Create a LOCAL Python AI Chatbot In Minutes Using Ollama
Summary
TLDRIn this tutorial, you'll learn how to create a Python-based AI chatbot that runs locally using the Llama model. The process begins with downloading and installing Llama, verifying the setup, and running a basic model test. You'll then set up a virtual Python environment, install necessary dependencies, and write the Python code to interact with the model. The script includes creating a user-friendly chatbot interface that stores conversation history. By the end of the tutorial, you'll have a fully functional local AI chatbot that doesn't require external APIs or subscriptions.
Takeaways
- 😀 Download and install Llama from llama.com to run open-source language models locally on your machine.
- 😀 Test the Llama installation by running the command 'au llama' in the terminal to ensure it’s working.
- 😀 Choose and download a model (e.g., llama3 with 8 billion parameters) using the command 'llama pull llama3'.
- 😀 Ensure your system meets the hardware requirements (e.g., 8GB RAM for smaller models, more for larger ones).
- 😀 Use Python to interact with the model by importing the Llama library and invoking the model with a prompt.
- 😀 Create a virtual environment to isolate dependencies using the command 'python3 -m venv chatbot'.
- 😀 Activate the virtual environment with the appropriate command based on your operating system (Linux, Mac, Windows).
- 😀 Install required Python packages like 'langchain' and 'llama' inside the virtual environment.
- 😀 Write a Python script to interact with the model, invoking responses based on user input, such as 'Hello World'.
- 😀 Enhance the chatbot's functionality by passing context and creating dynamic conversation templates with LangChain.
- 😀 Handle continuous conversation by storing context and history to make the chatbot aware of previous exchanges.
- 😀 Test the chatbot by running the Python script and interacting with the model, including providing an option to exit.
Q & A
What is the first step in setting up the local AI chatbot?
-The first step is to download and install the LLaMA software from alama.com, which will allow you to run local models on your machine.
How do you test if the LLaMA software is installed correctly?
-To test if LLaMA is installed correctly, open a terminal or command prompt and type 'au llama'. If the software runs without issues, the installation is successful.
What is the purpose of the 'llama pull' command?
-The 'llama pull' command is used to download a specific LLaMA model, such as the LLaMA 3 model with 8 billion parameters, to your local machine.
How do you check if the LLaMA model has been downloaded correctly?
-You can verify the successful download of the model by running 'llama run llama3' in the terminal. This will initiate the model and provide a response.
What does the virtual environment in Python help with?
-A virtual environment helps isolate project dependencies, allowing you to manage packages for the AI chatbot without affecting other Python projects on your system.
What is the command to create a Python virtual environment on a Mac or Linux machine?
-On Mac or Linux, you can create a virtual environment by running 'python3 -m venv chatbot'. This will create a folder named 'chatbot' to store isolated dependencies.
How do you activate a virtual environment on Windows?
-On Windows, you can activate the virtual environment by running 'chatbot\Scripts\activate' in the command prompt or 'chatbot\Scripts\Activate.ps1' in PowerShell.
What dependencies are required to build the chatbot?
-You need to install the 'langchain', 'langchain-llama', and 'langchain-alama' packages in your virtual environment to work with the LLaMA model in Python.
How does the Langchain library help in building the AI chatbot?
-Langchain provides utilities for creating and chaining prompts to interact with LLaMA models more easily. It allows you to format input and output, and manage conversation context.
How do you maintain conversation history in the AI chatbot?
-Conversation history is maintained by storing the user's input and the bot's responses in a 'context' variable, which is passed to the model for more context-aware responses in subsequent exchanges.
Outlines
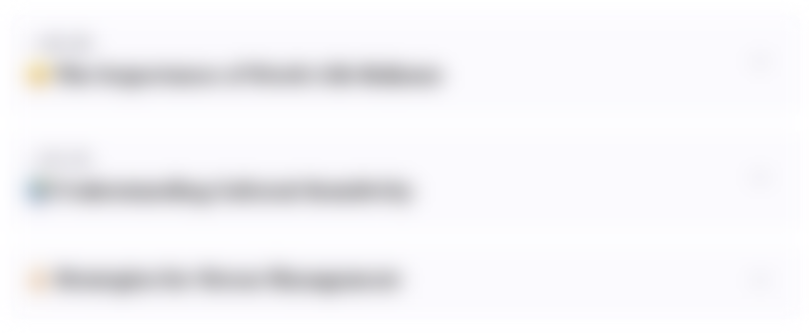
Esta sección está disponible solo para usuarios con suscripción. Por favor, mejora tu plan para acceder a esta parte.
Mejorar ahoraMindmap
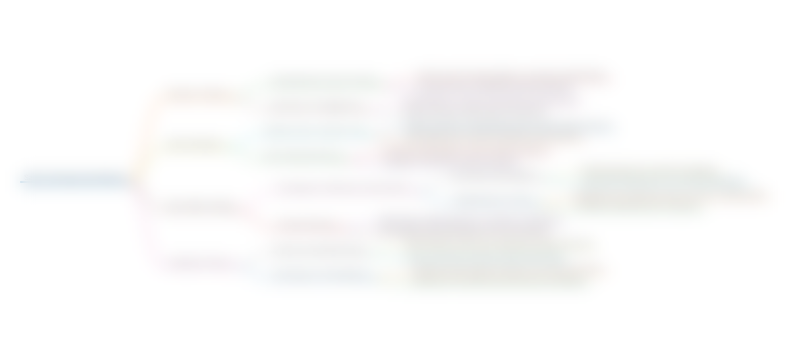
Esta sección está disponible solo para usuarios con suscripción. Por favor, mejora tu plan para acceder a esta parte.
Mejorar ahoraKeywords
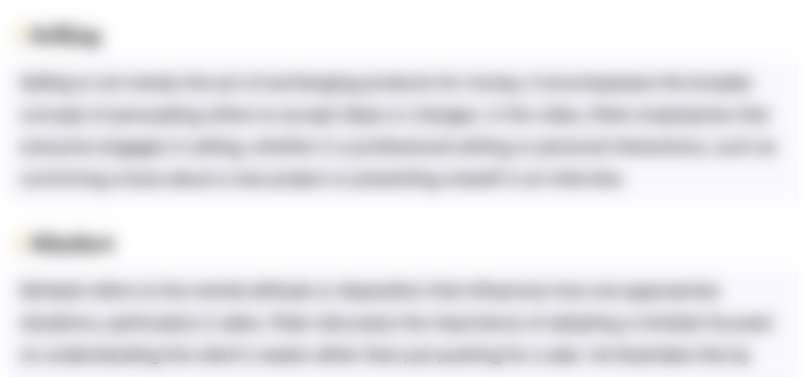
Esta sección está disponible solo para usuarios con suscripción. Por favor, mejora tu plan para acceder a esta parte.
Mejorar ahoraHighlights
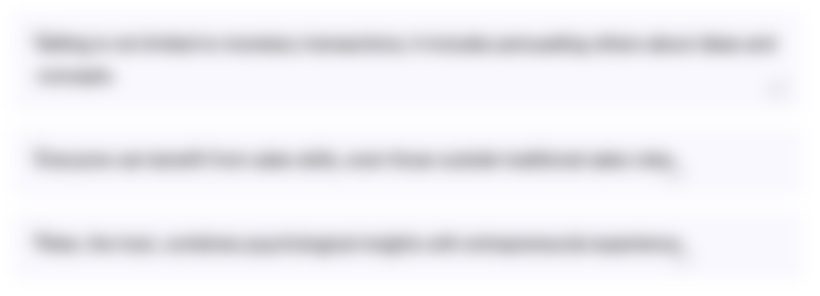
Esta sección está disponible solo para usuarios con suscripción. Por favor, mejora tu plan para acceder a esta parte.
Mejorar ahoraTranscripts
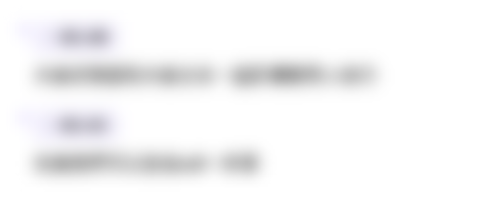
Esta sección está disponible solo para usuarios con suscripción. Por favor, mejora tu plan para acceder a esta parte.
Mejorar ahoraVer Más Videos Relacionados
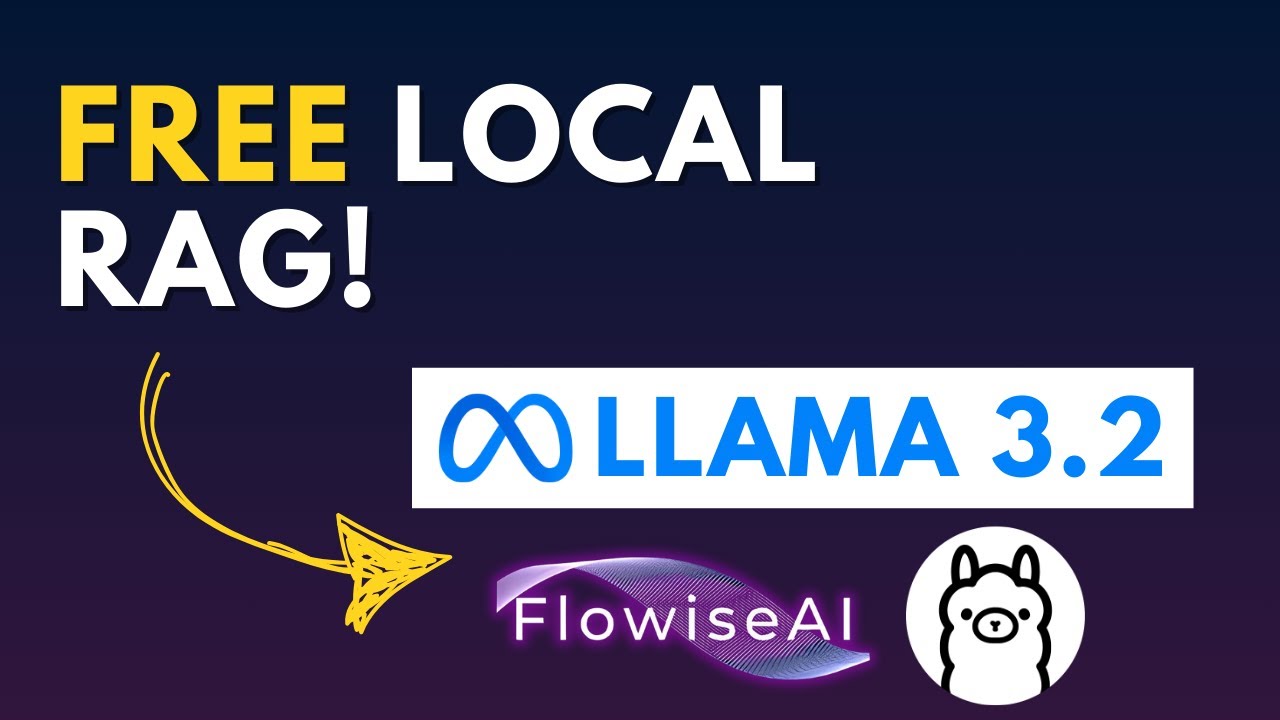
Build a FREE AI Chatbot with LLAMA 3.2 & FlowiseAI (NO CODE)
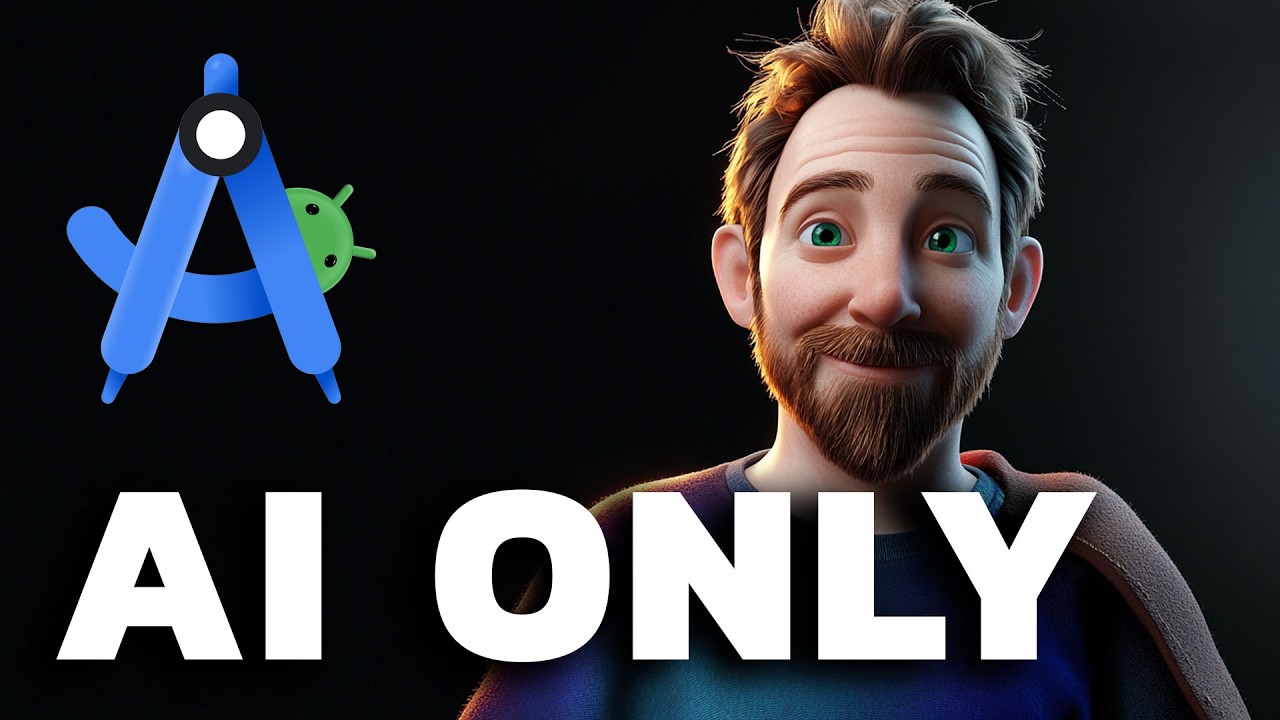
I Made an Android App in MINUTES with This AI Tool
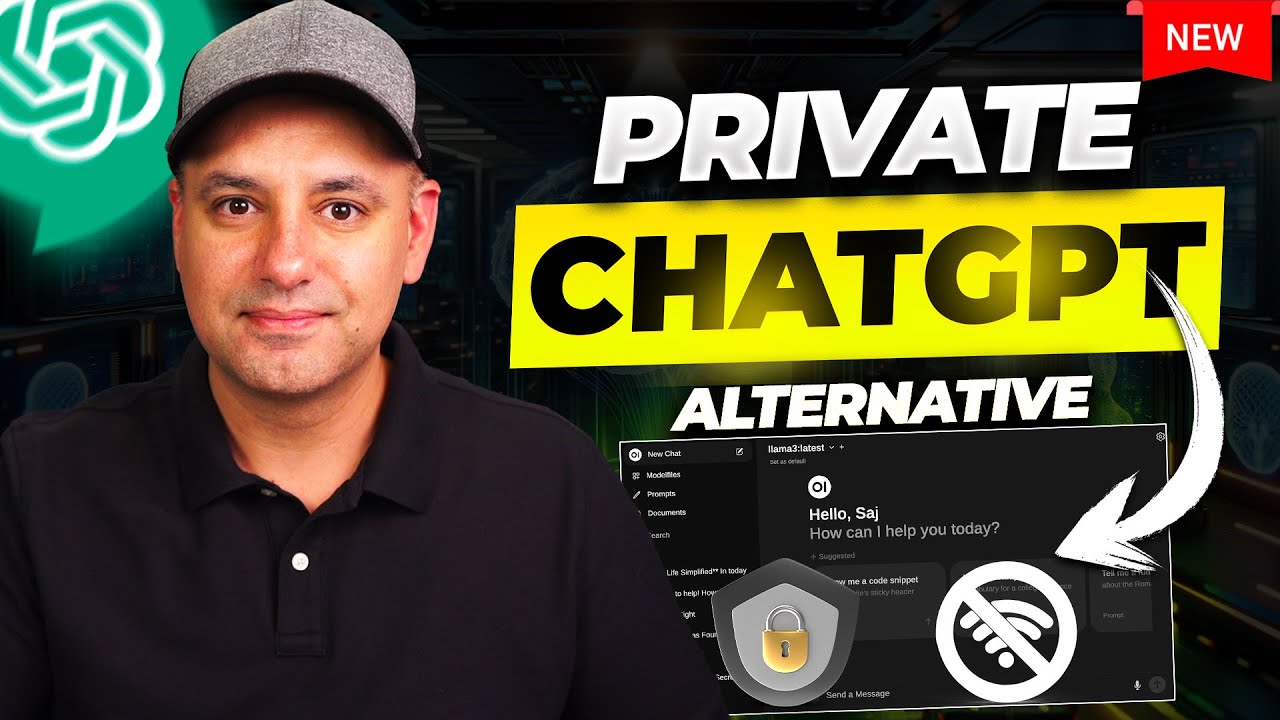
Private AI Chatbot on Your Computer - Step by Step Tutorial
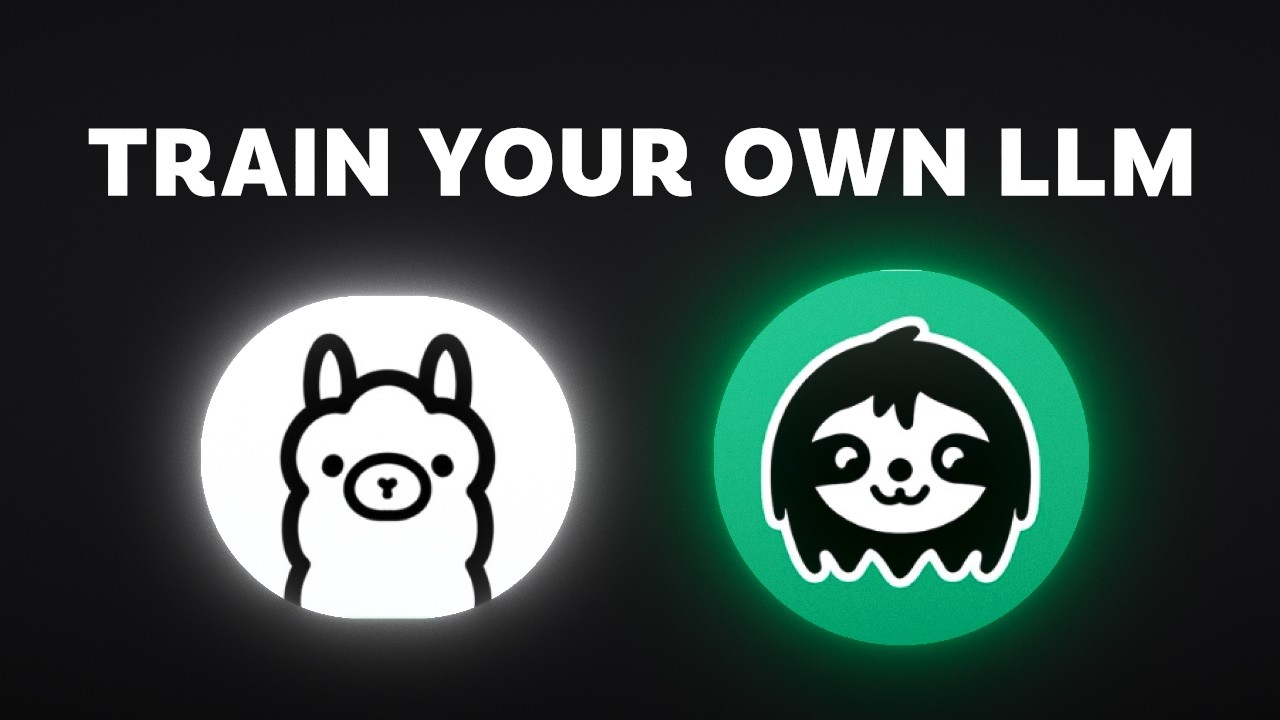
EASIEST Way to Fine-Tune a LLM and Use It With Ollama
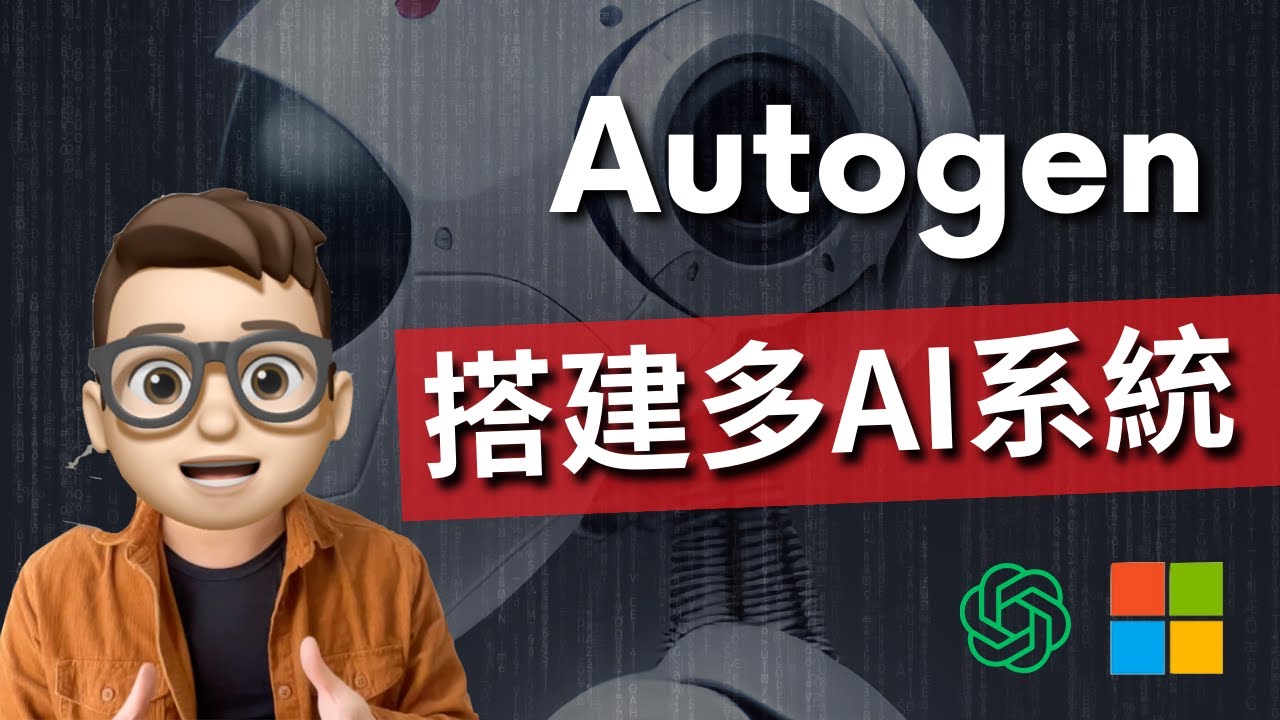
手把手教大家搭建Autogen|微軟的多智能體協作系統|多AI協調|#multi-agent #autogen #openai #chatpgt #gpt4 #gpt #web3
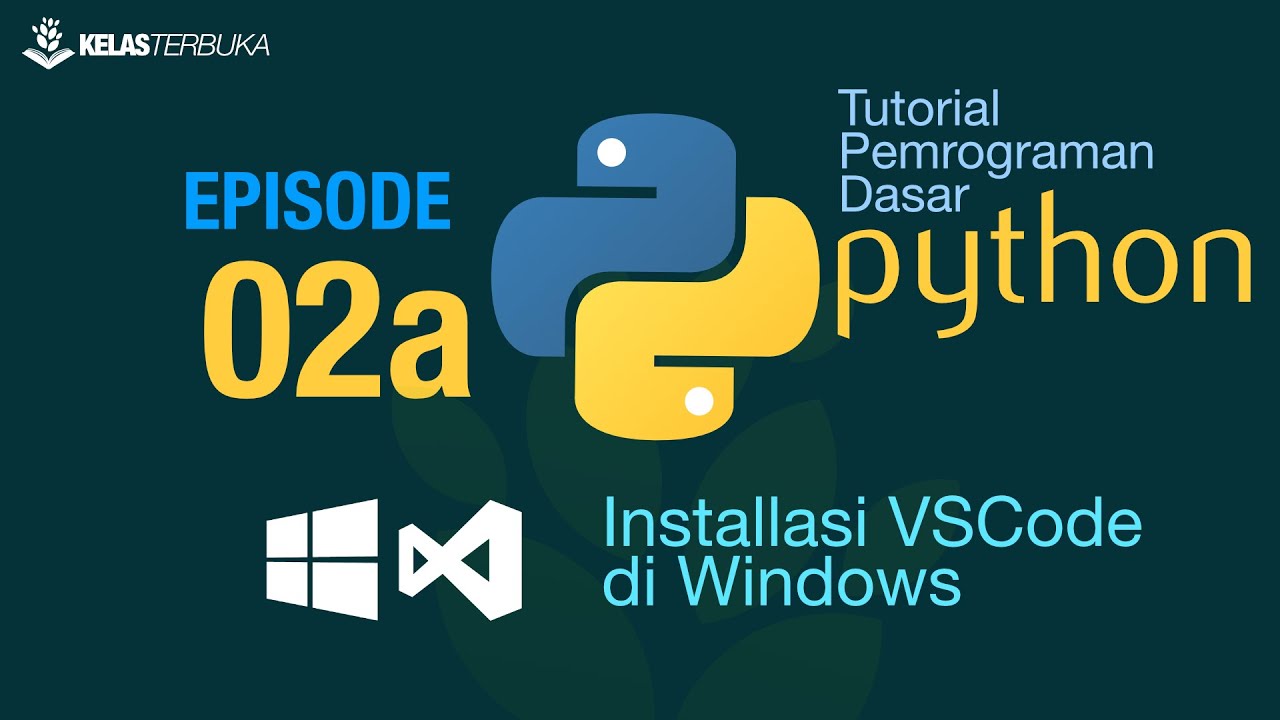
Belajar Python [Dasar] - 02a - Installasi Python dan VS Code di Windows
5.0 / 5 (0 votes)