Intro to Databricks Lakehouse Platform Architecture and Security
Summary
TLDREl script del video explica la importancia de la fiabilidad y el rendimiento de los datos en la arquitectura de plataformas, destacando Delta Lake y Photon como tecnologías fundamentales en la plataforma Lakehouse de Databricks. Delta Lake, un formato de almacenamiento de código abierto basado en archivos, garantiza transacciones ACID, manejo escalable de datos y metadatos, y evolución del esquema. Photon es el motor de consultas de nueva generación que ofrece ahorros en costos de infraestructura y mejora el rendimiento. El script también cubre la gobernanza unificada y la seguridad, con Unity Catalog y Delta Sharing, y presenta el concepto de computación sin servidor y sus beneficios en el Lakehouse de Databricks.
Takeaways
- 📈 El script destaca la importancia de la fiabilidad y el rendimiento de los datos en la arquitectura de la plataforma.
- 💧 Se menciona que los Data Lakes a menudo se llaman Data Swamps debido a su falta de características para la fiabilidad y calidad de datos.
- 🔄 Delta Lake es un formato de almacenamiento basado en archivos y de código abierto que proporciona garantías para transacciones ACID y manejo escalable de datos y metadatos.
- 🛠️ Photon es el nuevo motor de consultas que ofrece ahorros significativos en costos de infraestructura y mejora el rendimiento de las consultas en el Data Lake.
- 🔒 La plataforma Lakehouse de Databricks ofrece una estructura de gobernanza y seguridad unificada, lo que es crucial para proteger los datos y la marca de una empresa.
- 🌐 Unity Catalog es una solución de gobernanza unificada para todos los activos de datos, proporcionando control de acceso fino y auditoría de consultas SQL.
- 🔗 Delta Sharing es una herramienta de código abierto para compartir datos en vivo de manera segura y eficiente entre plataformas.
- 🛡️ La arquitectura de la plataforma se divide en dos planos: el plano de control y el plano de datos, lo que simplifica los permisos y reduce el riesgo.
- 🚀 El servidor de computación sin servidor de Databricks ofrece una solución completamente administrada que reduce los costos y aumenta la productividad de los usuarios.
- 🔑 Databricks ofrece varias formas de habilitar el acceso de los usuarios a sus datos, incluyendo ACLs de tabla, perfiles de instancia de AWS y el API de secretos.
Q & A
¿Qué problemas pueden enfrentar los ingenieros de datos al utilizar un lago de datos estándar?
-Los ingenieros de datos pueden enfrentar problemas como la falta de soporte para transacciones ACID, lo que impide mezclar actualizaciones y lecturas; la falta de aplicación de esquema, lo que resulta en datos inconsistentes y de baja calidad; y la falta de integración con el catálogo de datos, lo que lleva a datos oscuros y la ausencia de una única fuente de verdad.
¿Cómo Delta Lake mejora la fiabilidad y el rendimiento en la plataforma Lakehouse de Databricks?
-Delta Lake mejora la fiabilidad y el rendimiento proporcionando garantías para transacciones ACID, manejo escalable de datos y metadatos, historial de auditoría y viaje en el tiempo, aplicación de esquema y evolución del esquema, y soporte para eliminaciones, actualizaciones y fusiones.
¿Qué es Photon y cómo resuelve los desafíos de rendimiento en la plataforma Lakehouse de Databricks?
-Photon es el siguiente motor de consultas de generación que proporciona ahorros dramáticos en costos de infraestructura y es compatible con las API de Spark, implementando un marco de ejecución más general para un procesamiento eficiente de datos. Proporciona velocidades incrementadas para casos de uso como la ingesta de datos, ETL, transmisión de datos, ciencia de datos interactiva y consultas interactivas directamente en el lago de datos.
¿Qué beneficios ofrece la compatibilidad de Delta Lake con Apache Spark y otros motores de procesamiento?
-La compatibilidad de Delta Lake con Apache Spark y otros motores de procesamiento permite que los equipos de datos trabajen con una variedad de latencias de datos, desde la ingesta de datos en streaming hasta la retroalimentación histórica por lotes e consultas interactivas, todo desde el principio.
¿Cómo ayuda Unity Catalog a abordar los desafíos de gobernanza y seguridad en la plataforma Lakehouse de Databricks?
-Unity Catalog ofrece una solución de gobernanza unificada para todos los activos de datos, con control de acceso fino a nivel de fila, columna y vista, auditoría de consultas de SQL, control de acceso basado en atributos, control de versiones de datos y restricciones de calidad de datos, y monitoreo.
¿Qué es Delta Sharing y cómo ayuda a compartir datos de manera segura y eficiente?
-Delta Sharing es una herramienta abierta y entre plataformas para compartir datos en vivo de forma segura. Permite compartir datos en formatos Delta Lake y Apache Parquet sin tener que establecer nuevos procesos de ingesta y mantiene la administración y gobernanza de los datos por parte del proveedor de datos, con la capacidad de hacer un seguimiento y auditar el uso.
¿Cómo se divide la arquitectura de la plataforma Lakehouse de Databricks para mejorar la seguridad?
-La arquitectura se divide en dos planos separados: el plano de control y el plano de datos. El plano de control consiste en los servicios back-end administrados que Databricks proporciona y el plano de datos es donde se procesa los datos, asegurando que los datos se mantengan en la cuenta de la nube del propietario del negocio.
¿Qué ventajas ofrece el uso de Serverless Compute en la plataforma Lakehouse de Databricks?
-El Serverless Compute es un servicio completamente administrado que Databricks proporciona y maneja los recursos de cómputo para un negocio en la cuenta de nube de Databricks. Reduce el costo total de propiedad, elimina la sobrecarga administrativa y aumenta la productividad de los usuarios, con un inicio inmediato y un escalado en segundos.
¿Cuáles son algunas de las características clave de Unity Catalog que son importantes para entender cómo funciona la administración de datos en Databricks?
-Unity Catalog incluye elementos clave como el metastore, que es el contenedor lógico de nivel superior en Unity Catalog y representa los metadatos; el catálogo, que es el contenedor de nivel más alto para objetos de datos; y el esquema, que es un contenedor para activos de datos como tablas y vistas y forma parte del tercer nivel del espacio de nombres de tres niveles.
¿Qué son las vistas en el contexto de Unity Catalog y cómo se relacionan con las consultas SQL?
-Las vistas son consultas almacenadas que se ejecutan cuando se realiza una consulta en la vista. Realizan transformaciones SQL arbitrarias en tablas y otras vistas y son de solo lectura, lo que significa que no tienen la capacidad de modificar los datos subyacentes.
Outlines
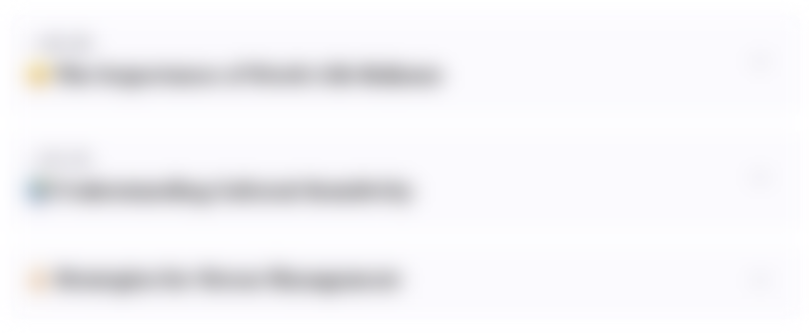
Esta sección está disponible solo para usuarios con suscripción. Por favor, mejora tu plan para acceder a esta parte.
Mejorar ahoraMindmap
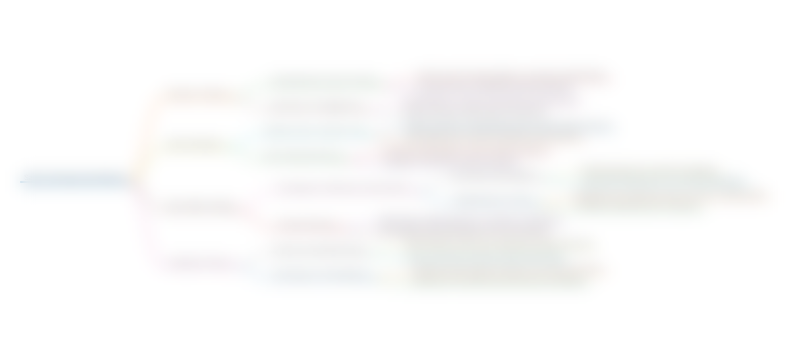
Esta sección está disponible solo para usuarios con suscripción. Por favor, mejora tu plan para acceder a esta parte.
Mejorar ahoraKeywords
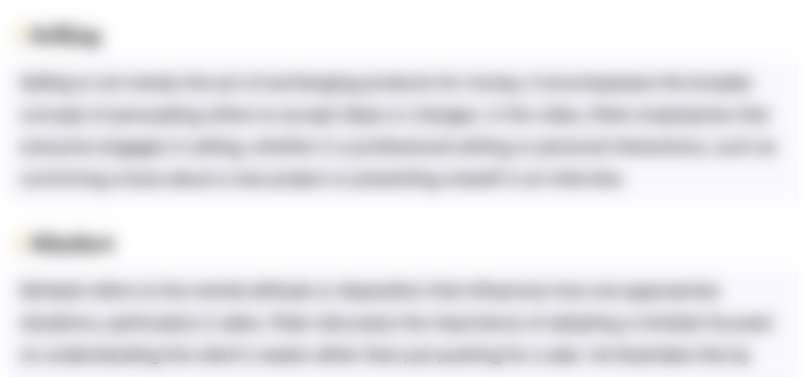
Esta sección está disponible solo para usuarios con suscripción. Por favor, mejora tu plan para acceder a esta parte.
Mejorar ahoraHighlights
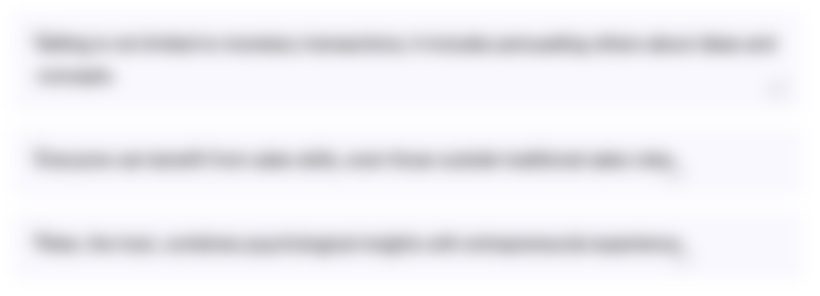
Esta sección está disponible solo para usuarios con suscripción. Por favor, mejora tu plan para acceder a esta parte.
Mejorar ahoraTranscripts
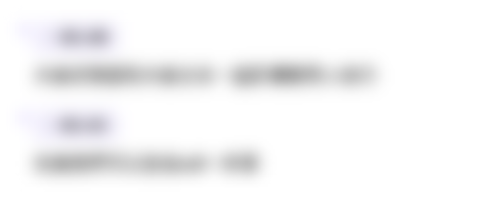
Esta sección está disponible solo para usuarios con suscripción. Por favor, mejora tu plan para acceder a esta parte.
Mejorar ahoraVer Más Videos Relacionados

Aplicación del TPM & RCM | Caso práctico | Gerencia de mantenimiento | Ingeniería industrial

TOGAF Arquitectura de sistemas de Información

Modelo de referencia TCP/IP | | UPV
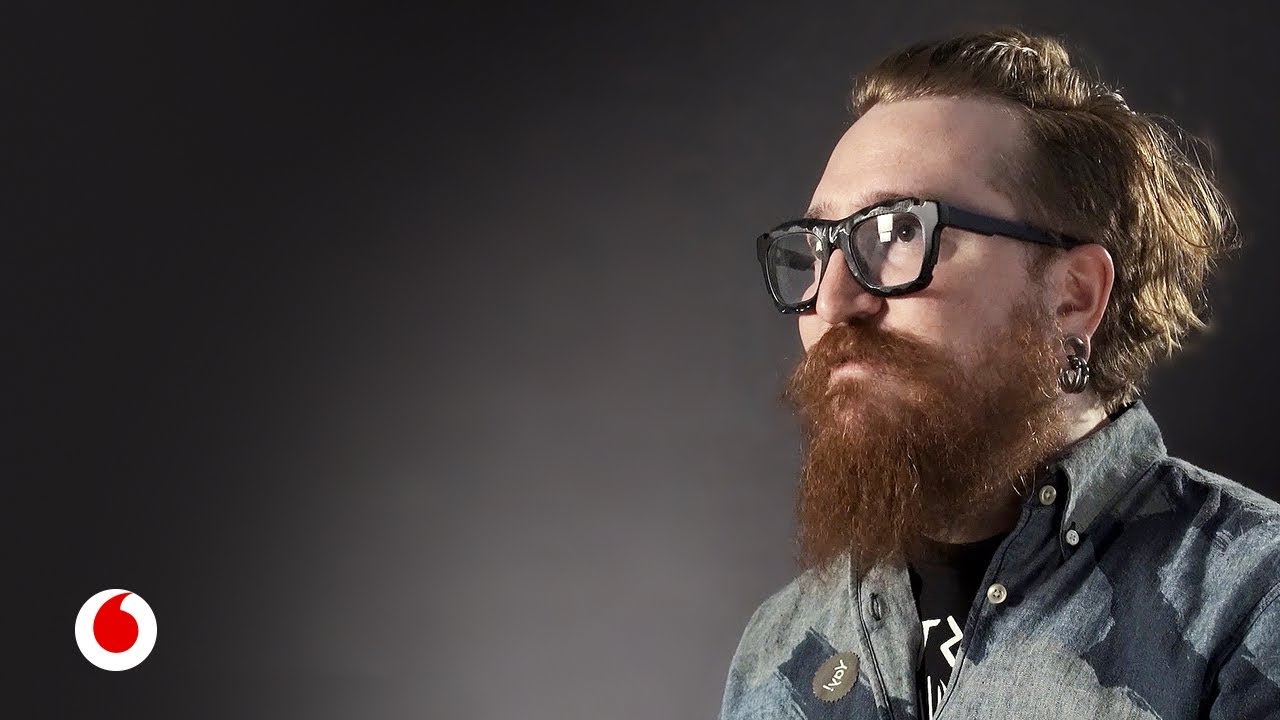
Harper Reed, el ingeniero que ayudó a ganar sus segundas elecciones a Obama

Arquitectura de Productos

LOCTITE HENKEL ADHESIVOS PEGAMENTOS Y SELLADORES www.baselgalizaga.es
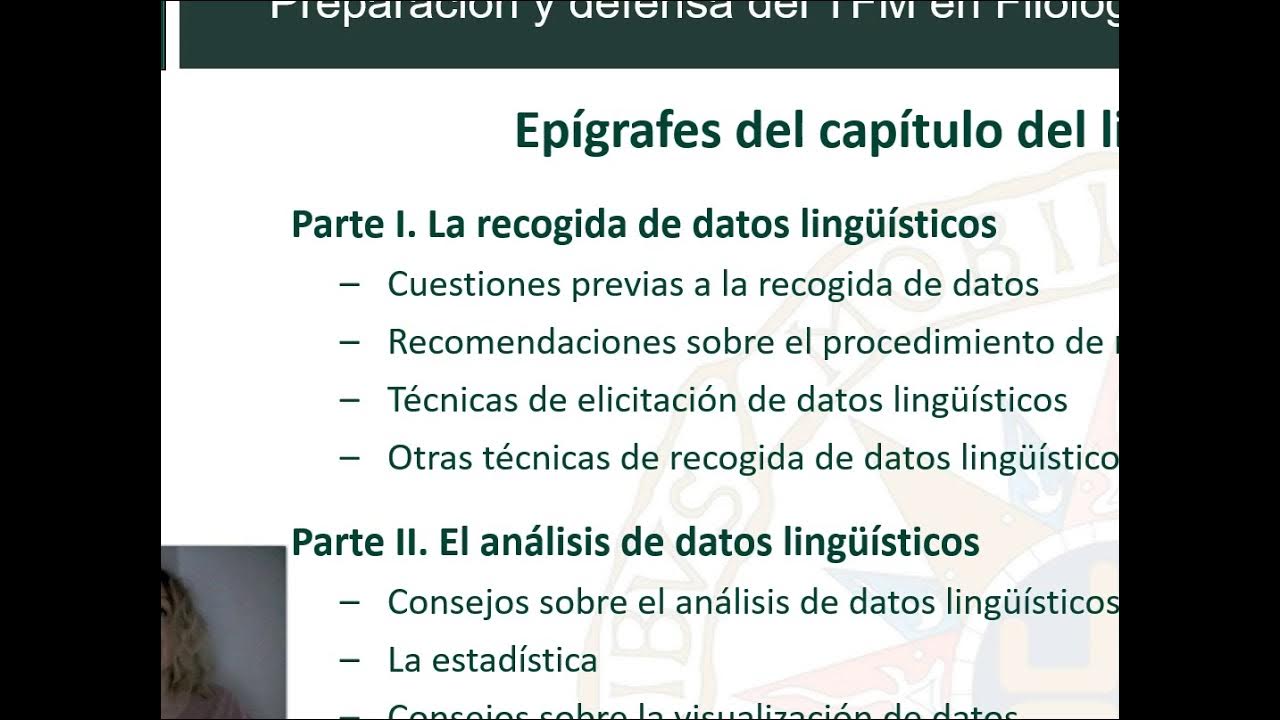
3.2. Recopilación y análisis de datos
5.0 / 5 (0 votes)