03. Cómo describir una variable numérica | Curso de SPSS
Summary
TLDRThis script discusses key statistical measures for summarizing numerical data. It covers measures of central tendency (mean, median, mode), dispersion (standard deviation, variance, and standard error), position (percentiles, quartiles, deciles), and shape (skewness, kurtosis). The script uses examples like age, weight, and height to explain these concepts, highlighting how they help understand data distribution and variability.
Takeaways
- 📊 Describing a numerical variable involves summarizing it through various measures: central tendency, dispersion, position, and shape.
- 🔢 Central tendency measures include the mean (average), median (middle value), and mode (most frequent value). A normal distribution has the same mean, median, and mode.
- 🧮 Mean is calculated by summing all data points and dividing by their count. Median is the middle value when data is ordered. Mode is the most repeated value.
- 📉 Dispersion measures like standard deviation, variance, and standard error indicate how spread out the data is relative to the mean.
- 📏 Standard deviation shows data spread relative to the mean, variance is the square of standard deviation, and standard error is the standard deviation of the mean.
- 📋 Position measures such as percentiles, quartiles, and deciles divide data into 100, 4, and 10 equal parts, respectively, to understand data distribution.
- 📊 Quartiles (Q1, Q2, Q3) and percentiles (e.g., P23) are specific types of position measures that provide insights into data distribution.
- 📈 Shape measures like skewness and kurtosis are quantified by the skewness coefficient and kurtosis coefficient, indicating the symmetry and peakedness of the data distribution.
- 🔄 Positive skewness indicates a right tail, negative skewness indicates a left tail, and zero skewness suggests a symmetrical distribution.
- 📊 Kurtosis measures the 'tailedness' of the distribution; positive kurtosis indicates a leptokurtic distribution, negative indicates a platykurtic distribution, and zero suggests a mesokurtic distribution.
Q & A
What are the main types of measures used to summarize numerical variables?
-The main types of measures used to summarize numerical variables are measures of central tendency, measures of dispersion, measures of position, and measures of shape.
What are the three most common measures of central tendency?
-The three most common measures of central tendency are the mean (average), median, and mode.
How is the mean calculated and what is an example from the script?
-The mean is calculated by summing all the data points and dividing by the number of data points. An example from the script is the mean age of a group of people, which is 31 years.
What is the median and how does it relate to the data set's order?
-The median is the value that lies in the middle of a data set when it is ordered from least to greatest. In the script, the median age is 29 years.
What is the mode and what does it indicate about the data?
-The mode is the value that appears most frequently in a data set. It indicates the most common value among the data points. In the script, the mode of age is 27 years.
If the mean, median, and mode of a distribution are the same, what does this suggest about the distribution?
-If the mean, median, and mode are the same, it suggests that the distribution is normal.
What are the primary measures of dispersion and how do they differ?
-The primary measures of dispersion are the standard deviation, variance, and the standard error. The standard deviation measures how spread out the data is from the mean. Variance is the square of the standard deviation and is used in most statistical procedures. The standard error is a measure of how spread out the sample means are from the population mean.
What is the standard error of the mean and why is it important?
-The standard error of the mean is a measure of how much variability or 'spread' exists in the sample means over all possible samples. It is important because it provides an estimate of how close the sample mean is likely to be to the true population mean.
What are measures of position and how are they calculated?
-Measures of position include percentiles, quartiles, and deciles. They are calculated by dividing the data set into 100, 4, and 10 equal parts respectively, and identifying the values that correspond to these divisions.
What is the significance of the 23rd percentile mentioned in the script?
-The 23rd percentile mentioned in the script is an example of a measure of position that divides the data set into 100 parts and identifies the value that corresponds to the 23rd part.
What are measures of shape and how are they used to describe a distribution?
-Measures of shape include skewness and kurtosis. They are used to describe the shape of a distribution's tails. Skewness measures the asymmetry of the distribution, while kurtosis measures whether the distribution is peaked or flat relative to a normal distribution.
What does a positive skewness coefficient indicate about a distribution?
-A positive skewness coefficient indicates that the distribution has a longer tail on the right side, meaning there are more values on the higher end of the distribution.
What does a positive kurtosis value suggest about the distribution?
-A positive kurtosis value suggests that the distribution is leptokurtic, meaning it is more concentrated around the mean and has heavier tails compared to a normal distribution.
Outlines
📊 Descriptive Statistics Overview
This paragraph introduces the concept of descriptive statistics for numerical variables. It explains that numerical variables can be summarized using measures of central tendency, dispersion, position, and shape. The paragraph then delves into the specifics of each category, starting with measures of central tendency such as the mean (average), median (middle value), and mode (most frequent value). It provides an example using the variable 'age', where the mean age is 31 years, the median is 29 years, and the mode is 27 years. The paragraph also discusses measures of dispersion, including standard deviation, variance, and standard error, using 'weight' as an example. It mentions that standard deviation and variance are used to understand how spread out the data is from the mean, with standard deviation being in the same units as the original variable and standard error being an estimate of the variability of the mean.
📈 Position and Shape Measures in Statistics
The second paragraph continues the discussion on descriptive statistics by focusing on measures of position and shape. It explains the concept of percentiles, quartiles, and deciles as measures of position, which divide a dataset into equal parts to identify specific values at certain points. The paragraph gives an example using the variable 'height', where quartiles (Q1, Q2, Q3) and the 23rd percentile are calculated. Measures of shape, such as skewness and kurtosis, are also introduced. Skewness is measured by the coefficient of asymmetry, which indicates whether the data distribution is skewed to the right (positive) or left (negative). Kurtosis is measured by the coefficient of peakedness, which describes the shape of the distribution's tails. The paragraph concludes with an example using the variable 'body mass index', where the coefficient of asymmetry is positive (right skew) and the kurtosis is also positive, indicating a leptokurtic distribution.
Mindmap
Keywords
💡Central Tendency Measures
💡Dispersion Measures
💡Position Measures
💡Shape Measures
💡Mean
💡Median
💡Mode
💡Standard Deviation
💡Variance
💡Standard Error
💡Quartiles
Highlights
Numeric variables can be summarized using measures of central tendency, dispersion, position, and shape.
Central tendency measures include the mean, median, and mode.
The mean is calculated by summing all data points and dividing by the number of data points.
The median is the middle value when all data points are ordered.
The mode is the value that appears most frequently in the data set.
A normal distribution has the same mean, median, and mode.
Dispersion measures include standard deviation, variance, and standard error.
Standard deviation indicates how spread out the data is relative to the mean.
Variance is the square of standard deviation and is used in most statistical procedures.
Standard error is a measure of how spread out the sample mean is from the population mean.
Position measures include percentiles, quartiles, and deciles.
Percentiles divide the data into 100 parts, quartiles into four, and deciles into ten.
Shape measures include skewness and kurtosis, which are measured by skewness and kurtosis coefficients.
Skewness is positive for right-skewed data and negative for left-skewed data.
Kurtosis is positive for leptokurtic distributions, negative for platykurtic distributions, and zero for mesokurtic distributions.
The transcript provides a detailed analysis of descriptive statistics for variables such as age, weight, height, and body mass index.
The mean age of the group is 31 years, with a median of 29 years and a mode of 27 years.
The standard error for weight is 1.2, and the standard deviation is 9.33 kilograms.
Quartiles for height are the 25th, 50th, and 75th percentiles.
The body mass index has a positive skewness coefficient, indicating right-skewed data.
The body mass index also has a positive kurtosis coefficient, indicating a leptokurtic distribution.
Transcripts
Cómo describir una variable
numérica existe un sin número de medidas
con las que podemos resumir a una
variable numérica y las podemos agrupar
de la siguiente manera medidas de
tendencia central medidas de dispersión
medidas de posición y medidas de
forma dentro de cada uno de estos grupos
de medidas de resumen también hay
diferentes y numerosos indicadores para
resumir la información vamos a ver los
más importantes y los más
usados dentro de las medidas de
tendencia central tenemos a la media
mediana y
moda la media o media aritmética no es
más que el promedio que se obtiene de
sumar todos los datos y dividirlos entre
el número de ellos quién no ha sacado su
promedio de calificaciones para una
determinada asignatura esto es la
media la mediana corresponde al valor
central al valor que se encuentra en el
medio de haber ordenado a todos los
elementos que conforman el grupo y la
moda no es más que el valor que más se
repite si distribución tiene la media
mediana y moda con el mismo valor
Entonces se trata de una
distribución normal Cómo se calculan
estos tres medidas de resumen vamos a
analizar estadísticos descriptivos
frecuencias y para nuestra primera
variable numérica llamada edad en
estadísticos media mediana y moda
continuar y aceptar el número medio o el
valor promedio de edad de este grupo de
personas es 31 años una mediana de 29
años y una moda de 27 No necesariamente
coinciden en este
caso las medidas de dispersión por
excelencia son la desviación estándar la
varianza y el error
típico la desviación estándar nos indica
cuán dispersos están los datos respecto
de su valor central o respecto de la
media en cambio la varianza es el
cuadrado de la desviación estándar y
para la mayoría de los procedimientos
estadísticos se trabaja con la varianza
y no con la desviación estándar otra
diferencia es que la desviación estándar
tiene las unidades de la variable
original y el error típico es una
especie de desviación estándar pero no
para los datos sino más bien para la
media por eso se le conoce también como
error típico de la media y cómo se
calculan vamos a analizar estadísticos
descriptivos frecuencias retiramos la
variable edad y ahora coloquemos la
segunda variable peso vamos a
deseleccionar las tablas de frecuencias
porque se trata de una variable numérica
y en estadísticos ya no queremos las
medidas de tendencia central sino las de
dispersión desviación típica o estándar
que son sinónimos la varianza y el error
típico de la media continuar y aceptar y
ya tenemos que el error típico es 1,2
para la variable
peso Tenemos también la desviación
típica o estándar que es
9,33 en las unidades de la variable
original en este caso
kilogramos y la varianza que no es más
que el cuadrado de la desviación
estándar las medidas de posición son los
percentiles cuartiles y
desiles son percentiles si dividimos a
todo el grupo en 100 partes Entonces
tenemos 99 cortes son cuartiles si
dividimos a todo el grupo en cuatro
partes Entonces tenemos tres Cortes y
son deciles si dividimos a todo el grupo
en 10 partes Entonces tenemos nueve
cortes vamos a la matriz de datos
analizar estadísticos descriptivos
frecuencias retiramos el peso y
colocamos esta vez la talla y en
estadísticos vamos a colocar los
cuartiles q1 q2 y q3 podemos pedir los
los deciles para dividir en 10 grupos
iguales y también podríamos seleccionar
los percentiles de cualquier magnitud
por ejemplo el percentil
23 Vamos a continuar y aceptar y tenemos
Entonces los desiles tenemos 1 2
3 3 4 5 6 7 8 y 9 los cuartiles son el
percentil 25 el 50 y el 75 y también
habíamos solicitado el percentil 23 que
lo tenemos
aquí finalmente tenemos las medidas de
forma y Estos son la asimetría y la
curtosis se miden con el coeficiente de
asimetría de pirum y con el coeficiente
de apuntamiento o curtosis
respectivamente en la asimetría el valor
del coeficiente es positivo si la
asimetría es derecha o la cola Está
hacia la derecha el valor es Negativo si
la cola Está hacia la izquierda y se
denomina asimetría izquierda ahora si el
valor es cer0 Entonces es una curva
simétrica en cuanto a la curtosis si el
valor es positivo entonces es una
campana muy concentrada o leptocúrtica
si es que es muy aplanada muy dispersa
es platicúrtica y el valor del
coeficiente es negativo ahora si el
coeficiente es cero se trata de una
campana ni muy elevada ni muy aplanada
es decir mesocúrtica
en la matriz de datos vamos a analizar
estadísticos descriptivos frecuencias
retiramos la talla y nuestra última
variable numérica índice de masa
corporal en estadísticos vamos a
seleccionar asimetría y curtosis
continuar y aceptar y ya tenemos que el
coeficiente de asimetría es positivo es
decir asimetría derecha y la curtosis
también tiene un valor positivo es decir
se trata de una distribución
leptocúrtica
Ver Más Videos Relacionados
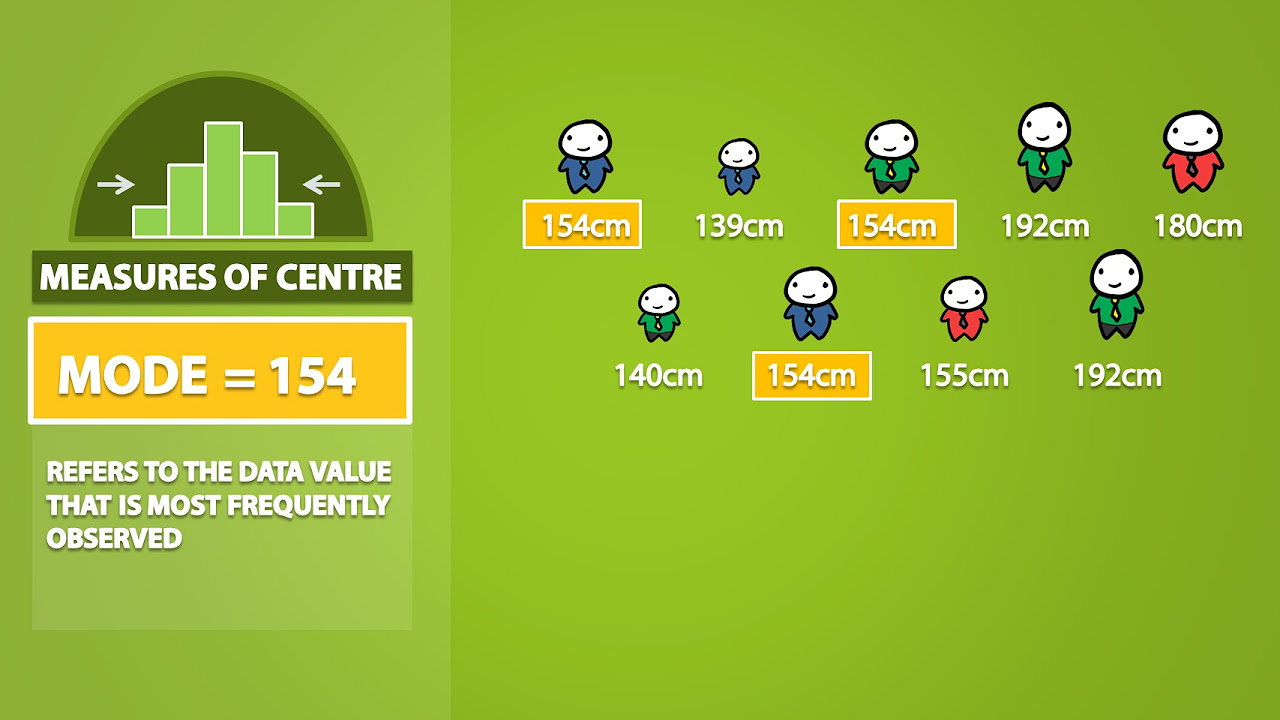
Mode, Median, Mean, Range, and Standard Deviation (1.3)

STATISTIK DESKRIPTIF (MEAN, MEDIAN, MODE, KUARTIL, VARIAN, STANDAR DEVIASI) UNTUK DATA TUNGGAL
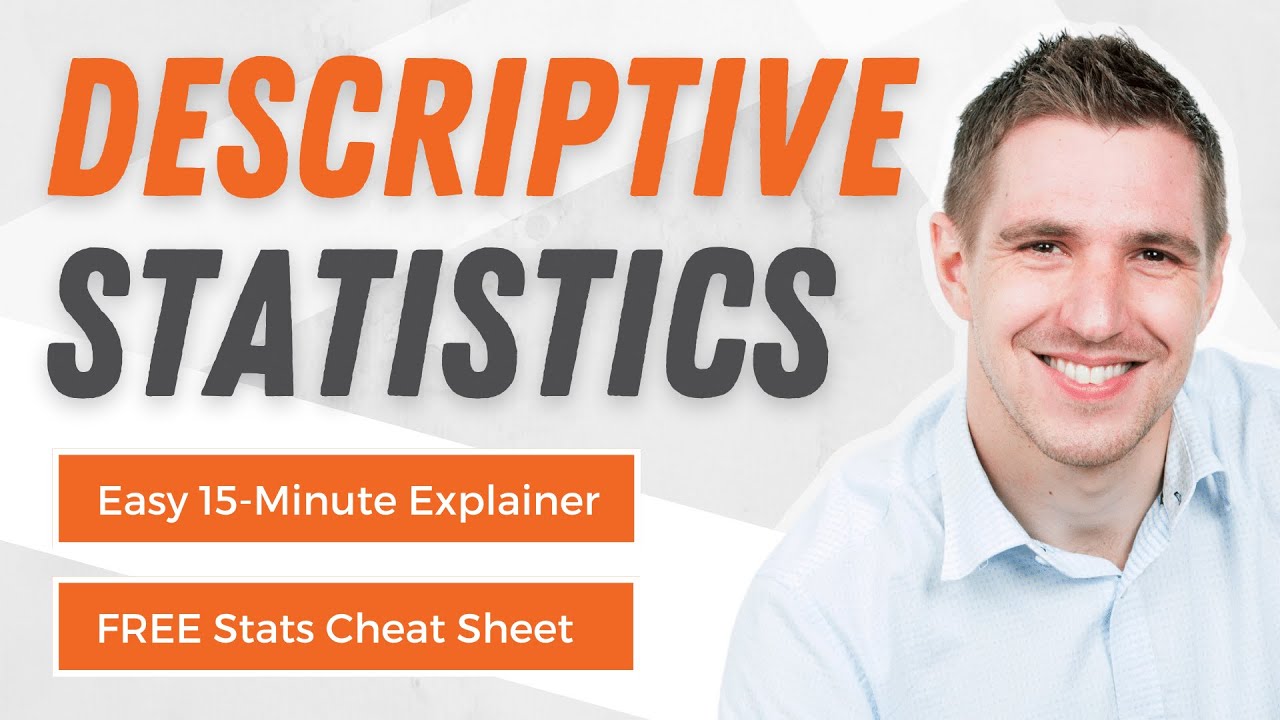
Descriptive Statistics: FULL Tutorial - Mean, Median, Mode, Variance & SD (With Examples)

Statistics - Module 3 - Numerical Summaries
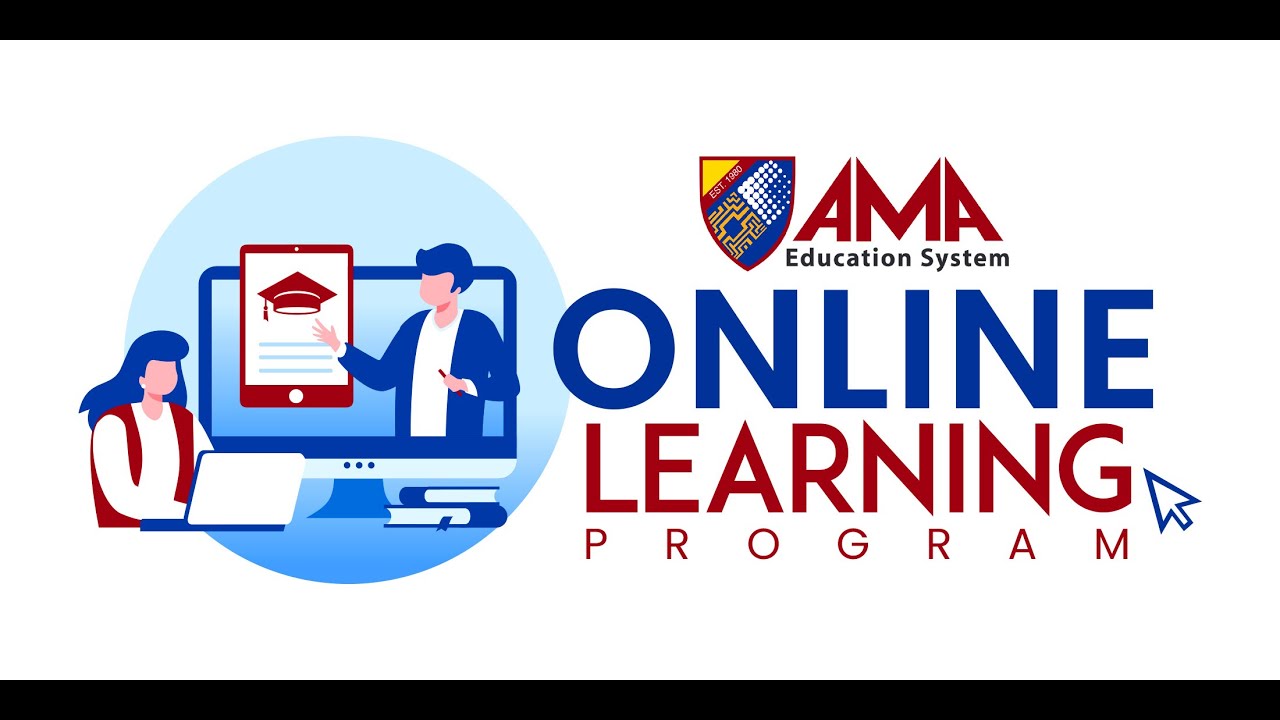
Mathematics in the Modern World - Data Management (Part 1)

Tutorial 4- Measure Of Central Tendency- Mean, Median And Mode In Hindi
5.0 / 5 (0 votes)