Fine Tuning ChatGPT is a Waste of Your Time
Summary
TLDRThe video script discusses the complexities and potential pitfalls of fine-tuning AI models, suggesting it might be overrated. It highlights the context window problem, where AI struggles with large contexts, and the challenge of defining training data to avoid overtraining. The script introduces Retrieval Augmented Generation (RAG) as a more flexible alternative to fine-tuning, allowing for easier updates and better data control. It concludes by emphasizing the vast potential of RAG and its applications in creating more autonomous AI systems.
Takeaways
- 🔧 Fine-tuning is a technique for customizing AI models but is complex and data-intensive.
- 📈 Major AI companies like OpenAI, AWS, and Microsoft are investing in fine-tuning capabilities.
- 📚 Fine-tuning is popular due to its potential to make AI models more specialized and personalized.
- 🧠 AI models have limitations, particularly with context memory, which fine-tuning aims to address.
- 🔍 Defining the right data for fine-tuning is challenging, as it requires understanding the model's current knowledge gaps.
- 🚧 Overtraining is a risk with fine-tuning, where the model becomes too specialized and loses general applicability.
- 🌐 OpenAI's approach to training uses a diverse corpus from the internet to create more believable responses.
- 🔄 Fine-tuning is static; once trained, the model's knowledge is fixed until retrained.
- 🔑 Retrieval Augmented Generation (RAG) offers a more flexible alternative to fine-tuning by using searchable data chunks.
- 🔄 RAG allows for updating and managing data chunks easily, providing a dynamic approach to AI model enhancement.
- 🛡️ Security is a concern with AI systems, as they can expose sensitive data through user interactions.
- 🚀 RAG opens up possibilities for more advanced AI applications, such as autonomous agents with complex decision-making abilities.
- 📢 The speaker encourages following their platform for more discussions on AI, indicating the ongoing relevance of these topics.
Q & A
What is fine-tuning in the context of AI models?
-Fine-tuning is a technique where an AI model is further trained on a specific dataset to adapt to a particular task or to better suit a user's needs, making it more personalized.
Why is fine-tuning considered complex and data-intensive?
-Fine-tuning is complex and data-intensive because it requires a significant amount of relevant data to effectively retrain the model to perform well on a specific task, and it involves understanding the nuances of the data to avoid issues like overfitting.
How has OpenAI made fine-tuning more accessible?
-OpenAI has made fine-tuning more affordable and provided a series of guides to help users fine-tune their models, making it easier for them to adapt the latest AI models to their needs.
What is the context window problem in AI?
-The context window problem refers to the limitation in the amount of contextual information an AI model can process and remember when generating responses, which can lead to loss of context and understanding in longer conversations or responses.
Why is defining the data for fine-tuning challenging?
-Defining the data for fine-tuning is challenging because it requires identifying what the model does not know and how to provide it with the necessary information without overtraining or causing the model to become too narrowly focused.
What is overtraining in the context of AI models?
-Overtraining occurs when an AI model is trained too much on a specific set of data, leading it to perform well on that data but poorly on new, unseen data, as it fails to generalize well.
How does Retrieval Augmented Generation (RAG) differ from fine-tuning?
-RAG differs from fine-tuning by using smaller chunks of data that can fit within the model's memory space, allowing for more flexible and updatable responses. It involves searching for relevant data chunks to answer questions rather than relying on a pre-trained model's knowledge.
What are the benefits of using Retrieval Augmented Generation over fine-tuning?
-RAG allows for more updatable and flexible responses as it breaks down data into smaller, manageable chunks. It also provides better control over which documents are sent to users, enhancing security and the ability to customize the AI's knowledge base.
How does the security of data differ between fine-tuning and Retrieval Augmented Generation?
-With RAG, there is a stronger ability to control which documents are sent to specific users, allowing for better data security and customization of the AI's knowledge based on user needs. Fine-tuning, on the other hand, locks the model's knowledge in time, making it less adaptable.
What are some potential applications of Retrieval Augmented Generation?
-RAG can be used to develop autonomous agents with the ability to perceive, plan, and act based on stored details about their environment, simulating a more human-like decision-making process in various applications.
How can one stay updated with more insights on AI like the ones discussed in the script?
-One can follow the Disabled Discussion podcast and publication for regular updates and discussions on various AI topics, providing further insights and exploration of AI capabilities.
Outlines
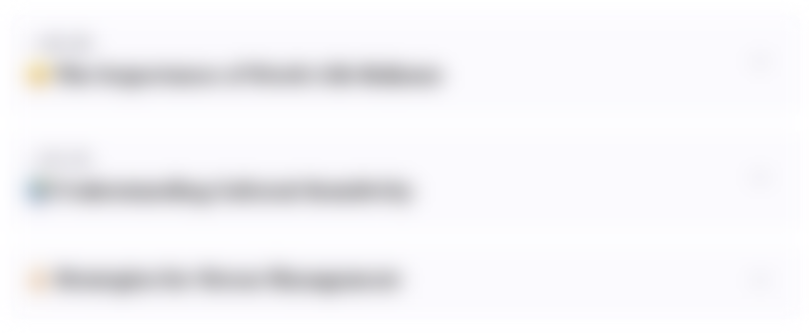
Esta sección está disponible solo para usuarios con suscripción. Por favor, mejora tu plan para acceder a esta parte.
Mejorar ahoraMindmap
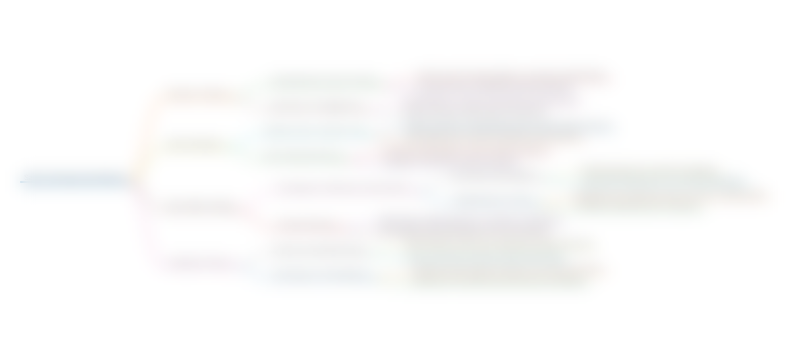
Esta sección está disponible solo para usuarios con suscripción. Por favor, mejora tu plan para acceder a esta parte.
Mejorar ahoraKeywords
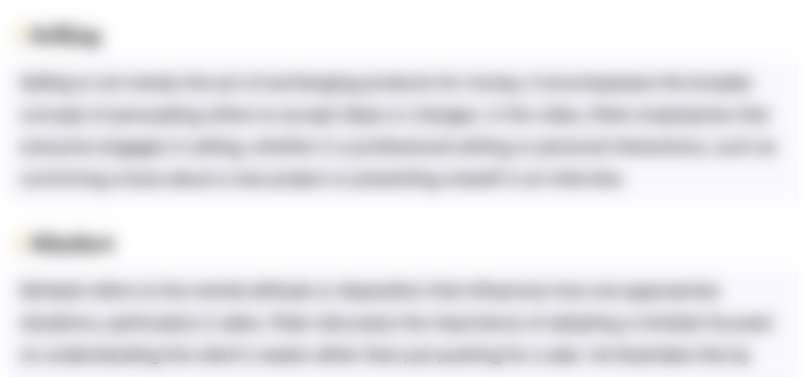
Esta sección está disponible solo para usuarios con suscripción. Por favor, mejora tu plan para acceder a esta parte.
Mejorar ahoraHighlights
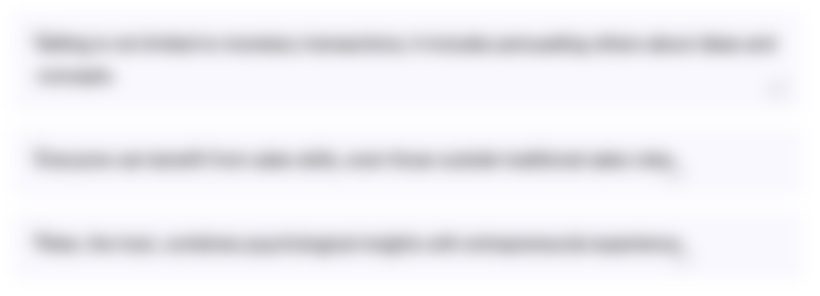
Esta sección está disponible solo para usuarios con suscripción. Por favor, mejora tu plan para acceder a esta parte.
Mejorar ahoraTranscripts
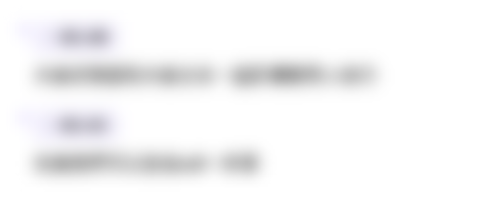
Esta sección está disponible solo para usuarios con suscripción. Por favor, mejora tu plan para acceder a esta parte.
Mejorar ahoraVer Más Videos Relacionados
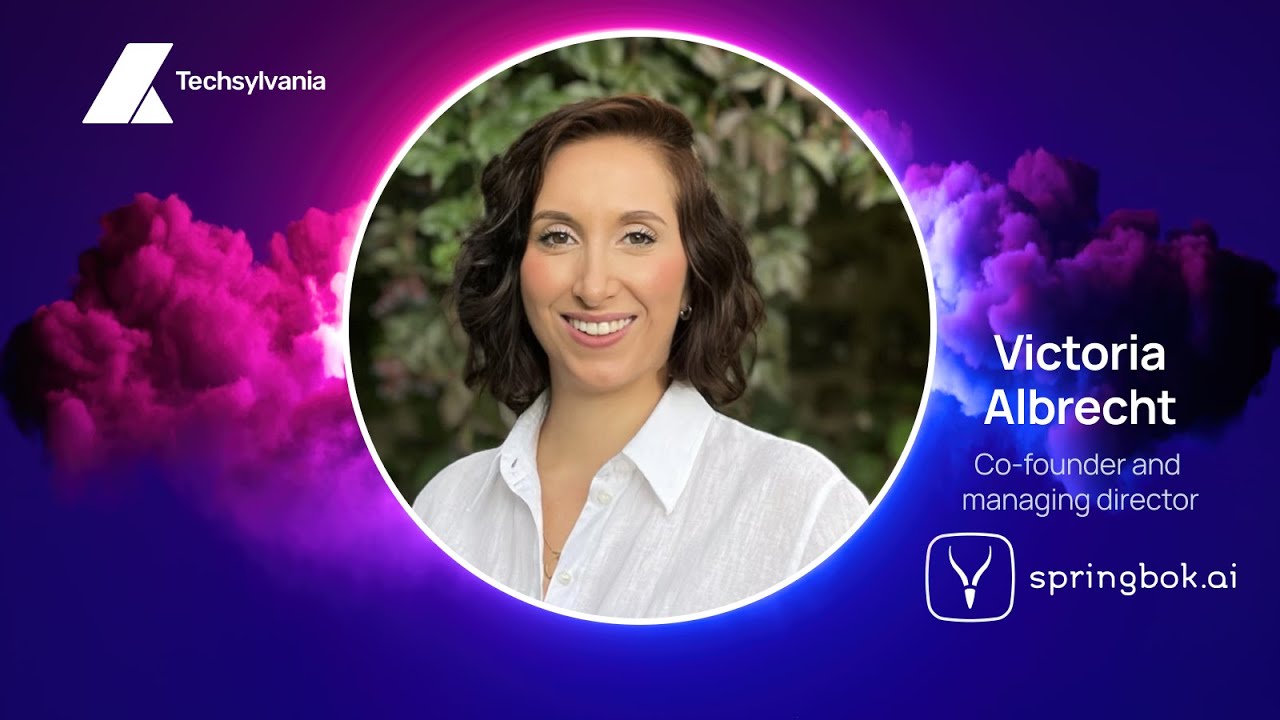
Conversation w/ Victoria Albrecht (Springbok.ai) - How To Build Your Own Internal ChatGPT
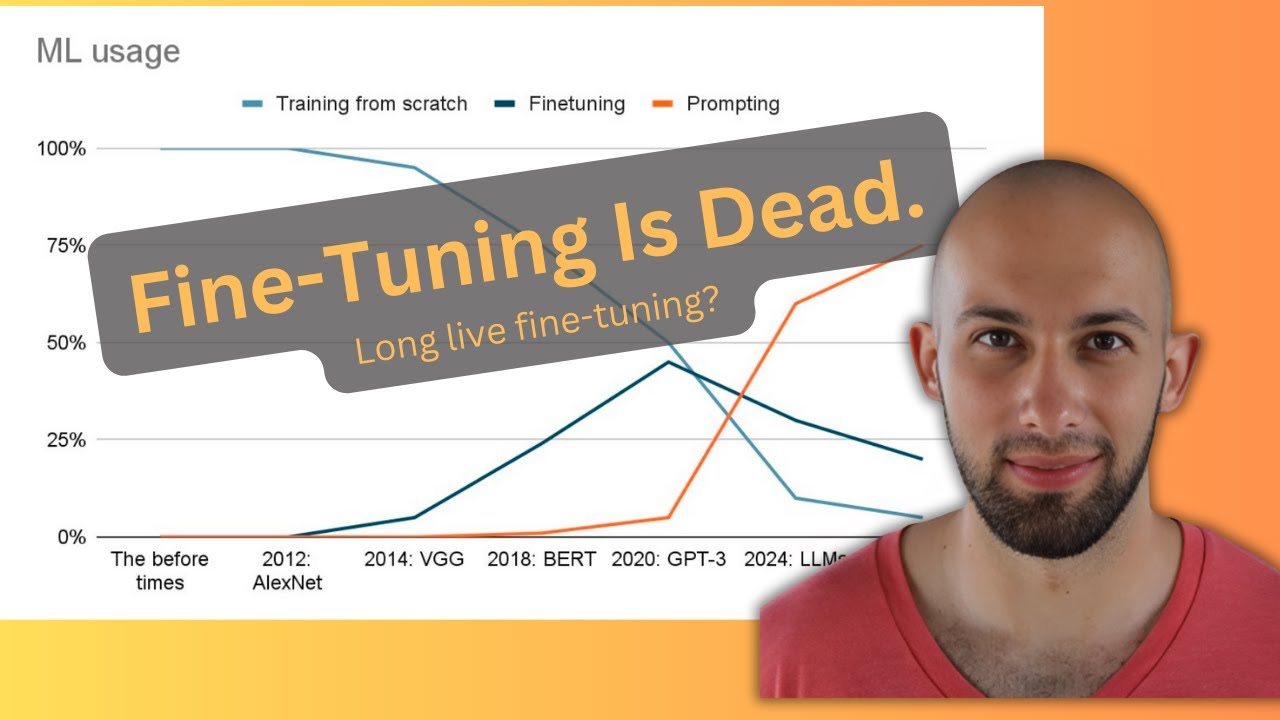
Why Fine Tuning is Dead w/Emmanuel Ameisen
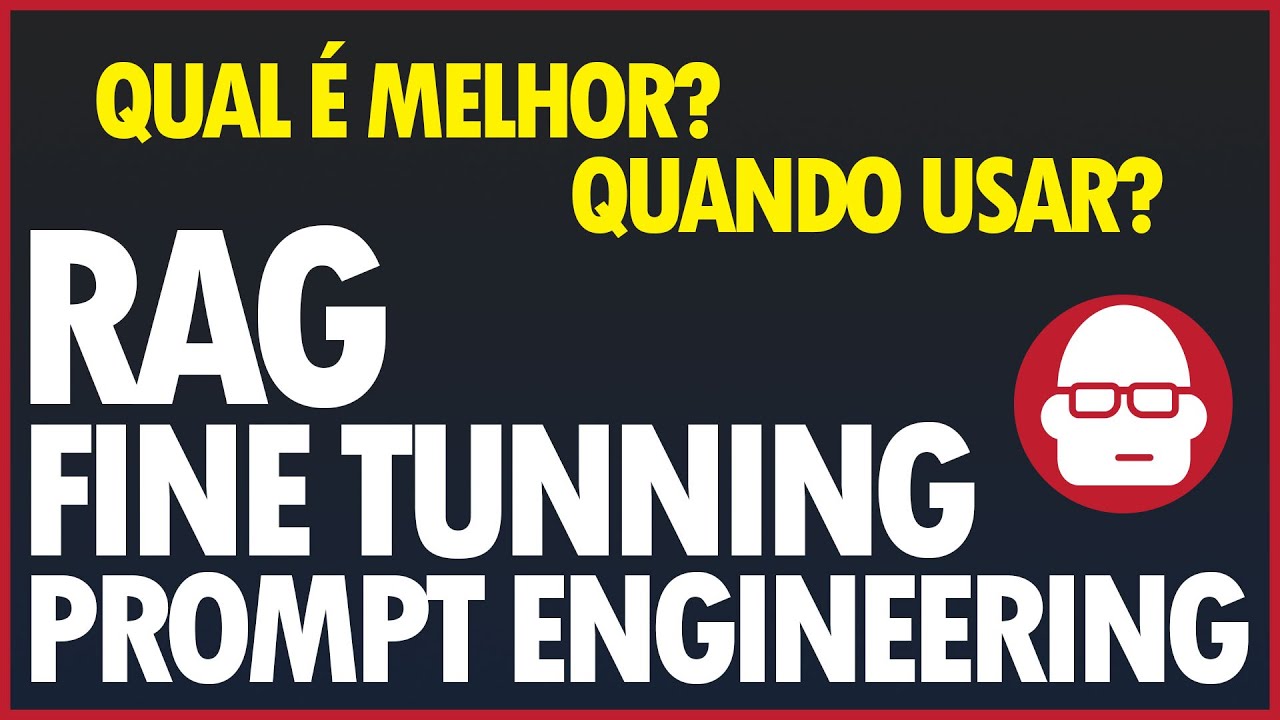
Fine Tuning, RAG e Prompt Engineering: Qual é melhor? e Quando Usar?
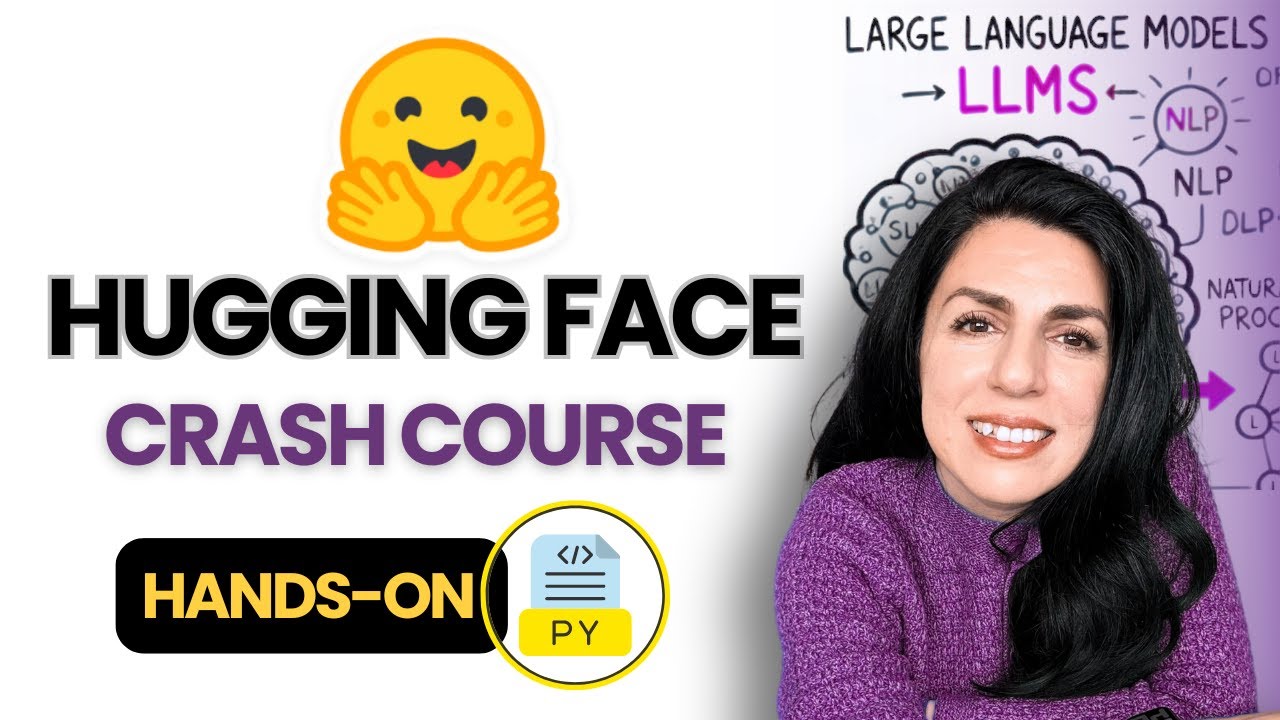
Hands-On Hugging Face Tutorial | Transformers, AI Pipeline, Fine Tuning LLM, GPT, Sentiment Analysis
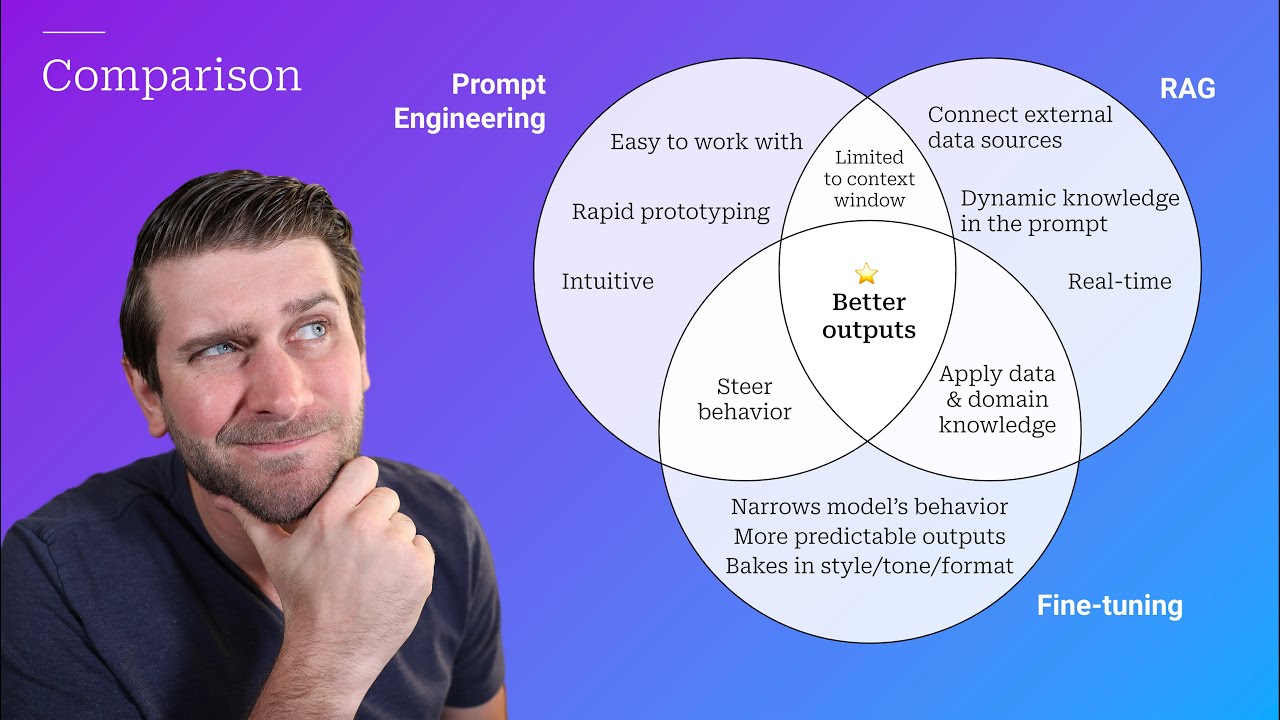
Prompt Engineering, RAG, and Fine-tuning: Benefits and When to Use
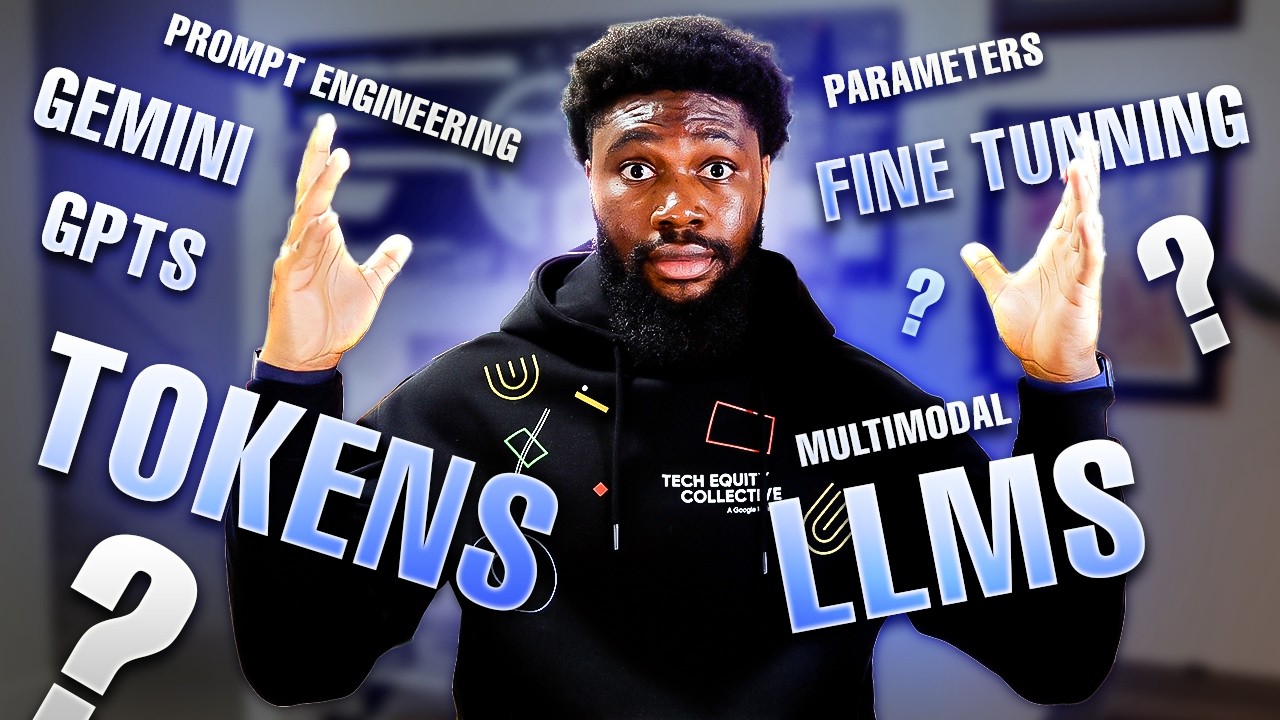
Simplifying Generative AI : Explaining Tokens, Parameters, Context Windows and more.
5.0 / 5 (0 votes)