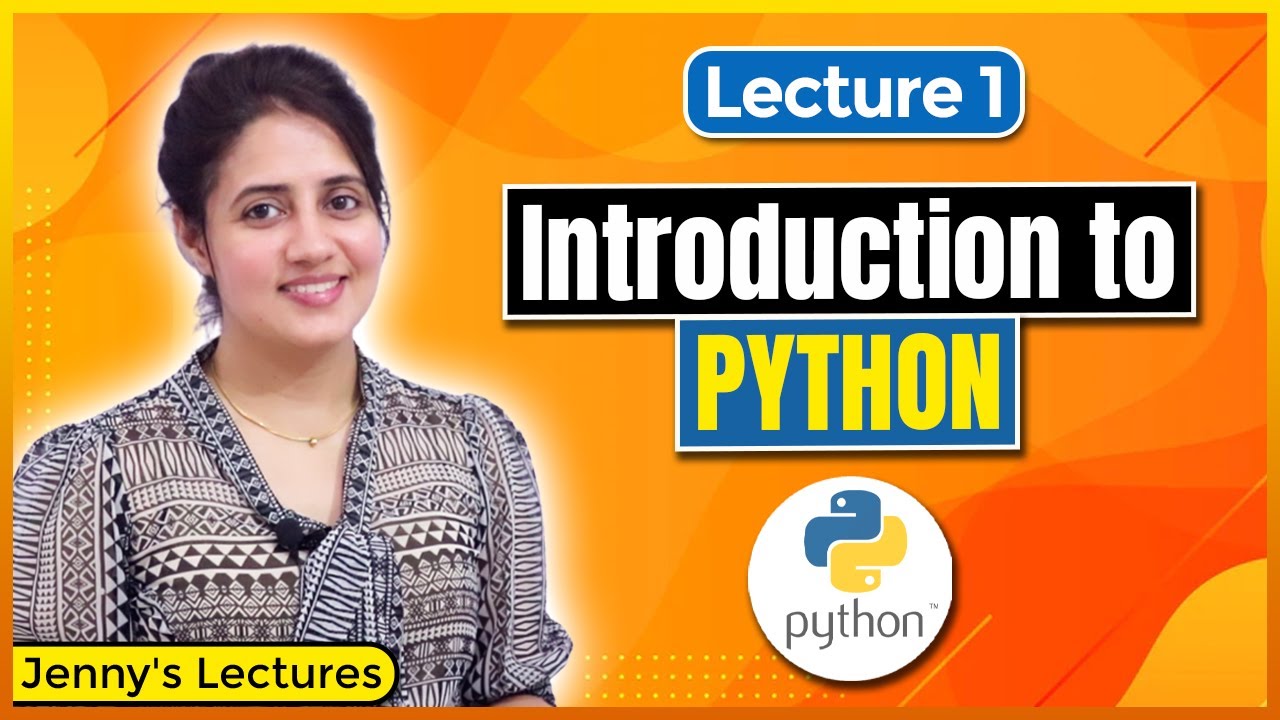
Introduction to Python Programming | Python for Beginners #lec1
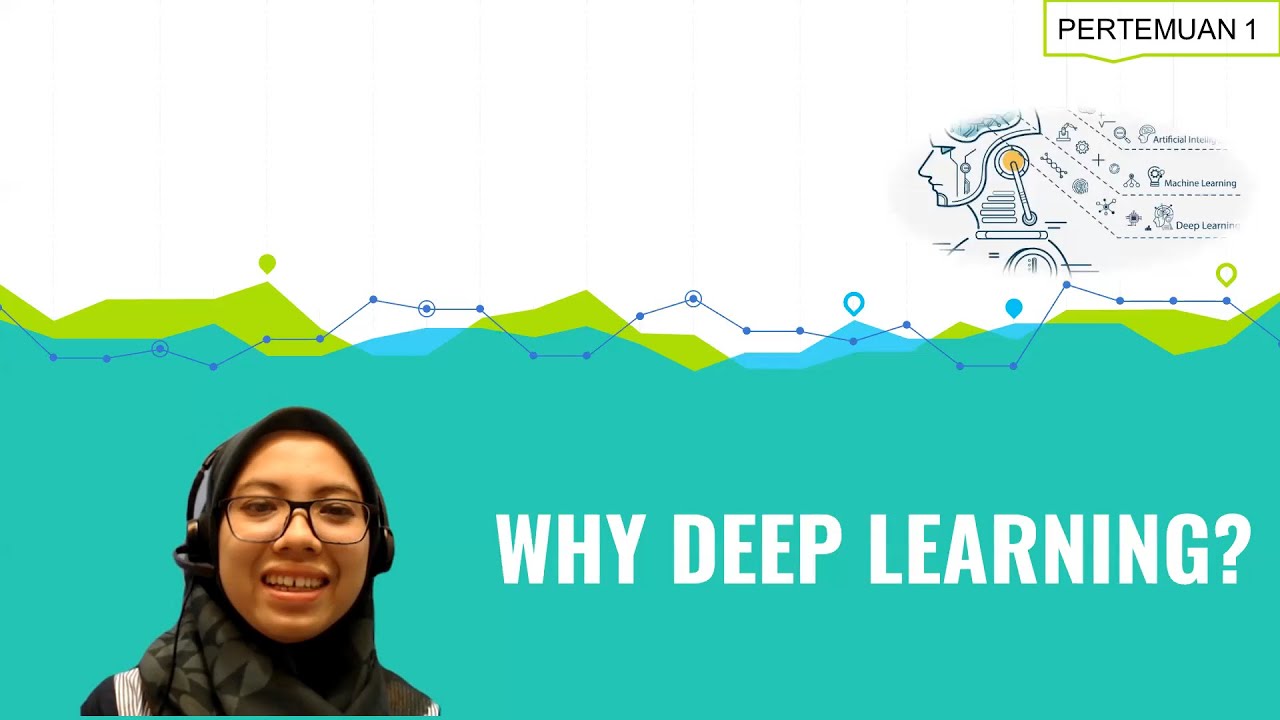
Konsep Cepat Memahami Deep Learning
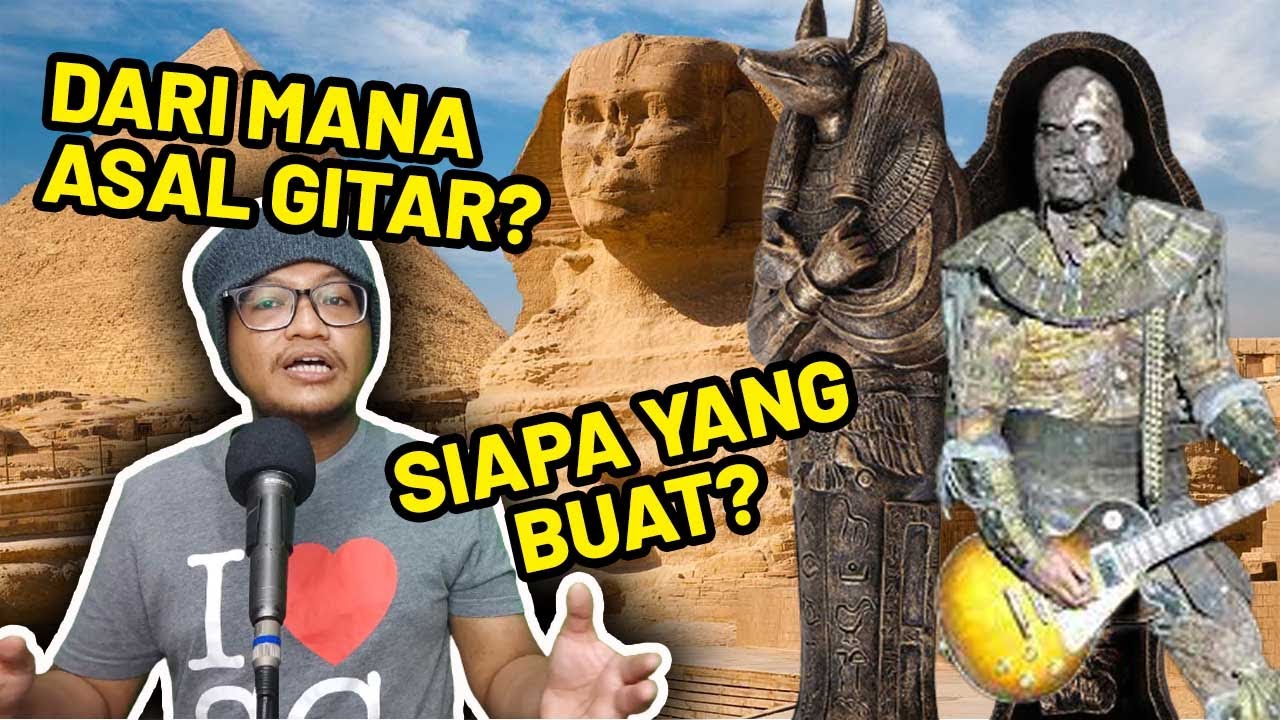
SEJARAH DAN ASAL USUL GITAR YANG TIDAK DIKETAHUI BANYAK ORANG‼
5.0 / 5 (0 votes)