Types of Data in Statistics - Nominal, Ordinal, Interval, and Ratio
Summary
TLDRThis video explains the four levels of measurement in statistics: nominal, ordinal, interval, and ratio. It emphasizes their importance in data collection, analysis, and interpretation. Nominal data categorizes without order, ordinal data allows ranking but with uneven intervals, and interval data has equal intervals but lacks a true zero. Ratio data has both equal intervals and a true zero, enabling meaningful ratios. The video provides real-world examples for each level, showcasing their relevance in surveys, statistical tests, and visualizations, and highlights the importance of identifying the correct measurement level from the start for accurate analysis.
Takeaways
- 😀 Levels of measurement in statistics refer to how data can be organized, compared, and analyzed.
- 😀 The four primary levels of measurement are Nominal, Ordinal, Interval, and Ratio.
- 😀 Nominal data can only be categorized and not ranked; examples include gender and types of animals.
- 😀 Ordinal data can be ranked, but the differences between the ranks are not quantifiable or meaningful.
- 😀 Interval data has equal intervals between values, but lacks a true zero point, e.g., temperature in Celsius.
- 😀 Ratio data has equal intervals and a true zero point, making it suitable for all mathematical operations.
- 😀 Understanding the level of measurement is crucial for determining the type of statistical analysis and visualization to use.
- 😀 Nominal data can be visualized using frequency tables or bar charts, whereas interval and ratio data are better suited for histograms.
- 😀 The metric level of measurement can be subdivided into Interval and Ratio scales, with Ratio having a true zero point.
- 😀 Ratio data allows for meaningful multiplication and division (e.g., income, weight), while Interval data only supports addition and subtraction.
- 😀 Different statistical analyses are appropriate for different levels of measurement, such as calculating the mean for metric data but not for nominal data.
Q & A
What are the four levels of measurement in statistics?
-The four levels of measurement in statistics are nominal, ordinal, interval, and ratio. Each level categorizes data in different ways, affecting how the data can be analyzed.
How does the nominal level of measurement differ from the ordinal level?
-Nominal data can only be categorized, with no inherent order or ranking, while ordinal data can be ranked, but the intervals between ranks are not necessarily equal or meaningful.
What kind of data can be used to calculate the mean and standard deviation?
-Only interval and ratio data can be used to calculate the mean and standard deviation, as these levels involve equally spaced intervals between values.
Can you give an example of nominal data and explain how it's used?
-An example of nominal data is gender, with categories such as male and female. Nominal data is used for categorization and counting but does not allow for ranking or meaningful comparison between categories.
What is the primary difference between interval and ratio data?
-The key difference between interval and ratio data is the presence of a true zero point. Ratio data has a true zero point (e.g., weight or age), which allows for meaningful ratios, while interval data lacks a true zero (e.g., temperature in Celsius).
Why is it important to consider the level of measurement at the beginning of the research process?
-Considering the level of measurement from the start is crucial because it helps determine the appropriate statistical tests, data collection methods, and visualization techniques that can be applied to the data.
How would you visualize nominal data?
-Nominal data is typically visualized using frequency tables or bar charts, as it involves categorizing data into distinct, non-ordered groups.
Can you perform mathematical operations like addition or subtraction on ordinal data?
-No, ordinal data allows for ranking but does not provide equal intervals between ranks, meaning mathematical operations like addition or subtraction do not produce meaningful results.
How would you collect and analyze ordinal data in a survey?
-To collect ordinal data in a survey, you might ask respondents to rate something on a scale (e.g., very unsatisfied to very satisfied). The responses can be ranked, but the exact differences between levels cannot be quantified.
What is an example of ratio data, and how does it differ from interval data in terms of analysis?
-An example of ratio data is weight, where zero represents the complete absence of the measured quantity. Ratio data allows for meaningful comparisons like 'twice as much' or 'half as much,' unlike interval data, where such comparisons aren't possible due to the lack of a true zero point.
Outlines
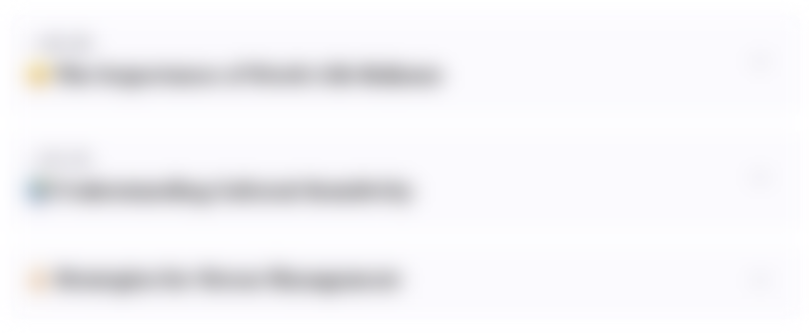
Esta sección está disponible solo para usuarios con suscripción. Por favor, mejora tu plan para acceder a esta parte.
Mejorar ahoraMindmap
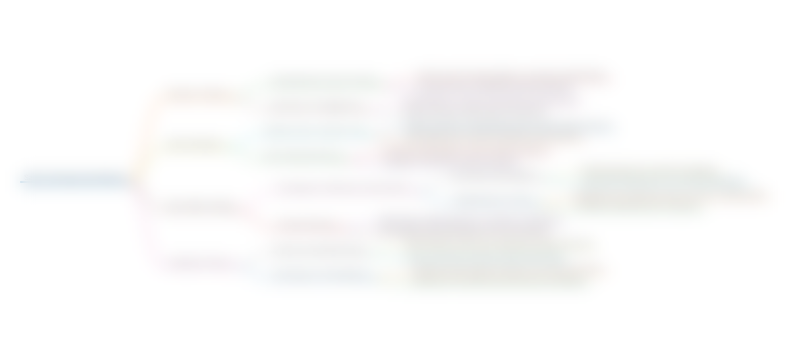
Esta sección está disponible solo para usuarios con suscripción. Por favor, mejora tu plan para acceder a esta parte.
Mejorar ahoraKeywords
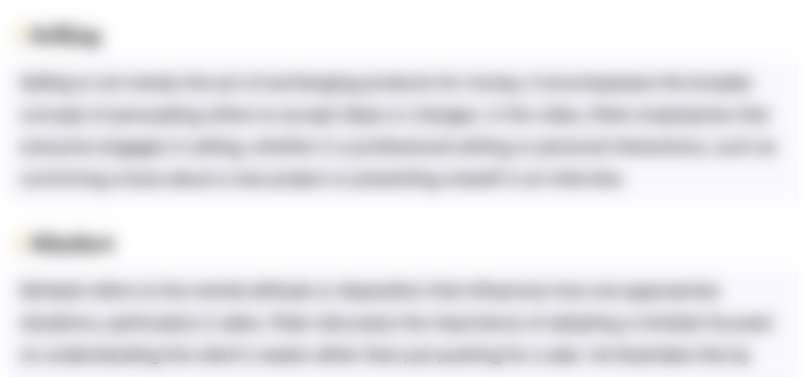
Esta sección está disponible solo para usuarios con suscripción. Por favor, mejora tu plan para acceder a esta parte.
Mejorar ahoraHighlights
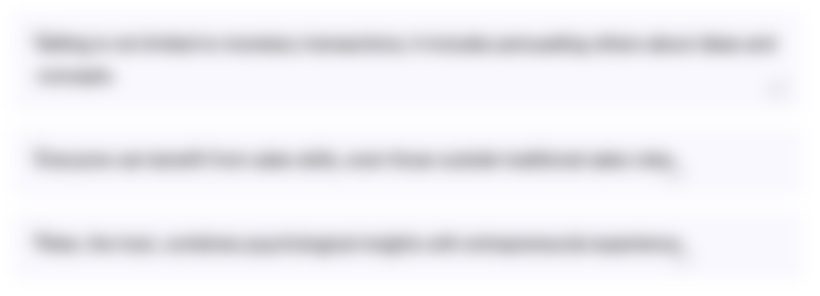
Esta sección está disponible solo para usuarios con suscripción. Por favor, mejora tu plan para acceder a esta parte.
Mejorar ahoraTranscripts
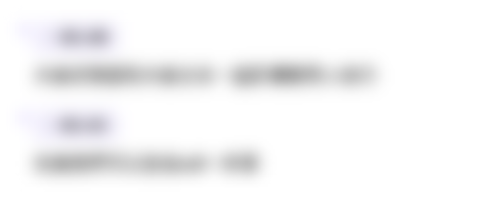
Esta sección está disponible solo para usuarios con suscripción. Por favor, mejora tu plan para acceder a esta parte.
Mejorar ahoraVer Más Videos Relacionados
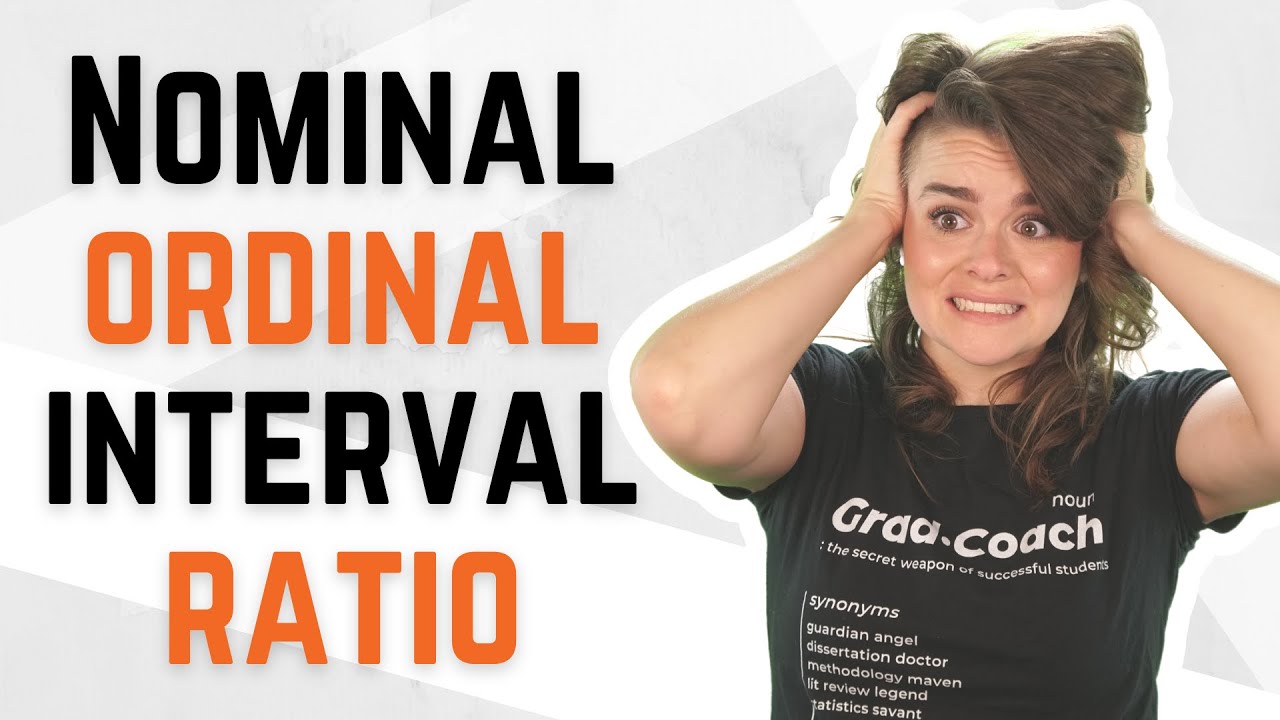
Nominal, Ordinal, Interval & Ratio Data: Simple Explanation With Examples
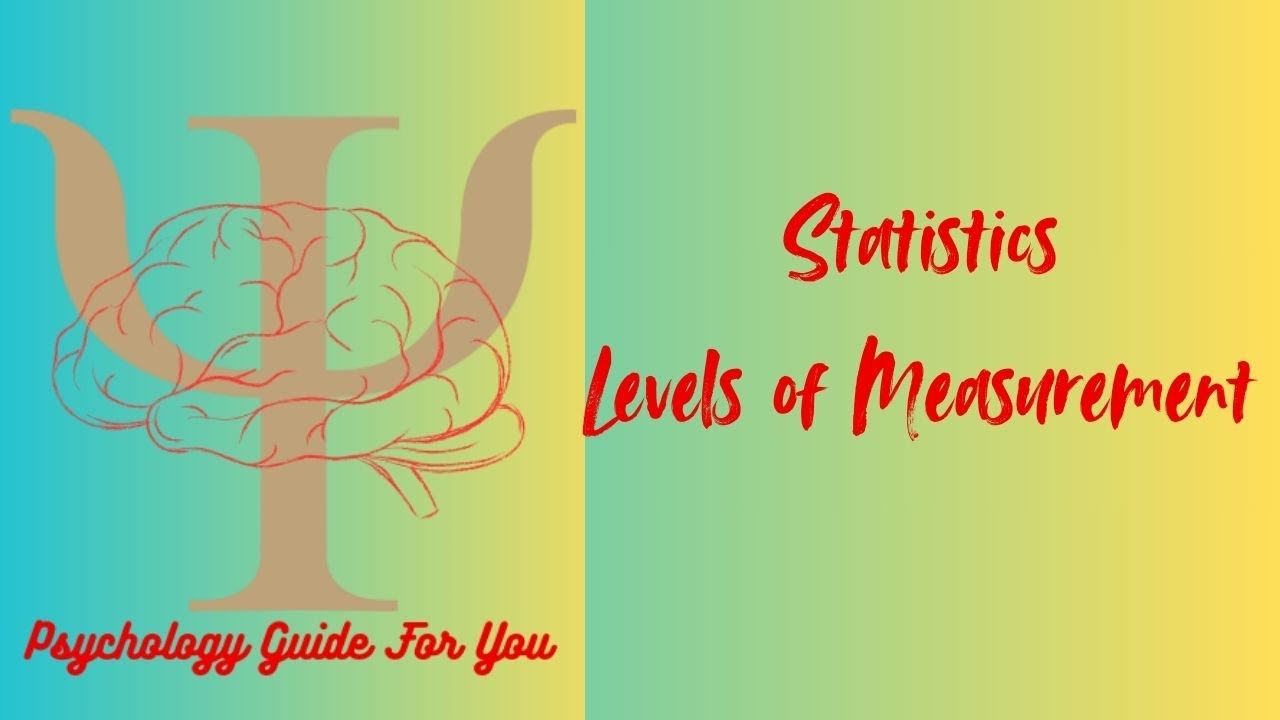
Levels of Measurement in Statistics: Nominal, Ordinal, Interval and Ratio
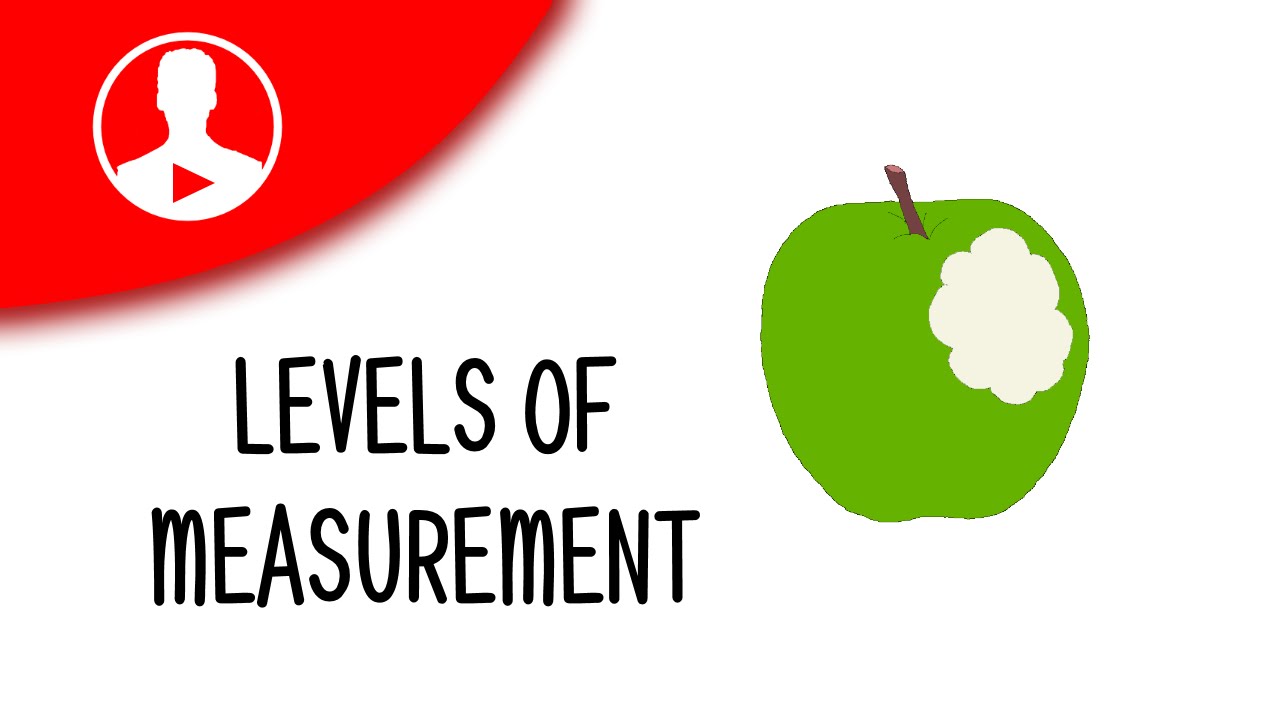
Scales of Measurement: Nominal, Ordinal, Interval and Ratio Scale
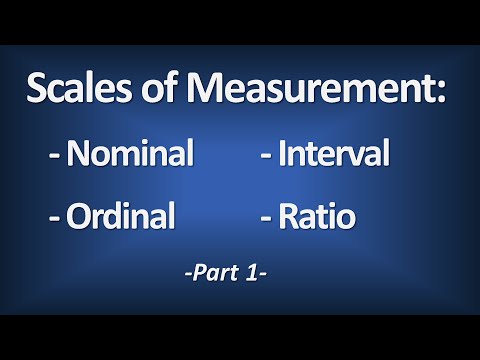
Scales of Measurement - Nominal, Ordinal, Interval, Ratio (Part 1) - Introductory Statistics
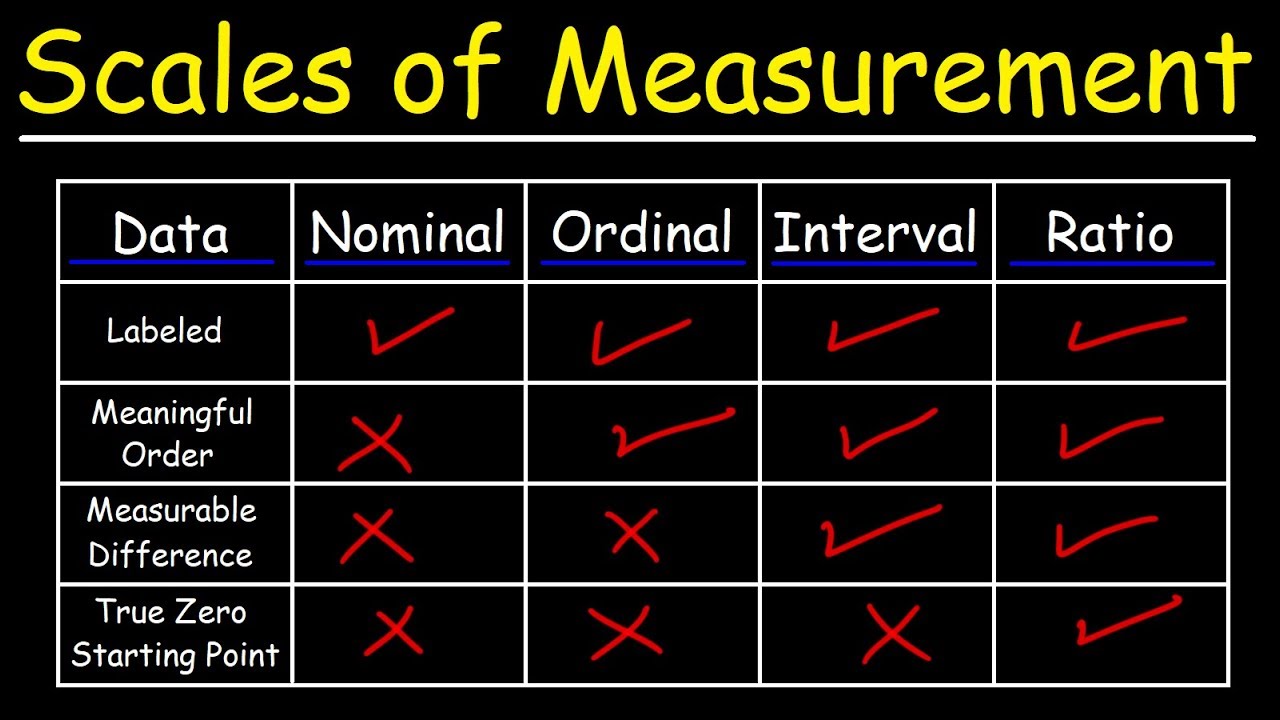
Scales of Measurement - Nominal, Ordinal, Interval, & Ratio Scale Data
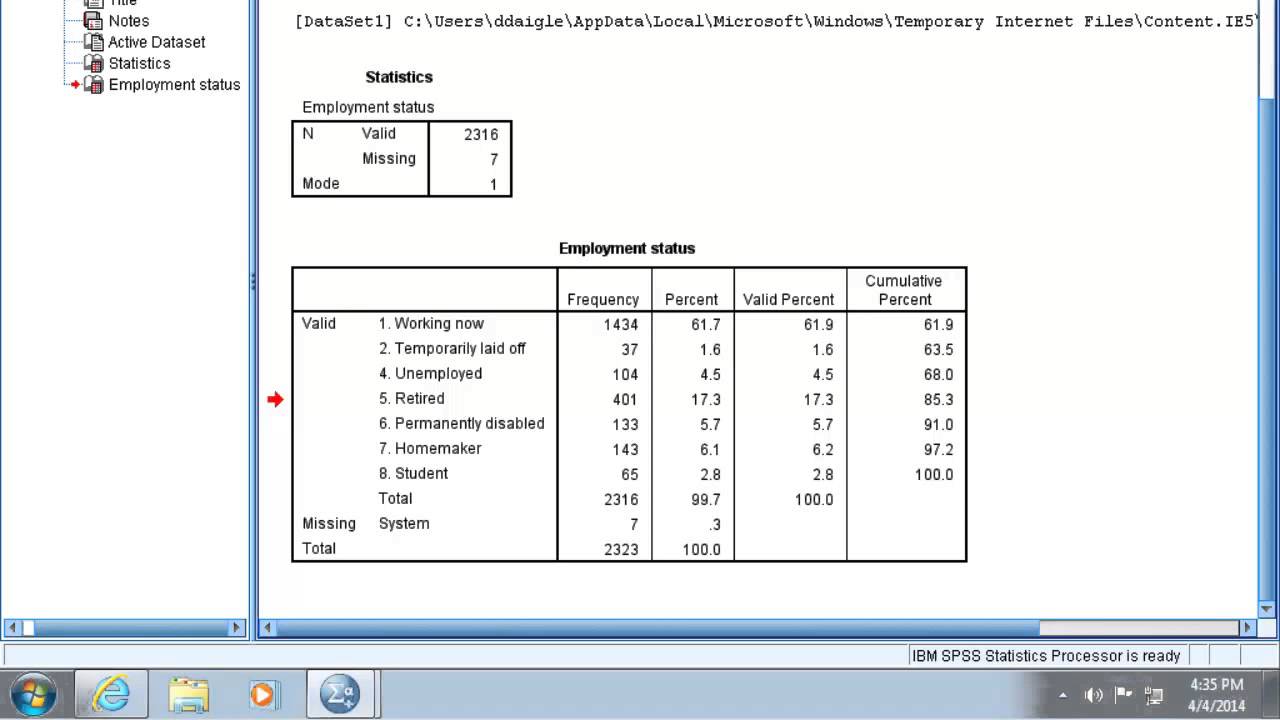
Introduction to Univariate Analysis
5.0 / 5 (0 votes)