This is How Easy It Is to Lie With Statistics
Summary
TLDRThis video explores the power and potential misuse of statistics in various scenarios, from marketing strategies like Target's pregnancy prediction algorithm to courtroom cases like the Sally Clark trial. It highlights how statistics can be manipulated or misrepresented, leading to significant consequences in advertising, legal judgments, and public perception.
Takeaways
- 🤰 Target's data analysis identified pregnant customers by analyzing their shopping patterns, leading to targeted marketing strategies.
- 🔍 Statistician Andrew Pole developed an algorithm to predict customers' pregnancy status and due dates, enhancing Target's marketing effectiveness.
- 🤔 Target's approach to sending coupons for baby products was subtle to avoid alarming customers, blending them with unrelated items.
- 😡 A father's initial anger over receiving baby-related coupons turned to embarrassment when he discovered his daughter was indeed pregnant, highlighting the predictive power of Target's algorithm.
- 👵 In a 1964 case, statistics were used in court to calculate the probability of an innocent couple matching witness descriptions, leading to a guilty verdict.
- 👶 The Sally Clark case demonstrated the misuse of statistics in a criminal trial, where the probability of two infants dying from SIDS was misinterpreted, resulting in a wrongful conviction.
- 📊 Misleading statistics can be created by omitting zero as a baseline in graphs, exaggerating differences and potentially influencing public opinion.
- 📈 The UK's advertising standards authority criticized Colgate's claim that '80% of dentists recommend Colgate' due to the misleading nature of the statement.
- 🤔 The difference between a 100% increase in a small number and a small percentage increase can be misleading, as shown in the high school dropout rate example.
- 🔗 Correlation does not necessarily imply causation, as seen in the examples of head lice and health, or ice cream sales and heat strokes.
- 📚 The Simpson's paradox illustrates how data can be misleading when not properly grouped, as seen in the Berkeley graduate school acceptance rates.
Q & A
What was the main challenge that Target presented to statistician Andrew Pole in 2002?
-The challenge was to develop an algorithm using only computers to determine which customers were pregnant, even if they didn't want Target to know, by analyzing their shopping patterns.
What common shopping behaviors did Andrew Pole identify among expectant mothers?
-Andrew Pole noticed behaviors such as an increase in lotion purchases, loading up on vitamins, and buying other pregnancy-related items, which he used to determine the likelihood of customers being pregnant.
How did Target use the information from the algorithm to benefit their marketing strategy?
-Target used the information to send coupons to customers at the right time, corresponding to their pregnancy stages and due dates, even after the baby was born, to enhance their marketing effectiveness.
Why did Target mix pregnancy-related coupons with unrelated products?
-Target mixed these coupons to avoid alarming customers who might not have disclosed their pregnancy, making the coupons seem more natural and less intrusive.
What incident led to the revelation of Target's pregnancy prediction algorithm?
-A man from Minnesota was upset because Target was sending his high school daughter coupons for baby-related items. Later, he realized that the algorithm had correctly predicted his daughter's pregnancy before he knew about it.
Can you explain the famous case of Janet Collins and her husband Malcolm involving the use of statistics in the courtroom?
-Janet Collins and Malcolm were accused of a crime based on witness descriptions. A mathematician calculated the probability of an innocent couple matching all the descriptions, concluding it was less than 1 in 12 million, which influenced the jury to find them guilty.
What is the issue with the claim '80% of dentists recommend Colgate' as used in a 2007 UK advertisement?
-The issue is that the study allowed dentists to recommend more than one toothpaste brand, so while 80% recommended Colgate, it was also true that 100% recommended other brands like Crest, which could mislead consumers.
How can a 100% increase sometimes be misleading when describing changes in percentages?
-A 100% increase can be misleading if the initial percentage is very small, as it might represent only a tiny absolute change. For example, going from 0.0001% to 0.0002% is a 100% increase but represents a very small actual change.
What is the difference between correlation and causation in statistics?
-Correlation indicates a statistical relationship between two variables, while causation implies that one variable causes the other. Just because two things are correlated does not mean one causes the other; they could be caused by a third factor or simply occur together by chance.
Can you provide an example of the misuse of statistics in a legal case?
-The case of Sally Clark is an example where the misuse of statistics led to her wrongful conviction for the murder of her two children. The court used the probability of two SIDS deaths in the same family without considering genetic or environmental factors, which later led to her conviction being overturned.
What is the 'Simpson's Paradox' mentioned in the script, and how can it mislead data interpretation?
-Simpson's Paradox occurs when a trend appears in different groups of data but disappears or reverses when these groups are combined. It can mislead data interpretation by showing a different overall story than the individual group trends.
What is the 'Prosecutor's Fallacy' and how can it lead to incorrect conclusions in legal cases?
-The Prosecutor's Fallacy is the incorrect assumption that the probability of A given B is the same as the probability of B given A. This can lead to incorrect conclusions in legal cases, as it may misrepresent the likelihood of guilt or innocence based on certain characteristics or evidence.
Why are bar graphs that don't start at zero potentially misleading?
-Bar graphs that don't start at zero can exaggerate differences between data points, making small changes appear much larger than they actually are. This can be used to mislead viewers by distorting the perception of the data's scale.
Outlines
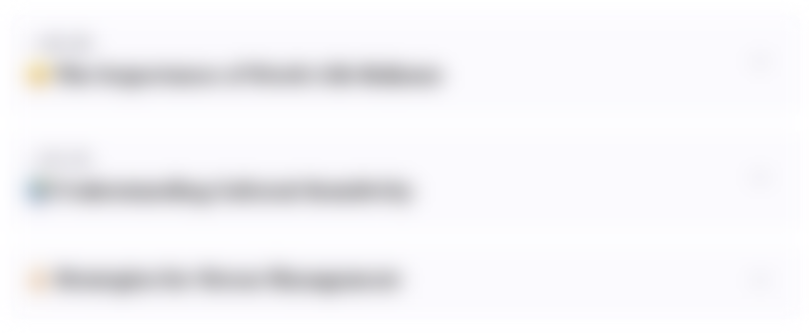
Esta sección está disponible solo para usuarios con suscripción. Por favor, mejora tu plan para acceder a esta parte.
Mejorar ahoraMindmap
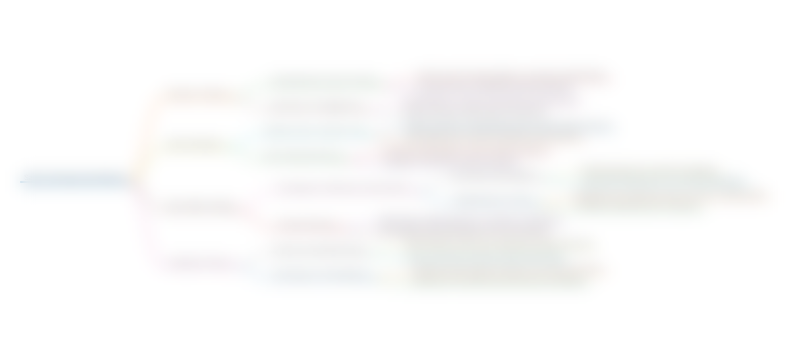
Esta sección está disponible solo para usuarios con suscripción. Por favor, mejora tu plan para acceder a esta parte.
Mejorar ahoraKeywords
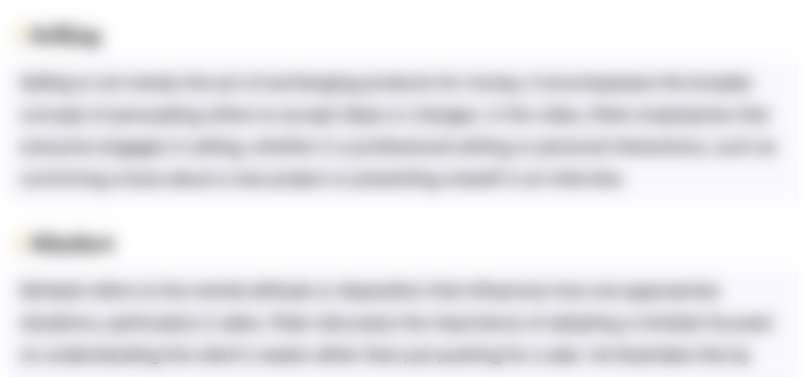
Esta sección está disponible solo para usuarios con suscripción. Por favor, mejora tu plan para acceder a esta parte.
Mejorar ahoraHighlights
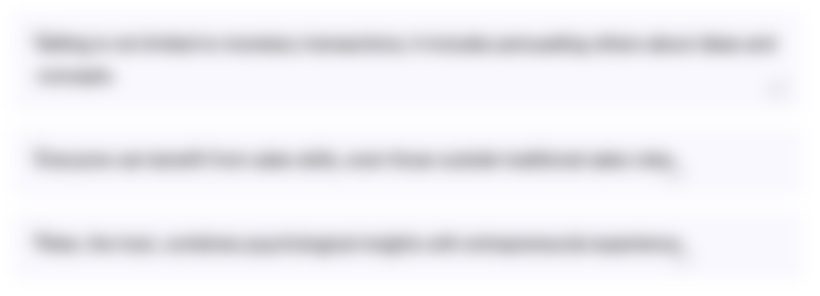
Esta sección está disponible solo para usuarios con suscripción. Por favor, mejora tu plan para acceder a esta parte.
Mejorar ahoraTranscripts
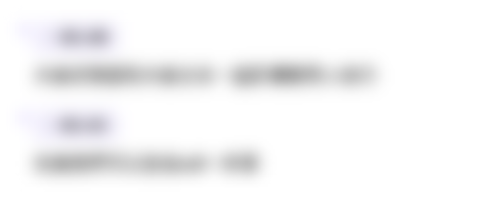
Esta sección está disponible solo para usuarios con suscripción. Por favor, mejora tu plan para acceder a esta parte.
Mejorar ahoraVer Más Videos Relacionados
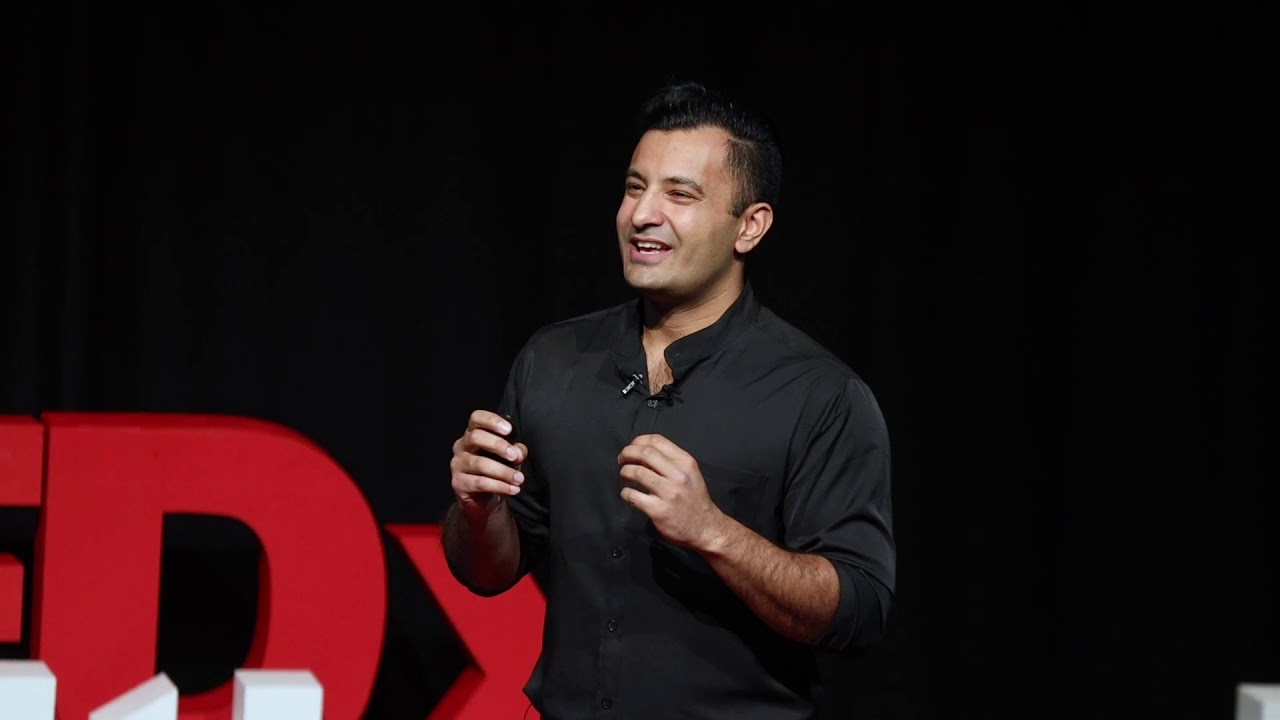
Neuromarketing and the Future of A.I. Driven Behavior Design | Prince Ghuman | TEDxHultLondon
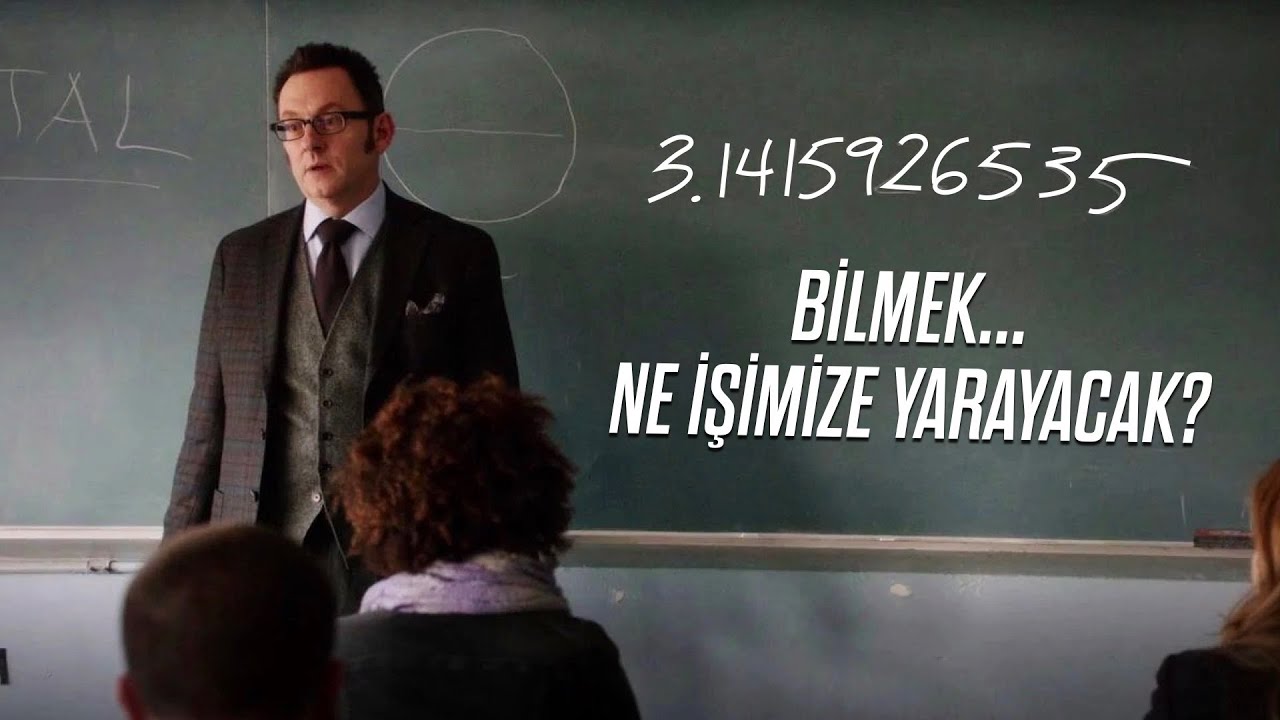
Bilmek... Ne işimize yarayacak?
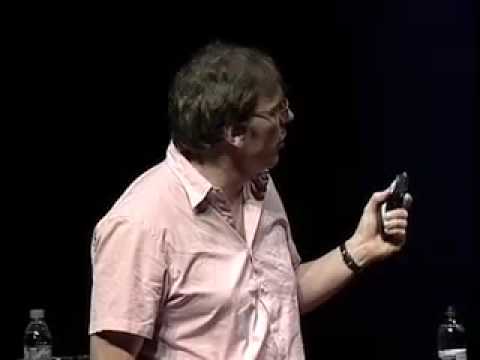
Peter Donnelly: How stats fool juries
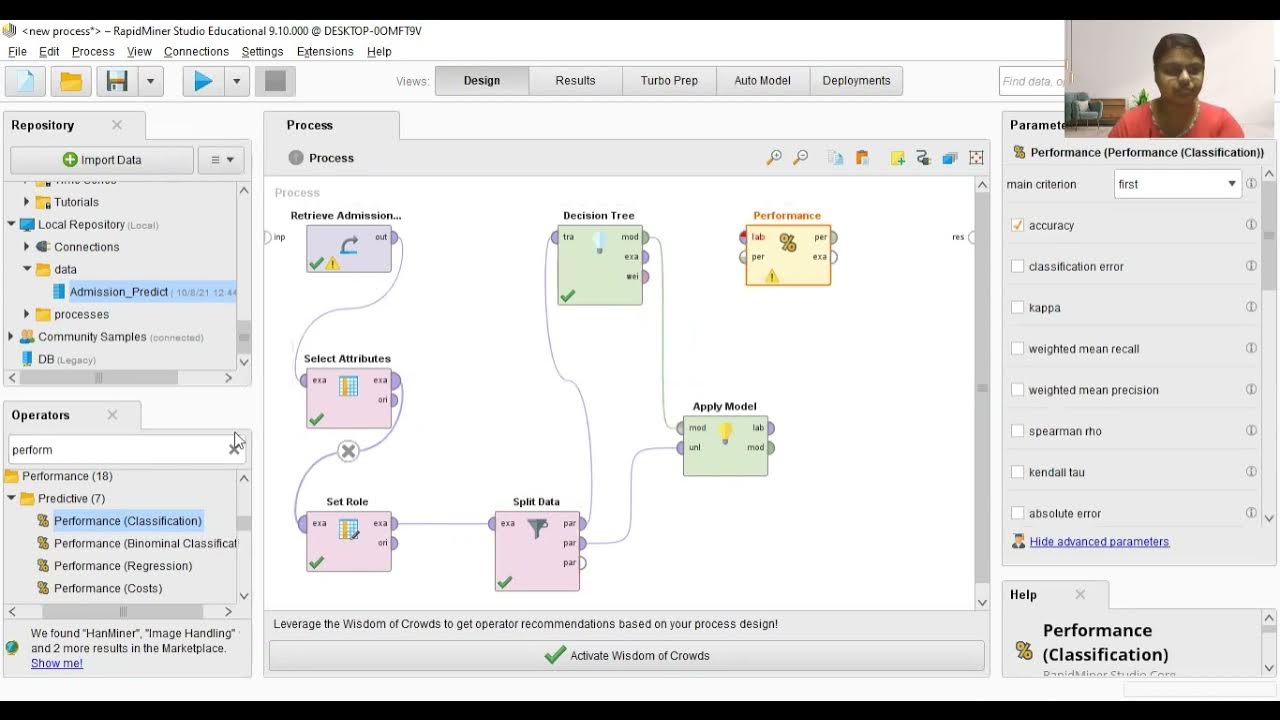
Decision Tree and Logistic Regression using RapidMiner Studio ( Gyanadipta Mohanty 19BCE1224)
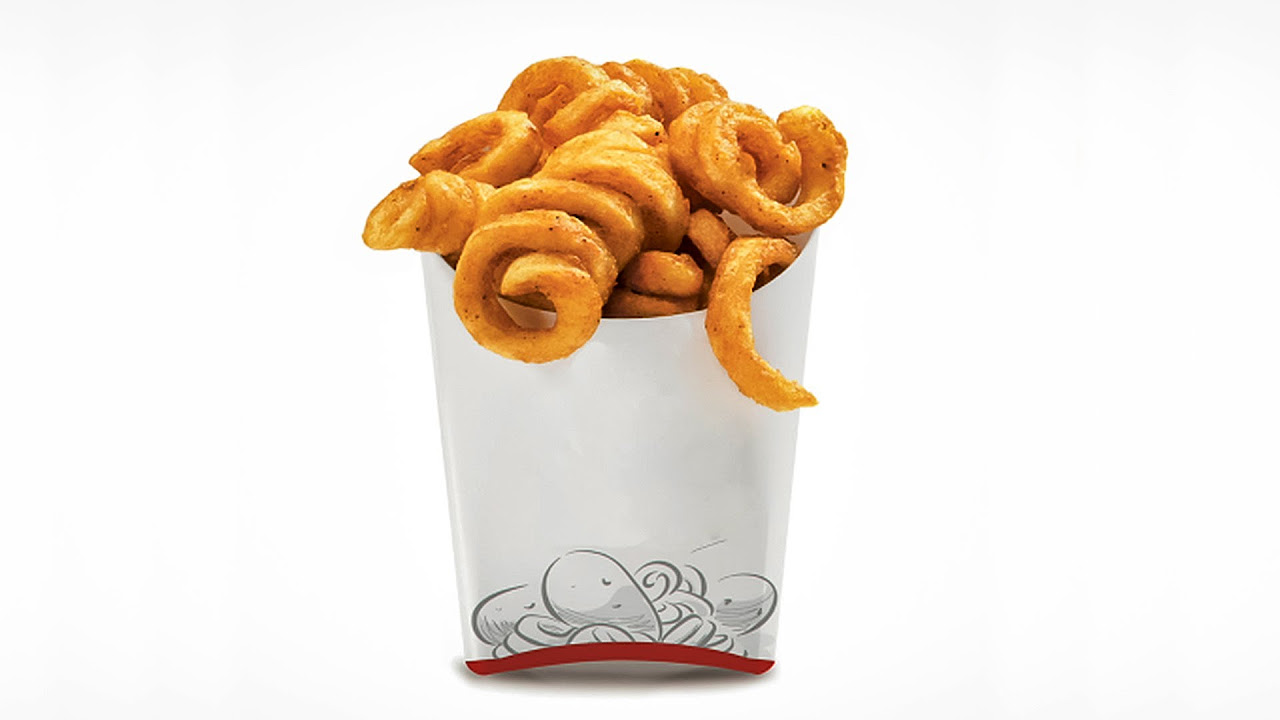
Jennifer Golbeck: The curly fry conundrum: Why social media "likes" say more than you might think
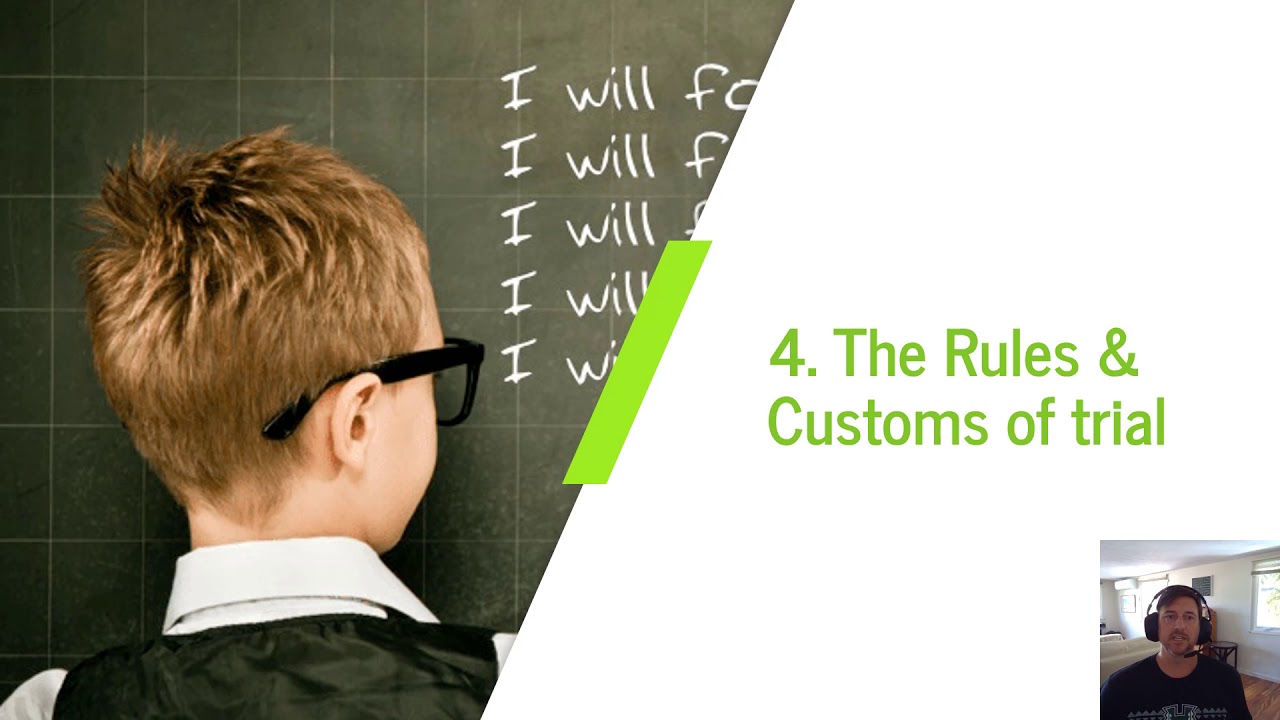
1.2 Mock Trial Basics (Intro to Mock Trial program)
5.0 / 5 (0 votes)