Ch 3 Lecture Video, Fall 2024: Measures of Central Tendency
Summary
TLDRThis educational video script delves into the significance of measures of central tendency in statistical analysis, focusing on mode, median, and mean. It explains how these measures help summarize data, revealing patterns and trends. The script clarifies the concept of range, the calculation of mode as the most frequent value, and the median as the middle value in a dataset. It also discusses calculating the mean, emphasizing its importance in various types of data, including interval, ratio, and ordinal. The script provides examples to illustrate these concepts, aiming to enhance understanding of statistical summaries.
Takeaways
- 📊 Measures of central tendency are crucial for summarizing and analyzing data, helping to understand the 'story' the data is telling.
- 🔢 The concept of 'multivariant' data is introduced, referring to datasets with two or more variables, emphasizing the complexity of modern data analysis.
- 📈 Understanding distributions is key, which involves recognizing how data is spread across different variables and values.
- 🎯 Central tendency focuses on identifying the 'middle point' and typical trends within a dataset, aiding in data summarization.
- 🏅 The mode is defined as the most frequently occurring value or category in a dataset, serving as an easy identifier of commonality.
- 🔢 The median is the middle value in a dataset when ordered from least to greatest, dividing the data into halves.
- 📐 The range is calculated as the difference between the highest and lowest values in a dataset, providing a sense of data spread.
- 📊 Percentiles are discussed as a way to understand the relative standing of data points within a dataset, differentiating them from percentages.
- 🧮 The mean, or average, is calculated using the formula \( \bar{Y} = \frac{\sum f \times y}{N} \), where \( f \) is frequency, \( y \) is the value, and \( N \) is the total number of cases.
- ⚖️ Different types of data (nominal, ordinal, interval, ratio) are considered in relation to how they can be analyzed using measures of central tendency, with a clarification that nominal data does not have a mean in the traditional sense.
Q & A
What are the measures of central tendency and why are they important?
-The measures of central tendency include mode, median, and mean. They are important because they help summarize data and provide a better understanding of the typical values or patterns within a dataset, allowing for easier analysis and interpretation.
What is the definition of 'distribution' in the context of statistics?
-In statistics, 'distribution' refers to how values are spread across categories and variables. It looks at the similarities and differences, essentially showing how data points are dispersed.
Can you explain the concept of 'range' in statistics?
-The 'range' in statistics is the difference between the highest and lowest values in a dataset, representing the spread of the data.
What is the mode and how is it determined?
-The mode is the value or category that occurs most frequently in a dataset. It is determined by identifying the data point with the highest frequency.
How is the median calculated for a dataset with an odd number of observations?
-For a dataset with an odd number of observations, the median is calculated by arranging the data in ascending order and selecting the middle value.
What is the formula used to calculate the mean?
-The formula to calculate the mean is the sum of all the values (ΣY) divided by the total number of observations (n), represented as Y Bar = ΣY / n.
How does the calculation of the median differ when the dataset has an even number of observations?
-When the dataset has an even number of observations, the median is the average of the two middle values after the data is arranged in ascending order.
What is the significance of assigning numerical values to non-numerical data?
-Assigning numerical values to non-numerical data allows for statistical analysis and interpretation, such as calculating means or modes, even when the data is ordinal or nominal.
Why can't nominal data be calculated with a mean in the traditional sense?
-Nominal data, which includes categories like gender or eye color, cannot be calculated with a mean in the traditional sense because they do not have a natural order or numerical value that can be averaged.
What is the difference between a percentage and a percentile?
-A percentage represents a proportion of a whole, while a percentile indicates the relative standing of a score within a dataset, showing the percentage of cases that fall below a particular value.
Outlines
📊 Introduction to Measures of Central Tendency
The paragraph introduces the concept of measures of central tendency, emphasizing its importance in statistical analysis. It mentions that understanding variables and frequency distribution tables is foundational, and now the focus shifts to central tendency to better comprehend data. The paragraph highlights the significance of summarizing data to grasp the underlying story it tells. It also touches on the relevance of multivariate data, explaining that it involves dealing with two or more variables. The measures of central tendency discussed include mode, median, and mean, each serving a distinct purpose but collectively aiding in understanding typical value distributions. The text also introduces the term 'distribution' and explains its role in analyzing how values are spread across categories and variables.
🔢 Exploring the Mode and Median
This paragraph delves into the specifics of the mode and median, two measures of central tendency. The mode is defined as the category or score with the highest frequency, using the example of Spanish being the mode among non-English languages in the United States due to the highest number of speakers. The concept of bimodal distribution is introduced, where two categories have the highest frequencies closely related but not identical. The median is then explained as the middle value of a distribution when data is ordered, with a detailed walkthrough of how to calculate it for both odd and even numbers of data points. The paragraph uses the example of hate crimes reported by states to illustrate the calculation of the median.
📈 Calculating the Mean and Understanding Percentiles
The paragraph discusses the mean, another measure of central tendency, and provides the formula for its calculation. It explains that the mean represents the average value and is crucial for interval and ratio variables. The text provides an example of calculating the mean for the ideal number of children based on survey responses, demonstrating how to use the formula and interpret the result. Additionally, the concept of percentiles is introduced, explaining how they indicate a score's relative standing within a range, differentiating them from percentages. The paragraph also addresses the calculation of medians in frequency distribution tables and the importance of organizing data to identify the mode.
📉 Assigning Numerical Values and Grouped Data
This paragraph addresses the process of assigning numerical values to non-numerical data for the calculation of the mean, such as in surveys using Likert scales. It explains how to calculate the mean by multiplying the frequency of each response by its assigned numerical value and then summing these products. The text provides an example involving political views and shows how to interpret the calculated mean in the context of the data. It also touches on the calculation of means for grouped data, such as hours worked, and the assignment of midpoint values to ranges. The paragraph concludes by clarifying that nominal data, which lacks order, does not have a mean calculated in the traditional sense but can be analyzed through percentage breakdowns.
🚫 Clarification on Nominal Data and Mean Calculation
The final paragraph clarifies that nominal data, such as gender or eye color, does not have a mean calculated in the same way as interval or ordinal data. It emphasizes that while numerical values can be assigned for statistical analysis, these do not represent a central point or average in the same sense. The text provides a clear distinction between calculating percentages, which is similar to means for categorical data, and calculating a mean for ordinal or interval data. It uses the example of calculating the average number of miles driven by individuals to illustrate a straightforward mean calculation. The paragraph reinforces the importance of understanding the nature of data when performing statistical analysis and calculating central tendencies.
Mindmap
Keywords
💡Central Tendency
💡Frequency Distribution Tables
💡Mode
💡Median
💡Mean
💡Range
💡Multivariate Data
💡Distribution
💡Percentiles
💡Nominal Data
Highlights
Measures of central tendency are crucial for statistical analysis and understanding data.
Understanding variables and frequency distribution tables is fundamental before delving into measures of central tendency.
Measures of central tendency help summarize data and reveal the story it tells.
Multivariate data involves two or more variables and is important for summarizing complex data sets.
Distribution refers to how data is spread across variables and values, showing similarities and differences.
Central tendency focuses on the average or typical patterns or trends within a data set.
Mode is the value or category with the highest frequency in a data set.
Bimodal distribution occurs when two values have the highest frequency.
Median is the middle value in a data set when arranged in order, dividing the data into halves.
Calculating the median involves finding the middle value or averaging the two middle values for even sets.
Mean is calculated by summing all values and dividing by the number of cases.
Mean can be influenced by assigning numerical values to categories in surveys or ratings.
Grouped data requires assigning a midpoint value to each group for mean calculation.
Nominal data, such as gender or eye color, cannot be calculated with a mean but can be presented in percentage breakdowns.
Ordinal data, like stress levels, can have a mean calculated to find a central point.
Understanding measures of central tendency is essential for analyzing and summarizing data effectively.
Transcripts
we're going to talk about the measures
of central tendency because um this is
really one of the the this is really
important part to um moving forward and
getting the work done and starting to
analyze things statistically um you know
we've been doing things in starting to
build our knowledge and understanding
right so far we understand what the
variables are we understand what
frequency distribution tables are we
have an understanding of these things
right so now what we're doing is we're
going to be working with the measures of
central tendency which helps us
understand our data a little bit
more now with this um understanding the
measures of central tendency is
important you know we can use it for our
visuals of course but really it's
important most important because it
allows us to summarize our data it
allows us to summarize our data and be
able to have a better understanding of
what's happening the story it's telling
the P picture that's it's painting right
and so from there um we're able to be
able to work with larger data sets and
more variables uh so the idea of
multivariate data is important because
we might need to summarize more we might
need to summarize things that have more
than just one variable in this case
multivariant means two or more variables
so multi being multiple and then the
variants right being variables so being
able to understand the measures of
central tendency and how that influences
our movement forward um is important
so in terms of the measures of central
tendency um there's a couple there's a
couple words that your book uses and
just kind of acts as if uh you should
understand what they are and one is um
distribution it's used it a lot we're
going to continue using that word and
with distribution it's essentially how
things are distributed right so how it's
spread how it's broken up uh across
variables and values so like it's it's
the similarities and differences so
we're looking at how are spread out how
values are spread out across categories
and
variables for the measures of central
tendency what we're looking at is we're
looking at basically the average or the
typical you know what's average or
typical about the distribution so
thinking about central tendency right
Central where is that kind of Middle
Point and tendency how do what is what
is the what does it tend to do right the
averages or typical um patterns or
trends that are happening with the data
it's essentially looking at categories
and scores and then being able to
describe these things what is typical
across these values so there's three
concepts that we're going to talk about
mode median and mean each of these serve
a different purpose um but they still
highlight typical distributions of
values so they all have a purpose a
different purpose but they still allow
us to see the measures of central
tendency the the distribution of values
within a
category so in chapter 4 on page 100
um it talks about range that's a little
bit of a head a jump ahead but at the
same time um at the same time uh it's
important I think that we talk briefly
about what range is so the range is
basically just that it's the range of
values um what that means is that it's
what is the distance between your lowest
and highest value so if you had like
points for instance 4 6 19 32 79 those
were points your range would be 75
because 79 - 4 right 79 is your highest
value four is your lowest value you'll
also see these little curly brackets and
those are called Curly brackets and they
basically mean discrete values and
that's where you'll see your range the
discrete value is basically um are
values that cannot be subdivided right
so go back to continuous and discreet um
from a couple weeks ago and that's what
we're looking at with our range and the
reason why I bring it range now is
because how can we understand you know
what our our or what the data does what
it tends to do where the center point is
if we don't understand kind of what the
range is our code word is Apple so the
next thing that we're going to talk
about is the mode um the mode is a is
the category or the score with the
largest frequency this does not mean
it's
it's if you had like a rating scale it
does not mean that 10 is your mode
simply because it's the highest number
it's the value or the category that has
the highest number of things that are
occurring it's the easiest to identify
so it's it's essentially the answer or
the selection that somebody chose or
that the most amount of people chose um
you find the category and you find the
highest frequency it's really the
easiest to identify it's the thing that
occurs the most amount of times
um it's the category or the score not
the frequency itself so if it occurred
17 times you're not saying the mode is
17 you're saying the mode is whatever
category or score or value is is there
um if you have something that has more
than you have like two categories that
have the same um are the the highest
though that's called was called bodal so
think about bu being to modal for the
modes um if they're also close but not
exact so like let's say 75 and then 74.8
that is essentially still bodal and you
would report those two highest high you
would still report the two highest
categories or scores um
now the next slide we're going to see
from your book on page it should be on
page 64 uh an example of how mode can
look so in this case they listed as
foreign languages but I'm referring to
it as languages outside of the outside
of languages in the United States out
that are not English and so this is like
the number of speakers on the right and
the language on the left and this is the
number of speakers in those
corresponding
languages in this case Spanish is the
mode now it may not be organized this
way though and it's important that you
understand and identify how you will be
able to organize your data as well
because you're going to have to organize
it it's best to organize it to be able
to see what the mode is in this case
it's easy to identify right we know what
the mode is the mode here then is
Spanish Spanish is the mode because it's
has the highest number of speakers code
word is
pumpkin now the median is different so
the mode is relatively simple because
you can identify it remember it's not
the frequency so in this case it's not
37 million that's not the mode the mode
is Spanish in this case the median is
different because you're going to want
to put things in or in order there is
some sort of logical order not
everything will fit into here but you
can still find the median in the vast
majority of things um but it's the exact
middle of the distribution or the spread
of numbers so in this case it divides it
in half half above and half below um so
you have to sort your data you have to
sort it from you know highest to lowest
or lowest to highest if if it has the
even number of cases you divide it in
half and you do the calculation based
off those if it has an odd number of
cases you use this Formula n+ 1 / 2
where you divide it in half and that is
your is your value and I'm going to show
you on the next couple of slides so it
makes a little more sense so essentially
though the median is where you have your
data and it's half above half below um
and the median is literally that middle
point of the data so in your book this
is the table that it presents to you um
if you look look at it right the number
of hate crimes on the left uh is not in
order it is not in any order it's just
randomly um randomly placed there now if
you look on the right it has the state
and its corresponding state with the
number of hate crimes that occur in it
so we see that there are nine cases
right so we have 1 2 3 4 5 6 7 8 nine so
we have nine cases nine states in this
case then that have reported the number
of hate
crimes now we've reorganized it now
we've ordered it so if you look right
there's no organization now we've
ordered it from fewest to greatest from
least to greatest in terms of amount so
now we can see that this is in some sort
of order still nine cases though now if
you look at this here on the left hand
side this is nine cases so because it's
it's odd we have to use this little
formula so we have nine cases right here
there's nine cases um plus one because
we need to be able to figure out the
middle divided by two so in this case we
get 10 divided two which is five so what
it means is that we need to look at the
fifth case so 1 2 3 4 5 which gives us
Texas right here and that means that 145
is our median that means that that one
Texas here gives us our meeting of 145
so that means half the number of cases
will be fewer than 145 and half the
number of cases will be more than 145 so
that's what the median means is the
middle point now this is for our odd
number of cases but what happens if we
have an even number of cases so we know
that our median is 145 here
let's according to like your textbook
did this so let's say for a minute
California didn't report um we have
eight so we still have this order we've
taken California off so we're pretending
it doesn't exist right now and we have
eight number of cases 1 2 3 4 5 6 7 8
right so what happens is you look at the
two middle so 1 2 3 4 hm 1 2 3 4 H so
that gives us North Carolina and Texas
well what you have to do is you have to
add these two together right here and
divide it by two so gives you your
median and your median is
142.5 so the median basically means that
there are going to be half the amount of
cases that are above 142.5 and half that
are below and this is if you have an
even number of cases you find the two
middle ones you add them together and
divide them into two to get you your
median
number and I'm going to end this video
here and I'm going to create another
video so that way you have them divided
into two
head okay so we're going to go ahead and
um leave off now from where the last
video was and the last video we were
talking about median now you have your
frequency distribution tables and um and
those are useful when we're coming to
when we're trying to figure out our
median right because you've already kind
of organized all the data but what
happens is when you are creating this um
sometimes you have certain categories
that are associated with it so we kind
of already talked about that a little
bit uh and essentially when there's some
sort of category though that isn't
numerical in
value um or is like a different category
in terms of
um what you're trying to measure so on
page 70 it talks about political views
um it says that the the mean uh or not
the mean sorry the the the number um
comes to
21.5 what it's saying is that value is
associated with the label of moderate
and political views and that's what
you're going to go to um so in a couple
slides we'll talk about a little I'll
talk about it a little bit more um in
more detail but that's just something to
think about when it comes to
calculations of um of median for these
categories another thing about this is
um percentiles so you've probably
encountered and experience percentiles a
lot of standardized testing has
percentiles um and with these
percentiles it gives you an idea so a
percentile is not the same as a
percentage it is a general location
within the a range it's a score at or
below a specific range so if you scored
in the 70th 75th percentile it means
that 75% of cases are below it
um and that's a typo so I apologize but
75% of the cases are below it so so that
means that 25% of cases are above that
and it doesn't mean you scored a 75% you
scored could have scored a 20% but you
still are 75 uh in the 75th percentile
or you could have scored 100% but you're
still in the 7 maybe the 75th percentile
because there was extra credit stuff um
so these are things to consider when
we're talking about the calculations of
the medians we'll talk about percentiles
in more detail um at a later point so
now with that um is our mean now and
this calculation this formula here is
super important when I refer to these
things this y here stands for Y Bar this
e is Sigma which means sum the Y so the
combination of these two is the sum of
all your scores your variable scores um
Y Bar means your mean and this n is the
total number of cases and this is the
formula that you use to calculate mean
um there's a couple different ways that
you're going to calculate data values
it's mostly for Interval ratio VAR
variables but it's anything that has a
data value to it and we're going to talk
about this in more you might also hear
it referred to as um average okay so
we're going to talk about that in more
detail um on the next couple of sides to
see how it's applied depending on what
type of data we're using that data
informs um how we move forward with it
so um we're going to go to the next
slide
and oh too far okay so this is um on
page 73 it's in chapter 3 and this is
about the ideal number of children so
essentially this is a survey um
about this is a survey about
um the ideal number of children
and that means that
they were asked what do you believe is
the ideal number of children so the list
here is you know 0 1 2 3 4 5 6 with the
number of
children and frequency is the number of
responses that selected that option so
in this case these were the options zero
children one zero children one child two
children three children four five six
and this is the number of people who
selected these options so 13 people said
zero 16 people said 1 5006 said 2 Etc
which gives us our n at
868 so if you look over at this ex I'm
going to move
this so if you look over at this this
here is Sigma times you or S yeah
Sigma is the sum of your frequency times
your yvalue so your frequency times your
yvalue in this case is frequency times
your y-value the reason you're doing it
this way is because you're trying to
measure the average average number of
children all right that it seems to be
ideal so in this case you have zero you
have 16 you have 1,2 because what you're
doing is you're multiplying your y value
with your frequency so this is 506 * 2
is here so this gives you your n of 868
and then it gives you your your um your
sum of all your y's which is
2139 so what you do if you go back to
that formula is you have
2,100 sorry
2,139 um divided by 868 which comes to
2.46 and so oops so in this case right
that means the ideal number of children
is
2.46 uh we'll talk about how we can
report on this or the different ways
that we can report on this but
ultimately it's totally cool to save
2.46 children um and go and go from
there so what we do is um we're making
this calculation and you're trying to
figure out what what the answer is so in
this case again the ideal number of
children is um
2.46 all
right
now here's a couple things to think
about in terms of calculating mean in a
different in a variety of other ways um
so when we're looking at means this this
one's a little more straightforward in
some ways not this
ah all right so well okay so before this
was a little more straightforward in
terms of the calculations but sometimes
what happens is we have to actually
assign a numerical value to something so
when you're using a linear scale or a
lyer scale um generally these generally
you're you know if or let's say you have
like a value a number value you've
already assigned a numerical value to it
but sometimes you don't and so you'll
just have strongly agree agree neither
disagree Etc and you'll assign a
numerical value to it if you haven't
already now this is then if you have
your frequency this is the number of
times that somebody selected this
option then your frequency same thing
and this is what it comes to the way you
analyze this and you interpret it though
is your mean comes to
2.25 you don't have a 2.25 over here
that doesn't have any value or any
meaning to us right so what happens is
you identify which numerical value
closely aligns to the
2.25 which basically is this neither
agree or disagree so this means that
most people are um you know the average
comes to 2.25 which aligns with neither
agree or disagree so that's essentially
how you're identifying that why you're
how you're using the mean in these types
of linear likey scale
calculations
now there we go um okay so oops okay so
sometimes you're going to have grouped
um things too all right or frequency
ranges and so for you for a lot of you
you use things like hours works or
credits and so similarly like on the
left side hours work 0 1 through 5 6- 10
11 through 15 16 through 20 you assign a
numerical value to that as well because
again you're not calculating this per se
on its own you assign a numerical value
and the reason this is also a good
practice is because if you were to do a
quantitative reasoning class where you
have to do some additional statistical
analysis being able to understand how
you assign a numerical value to it is
important so then you create a frequency
your frequ you include your frequency so
in this case we have this it comes to 32
um and similar to before sorry for
having to move this similar to before
you have the same process right so it's
0 3 10 24
16 and then this comes 2 53 so darn it
um okay so in this
case your mean is 166 now this is kind
of Where It's tricky because it's kind
of arbitrary which means there's no real
rule for it so the way I would say is
like you figure okay well the numerical
value is 1.66 it's kind of divided here
well which really kind of leans more
towards 6.10 but it also means we're not
completely there so really you could say
that most students work within the range
of 1.5 per week but they probably work a
little bit more than that range because
that's essentially what it's telling you
right based off of this mean so that's
how you would calculate some sort of G
grouped frequencies or ranges that you
have
here now um when it so um I realized
that with the nominal data
um I was thinking about it in terms of
your individual questions and I had
worked with most of you and you had like
group frequencies and ranges you had um
a variety of other things and so I
wanted to kind of clarify a things that
I may have said so nominal data itself
can't be calculated with a mean some of
you may actually have Nom and I when I
say nominal data I don't necessarily
mean
um I don't necessarily mean it in terms
of like hours work and such I mean
nominal data specifically in terms of uh
like gender and eye color and even major
uh and so you're not going to have a
mean calculation in the same way you
will have the percent breako breakdown
which is calculated similar to the mean
um but it's not the mean in the typical
way um and the reason I say this is
that you will assign a value to them so
similar to like the previous um slides
and that gives you insight to be able to
run things
statistically but it's not a mean in the
sense that it shows you a point of the
average a use of something or average
water or average hours so if I said that
you would have a mean for everything the
vast majority of things will have a mean
some things will only have a mode if
it's only a category um so sometimes
your data you know if it's ordinal data
you will have it and for a lot of your
projects most of you have like interval
Ratio or ordinal um but thinking about
some of the major ones like major that
is nominal and that's not going it
doesn't have any order so you can
calculate these averages right um but
they don't have a statistical meaning in
the same way and so I just wanted to
make sure I clear that up if there was
any confusion about what I was saying um
which is why for some of you when I said
to have these uh I perhaps the better
way I should have said is like have it
set up to where you will have two rows
two columns um and you can calculate
those things in terms of that break so
some of your frequency distribution
tables will be enough for that but
essentially in this case you're um you
you can calculate percentages which is
essentially very similar um and that was
my confusion um based off of the number
of projects that I've been working on
closely with all of you um so I just
wanted to kind of clear that up um but
it is important when we're talking about
some of the two column ones that I was
asking you to do uh which will arise um
in terms of your projects so for
instance you're thinking let's say you
want to know you have been talking to
these five people Sam Charlie Sarah Max
and Joe and you want to know what the
average or mean number of miles driven
in a year between these people are so
you asked you ask them how many miles
have you driven in this past year and
they give you this variety of things so
Sam gives you
7,335 Charlie's
36295 Sarah is
21523 Max is 10,23 5 and Jo's 12
567 so in order to come up with your
mean it's going to be very similar to
where you calculate all of those numbers
um and you divide it by the number of
cases so in this case you add these all
up comes to 87,000 955 you divide it by
the total number of people which is five
um which means that your mean miles
driven is 17591 and that's it that's all
you have to do for this uh mean um or
average calculation so really the big
thing is to think about like because
there's no order to eye color there's no
order to um any of those things but if
you had like a category that was ordinal
in terms of like stress levels or
um a variety of those things then that's
where you would calculate the mean you
will calculate percentages which are
still kind of like averages in a way but
you're not assigning some sort of
hierarchal value or statistical value to
it in the sense that it will inform or
influence some sort of Central Point and
so that was that's kind of what I wanted
to clear up and clarify here um so
that's all there is for measures of
central tendency so you are going to be
thinking about these things as you move
forward
Ver Más Videos Relacionados

Mean, Median and Mode in Statistics | Statistics Tutorial | MarinStatsLectures
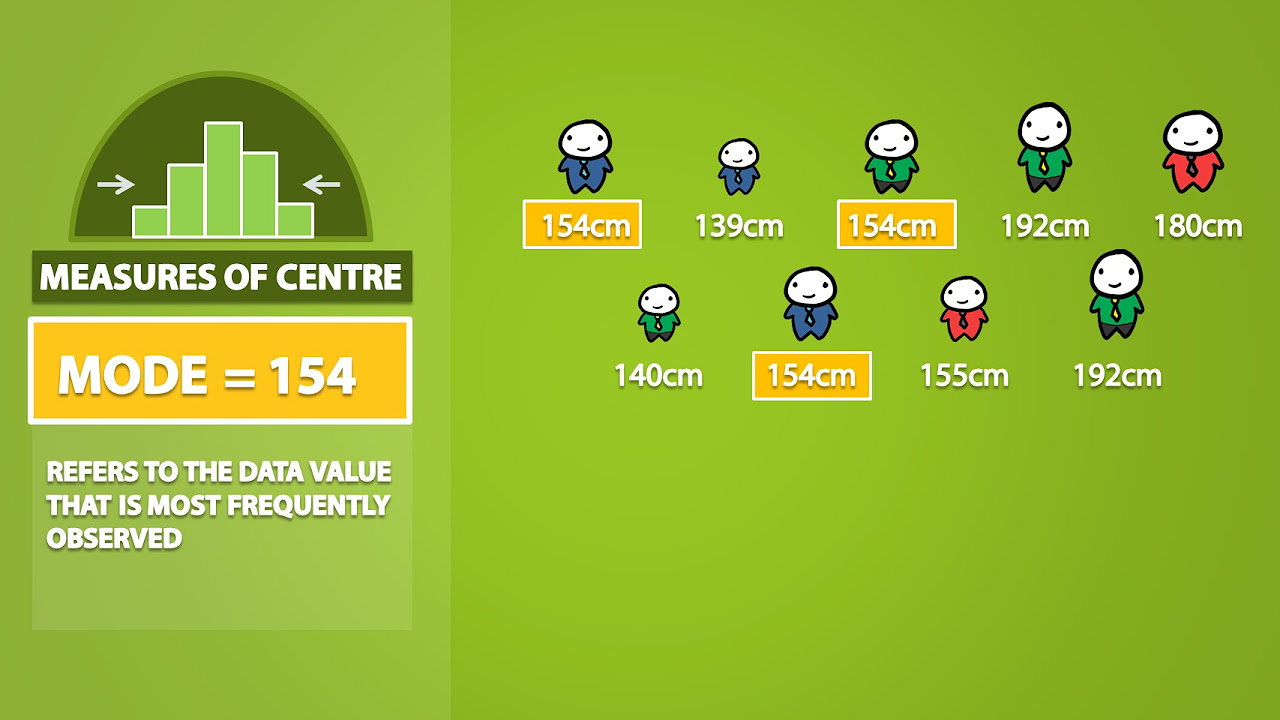
Mode, Median, Mean, Range, and Standard Deviation (1.3)
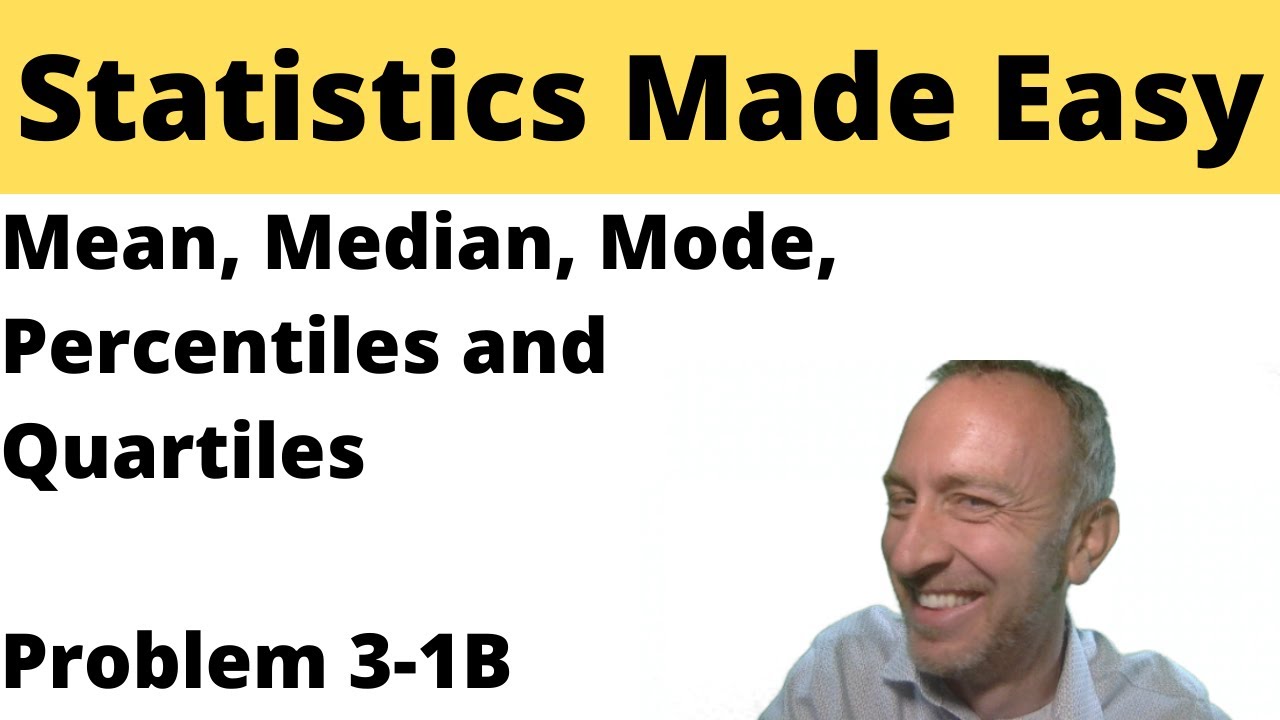
Statistics - Module 3 - Mean, Median, Mode, Percentiles and Quartiles - Problem 3-1B

MAT 152 SAS 8 Video

Tutorial 4- Measure Of Central Tendency- Mean, Median And Mode In Hindi
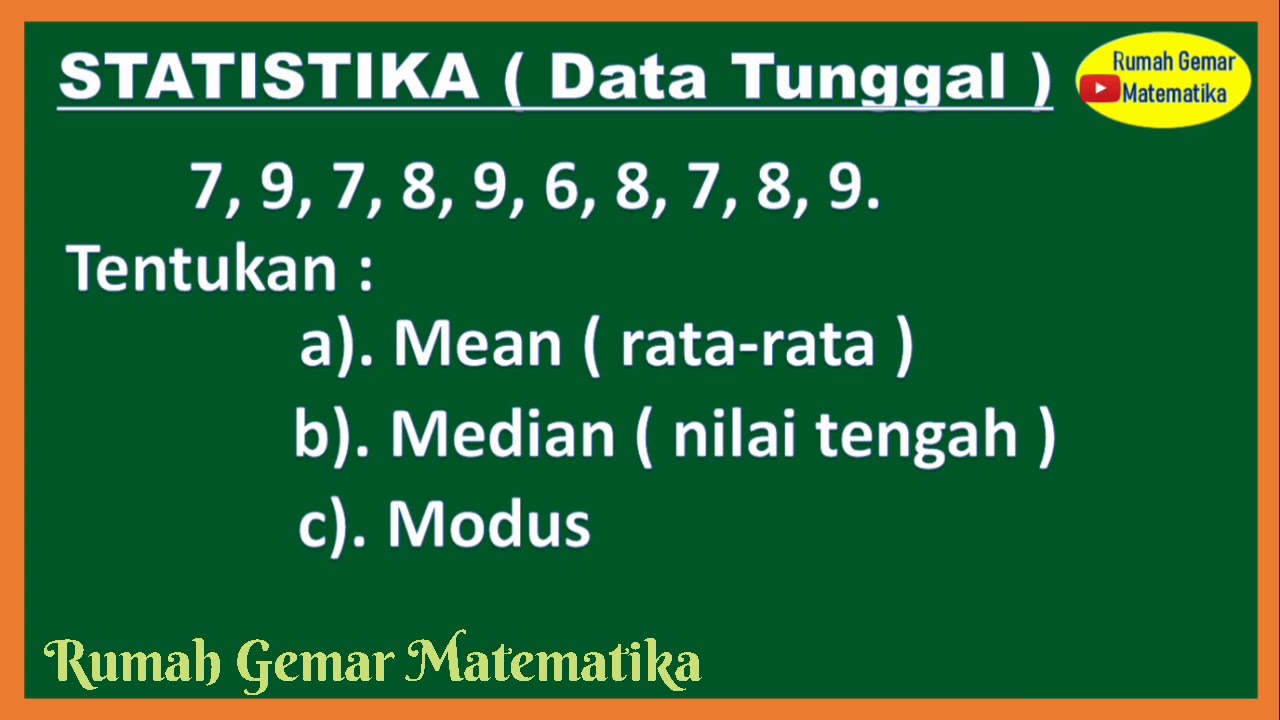
CARA MENENTUKAN MEAN MEDIAN MODUS DATA TUNGGAL||STATISTIKA
5.0 / 5 (0 votes)