Types of Research Designs – Correlational Studies
Summary
TLDRThis video script delves into correlational studies, a prevalent research design in psychology. It explains how these studies explore the relationship between two variables, such as marital satisfaction and parenting quality, or creativity and academic performance. The script clarifies the importance of interpreting correlation coefficients, emphasizing the magnitude and valence of correlations. It visually illustrates positive and negative correlations through scatterplots and cautions against the common mistake of assuming causation from correlation, a fallacy evident in sensational headlines. The script concludes by stressing the utility of correlations while advising skepticism and a search for alternative explanations.
Takeaways
- 🔍 Correlation studies are widely used in psychology to examine the relationship between two variables.
- 🤔 Research questions suitable for correlational research include those examining the link between marital satisfaction and parenting quality, or creativity and academic performance.
- 🧑🔬 Correlational studies often involve many participants and can be conducted through surveys or in a lab setting.
- 📊 The outcome of a correlational study is a correlation coefficient, which is quantified using Pearson's r-value.
- 📉 The magnitude of the correlation coefficient indicates the strength of the relationship, with values closer to 1 or -1 indicating stronger relationships.
- ➡️ Positive correlations mean that as one variable increases, the other tends to increase as well.
- ⬅️ Negative correlations indicate that as one variable increases, the other tends to decrease.
- 🔄 Zero correlation implies no relationship between the variables, often appearing as a random scatter of data points.
- 🚫 Correlation does not imply causation; seeing a correlation between two variables does not mean one causes the other.
- ❗️ Misinterpreting correlations as causation is known as the causation fallacy and is a common mistake in interpreting research findings.
- 📈 The next video will discuss experimental research designs, which allow for making causal inferences about the world.
Q & A
What is a correlational study in research?
-A correlational study is a type of research design used to examine the extent to which two variables are correlated with each other, meaning there is an association or relationship between them.
Why might a developmental psychologist be interested in a correlational study?
-A developmental psychologist might be interested in a correlational study to explore relationships between variables such as marital satisfaction and parenting quality or ability.
What are some real-world applications of correlational studies?
-Real-world applications of correlational studies include conducting surveys online to find relationships between variables like creativity and academic performance.
How does a correlational study typically differ from a case study in terms of participants?
-A correlational study typically involves a large number of participants, unlike a case study which focuses on an in-depth examination of a single individual or a small group.
What is the end result of a correlational study?
-The end result of a correlational study is a correlation, which is a measure of the strength and direction of a relationship between two variables.
Who invented the Pearson correlation coefficient?
-The Pearson correlation coefficient was invented by Karl Pearson.
What are the two important aspects to consider when interpreting a correlation coefficient?
-The two important aspects to consider when interpreting a correlation coefficient are the magnitude, which describes the strength of the relationship, and the valence, which indicates the direction or nature of the relationship.
What does a correlation close to zero indicate?
-A correlation close to zero indicates a weak relationship between the two variables, suggesting there is little to no association.
How is a positive correlation represented graphically?
-A positive correlation is represented graphically on a scatterplot by a line that goes from the bottom left to the top right, indicating that as one variable increases, the other also tends to increase.
What does a negative correlation imply?
-A negative correlation implies that as one variable increases, the other variable tends to decrease, indicating an inverse relationship between the two variables.
Why is it incorrect to assume causation from a correlation?
-It is incorrect to assume causation from a correlation because correlation only indicates a relationship between two variables, not that one variable causes the other to change. This mistake is known as the causation fallacy.
What is the difference between a positive, negative, and zero correlation?
-A positive correlation indicates that both variables increase or decrease together, a negative correlation indicates that as one variable increases, the other decreases, and a zero correlation indicates no discernible relationship between the variables.
Outlines
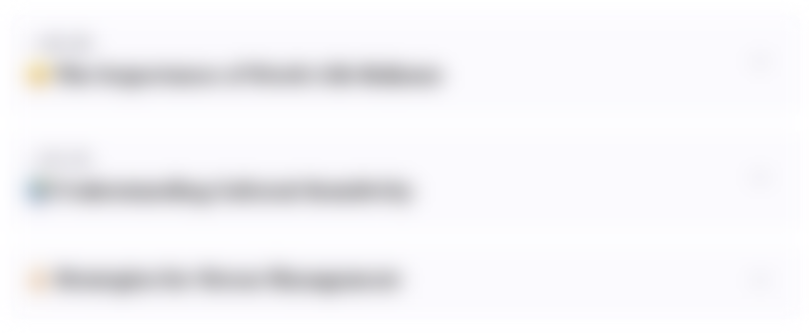
Esta sección está disponible solo para usuarios con suscripción. Por favor, mejora tu plan para acceder a esta parte.
Mejorar ahoraMindmap
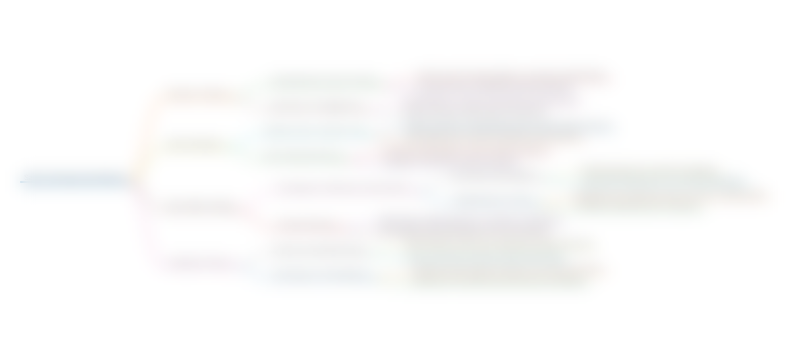
Esta sección está disponible solo para usuarios con suscripción. Por favor, mejora tu plan para acceder a esta parte.
Mejorar ahoraKeywords
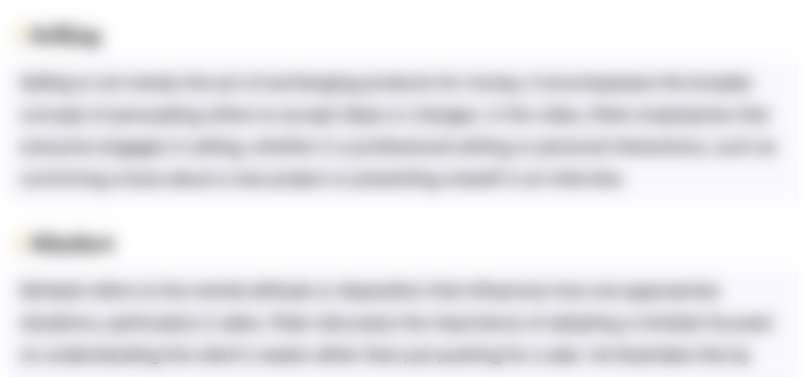
Esta sección está disponible solo para usuarios con suscripción. Por favor, mejora tu plan para acceder a esta parte.
Mejorar ahoraHighlights
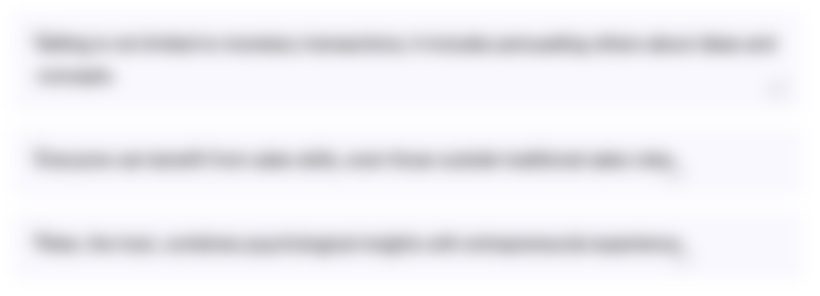
Esta sección está disponible solo para usuarios con suscripción. Por favor, mejora tu plan para acceder a esta parte.
Mejorar ahoraTranscripts
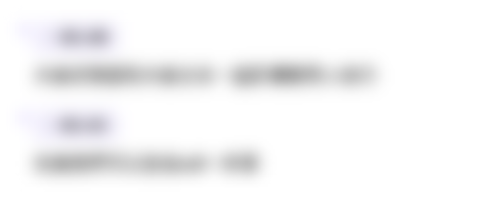
Esta sección está disponible solo para usuarios con suscripción. Por favor, mejora tu plan para acceder a esta parte.
Mejorar ahoraVer Más Videos Relacionados
5.0 / 5 (0 votes)